Transcriptomic analysis and laboratory experiments reveal potential critical genes and regulatory mechanisms in sepsis-associated acute kidney injury
Introduction
Sepsis is defined as a life-threatening organ dysfunction that results from the host’s dysregulated response to infection (1). The kidney is one of the most common organs affected by sepsis, and it results in sepsis-associated acute kidney injury (SA-AKI), which poses a great challenge for clinicians (2,3). In critically ill patients with sepsis, acute kidney injury (AKI) is one of the most frequent and serious complications. It occurs in approximately 35–50% of patients with sepsis, and the mortality rate is as high as 35% (4,5). AKI increases the potential risk of chronic kidney disease, and surviving patients inevitably develop end-stage renal disease, which threatens the life and health of all humans (6,7). Therefore, it is vital to determine the pathophysiological mechanism of SA-AKI, and research on clinical therapeutic strategies for the treatment of SA-AKI is beneficial.
Transcriptomic sequencing analyses have been widely applied in a variety of diseases, including cancer, immune system diseases and infectious diseases, to seek novel pathways to improve diagnosis and treatment (8-10). Competitive endogenous RNA (ceRNA) networks are widely used to clarify new binding mechanisms between messenger RNAs (mRNAs), microRNAs (miRNAs), circular RNAs (circRNAs) and long noncoding RNAs (lncRNAs) to promote disease development at the transcriptome level (11,12). Bioinformatics analyses and related experimental verification using molecular biology analyses may help reveal potential key genes and critical pathways that contribute to the occurrence and progression of diseases (13). However, few studies focused on the bioinformatic analysis of SA-AKI. Tang (14) identified seven co-differentially expressed genes (DEGs) of septic shock and AKI, including VMP1, SLPI, PTX3, TIMP1, OLFM4, LCN2 and S100A9, based on gene expression datasets of the Gene Expression Omnibus (GEO), but their gene expression datasets were obtained from peripheral blood samples of humans, not directly from kidney samples of sepsis. Yang et al. (15) revealed the gene expression profile of SA-AKI by identifying prognosis-related genes, transcription factors, miRNAs and pathways. However, the hub genes among the DEGs were not identified using PPIs.
N6-adenosine methylation (m6A) is the most common posttranscriptional dynamic and reversible modification in eukaryotic mRNAs, and it constitutes approximately 0.4% of all adenosine nucleotides in RNAs (16). Three types of factors regulate m6A mRNA methylation: methyltransferases (writers, such as METTL3, METTL14 and WTAP), which install the methyl group on adenosine; demethylases (erasers, such as FTO and ALKHB5), which remove the methyl group; and binding proteins (readers, such as YTHDF, IGF2BPs and eIF3), which decode and interact with m6A modification (17).
m6A RNA methylation-related regulators influence mRNA fate decisions because they change local structure, affect the exportation of m6A-modified mRNA transcripts, facilitate mRNA translation and protein synthesis, and trigger the de-adenylation and degradation of transcripts (18). Moreover, m6A modifications are found in noncoding RNAs, such as lncRNAs, miRNAs and snRNAs, affect RNA-protein and RNA-RNA interactions or chromatin remodeling (19). LncRNAs also interact with m6A regulators to affect their function. It has been demonstrated that m6A RNA methylation plays important roles in a variety of biological processes, including cell differentiation, embryonic development, cell cycle regulation, circadian cycle, and stress responses (20,21). Growing evidence from in vivo and in vitro experiments and bioinformatic studies suggest that m6A RNA methylation regulators are closely related to the occurrence, developmental pathogenesis, progression and clinical prognosis of various cancers and cardiovascular diseases (22,23). However, the role of m6A RNA methylation regulators in SA-AKI and their associations with SA-AKI genes were rarely studied.
The current study sequenced the RNAs of kidneys from a sepsis model group and control group mice, identified the DEGs and hub genes, and constructed co-expression and ceRNA networks. We verified the predicted results using laboratory-based experiments. We studied and validated key m6A RNA methylation regulators in sepsis and revealed a significant correlation between m6A RNA methylation regulators and DEGs. This study provides a novel perspective and lays the foundation to clarify the underlying pathophysiological mechanisms of SA-AKI. We present the following article in accordance with the ARRIVE reporting checklist (available at https://atm.amegroups.com/article/view/10.21037/atm-22-845/rc).
Methods
Animals and groups
C57BL/6 mice (male, 6–8 weeks old) were purchased from Guangdong Yaokang Biotechnology Co., Ltd. (China). We housed all mice in a standard environment, with a 12-h/12-h light-dark cycle, and the mice were free to eat and drink. According to a common sepsis protocol, we used the cecal ligation and puncture (CLP) method to construct a model of sepsis (24,25). For RNA sequencing, 5 mice were randomly divided into 2 groups: the CLP group (n=3) and the sham group (n=2). We anesthetized mice in the CLP group with 2–4% isoflurane. Under aseptic conditions, a 2-cm midline laparotomy was created below the diaphragm to expose the cecum. Two-thirds of the cecum was ligated with a 5-0 silk suture and punctured twice using a 22-gauge needle. The cecum was gently squeezed to extrude a small amount of feces through the perforation site. Animals were resuscitated with 1 mL of subcutaneous saline after CLP. The procedures of the sham group (controls) were the same as the CLP group, except for the ligation and perforation. The mice were sacrificed via neck fracture 6 hours after CLP, and the kidneys were taken for subsequent RNA sequencing. Six to eight weeks mice were used for quantitative real-time polymerase chain reaction (qPCR) (3 mice per group) and Western blotting (3 mice per group) verification, as described above, and the only difference was that the kidneys for Western blotting analysis were taken 24 hours after CLP/sham surgery. To minimize variability across experiments, the same researcher performed all of the CLP/sham surgeries, and the other researchers who collected specimens and performed the subsequent experiments and data analyses were blinded to the group allocation. Experiments were performed under a project license (No. AMUWEC20201509) granted by the Laboratory Animal Welfare and Ethics Committee of Third Military Medical University, in compliance with the Third Military Medical University institutional guidelines for the care and use of animals.
RNA isolation and library preparation
Total RNA from kidney samples [CLP group (n=3) and sham group (n=2)] was extracted using TRIzol (Thermo Scientific, USA) reagent according to the manufacturer’s protocol. RNA purity and quantification were evaluated using a NanoDrop 2000 spectrophotometer (Thermo Scientific, USA). RNA integrity was assessed using an Agilent 2100 Bioanalyzer (Agilent Technologies, Santa Clara, CA, USA). A total of 1 µg of RNA per sample was used as initial material for the RNA sample preparations. Ribosomal RNA was removed using a Ribo-off™ rRNA Depletion Kit (Human/Mouse/Rat) (Vazyme, China). The sequencing libraries were generated following the manufacturer’s recommendations with varied index labels using the NEBNext® Ultra™ Directional RNA Library Prep Kit for Illumina (NEB, USA).
RNA sequencing and differentially expressed gene analysis
The libraries were sequenced on an Illumina NovaSeq 6000 platform, and 150-bp paired-end reads were generated. Raw data (raw reads) in FASTQ format were processed using fastp (version 0.20.0) (26) software with default parameters in paired end mode. Clean data were obtained for downstream analyses by removing reads containing adapters, reads containing poly-N and low-quality reads from the raw data. The clean reads were mapped to references (GRCm38) of mouse mRNAs and lncRNAs using bowtie2 (version 2.3.1) (27) with the parameters −k30 −t −p20. FPKM values and read counts of each transcript were obtained by eXpress (version 1.5.1) with the parameters—no-update-check—rf-stranded. Differential expression analysis was performed using the DESeq R package (27). The functions estimateSizeFactors and nbinomTest were used to normalize the data and to calculate differential P values, respectively. A P value <0.05 and log2(fold change) >1 or <−1 were set as the thresholds for significantly differential expression. Hierarchical cluster analysis of DEGs was performed to explore gene expression patterns.
Heatmap and volcano plot analyses
To better visualize these DEGs, the R packages pheatmap and ggplot2 were used to draw heatmaps and volcano plots (28).
Enrichment analysis
Gene Ontology (GO) and Kyoto Encyclopedia of Genes and Genomes (KEGG) pathway enrichment analyses of DEGs were performed using R based on the hypergeometric distribution. To determine whether a gene set correlated with some phenotypic class distinction and assess the distribution trend of the genes of a predefined set, gene set enrichment analysis (GSEA) (29) software was used to analyze the gene expression profile at an overall level, and C5 (ontology gene sets) was chosen for functional enrichment analyses.
Construction of the PPI network
The protein-protein interaction (PPI) network was constructed based on DEGs using the online tool STRING (v11.5) (30). Considering the large number of DEGs, we further restricted the number of significant DEGs using stricter filter conditions: adjusted P value <0.01 and log2FoldChange >3 or <−3. Ultimately, 814 DEGs were included to construct the PPI network.
For better analysis and visualization, we downloaded the interaction data and optimized the PPI network using Cytoscape software (v3.8.2). The Minimal Common Oncology Data Elements (MCODE) plugin was used to identify significant gene clusters (parameter: Degree Cutoff =2; Node Score Cutoff =0.2; K-Core =2; Max. Depth =100). The CytoHubba plugin was used to identify hub genes in the significant gene clusters. We used two different algorithms, degree and maximal clique centrality (MCC), to calculate the top 30 hub genes. All of the results of MCODE and CytoHubba (degree and MCC) were intersected to obtain the final hub genes.
Prediction of target miRNAs
We used three online miRNA databases [TargetScan (31), miRWalk (32), miRDB (33)] to predict the target miRNAs of hub genes involved in KEGG pathways. The target miRNAs were selected by taking the intersections of the 3 databases. The mRNA-miRNA co-expression network was constructed using Cytoscape software (v3.8.2).
Construction of the ceRNA network
Predicted target lncRNAs were obtained from the DIANA tools (LncBase Predicted v.2), LNCediting (34) and lncRNASNP2 databases, and the first three predicted lncRNAs of each database were selected based on the score. A ceRNA network was constructed based on the interactions among mRNAs, miRNAs, and lncRNAs using Cytoscape software (v3.8.2).
Correlation analysis of m6A RNA methylation-related regulators and hub genes
We selected 26 m6A RNA methylation-related regulators based on published reviews (35,36), and we restricted the regulators to 23 genes that were detected in these high-throughput sequencing data.
The PPI network of 23 m6A RNA methylation-related regulators was analyzed using STRING (v11.5) (30). The correlation of m6A RNA methylation-related regulators and hub genes was analyzed using the “Corrplot” package of R software (confidence level =0.95).
The m6A modifications analysis
The m6A modification sites were obtained from the RMBase (v2.0) database (37), which was based on the reanalysis of m6A-seq and methylated RNA immunoprecipitation sequencing (MeRIP-Seq) raw data.
Cell culture
Mouse kidney tubular epithelium cells (TCMK-1) were obtained from BeNa Culture Collection (Henan, China). The cells were maintained in a cell incubator at 37 ℃ in a 5% CO2 humidified atmosphere in DMEM/F12 medium (Gibco; Thermo Fisher Scientific, Inc., MA, USA) supplemented with 10% fetal bovine serum (Gibco; Thermo Fisher Scientific, Inc., CA, USA) and 1% penicillin/streptomycin.
Cell treatments and transfection
TCMK-1 cells were stimulated with lipopolysaccharide (LPS, L2630, Sigma-Aldrich, Darmstadt, Germany) to mimic sepsis in vitro. The mimics and inhibitors of miR-7212-5p were synthesized by RiboBio (Guangzhou, China). The cells were divided into six groups: (I) control; (II) miR-7212-5p mimic; (III) miR-7212-5p inhibitor; (IV) Control + LPS; (V) miR-7212-5p mimic + LPS; and (VI) miR-7212-5p inhibitor + LPS. Before transfection, TCMK-1 cells were plated in a 6-well plate at 1×106 cells/well, antibiotic-free medium was added, and the cells were 50–60% confluent after 24 h. The cell culture medium was changed to serum-free DMEM/F12 medium to prepare for transfection. The transfection process was performed according to the instructions of the riboFECTTMCP Transfection Kit (RiboBio, China). The cells in the miR-7212-5p mimic and miR-7212-5p mimic + LPS groups were transfected with 50 nM of a miR-7212-5p mimic using a riboFECTTMCP Transfection Kit and cultured for 48 h. The cells in the miR-7212-5p inhibitor and miR-7212-5p inhibitor + LPS groups were transfected with 100 nM of miR-7212-5p inhibitor using a riboFECTTMCP Transfection Kit and cultured for 48 h, following the manufacturer’s instructions. After transfection for 48 h, the cells in the Control + LPS, miR-7212-5p mimic + LPS and miR-7212-5p inhibitor + LPS groups were stimulated with 100 µg/mL LPS for 6 h (qPCR) or 24 h (Western blotting).
RNA extraction and qPCR
Total RNA was extracted from the kidney specimens, and the cells were collected using TRIzol reagent (Thermo Fisher Scientific, USA) following the manufacturer’s instructions. The purity and quality of the total RNA were determined using a Nano Drop 1000 spectrophotometer (Thermo Fisher Scientific, USA). Briefly, 1,000 ng RNA derived from kidney tissues or TCMK-1 cells was reverse transcribed into cDNA using a PrimeScript TM RT reagent Kit (Takara, Japan) according to the manufacturer’s protocol. The qRT-PCR was performed using the CFX96 real-time PCR system (Bio-Rad, CA, USA) with SYBR Premix Ex Taq TM II (Takara, Japan). Glyceraldehyde 3-phosphate dehydrogenase (GAPDH) served as an internal control for real-time PCR. PCR primers were designed and synthesized by Tsingke Biological Technology (Beijing, China). The relative expression of the genes in each experimental group was analyzed using the 2−∆∆Ct method. The sequences of the primers used in the qPCR experiments are listed in Table 1. All experiments were performed in triplicate.
Table 1
Resource | Gene | Sequence (5'→3') |
---|---|---|
Mouse | Gaphd | |
Forward | TGGAAAGCTGTGGCGTGAT | |
Reverse | TGCTTCACCACCTTCTTGAT | |
Mouse | Hmox1 | |
Forward | CGCCTTCCTGCTCAACAT | |
Reverse | ACGAAGTGACGCCATCTG | |
Mouse | Socs3 | |
Forward | TGCGCCTCAAGACCTTCAG | |
Reverse | GCTCCAGTAGAATCCGCTCTC | |
Mouse | Cxcl12 | |
Forward | GCTCTGCATCAGTGACGGTA | |
Reverse | TAATTTCGGGTCAATGCACA | |
Mouse | Cxcl1 | |
Forward | ACCGAAGTCATAGCCACACTC | |
Reverse | CTCCGTTACTTGGGGACACC | |
Mouse | Mapk14 | |
Forward | GGACTGTGAGCTGAAGATCCTA | |
Reverse | CGCATCCAATTCAAGATGACCT | |
Mouse | Spp1 | |
Forward | AAGAGCGGTGAGTCTAAGGAGTCC | |
Reverse | TGGCTGCCCTTTCCGTTGTTG | |
Mouse | Lcn2 | |
Forward | ACCACGGACTACAACCAGTTCGCC | |
Reverse | ACTTGGCAAAGCGGGTGAAACG | |
Mouse | Acsl4 | |
Forward | CTCACCATTATATTGCTGCCTGT | |
Reverse | TCTCTTTGCCATAGCGTTTTTCT | |
Mouse | iNOS | |
Forward | TTGACGCTCGGAACTGTA | |
Reverse | ACCTGATGTTGCCATTGTT | |
Mouse | IL-6 | |
Forward | TAGTCCTTCCTACCCCAATTTCC | |
Reverse | TTGGTCCTTAGCCACTCCTTC | |
Mouse | mmu-miR-7212-5p mimic | |
Sense | UCUGGGGGCUUGUGUGGUAGG | |
Mouse | mmu-miR-7212-5p inhibitor | |
Antisense | CCUACCACACAAGCCCCCAGA | |
Sense | CCUACCACACAAGCCCCCAGA | |
Mouse | mmu-miR-NC | |
Sense | CAGUACUUUUGUGUAGUACAAA |
qPCR, quantitative real-time polymerase chain reaction; miRNA, microRNA.
Western blotting analysis
Frozen kidney tissue specimens from 6 mice (3 CLP mice, 3 sham mice) were weighed and cut into small pieces. Kidney single-cell suspensions were obtained by grinding followed by filtration through a 70-µm cell strainer. The pellets were treated with red blood cell lysis buffer (Beyotime Biotechnology Co., Ltd., Jiangsu, China) and washed twice with phosphate-buffered saline (PBS, HyClone Laboratories Inc., UT, USA) to remove red blood cells. The kidney tissue pellets or the TCMK-1 cell samples were added to protein lysis buffer with protease inhibitors. The samples were centrifuged at 12,000 ×g for 30 minutes at 4 ℃, and the supernatant was collected. The protein concentrations were measured using a BCA Protein Assay Kit (Beyotime Biotechnology Co., Ltd., Jiangsu, China). Equal amounts of total protein were separated using 10% Tris-Glycine extended stain-free polyacrylamide gels (Bio-Rad, CA, USA), and the proteins were transferred to polyvinylidene difluoride (PVDF) membranes (Roche, USA) using a wet blotting apparatus (Bio-Rad, CA, USA). The cells were blocked with 5% fat-free milk for 2 h at room temperature. The membranes were incubated with the following primary antibodies overnight at 4 ℃: anti-METTL3 antibody (1:2,000, Arigobio, ARG56406), anti-ACSL4 (1:20,000, Abcam, ab155282), anti-ALKBH5 antibody (1:2,000, Abcam, ab69325), and anti-Hmox1 (1:1,000, Proteintech, 10701-1-AP). The cells were incubated with secondary antibodies (anti-rabbit IgG, 1:3,000, Cell Signaling Technology, 7074S; goat anti-mouse IgG, 1:10,000, ZSGB-BIO, 117228) at room temperature for 1 h. The blots were washed with TBST and visualized by enhanced chemiluminescence using a luminescent imaging workstation. The amount of protein was proportional to the optical density (OD) intensity, which was measured using ImageJ software (National Institutes of Health, MD, USA). Total protein was measured using a ChemiDoc Touch Imaging System (Bio-Rad, CA, USA) and used as a loading control. All the reactions were repeated 3 times.
ROS assay
TCMK-1 cells were divided into six groups: (I) Control; (II) miR-NC; (III) miR-7212-5p inhibitor; (IV) Control + LPS; (V) miR-NC + LPS; and (VI) miR-7212-5p inhibitor + LPS. After transfection for 48 h, the cells in the Control + LPS, miR-NC + LPS and miR-7212-5p inhibitor + LPS groups were stimulated with 100 µg/mL LPS for 24 h. Intracellular ROS levels were estimated using flow cytometry and an ROS assay kit (S0033S, Beyotime Biotechnology Co., Ltd., Jiangsu, China) according to the manufacturer’s protocol. Briefly, TCMK-1 cells were incubated with the fluorescent probe DCFH-DA. The cells were analyzed using flow cytometry with an excitation wavelength of 488 nm and an emission wavelength of 525 nm. The ROS levels were measured using the mean fluorescence intensity with FlowJo software (version 10.7, BD Biosciences, NJ, USA).
Determination of malondialdehyde (MDA) level
Grouping and treatment were the same as the ROS assay. The level of MDA was determined using a colorimetric method and an MDA content assay kit (Solarbio Life Sciences, BC0025, Beijing, China) according to the manufacturer’s instructions.
Statistical analysis
SPSS 25.0 software (IBM Corp., Armonk, NY, USA) was used for statistical analyses. Normally distributed variables were assessed using Student’s t-test to compare the differences between the two groups, and nonnormally distributed variables were detected using the nonparametric Mann-Whitney U test. Statistical significance was set at a P value of <0.05.
Results
Identification of DEGs for enrichment analysis
As shown in the experimental flow chart (Figure 1A), five kidney RNA samples [CLP group (n=3), sham group (n=2)] were successfully sequenced. We identified a total of 4,754 DEGs in the CLP samples, including 2,322 upregulated genes and 2,432 downregulated genes. Heatmap and volcano plot analyses were used to visualize these DEGs, which are shown in Figure 1B,1C.
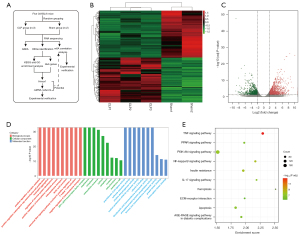
The DEGs were subjected to GO and KEGG pathway enrichment analyses, and C5 gene sets were used for GSEA to analyze the gene expression profile at an overall level. As shown in Figure 1D, GO enrichment analysis of DEGs revealed that the immune response in CLP samples was stronger than the sham samples. For example, biological processes were significantly enriched in neutrophil aggregation and T-helper 1-cell activation. For the molecular function enrichment, we noted that ferrous iron transmembrane transporter activity was enriched. As shown in Figure 1E, KEGG pathway enrichment analysis revealed that DEGs were enriched in ferroptosis (the highest enrichment score), the IL-17 signaling pathway, apoptosis, the PI3K-Akt signaling pathway, and the NF-kappa B signaling pathway. The GSEA results showed that 189 gene sets were significant at a false discovery rate (FDR) <25%, and most of the enriched gene sets were related to various immune responses. Figure 2 shows the 9 most enriched immune-related gene sets based on the normalized enrichment score (NES). In summary, GSEA revealed that the enriched gene sets were related to the activation or regulation of immune cells, the cytoplasmic patterning recognition receptor signaling pathway, and the type I interferon signaling pathway, such as the gene sets of the positive regulation of mast cell activation, natural killer cell activation involved in the immune response, and the positive regulation of myeloid leukocyte-mediated immunity.
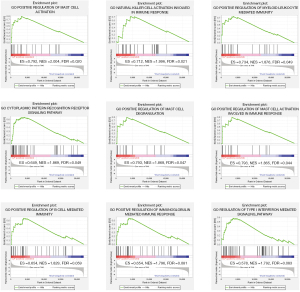
PPI network analysis for identifying hub genes and the prediction of target miRNAs for constructing the co-expression network
As shown in Figure 3A, the PPI network based on DEGs consisted of 242 nodes and 413 edges. The MCODE plugin was used to identify gene cluster modules, and the results were intersected using cytoHubba (degree and MCC algorithms) to identify hub genes. Fifteen hub genes were identified and are shown in Figure 3B. These 15 genes are the key genes in the PPI network and may play important roles in the pathological process of sepsis (Table 2). We observed that 7 of the 15 hub genes were involved in the KEGG pathway, including Hmox1 in ferroptosis, Lcn2 in the IL-17 signaling pathway, Cxcl1 and Cxcl12 in the NF-kappa B signaling pathway, Mapk14 in the AGE-RAGE signaling pathway in diabetic complications, Socs3 in the TNF signaling pathway, and Spp1 in the PI3K-Akt signaling pathway. A total of 183 target miRNAs were predicted based on the 7 hub genes (Hmox1, Spp1, Socs3, Mapk14, Lcn2, Cxcl1 and Cxcl12), which were involved in the KEGG pathway. Based on the prediction results, a co-expression network of miRNAs and mRNAs was constructed with 190 nodes and 201 edges, as shown in Figure 3C.
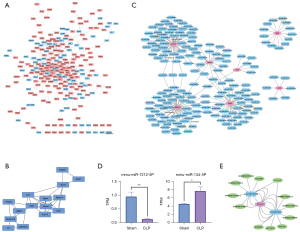
Table 2
Gene symbol | Description | log2FC | Adjusted P value | Regulation |
---|---|---|---|---|
Hmox1 | Heme oxygenase 1 | 4.761 | 2.753e-05 | Up |
Cxcl1 | Chemokine (C-X-C motif) ligand 1 | 5.616 | 3.556e-04 | Up |
Cxcl12 | Chemokine (C-X-C motif) ligand 12 | −3.238 | 5.987e-08 | Down |
Igfbp5 | Insulin-like growth factor binding protein 5 | −3.481 | 2.121e-03 | Down |
Itgam | Integrin alpha M | 4.758 | 5.308e-05 | Up |
Klhl21 | Kelch-like 21 | 4.311 | 1.141e-10 | Up |
F8 | Coagulation factor VIII | −3.626 | 7.876e-07 | Down |
Lcn2 | Lipocalin 2 | 6.298 | 1.599e-10 | Up |
Mapk14 | Mitogen-activated protein kinase 14 | 4.988 | 1.030e-06 | Up |
Nedd4l | Neural precursor cell expressed, developmentally down-regulated gene 4-like | 3.150 | 5.767e-07 | Up |
Pnpla2 | Patatin-like phospholipase domain containing 2 | 4.134 | 4.713e-04 | Up |
Serpina1a | Serine (or cysteine) peptidase inhibitor | 7.563 | 3.742e-25 | Up |
Serpina3n | Serine (or cysteine) peptidase inhibitor, clade A, member 3N | 4.963 | 9.239e-07 | Up |
Socs3 | Suppressor of cytokine signaling 3 | 4.914 | 2.297e-09 | Up |
Spp1 | Secreted phosphoprotein 1 | 3.503 | 6.272e-07 | Up |
FC, fold change.
Because Hmox1 was the hub gene of ferroptosis, which showed the highest enrichment score from KEGG pathway enrichment analysis, 22 target miRNAs for Hmox1 were predicted, and the miRNA sequencing results further verified the expression difference between the CLP and sham groups. There were 2 miRNAs, mmu-miR-7212-5p and mmu-miR-134-5p, which were detected using our miRNA sequencing, and the difference in transcripts per million (TPM) between these 2 groups was statistically significant (P<0.05) (Figure 3D).
Thirteen target lncRNAs were predicted based on the differentially expressed miRNAs (mmu-miR-7212-5p and mmu-miR-134-5p) and used to construct ceRNA networks of the Hmox1 gene (Figure 3E).
Verification of the 7 specifically expressed hub genes and the miRNA-Hmox1 regulatory axis
To verify the expression differences of the predicted 7 hub genes involved in the KEGG pathway, we performed qPCR analysis of mouse kidneys between the CLP and sham groups. We noticed that the mRNA expression levels of the 6 genes (Hmox1, Spp1, Socs3, Lcn2, Cxcl1 and Cxcl12) in the 7 expressed hub genes were consistent with the RNA sequencing results (Figure 4A).
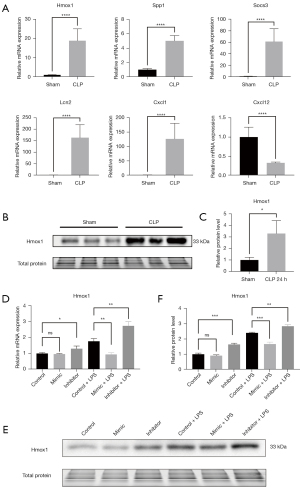
To further verify the hub gene of Hmox1 in ferroptosis, we performed Western blotting (Figure 4B) to assess the protein expression levels between the CLP and sham groups. Consistent with the qPCR and RNA sequencing results, the expression of Hmox1 in CLP kidneys was higher than the sham kidneys (Figure 4C).
The mmu-miR-7212-5p-Hmox1 regulatory axis was also verified. We noted that in the mmu-miR-7212-5p-Hmox1 regulatory axis, the mimic of mmu-miR-7212-5p reduced the mRNA (Figure 4D) and protein expression (Figure 4E,4F) of Hmox1 in TCMK-1 cells stimulated with LPS, and its inhibitor increased the mRNA (Figure 4D) and protein expression (Figure 4E,4F) of Hmox1 in TCMK-1 cells stimulated with or without LPS. These results suggest that mmu-miR-7212-5p-Hmox1 may be a key RNA regulatory axis that participates in the ferroptosis pathway attributed to the pathophysiological process of SA-AKI.
Inhibitor of mmu-miR-7212-5p attenuates ferroptosis and inflammatory factor release in TCMK-1 cells stimulated with LPS
A previous study demonstrated that Hmox1 played an anti-ferroptotic role in renal epithelial cells. We wondered whether an inhibitor of mmu-miR-7212-5p would attenuate ferroptosis in TCMK-1 cells stimulated with LPS. Acsl4 is an enzyme that converts fatty acids to fatty acyl-CoA, and it is considered a ferroptosis marker (38). We found that the LPS-induced enhanced mRNA (Figure 5A) and protein expression (Figure 5B,5C) of Acsl4 in TCMK-1 cells would be attenuated by the mmu-miR-7212-5p inhibitor. Flow cytometry results showed that the enhanced ROS level (mean fluorescence intensity of DCFH-DA) in TCMK-1 cells induced by LPS was also attenuated by the mmu-miR-7212-5p inhibitor (Figure 5D). MDA is a lipid peroxidation byproduct that reflects the degree of ferroptosis in cells. The MDA content was reduced by the mmu-miR-7212-5p inhibitor after LPS challenge (Figure 5E). These data showed that the inhibitor of mmu-miR-7212-5p reduced LPS-induced ferroptosis. The mmu-miR-7212-5p inhibitor significantly reduced the mRNA expression of iNOS and IL-6, which indicates that it inhibits the release of LPS-induced renal inflammatory factors (Figure 5F,5G).
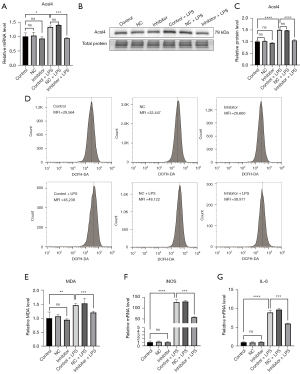
m6A RNA Methylation and related regulators involved in SA-AKI
High-throughput sequencing showed that the expression levels of Alkbh5, Igf2bp2 and Eif3a were significantly increased in the CLP groups compared to the controls (P<0.05), and the expression levels of Hnrnpa2b1, Igf2bp1, Ythdf3, Wtap and Mettl16 were significantly decreased (P<0.05) (Figure 6A). Wtap and Mettl16 are m6A “writers” in the RNA methylation process, Alkbh5 is an m6A “eraser”, and Igf2bp1, Igf2bp2, Eif3a, Hnrnpa2b1 and Ythdf3 are m6A “readers”.
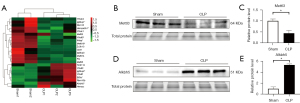
As methyltransferases, Mettl3 and demethylase Alkbh5 play core roles in regulating m6A methylation levels. To further investigate whether Mettl3 and Alkbh5 were involved in SA-AKI, Western blotting was performed to identify the expression levels of these proteins. As shown in Figure 6B-6E, the results demonstrated that the protein expression of Mettl3 was decreased in SA-AKI, and the expression of Alkbh5 was increased. From the data above, we inferred that the total m6A RNA methylation level may be decreased in SA-AKI.
Interaction of m6A RNA methylation-related regulators and CLP hub genes
The interactions of m6A RNA methylation-related regulators were analyzed (Figure 7A), and they were highly connected among writers, erasers and readers. Mettl3, Hnrnpa2b1, Hnrnpc and Ythdc2 were hub genes in the RNA methylation process because they interacted with at least 18 m6A RNA methylation-related regulators (Figure 7B). The expression levels of Mettl3, Mettl14, Mettl16, Zc3h13, Rbm15b, Fto, Alkbh5, Ythdf1, Ythdf2, Ythdf3, Igf2bp1, Igf2bp2 and Igf2bp3 significantly correlated with the expression levels of other m6A RNA methylation-related regulators (P<0.05) (Figure 7C).
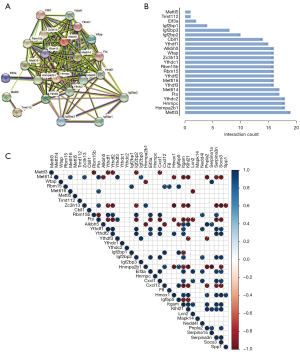
To further study the interaction of the m6A RNA methylation-related regulators and 15 hub genes in CLP, we analyzed the correlations between these factors. The correlation analysis of expression revealed that 14 hub genes in CLP were significantly associated with m6A regulators (Figure 7C). The hub gene Hmox1 positively correlated with the expression of Ythdf2 and negatively correlated with the expression of Mettl14, Fto, and Zc3yh13 (P<0.05).
The possible m6A modification sites for Hmox1 were further analyzed. There were at least 21 m6A modification sites, which were supported by at least one m6A-seq or MeRIP-Seq dataset, in the gene sequence of Hmox1 based on the RMBase database. ‘Motif score’ is an alignment score to evaluate the accuracy of identified motif regions of m6A, and the sites of the top 10 motif scores are listed in Table 3.
Table 3
Sequence |
CTCTCCTTAGCCCAGCTGGGACTTCTTTACTCTCCTCTTTG |
AAGGGCTGCCCTGGAGCAGGACATGGCCTTCTGGTATGGGC |
ATCGAGCAGAACCAGCCTGAACTAGCCCAGTCCGGTGATGG |
GAATGCTGAGTTCATGAAGAACTTTCAGAAGGGTCAGGTGT |
CTGGCCCCCAGGGGCTGTGAACTCTGTCCAATGTGGCCTTC |
ACATCCAGCCAGTGGCCTGAACTTTGAAACCAGCAGCCCCA |
GGCTTTTTTTACCTTCCCGAACATCGACAGCCCCACCAAGT |
GCTCTATCGTGCTCGAATGAACACTCTGGAGATGACACCTG |
CAGGTGATGCTGACAGAGGAACACAAAGACCAGAGTCCCTC |
AAATCAGAAATAGGGTACAGACAAAAGCGCCCAGGGTAAGC |
m6A, N6-adenosine methylation; Mod, modification; italic A, m6A modification site.
Discussion
Sepsis is a highly heterogeneous syndrome that is associated with a dysregulated systemic inflammatory host response to infection and results in organ dysfunction (39). AKI is a major and severe complication in septic patients, but no specific and effective therapeutic strategies are available to treat sepsis-related AKI (40). Therefore, exploring the mechanism of AKI in sepsis is critical for the prevention and treatment of sepsis.
The current study demonstrated that the biological process of GO enrichment analysis showed neutrophil aggregation and T-helper 1-cell activation, and GSEA indicated that most of the enriched gene sets were related to various immune responses, such as the positive regulation of mast cell activation, nature killer cell activation involved in the immune response and the positive regulation of myeloid leukocyte-mediated immunity. These results showed that AKI related to sepsis was accompanied by strong immune activation, which caused inflammation and injury of the renal corpuscle and renal tubule. The current study is the first SA-AKI study to show enriched immune-related pathways using GSEA instead of DEGs. GSEA, which focuses on gene sets, has some advantages compared to single-gene analysis methods (29). GSEA boosts the signal-to-noise ratio, which makes it possible to detect modest changes in individual genes and effectively reveal important immune-related pathways.
A total of 15 hub genes in the PPI network were obtained by the intersection of two different algorithms, and we noted that 7 (Hmox1, Lcn2, Cxcl1, Cxcl12, Mapk14, Socs3 and Spp1) of these 15 hub genes were involved in KEGG pathways. The upregulated Lcn2 in the 7 hub genes was also reported as a hub gene in a previous bioinformatic analysis study of SA-AKI (14), but the other 6 hub genes in the current study had not been reported. To increase the credibility of our bioinformatic analysis, we verified the expression differences of the 7 hub genes in vivo. The qPCR results were very consistent with the RNA sequencing results. The upregulated or downregulated expression of the 7 genes played key roles in different signaling pathways. We further constructed a co-expressed network of the 7 mRNAs and their target miRNAs. This network demonstrated the interaction mechanism by which the most key genes were regulated at the transcriptome level.
Our enrichment pathway results were generally consistent with a previous bioinformatics study (15), such as the apoptotic process and the responses to cytokine and immune system process pathways. However, we also noted that the ferroptosis pathway was enriched and had the highest enrichment score. The molecular function of GO enrichment analysis found that ferrous iron transmembrane transporter activity was enriched. Among these 7 hub genes, Hmox1 in the ferroptosis pathway was in the center of the hub gene PPI network, with 6 edges connected with other hub genes. The mRNA and protein expression of Hmox1 was upregulated in the kidneys of the CLP group. These results indicate that the ferroptosis pathway plays an important role in the pathophysiological events of the kidney in sepsis.
Ferroptosis is a novel iron-dependent cell death pathway that is characterized by increases in reactive oxygen species (ROS) and lipid peroxidation caused by iron overloading (41). Ferroptosis is the key mechanism in the pathogenesis of AKI, and the disruption of iron homeostasis is a significant contributor to tissue injury (42). Deferoxamine (DFO) inhibits lipid peroxidation and renal tubular epithelial cell necrosis to prevent renal failure (43).
Hmox1, namely, heme oxygenase-1 (HO-1), is a phase II enzyme that metabolizes heme into biliverdin/bilirubin and ferrous iron, and it triggers the generation of carbon monoxide and H-ferritin (42,44). To further elucidate the potential mechanism of Hmox1, the present study constructed a ceRNA network of the target lncRNAs and miRNAs for Hmox1. The Hmox1 gene was upregulated in the CLP group, and its target miRNA, mmu-miR-7212-5p, was downregulated in the CLP group. According to the miRNA hypothesis, we hypothesized that mmu-miR-7212-5p-Hmox1 was a key RNA regulatory pathway. To test this hypothesis, we verified the mmu-miR-7212-5p-Hmox1 regulatory axis. LPS stimulation led to a distinct compensatory elevation of Hmox1 expression in TCMK-1-cell lines, and a mmu-miR-7212-5p mimic attenuated LPS-induced enhanced Hmox1 expression but further increased by the inhibitor. However, without LPS stimulation, this phenomenon was only observed in the mmu-miR-7212-5p inhibitor, and the reduction in Hmox1 levels was not significant for the mimic. The reasons for this result may be that without LPS stimulation, the expression of Hmox1 is relatively low, and the expression of mmu-miR-7212-5p is relatively high. The expression level of Hmox1 could not be further decreased by exogenous additional mmu-miR-7212-5p mimic.
Ferroptosis is a pro-inflammatory agent that recruits macrophages and causes inflammation in AKI (45). Recent studies revealed that Hmox1 played an anti-ferroptotic role during AKI against oxidative stress and inflammation (43). By upregulating the Hmox1 pathway, LPS-induced acute lung injury was reduced, and lung pathological changes were ameliorated (46). We found that an inhibitor of mmu-miR-7212-5p increased the expression of Hmox1 and attenuated ferroptosis by reducing the expression of Acsl4. Acsl4 is a known promotor of ferroptosis that regulates lipid biosynthesis by converting fatty acids to fatty acyl-CoA esters. Ablation of the Acsl4 gene attenuates the pathological and functional injury of AKI mice (38). ROS formation is considered the executioner of ferroptosis. Excessive ROS cause oxidative stress and exacerbate mitochondrial dysfunction, which lead to renal injury directly (47). The increased ROS level activates the inflammatory response to remote organ injury (48). The LPS-induced ROS levels and inflammatory factor release in TCMK-1 cells were reduced as a result of attenuated ferroptosis. Taken together, our study indicates that the mmu-miR-7212-5p-Hmox1 axis participates in the pathophysiological process of sepsis-induced AKI induced. The inhibitor of mmu-miR-7212-5p may be a potential clinical therapeutic target for sepsis-related AKI.
m6A is one of the most ubiquitous and abundant mRNA methylation modifications that occur in eukaryotes (49), and it is closely related to the heterogeneity and prognosis of sepsis (50). There were 21 m6A modification sites in the sequence of the hub gene Hmox1, which indicated that Hmox1 may be modified by m6A RNA methylation. Meanwhile, it has been reported that mmu-miR-7212-5p in the predicted regulatory pathway may also be regulated by Mettl3-mediated m6A modifications (51). These results suggested that SA-AKI may be regulated by m6A methylation.
To further verify this assumption, we first identified 8 differentially expressed m6A RNA methylation-related regulators. As shown in the heatmap in Figure 6A, in addition to the 8 significantly differentially expressed m6A genes, the overall gene expression levels of 23 m6A RNA methylation-related regulators were obviously different between the CLP and control groups. The Western blotting results showed that the protein expression of Mettl3 (a core writer) was decreased in SA-AKI, and the expression of Alkbh5 (a core eraser) was increased. Notably, the mRNA expression levels of Mettl3 between sham and CLP in the heatmap were not significant due to the individual differences of mice. The Mettl3 protein levels are more persuasive because of the methyltransferase function in the form of protein. These results suggested that RNA methylation modification played an important role in the pathophysiological process of AKI induced by sepsis, and the total abundance of m6A may be downregulated.
The PPI network and expression correlation analyses showed close interactions among m6A RNA methylation regulators. We noted that Hmox1, with 21 m6A modification sites, showed numerous correlations with m6A methylation regulators, such as Mettl14 and Hnrnpa2b1. These results indicated that m6A “writers”, “erasers” and “readers” may interact with hub genes and play a synergistic role in RNA methylation modifications to influence the expression of hub genes, especially Hmox1. To the best of our knowledge, the present study is the first transcriptome-wide study to propose the possible involvement of m6A RNA methylation in the pathophysiological process of AKI induced by sepsis and provide a possible mechanism.
The primary goal of this study was to provide as many novel directions as possible using bioinformatics analyses for further mechanistic research and potential clinical therapeutic strategies for SA-AKI. The association between m6A RNA methylation modifications and SA-AKI was exported in silicon. However, the limitations of this study were that the roles of m6A RNA methylation-related regulators in AKI induced by sepsis were primarily deduced from high-throughput sequencing analysis. Although we performed qPCR and Western blotting experiments in vivo and in vitro to verify the bioinformatic analysis results, the mechanisms underlying m6A RNA methylation regulation and the verification of signaling pathways must be demonstrated in further experiments, such as MeRIP-Seq. The sample size was relatively limited. Therefore, future studies should increase the sample size.
Conclusions
The present study identified 7 key genes (Hmox1, Spp1, Socs3, Mapk14, Lcn2, Cxcl1 and Cxcl12) that were significantly involved in signaling pathways. We found that mmu-miR-7212-5p-Hmox1 in ferroptosis was a key RNA regulatory pathway that participated in the pathophysiological process of SA-AKI. Our study demonstrated the expression profiles and potential functions of m6A RNA methylation regulators in SA-AKI, which significantly correlated with some hub genes and promoted the progression of AKI.
Acknowledgments
Funding: This work was supported by the Fund of the Improvement of Scientific and Technological Innovation Ability Project of Army Medical University (No. 2019XQY18 to Xia Yang) and the Fund of the National Natural Science Foundation of China (Nos. 82070072 and 81873644 to Xinjin Chi).
Footnote
Reporting Checklist: The authors have completed the ARRIVE reporting checklist. Available at https://atm.amegroups.com/article/view/10.21037/atm-22-845/rc
Data Sharing Statement: Available at https://atm.amegroups.com/article/view/10.21037/atm-22-845/dss
Conflicts of Interest: All authors have completed the ICMJE uniform disclosure form (available at https://atm.amegroups.com/article/view/10.21037/atm-22-845/coif). The authors have no conflicts of interest to declare.
Ethical Statement: The authors are accountable for all aspects of the work in ensuring that questions related to the accuracy or integrity of any part of the work are appropriately investigated and resolved. Experiments were performed under a project license (No. AMUWEC20201509) granted by the Laboratory Animal Welfare and Ethics Committee of Third Military Medical University, in compliance with the Third Military Medical University institutional guidelines for the care and use of animals.
Open Access Statement: This is an Open Access article distributed in accordance with the Creative Commons Attribution-NonCommercial-NoDerivs 4.0 International License (CC BY-NC-ND 4.0), which permits the non-commercial replication and distribution of the article with the strict proviso that no changes or edits are made and the original work is properly cited (including links to both the formal publication through the relevant DOI and the license). See: https://creativecommons.org/licenses/by-nc-nd/4.0/.
References
- Singer M, Deutschman CS, Seymour CW, et al. The Third International Consensus Definitions for Sepsis and Septic Shock (Sepsis-3). JAMA 2016;315:801-10. [Crossref] [PubMed]
- Poston JT, Koyner JL. Sepsis associated acute kidney injury. BMJ 2019;364:k4891. [Crossref] [PubMed]
- Dou Q, Tong H, Yang Y, et al. PICK1 Deficiency Exacerbates Sepsis-Associated Acute Kidney Injury. Biomed Res Int 2021;2021:9884297. [Crossref] [PubMed]
- Peerapornratana S, Manrique-Caballero CL, Gómez H, et al. Acute kidney injury from sepsis: current concepts, epidemiology, pathophysiology, prevention and treatment. Kidney Int 2019;96:1083-99. [Crossref] [PubMed]
- Yoshimoto K, Komaru Y, Iwagami M, et al. Acute Kidney Injury in Sepsis: Evidence From Asia. Semin Nephrol 2020;40:489-97. [Crossref] [PubMed]
- Kellum JA, Romagnani P, Ashuntantang G, et al. Acute kidney injury. Nat Rev Dis Primers 2021;7:52. [Crossref] [PubMed]
- Scholz H, Boivin FJ, Schmidt-Ott KM, et al. Kidney physiology and susceptibility to acute kidney injury: implications for renoprotection. Nat Rev Nephrol 2021;17:335-49. [Crossref] [PubMed]
- Yong WP, Rha SY, Tan IB, et al. Real-Time Tumor Gene Expression Profiling to Direct Gastric Cancer Chemotherapy: Proof-of-Concept “3G” Trial. Clin Cancer Res 2018;24:5272-81. [Crossref] [PubMed]
- Almansa R, Ortega A, Ávila-Alonso A, et al. Quantification of Immune Dysregulation by Next-generation Polymerase Chain Reaction to Improve Sepsis Diagnosis in Surgical Patients. Ann Surg 2019;269:545-53. [Crossref] [PubMed]
- Wu K, Nie B, Li L, et al. Bioinformatics analysis of high frequency mutations in myelodysplastic syndrome-related patients. Ann Transl Med 2021;9:1491. [Crossref] [PubMed]
- Zhang Q, Hu H, Chen SY, et al. Transcriptome and Regulatory Network Analyses of CD19-CAR-T Immunotherapy for B-ALL. Genomics Proteomics Bioinformatics 2019;17:190-200. [Crossref] [PubMed]
- Wu J, Qin Y, Li Z, et al. Comprehensive analysis of lncRNA and miRNA expression profiles and ceRNA network construction in negative pressure wound therapy. Ann Transl Med 2021;9:1383. [Crossref] [PubMed]
- Cheng Q, Chen X, Wu H, et al. Three hematologic/immune system-specific expressed genes are considered as the potential biomarkers for the diagnosis of early rheumatoid arthritis through bioinformatics analysis. J Transl Med 2021;19:18. [Crossref] [PubMed]
- Tang Y, Yang X, Shu H, et al. Bioinformatic analysis identifies potential biomarkers and therapeutic targets of septic-shock-associated acute kidney injury. Hereditas 2021;158:13. [Crossref] [PubMed]
- Yang JJ, Wu BB, Han F, et al. Gene expression profiling of sepsis-associated acute kidney injury. Exp Ther Med 2020;20:34. [Crossref] [PubMed]
- Wei CM, Gershowitz A, Moss B. Methylated nucleotides block 5' terminus of HeLa cell messenger RNA. Cell 1975;4:379-86. [Crossref] [PubMed]
- Du J, Liao W, Liu W, et al. N6-Adenosine Methylation of Socs1 mRNA Is Required to Sustain the Negative Feedback Control of Macrophage Activation. Dev Cell 2020;55:737-753.e7. [Crossref] [PubMed]
- Huang H, Weng H, Chen J. m6A Modification in Coding and Non-coding RNAs: Roles and Therapeutic Implications in Cancer. Cancer Cell 2020;37:270-88. [Crossref] [PubMed]
- Tong J, Flavell RA, Li HB. RNA m6A modification and its function in diseases. Front Med 2018;12:481-9. [Crossref] [PubMed]
- Zhao BS, Roundtree IA, He C. Post-transcriptional gene regulation by mRNA modifications. Nat Rev Mol Cell Biol 2017;18:31-42. [Crossref] [PubMed]
- Qu F, Tsegay PS, Liu Y. N6-Methyladenosine, DNA Repair, and Genome Stability. Front Mol Biosci 2021;8:645823. [Crossref] [PubMed]
- Sun T, Wu R, Ming L. The role of m6A RNA methylation in cancer. Biomed Pharmacother 2019;112:108613. [Crossref] [PubMed]
- Qin Y, Li L, Luo E, et al. Role of m6A RNA methylation in cardiovascular disease Int J Mol Med 2020;46:1958-72. (Review). [Crossref] [PubMed]
- Fan H, Le JW, Sun M, et al. Sirtuin 3 deficiency promotes acute kidney injury induced by sepsis via mitochondrial dysfunction and apoptosis. Iran J Basic Med Sci 2021;24:675-81. [PubMed]
- Li Y, Zhai P, Zheng Y, et al. Csf2 Attenuated Sepsis-Induced Acute Kidney Injury by Promoting Alternative Macrophage Transition. Front Immunol 2020;11:1415. [Crossref] [PubMed]
- Chen S, Zhou Y, Chen Y, et al. fastp: an ultra-fast all-in-one FASTQ preprocessor. Bioinformatics 2018;34:i884-90. [Crossref] [PubMed]
- Langmead B, Salzberg SL. Fast gapped-read alignment with Bowtie 2. Nat Methods 2012;9:357-9. [Crossref] [PubMed]
- Wickham H. ggplot2: Elegant Graphics for Data Analysis. 2nd ed. Use R! Cham: Springer International Publishing: Imprint: Springer, 2016. doi:
10.1007/978-3-319-24277-4 .10.1007/978-3-319-24277-4 - Subramanian A, Tamayo P, Mootha VK, et al. Gene set enrichment analysis: a knowledge-based approach for interpreting genome-wide expression profiles. Proc Natl Acad Sci U S A 2005;102:15545-50. [Crossref] [PubMed]
- Szklarczyk D, Gable AL, Lyon D, et al. STRING v11: protein-protein association networks with increased coverage, supporting functional discovery in genome-wide experimental datasets. Nucleic Acids Res 2019;47:D607-13. [Crossref] [PubMed]
- Agarwal V, Bell GW, Nam JW, et al. Predicting effective microRNA target sites in mammalian mRNAs. Elife 2015;4:e05005. [Crossref] [PubMed]
- Sticht C, De La Torre C, Parveen A, et al. miRWalk: An online resource for prediction of microRNA binding sites. PLoS One 2018;13:e0206239. [Crossref] [PubMed]
- Chen Y, Wang X. miRDB: an online database for prediction of functional microRNA targets. Nucleic Acids Res 2020;48:D127-31. [Crossref] [PubMed]
- Gong J, Liu C, Liu W, et al. LNCediting: a database for functional effects of RNA editing in lncRNAs. Nucleic Acids Res 2017;45:D79-84. [Crossref] [PubMed]
- Yang C, Hu Y, Zhou B, et al. The role of m6A modification in physiology and disease. Cell Death Dis 2020;11:960. [Crossref] [PubMed]
- Li Y, Ge YZ, Xu L, et al. The Potential Roles of RNA N6-Methyladenosine in Urological Tumors. Front Cell Dev Biol 2020;8:579919. [Crossref] [PubMed]
- Xuan JJ, Sun WJ, Lin PH, et al. RMBase v2.0: deciphering the map of RNA modifications from epitranscriptome sequencing data. Nucleic Acids Res 2018;46:D327-34. [Crossref] [PubMed]
- Wang Y, Zhang M, Bi R, et al. ACSL4 deficiency confers protection against ferroptosis-mediated acute kidney injury. Redox Biol 2022;51:102262. [Crossref] [PubMed]
- Vandewalle J, Libert C. Sepsis: a failing starvation response. Trends Endocrinol Metab 2022;33:292-304. [Crossref] [PubMed]
- Guo C, Ye FX, Jian YH, et al. MicroRNA-214-5p aggravates sepsis-related acute kidney injury in mice. Drug Dev Res 2022;83:339-50. [Crossref] [PubMed]
- Lei P, Bai T, Sun Y. Mechanisms of Ferroptosis and Relations With Regulated Cell Death: A Review. Front Physiol 2019;10:139. [Crossref] [PubMed]
- Scindia Y, Leeds J, Swaminathan S. Iron Homeostasis in Healthy Kidney and its Role in Acute Kidney Injury. Semin Nephrol 2019;39:76-84. [Crossref] [PubMed]
- Adedoyin O, Boddu R, Traylor A, et al. Heme oxygenase-1 mitigates ferroptosis in renal proximal tubule cells. Am J Physiol Renal Physiol 2018;314:F702-14. [Crossref] [PubMed]
- Chiang SK, Chen SE, Chang LC. A Dual Role of Heme Oxygenase-1 in Cancer Cells. Int J Mol Sci 2018;20:39. [Crossref] [PubMed]
- Wang Y, Quan F, Cao Q, et al. Quercetin alleviates acute kidney injury by inhibiting ferroptosis. J Adv Res 2021;28:231-43. [Crossref] [PubMed]
- Li J, Lu K, Sun F, et al. Panaxydol attenuates ferroptosis against LPS-induced acute lung injury in mice by Keap1-Nrf2/HO-1 pathway. J Transl Med 2021;19:96. [Crossref] [PubMed]
- Ueda N. A Rheostat of Ceramide and Sphingosine-1-Phosphate as a Determinant of Oxidative Stress-Mediated Kidney Injury. Int J Mol Sci 2022;23:4010. [Crossref] [PubMed]
- Li JY, Yao YM, Tian YP. Ferroptosis: A Trigger of Proinflammatory State Progression to Immunogenicity in Necroinflammatory Disease. Front Immunol 2021;12:701163. [Crossref] [PubMed]
- Gu J, Zhan Y, Zhuo L, et al. Biological functions of m6A methyltransferases. Cell Biosci 2021;11:15. [Crossref] [PubMed]
- Zhang S, Liu F, Wu Z, et al. Contribution of m6A subtype classification on heterogeneity of sepsis. Ann Transl Med 2020;8:306. [Crossref] [PubMed]
- Mi B, Xiong Y, Yan C, et al. Methyltransferase-like 3-mediated N6-methyladenosine modification of miR-7212-5p drives osteoblast differentiation and fracture healing. J Cell Mol Med 2020;24:6385-96. [Crossref] [PubMed]