The feasibility of granzyme B levels using an amperometric immunosensor for lung cancer detection
Introduction
Lung cancer, one of the most common cancers worldwide, accounts for approximately 18% of all cancer-related deaths (1). The 5-year survival rate is 58–73% for patients with stage I lung cancer but only 3.5% for those with later stages of the disease (2,3). Approximately 75% of the patients are diagnosed when the disease has progressed to stage III or IV. The mortality rate remains remarkably high despite significant advances in the treatment of advanced-stage lung cancer (3). Therefore, the diagnosis of early-stage lung cancer is essential for survival and a favorable prognosis (4,5).
Studies on lung cancer screening and early detection have been carried out in recent decades. The National Lung Screening Trial demonstrated a 6.7% improvement in overall survival and a 20% reduction in mortality for patients screened using low-dose computed tomography (LDCT) (6). However, LDCT scans present several disadvantages, including the abundance of false-positive results, which lead to a high socioeconomic cost, psychological burden, and repeated exposure to radiation (7). To minimize these drawbacks and maximize efficacy, screening should be limited to individuals at high risk of lung cancer (8). Therefore, discovering novel biomarkers with higher diagnostic performance in lung cancers is sorely needed. Recently, tumor-related proteins, miRNAs, and DNA biomarkers in the blood have been used as complementary biomarkers for lung cancer detection (9-11). However, biomarkers with high sensitivity and specificity have not been identified.
Granzyme B is a cytolytic serine protease that mediates cancer cell apoptosis by triggering natural killer (NK) cells and CD8+ T cell responses along with perforin and interferon (IFN)-γ production (12). The use of granzyme B nanoparticles and positron emission tomography imaging has enabled us to quantitatively detect responsiveness to immunotherapy in accordance with tumor volume reduction (13). Previously, we also reported that the measurement of granzyme B levels in blood samples from patients with lung cancer could predict immunotherapy outcomes (14). Interestingly, the number of granzyme B-secreting NK T-like, NK, and CD8+ T cells and their granzyme B contents are decreased in lung cancer tissues compared to those in non-lung cancer tissues (15,16). Because of these differential granzyme B levels, we hypothesized that granzyme B levels in peripheral blood of lung cancer patients might serve as an additional biomarker for lung cancer diagnosis.
This feasibility study investigated the diagnostic performance of granzyme B testing by measuring serum granzyme B levels with a novel amperometric biosensor. We also used a machine learning approach to validate the diagnostic accuracy of granzyme B testing and investigated whether its combination with clinical parameters improves the diagnostic performance compared to predictors in practice for selecting high-risk patients in lung cancer screening. We present the following article in accordance with the STARD reporting checklist (available at https://atm.amegroups.com/article/view/10.21037/atm-22-470/rc).
Methods
Patients
This study was a retrospective, diagnostic accuracy study involving 95 participants enrolled at Pusan National University Yangsan Hospital in Yangsan, South Korea. Recruitment occurred between From March 2018 to September 2019. Patients with histologically confirmed primary lung cancer were enrolled. Lung tumors were staged following the American Joint Committee on Cancer TNM guideline. Patients with regular check-ups who visited a health promotion center were considered a “healthy control individuals” group. All patients selected from the health promotion center underwent chest radiography to exclude lung malignancy. Adults aged 50-80 at increased risk of developing lung cancer than the general populations were eligible to participate. There were defined as current smokers with at least 20 pack years. Potential participants were reviewed from the electronic medical records. All patients had a terminal illness or previous interventions, including chemotherapy, and had an Eastern Cooperative Oncology Group performance status of 0–2 at recruitment. Samples of peripheral blood were collected by venipuncture. Blood samples (10 mL) were collected in SST tubes from each participant. The blood was allowed to clot in an upright position for approximately 30 minutes and then centrifuged for 10 minutes at 1.4 ×g within one hour of collection. The serum was collected and transferred to a plastic screw-cap vial for transport to the laboratory. The study was conducted in accordance with the Declaration of Helsinki (as revised in 2013) and was approved by the Institutional Review Board of Pusan National University Yangsan Hospital (IRB No. 05-2020-199). Written informed consent was obtained from all the patients.
Immunosensor development
Our developed immunobiosensor was employed to determine serum granzyme B levels in patients in this study. Briefly, the immunosensor was engineered by covalent immobilization of bioreceptors on a conductive polymer/gold nanoparticle-modified screen-printed carbon electrode (CP/AuNPs/SPCE) (17). We added different volumes (0, 5, 10, 15, and 20 µL) of 50 pg/mL granzyme B to five tubes containing 15 µL serum each. Next, we adjusted the total volume to 50 µL with 0.1 M PBS (pH 7.4). The concentrations of granzyme B added to tubes 1–5 were determined to be 0, 5, 10, 15, and 20 pg/mL, respectively. The mixture in each tube was then mixed thoroughly. Afterward, 4 µL of each spiked sample was drop-casted onto the sensing probe, followed by incubation for 20 min at room temperature. After the reaction and subsequent washes with PBS, 4 µL of the freshly prepared bioconjugate was drop-casted onto the electrode surface. Twenty minutes after drop-casting, the sensor surface was washed three times with PBS, and then the sensor was inserted into the detection device. All experiments were repeated at least in triplicate.
Model selection and cross-validation of the machine learning technique
We used four machine learning models [random forest (18), XGBoost (19), logistic regression (20), and support vector machine (21)] in the scikit-learn package (22) of Python to select the best model for lung cancer diagnosis. We then performed binary classification to predict whether the patients had lung cancer or not. Owing to the insufficient number of samples, we conducted a total of 25 experiments by repeating a 5-fold cross-validation five times with different randomizations in each repetition. We used the mean value of the repeated cross-validation to calculate the performance across all folds and repeats for a more reliable evaluation.
Statistical analysis
Statistical data analysis was performed using the R program (version 4.0.5). The t-test and chi-square test were used to compare the general characteristics. Receiver operating characteristic (ROC) curves were constructed, and the area under the ROC curve (AUC) was calculated to evaluate sensitivity, specificity, positive predictive value, and negative predictive value. The cut-off point on the ROC curves, at which the maximal granzyme B detection accuracy was selected. Pearson correlation was performed to examine the correlation between granzyme B levels and inflammatory markers, including C-reactive protein (CRP). The paired t-test was performed to examine whether granzyme B levels play a significant role in improving predictive power (95% CI: −0.13 to −0.08). We compared the AUC of the base features (age, pack-years, and smoking status) with the AUC of the combination of the base features and granzyme B levels, consisting of 25 experiments in repeated cross-validation.
Results
Patient characteristics
From March 2018 to September 2019, we enrolled 44 patients with lung cancer and 51 healthy normal patients. Among lung cancer patients, 9 (20.5%) were diagnosed as small-cell lung cancer, 35 (79.5%) as non-small cell lung cancer. No significant baseline differences were found in age, sex, or smoking status. However, pack-years showed a significant difference between the lung cancer and normal healthy controls groups. The fractions of patients at each stage were as follows: 20.5% stage I, 20.5% stage II, 13.5% stage III, and 45.5% stage IV. The patients were also divided according to histological tumor type. Baseline characteristics are summarized in Table 1.
Table 1
Characteristic | Healthy controls (N=51) | Lung cancer (N=44) | P value |
---|---|---|---|
Mean age, y | 65.4 | 66.5 | 0.439 |
Sex (male), n (%) | 36 (70.6) | 37 (84.1) | 0.122 |
Currently smoking, n (%) | 25 (49.0) | 21 (47.7) | 0.901 |
Mean pack-years | 28.6 | 43.5 | 0.014 |
Stage, n (%) | |||
1 | 9 (20.5) | ||
2 | 9 (20.5) | ||
3 | 6 (13.5) | ||
4 | 20 (45.5) | ||
Histologic subtype, n (%) | |||
SCLC | 9 (20.5) | ||
NSCLC | 35 (79.5) |
SCLC, small-cell lung cancer; NSCLC, non-small cell lung cancer.
Diagnostic value of granzyme B levels in patients with lung cancer
The mean scores of granzyme B levels for the healthy control individuals and patients with lung cancer were 25.98 and 10.88 pg/mL, respectively (Figure 1A). Analysis of these data revealed a sensitivity, specificity, positive predictive value, and negative predictive value of 100%, 80%, 82%, and 100%, respectively (Table 2). The ROC curve of the data is shown in Figure 1B. The AUC was 0.938, and an 18.19 pg/mL cut-off value was imposed. The individual classification of all healthy control individuals and patients with lung cancer is presented in a scatter graph (Figure 1C). Patients with granzyme B levels greater than the cut-off value were regarded as negative, and those with values less than 18.19 pg/mL were regarded as positive for lung cancer. These findings suggested that the predictive power of granzyme B for lung cancer detection was even superior to that of the known tumor markers carcinoembryonic antigen (CEA) and pro-gastrin-releasing peptide (pro-GRP) (Table S1). Granzyme B performs extracellular functions in inflammation by triggering cytokine production. Therefore, we studied whether serum granzyme B levels were directly associated with inflammatory markers such as CRP. No correlations between granzyme B and inflammatory mediator levels were observed (Table S2). These results indicate that the expression level of inflammatory markers has no significant effect on the analysis of granzyme B levels in patients with lung cancer.
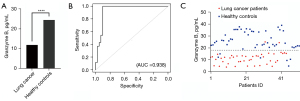
Table 2
Metric | Value |
---|---|
Sensitivity | 1.00 |
Specificity | 0.80 |
Positive predictive value | 0.82 |
Negative predictive value | 1.00 |
Model selection, performance, and cross-validation
We used a machine learning approach for cross-validation to reduce biased results with small sample sizes. In this study, we conducted experiments using granzyme B levels measured in triplicate with the biosensor and conventional clinical predictors such as age, smoking status, and mean pack-years. Of the four machine learning models tested (random forest, XGBoost, logistic regression, and support vector machine), the random forest model had the highest AUC (0.977 vs. 0.963, 0.961, and 0.957 for logistic regression, XGBoost, and SVC, respectively), as shown in Table 3. Thus, the random forest was used in all subsequent analyses. Table 4 shows that the model performed well in predicting lung cancer, with an accuracy of 92.0%, a sensitivity of 92.0%, specificity of 92.2%, a negative prediction value of 92.6%, and positive prediction value of 91.8%.
Table 3
Model | w/o granzyme B | With granzyme B |
---|---|---|
Random forest | 0.878 | 0.977 |
XGBoost | 0.848 | 0.963 |
Logistic regression | 0.809 | 0.961 |
SVM | 0.825 | 0.957 |
XGBoost, extreme gradient boosting; SVM, support vector machine.
Table 4
Metric | w/o granzyme B | With granzyme B |
---|---|---|
Sensitivity | 0.728 | 0.920 |
Specificity | 0.802 | 0.922 |
Positive predictive value | 0.772 | 0.918 |
Negative predictive value | 0.785 | 0.926 |
Accuracy | 0.763 | 0.920 |
ROC AUC | 0.878 | 0.977 |
ROC, receiver operating characteristics; AUC, area under the curve.
To determine the effect of granzyme B levels on lung cancer diagnosis, we conducted several experiments with and without adding granzyme B levels. As shown in Table 3, all four models, including granzyme B testing, outperformed those without it. As shown in Figure S1, the mean AUC of the four models with granzyme B testing (0.964) was higher than that without granzyme B testing (0.840). We also compared the ROC curve for granzyme B testing in the random forest, and the performance of the model with granzyme B testing (AUC =0.976) was better than that without it (AUC =0.878) (Figure 2). The paired t-test checked whether granzyme B testing improved the predictive rate. There was a significant difference between the AUC with granzyme B testing and without it (P=2.03e-10). These findings are in line with the statistical results suggesting that granzyme B testing has diagnostic value in lung cancer.
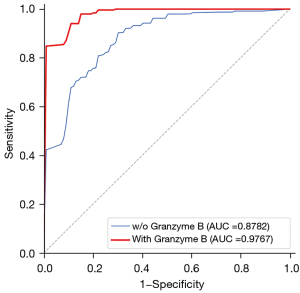
Furthermore, the performances of the tests combining granzyme B testing with each of the nine single features are shown in the Table S3. The two best AUCs corresponded to the tests combining granzyme B testing with either pack-years (AUC 0.975) or smoking status (AUC =0.974). These results suggest that granzyme B could improve the diagnostic performance for lung cancer detection.
Discussion
LDCT scans have demonstrated significant improvements in overall survival and reduced mortality for patients with lung cancer; however, they also present several drawbacks. Therefore, screening should be done on individuals at high risk of lung cancer (8). To ensure this, discovering novel biomarkers with higher diagnostic performance in lung cancer is crucial. This feasibility study hypothesized that granzyme B levels could potentially be used as a biomarker for lung cancer detection. Here, we sought to investigate the diagnostic performance of granzyme B testing. To that aim, we measured serum granzyme B levels in samples from patients with lung cancer using a novel amperometric biosensor. Furthermore, we used machine learning approaches to validate the diagnostic performance of granzyme B testing and investigate whether the combination of granzyme B testing with clinical parameters improves the diagnostic effectiveness for selecting high-risk patients in lung cancer detection.
This study found that the mean value and range of granzyme B levels differed between healthy control individuals and patients with lung cancer (25.98 pg/mL in healthy control individuals vs. 10.88 pg/mL in patients with lung cancer). Using a cut-off value, the test identified 95.4% of the 44 patients with lung cancer; two patients were false negatives. However, due to the small sample size, we used machine learning to determine the optimal application of granzyme B testing for risk prediction. The random forest model with a 5-fold cross-validation showed that the integration of granzyme B testing and predictors such as age, tobacco status, and pack-years could raise the sensitivity from 72% to 92% and the mean AUC from 0.878 to 0.977 compared to the use of predictors alone for lung cancer detection. One aspect of selection bias is that pack-years were not matched between the two groups because the samples were collected prospectively. A recent study reported that cigarette smoking induces CD8+ T cell exhaustion and, consequently, granzyme B levels are lower in smokers than in non-smokers (23). We adjusted pack-year status between the two groups through propensity score matching to reduce bias (Table S4). As shown in Table S5, no significant differences in the diagnostic performance of granzyme B testing for lung cancer detection were found after propensity score matching. Consequently, our findings demonstrate that granzyme B testing can complement existing predictors, increasing the sensitivity and specificity.
Using nanotechnology, biosensors can identify biological elements that require ultra-high sensitivity by using a nanoscale detector as a component; thus, the disadvantages of existing diagnostic and monitoring methods can be overcome. There is considerable interest in immunosensors in the field of nanobiotechnology; several studies attempting to develop immunosensors for faster, more accurate, and more selective detection of biomarkers are underway (24,25). Our previous study showed that immunosensors could be used for peripheral blood analysis to detect lung cancer and have demonstrated relatively high diagnostic accuracies (26). In this study, our novel immunosensor showed a sensitivity of 100% and specificity of 80% for granzyme B detection in patients with lung cancer. In addition to the analysis with three replicates of granzyme B levels, we performed experiments with a single measurement (the first value of the three replicates) to examine the differences in the results. As shown in Table S6 and Figure S2, the results using either one or three replicates combined with base features did not show significant differences. Overall, the performances with three replicates for granzyme B levels outperformed those with one replicate. However, a one-time measurement appears sufficient given our results when granzyme B levels were combined with the base features.
As for early detection of lung cancer, the granzyme B levels were relatively lower than the cut-off value for samples obtained from patients with early-stage lung cancer [stage IA to IIA; 11.382 pg/mL (range, 4.076–16.342 pg/mL)]. However, this retrospective study was not confined to enrolled patients with early stages. For further study, we have recruited and evaluated the clinical utility of granzyme B levels in the context of abnormal LDCT findings among patients at high risk of lung cancer by detecting granzyme B levels among a cohort of patients with increased lung cancer risk undergoing LDCT imaging. Such an approach would represent great value in assessing which of these abnormal findings are false positives.
The limitation of this study was the relatively small number of enrolled patients. We performed a 5-fold cross-validation of the random forest model to delineate this limitation. However, the model should be externally validated with human samples obtained from a large-scale multicenter study in the future. Although any lung cancer stage showed a correlation with granzyme B compared to the normal group, there was no statistically significant difference between early and advanced lung cancer. Therefore, in addition to the above limitation, it is necessary to confirm this limitation through a future study involving many patients.
Furthermore, this study compared patients with lung cancer and healthy participants who had been selected from a health promotion center. Although all patients selected from the health promotion center underwent chest radiography to exclude a possible lung malignancy, they did not undergo LDCT scans. However, in a real-world clinic, LDCT screening is applicable in patients with high-risk factors such as aged 54–74 years, current smokers, and more than 30 pack-years. Thereby, patients who do not fit the criteria underwent the chest X-ray. We will design a further prospective study to include only patients with high risk to reduce these limitations. In conclusion, this feasibility study demonstrates that our novel electrochemical granzyme B sensor may potentially be used as a tool in existing screening methods for lung cancer detection. In addition, the combination of granzyme B can complement existing predictors and can thus improve the process of selection of high-risk patients for lung cancer screening.
Acknowledgments
This study was provided with biospecimens and clinical data from the Institutional Biobank Project (OF-2020-15) according to the individual research protocol.
Funding: This study was supported by the National Research Foundation of Korea (NRF) grant, funded by the Korean government (MSIT) (No. NRF-2022R1A2C1009379) and Research Institute for Convergence of Biomedical Science and Technology, Pusan National University Yangsan Hospital (No. 30-2021-020).
Footnote
Reporting Checklist: The authors have completed the STARD reporting checklist. Available at https://atm.amegroups.com/article/view/10.21037/atm-22-470/rc
Data Sharing Statement: Available at https://atm.amegroups.com/article/view/10.21037/atm-22-470/dss
Conflicts of Interest: All authors have completed the ICMJE uniform disclosure form (available at https://atm.amegroups.com/article/view/10.21037/atm-22-470/coif). JHC reports provision of study materials from Institutional Biobank Project (OF-2020-15), and funding from National Research Foundation of Korea (NRF) (No. NRF-2022R1A2C1009379) and Pusan National University Yangsan Hospital (No. 30-2021-020). The other authors have no conflicts of interest to declare.
Ethical Statement: The authors are accountable for all aspects of the work in ensuring that questions related to the accuracy or integrity of any part of the work are appropriately investigated and resolved. The study was conducted in accordance with the Declaration of Helsinki (as revised in 2013). The study was approved by the Institutional Review Board of Pusan National University Yangsan Hospital (IRB No. 05-2020-199) and informed consent was taken from all the patients.
Open Access Statement: This is an Open Access article distributed in accordance with the Creative Commons Attribution-NonCommercial-NoDerivs 4.0 International License (CC BY-NC-ND 4.0), which permits the non-commercial replication and distribution of the article with the strict proviso that no changes or edits are made and the original work is properly cited (including links to both the formal publication through the relevant DOI and the license). See: https://creativecommons.org/licenses/by-nc-nd/4.0/.
References
- Sung H, Ferlay J, Siegel RL, et al. Global Cancer Statistics 2020: GLOBOCAN Estimates of Incidence and Mortality Worldwide for 36 Cancers in 185 Countries. CA Cancer J Clin 2021;71:209-49. [Crossref] [PubMed]
- Camidge DR, Doebele RC, Kerr KM. Comparing and contrasting predictive biomarkers for immunotherapy and targeted therapy of NSCLC. Nat Rev Clin Oncol 2019;16:341-55. [Crossref] [PubMed]
- Goldstraw P, Chansky K, Crowley J, et al. The IASLC Lung Cancer Staging Project: Proposals for Revision of the TNM Stage Groupings in the Forthcoming (Eighth) Edition of the TNM Classification for Lung Cancer. J Thorac Oncol 2016;11:39-51. [Crossref] [PubMed]
- Pastorino U, Silva M, Sestini S, et al. Prolonged lung cancer screening reduced 10-year mortality in the MILD trial: new confirmation of lung cancer screening efficacy. Ann Oncol 2019;30:1162-9. [Crossref] [PubMed]
- International Early Lung Cancer Action Program Investigators. Survival of patients with stage I lung cancer detected on CT screening. N Engl J Med 2006;355:1763-71. [Crossref] [PubMed]
- de Koning HJ, van der Aalst CM, de Jong PA, et al. Reduced Lung-Cancer Mortality with Volume CT Screening in a Randomized Trial. N Engl J Med 2020;382:503-13. [Crossref] [PubMed]
- Oudkerk M, Liu S, Heuvelmans MA, et al. Lung cancer LDCT screening and mortality reduction - evidence, pitfalls and future perspectives. Nat Rev Clin Oncol 2021;18:135-51. [Crossref] [PubMed]
- Samet JM, Avila-Tang E, Boffetta P, et al. Lung cancer in never smokers: clinical epidemiology and environmental risk factors. Clin Cancer Res 2009;15:5626-45. [Crossref] [PubMed]
- Xiao T, Ying W, Li L, et al. An approach to studying lung cancer-related proteins in human blood. Mol Cell Proteomics 2005;4:1480-6. [Crossref] [PubMed]
- Sozzi G, Boeri M, Rossi M, et al. Clinical utility of a plasma-based miRNA signature classifier within computed tomography lung cancer screening: a correlative MILD trial study. J Clin Oncol 2014;32:768-73. [Crossref] [PubMed]
- Dama E, Colangelo T, Fina E, et al. Biomarkers and Lung Cancer Early Detection: State of the Art. Cancers (Basel) 2021;13:3919. [Crossref] [PubMed]
- Chávez-Galán L, Arenas-Del Angel MC, Zenteno E, et al. Cell death mechanisms induced by cytotoxic lymphocytes. Cell Mol Immunol 2009;6:15-25. [Crossref] [PubMed]
- LaSalle T, Austin EE, Rigney G, et al. Granzyme B PET imaging of immune-mediated tumor killing as a tool for understanding immunotherapy response. J Immunother Cancer 2020;8:e000291. [Crossref] [PubMed]
- Chung JH, Ha JS, Choi J, et al. Granzyme B for predicting the durable clinical benefit of anti-PD-1/PD-L1 immunotherapy in patients with non-small cell lung cancer. Transl Cancer Res 2022;11:316-26. [Crossref] [PubMed]
- Hurkmans DP, Basak EA, Schepers N, et al. Granzyme B is correlated with clinical outcome after PD-1 blockade in patients with stage IV non-small-cell lung cancer. J Immunother Cancer 2020;8:e000586. [Crossref] [PubMed]
- Soriano C, Mukaro V, Hodge G, et al. Increased proteinase inhibitor-9 (PI-9) and reduced granzyme B in lung cancer: mechanism for immune evasion? Lung Cancer 2012;77:38-45. [Crossref] [PubMed]
- Saputra HA, Chung JH, Yoon SH, et al. Disposable amperometric immunosensor with a dual monomers-based bioconjugate for granzyem B detection in blood and cancer progress monitoring of patients. Biosens Bioelectron 2022;198:113846. [Crossref] [PubMed]
- Breiman L. Random Forests. Machine Learning 2001;45:5-32. [Crossref]
- Chen T, Guestrin C. XGBoost: A Scalable Tree Boosting System. In Proceedings of the 22nd ACM SIGKDD International Conference on Knowledge Discovery and Data Mining. Association for Computing Machinery, New York, NY, USA 2016:785-94.
- Lai TL, Robbins H, Wei CZ. Strong consistency of least squares estimates in multiple regression. Proc Natl Acad Sci U S A 1978;75:3034-6. [Crossref] [PubMed]
- Cortes C, Vapnik V. Support-vector networks. Machine Learning 1995;20:273-297. [Crossref]
- Pedregosa F, Varoquaux G, Gramfort A, et al. Scikit-learn: Machine learning in Python. Journal of Machine Learning Research 2011;12:2825-30.
- Cheng CC, Lin HC, Chiang YW, et al. Nicotine exhausts CD8+ T cells against tumor cells through increasing miR-629-5p to repress IL2RB-mediated granzyme B expression. Cancer Immunol Immunother 2021;70:1351-64. [Crossref] [PubMed]
- Mahmudunnabi RG, Farhana FZ, Kashaninejad N, et al. Nanozyme-based electrochemical biosensors for disease biomarker detection. Analyst 2020;145:4398-420. [Crossref] [PubMed]
- Zhu Y, Chandra P, Shim YB. Ultrasensitive and selective electrochemical diagnosis of breast cancer based on a hydrazine-Au nanoparticle-aptamer bioconjugate. Anal Chem 2013;85:1058-64. [Crossref] [PubMed]
- Kim DM, Noh HB, Park DS, et al. Immunosensors for detection of Annexin II and MUC5AC for early diagnosis of lung cancer. Biosens Bioelectron 2009;25:456-62. [Crossref] [PubMed]