Bioinformatics analysis identifies diagnostic biomarkers and their correlation with immune infiltration in diabetic nephropathy
Introduction
Diabetic nephropathy (DN), a microvascular complication associated with the progression of diabetes, occurs in 30–40% in patients with diabetes (1,2). In 2017, there were approximately 451 million patients with diabetes globally, and this number is anticipated to increase to 693 million by 2045 (3). As the prevalence of diabetes continues to increase, DN has become the main cause of end-stage renal disease (ESRD) in both developed and developing countries. Even with costly and long-term therapeutic interventions, such as dialysis and kidney transplantation, patients with ESRD remain at risk for various complications such as anemia, mineral and bone disorder, and cardiovascular complications. Currently, microalbuminuria, serum creatinine level, estimated glomerular filtration rate (eGFR), and the urinary microalbumin to creatinine ratio (UACR) are used as the diagnostic markers of DN; however, comprehensive diagnosis is not yet possible with these methods. In addition, DN diagnosis is challenging as this condition is non-proteinuric (4). Consequently, there is a need to explore from multiple perspectives potential biomarkers DN.
Inflammation happens in the kidneys of patients with DN and plays a crucial role in its occurrence and development (5,6). The levels of circulating tumor necrosis factor (TNF)-α, monocyte chemoattractant protein (MCP)-1, interleukin (IL)-1, IL-6, and other inflammatory molecules are increased in patients with early DN (7). Activated monocytes and macrophages infiltrate the kidneys and release cytokines. Concomitantly, other cells, such as mast cells, can infiltrate the tubule interstitium and release pro-inflammatory factors and proteolytic enzymes (8). In the past few decades, multiple potential diagnostic markers have been identified. For example, serum TNF receptor 1 (TNFR1) and 2 (TNFR2) were found to be significantly positively correlated with some inflammation-related indicators of precocious glomerular lesions in DN, such as mesangial fractional volume, percentage of global glomerular sclerosis, and width of the glomerular basement membrane (9). Studies have shown that the activation of TNFR1 by TNFα promotes the formation of a complex containing TNFR-related death domain, Ser/Thr kinase receptor interacting protein 1, and TRAF-2, which triggers the activation of NF-κB. Signaling pathways, such as those of MAP kinase (MAPK) and p38 induce inflammation (10-12). However, with the exceptions of eGFR and UACR, other diagnostic markers which have been discovered have not been effectively applied in clinical practice, and no comprehensive analysis of immune cell infiltration in DN has been performed. Therefore, an in-depth exploration of immune cell infiltration should help to elucidate the molecular mechanisms underlying DN pathogenesis, and provide insights for the development of novel immunotherapeutic targets.
This study aimed to identify the pivotal genes and pathways associated with immune infiltration in patients with DN. We constructed and verified the diagnostic and prediction model of DN by performing least absolute shrinkage and selection operator (LASSO) analysis. Finally, to more specifically determine the key immune factors, we analyzed and screened the correlation between key genes and infiltrating immune cells. These analyses provided more accurate prognostic information regarding DN progression. We present the following article in accordance with the TRIPOD reporting checklist (available at https://atm.amegroups.com/article/view/10.21037/atm-22-1682/rc).
Methods
In this study, we first downloaded three datasets containing samples from DN patients from public databases and screened them for immune-related differentially expressed genes (DEGs), then further confirmed the relevance of these genes to immunity by enrichment analysis, followed by finding key genes by protein-protein interaction networks and building prediction models using these genes by LASSO and support vector machine recursive feature elimination (SVM-RFE), and finally validated the efficacy of the models with receiver-operating characteristic (ROC) curve, decision curve analysis (DCA), and nomogram. The flow chart of this study is shown in Figure S1. The study was conducted in accordance with the Declaration of Helsinki (as revised in 2013).
Data download and pre-processing
We downloaded 3 gene expression datasets [GSE30122 (13,14), GSE47184 (15), and GSE104948 (16)] were downloaded from Gene Expression Omnibus [GEO; GPL571 (HG-U133A_2) Affymetrix Human Genome U133A 2.0 Array; GPL14663 Affymetrix GeneChip Human Genome HG-U133A Custom CDF (Affy_HGU133A_CDF_ENTREZG_10); and GPL22945 (HG-U133_Plus_2) Affymetrix Human Genome U133 Plus 2.0 Array]. From these datasets, we obtained data related to kidney tissue and analyzed the messenger RNA (mRNA) gene expression profile; all analyses included DN and control cases, and the species was Homo sapiens. Subsequently, we merged datasets into a metadata cohort, corrected the background data, and normalized data using the normalizeBetweenArrays algorithm in Limma package (17) to obtain a gene expression matrix of 118 control and 37 DN kidney samples. The results were presented as box plots.
Analysis of differentially expressed genes (DEGs)
DEGs between the control and DN kidney samples were screened by Limma package, using ggplot2 package (18) (https://ggplot2.tidyverse.org), and used to plot a heat map and volcano plots. All DEGs satisfied P<0.05 and |log2 fold change| >0.5.
Immune-associated DEGs
To obtain immune-related DEGs, the DEGs were overlapped with 2,498 immune-related genes, which were obtained from the ImmPort database (19) and are presented as a Venn diagram (20).
Gene Ontology (GO)/Kyoto Encyclopedia of Genes and Genomes (KEGG) enrichment analysis
GO enrichment analyses, which involved biological processes (BP), cellular components (CC), and molecular functions (MF), and KEGG pathway analyses (21) were used to identify signaling pathways significantly associated with the DEGs. These analyses were executed using the clusterProfiler package (22).
Gene set enrichment analysis (GSEA) and gene set variation analysis enrichment analysis
To determine the contribution of genes to the phenotype, GSEA (23) was performed on the gene expression matrix using the clusterProfiler package. The reference gene set “c2.cp.kegg.v7.0.symbols.gmt” was derived from the Molecular Signatures Database (MSigDB) (24), and the top 30 items with P<0.05 (considered to be significantly enriched) were visualized. We also performed gene set variation analysis (GSVA) (25) of the overall gene expression profile matrix between DN and normal controls, with “msigdb.v7.0.symbols.gmt” as the background set, to select the phenotypic signature gene set.
Protein-protein interaction (PPI) construction
The Search Tool for the Retrieval of Interacting Genes/Proteins (STRING) database was used to search for known proteins and predict protein interactions (26). We used the STRING database to select genes with a combined score >400 to construct a differentially expressed mRNA-associated PPI, and then visualized the network model using Cytoscape (v3.7.0) (27). A molecular interaction network was constructed for these immune-related DEGs. Finally, we attained hub genes using Cytoscape’s Molecular Complex Detection (MCODE) (28) and CytoHubba (29) plug-ins.
Relationship between immune cell infiltration and hub genes
An immune cell infiltration expression matrix was obtained by filtering the output for samples with P<0.05 using CIBERSORT (30). Subsequently, heat maps of the immune cell infiltration matrix were drawn to show the allocation of 22 immune cell infiltrates in the respective kidney specimens using the pheatmap package (https://CRAN.R-project.org/package=pheatmap). Next, correlation heat maps were plotted to visualize correlations of the 22 immune cell infiltrates using the corrplot package (https://github.com/taiyun/corrplot), and violin plots were drawn to visualize diversity among immune cell infiltrates using the ggplot2 package (https://ggplot2.tidyverse.org).
Construction and validation of hub gene diagnostic marker prediction model
A hub gene diagnostic marker prediction model was initially structured using LASSO logistic regression by using the “glmnet” package in R. The LASSO algorithm was used to further analyze the prognosis-related hub gene for dimensionality reduction analysis and selection of features (31). The coefficients obtained from LASSO regression were weighted individual normalized gene expression values to
First, to demonstrate the personalized assessment of the immune-related hub gene diagnostic marker score to identify DN, we examined the correlation between the expression of immune-related hub genes in the control and DN groups, and the predictive power of the diagnostic marker score for the prognosis of patients with different stages of DN. The optimal parameter (λ) in the LASSO model was selected by minimal criteria using five-fold cross-validation. Secondly, we plotted partial likelihood deviation curves relative to log(λ). Vertical dashed lines were plotted at the optimal values by using the minimum criterion and the 1 standard error of the minimum criterion. Finally, the coefficient distributions were plotted for the log(λ) series.
Next, we screened for characteristic diagnostic marker genes in DN using the SVM-RFE method in “e1071” package (32). Ultimately, the intersection of diagnostic marker genes predicted by these two methods was obtained.
Diagnostic marker correlation analysis
A column line graph (nomogram) was used to demonstrate the efficacy of these predicted diagnostic marker genes for the discrimination of DN, and a differential expression heat map was generated to identify differences between the DN and control groups using LASSO. Calibration curves were used to demonstrate the discriminatory efficacy of these predicted diagnostic marker genes for diabetic nephropathy and to demonstrate the differences between DN and controls with gene expression heat maps. Calibration curves for non-correlated nomogram predictions in the cohort were analyzed and plotted, where the x-axis represents predicted diagnostic markers, the y-axis represents actual diagnostic nonconformity, the diagonal dashed line represents perfect prediction of the ideal model, and the solid line represents performance of the nomogram, where the dashed line closer to the diagonal represents better prediction.
Subsequently, DCA was performed to assess the net clinical benefit of the models for predicting DN (33). In the DCA curve, the x-axis represents the threshold probability and the y-axis represents the net benefit value, and the corresponding benefit value is calculated by continuously changing the threshold value of the positive probability. The horizontal line represents the case where all samples are negative and the net benefit is 0. The dotted line represents the case where all samples are positive. The model of DN has value if the plotted DCA curve is higher than these two lines.
Finally, the diagnostic efficacy of each independent gene and the combined index of these genes for DN were verified with ROC curves generated using the pROC package in R. An AUC >0.7 indicates that the model has moderate diagnostic efficacy, and an AUC >0.9 indicates that the model has high-level diagnostic efficacy, indicating that the screened diagnostic markers were of high diagnostic value.
Statistical analysis
Data were presented as the mean ± standard deviation. Data were analyzed using R software (version 4.0.2; The R Foundation for Statistical Computing, Vienna, Austria). Wilcoxon test or student t-test was used for comparisons between two groups. The AUC was calculated to assess the accuracy of the diagnostic marker scores in estimating prognosis. Results with two-side P<0.05 were considered statistically significant.
Results
Data processing and DEG analysis
A flow chart illustrating the study design is presented in Figure S1. The GSE30122, GSE47184, and GSE104948 datasets were combined and normalized, and are presented as box plots (Figure 1A,1B). After preprocessing, 1,515 DEGs between control and DN kidney tissues were extracted using R software; these included 759 and 756 genes with up- and down-regulated expression, respectively. A heat map and volcano map of DEGs are shown in Figure 1C,1D.
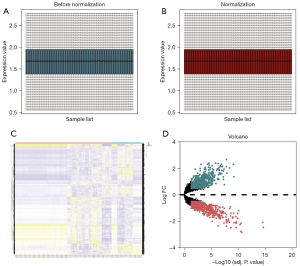
Identification of immune-related DEGs and GSVA associated with DN
Immune-related DEGs were identified at the junction between the DEGs for DN and the gene list from the ImmPort database. Next, the intersection of DEGs with immune-related genes was visualized using Venn diagrams for 191 DEGs (Figure 2A). Additionally, GSVA was conducted between groups, including pathway sets represented in the heat map (Figure 2B) and phenotypic related characteristic gene sets displayed in grouped box plots (Figure 2C).
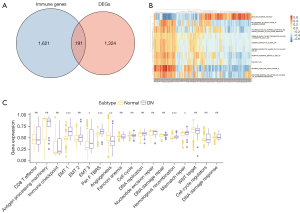
GSEA
Multiple biological pathways were found to be altered between the control and DN kidney tissues using GSEA. Enrichment plots (Figure 3A-3C) and heat maps (Figure 3D-3F) were generated for MsigDB, GO, and KEGG enrichment entries. Modules related to KEGG were investigated using UpSetR (Figure 3G). “Th1 and Th2 cell differentiation, rheumatoid arthritis, and viral protein interaction with cytokine and cytokine receptor” were significantly enriched in Top3 pathway (Figure 3H).
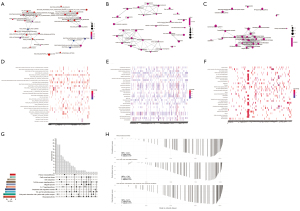
GO and KEGG enrichment analysis
Enrichment analysis of immune-related DEGs was performed using GO and KEGG, and visualized using bar (Figure 4A,4B) and bubble (Figure 4C,4D) graphs. The GO enrichment consequences of immune-related DEGs were focused on positive regulation of cell adhesion, T cell activation, leukocyte proliferation and migration, urogenital system development, lymphocyte differentiation and proliferation, and mononuclear cell proliferation. In addition, they were enriched in the PI3K-Akt signaling pathway, MAPK signaling pathway, Rap1 signaling pathway, proteoglycan in cancer, cytokine-cytokine receptor interaction, and WNT signaling pathway, among other pathways.
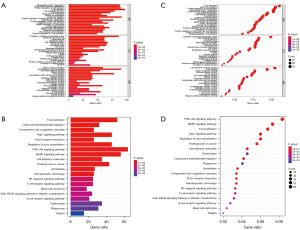
PPI network construction and hub gene identification
A PPI for 191 immune-associated DEGs was obtained and visualized using Cytoscape (Figure 5A). Subsequently, we intersected the most closely linked set of hub genes obtained from the MCODE plugin analysis (Figure 5B) with genes from the CytoHubba plugin MCC algorithm Top30 (Figure 5C). The 21 genes common to both algorithms were used as hub genes (Figure 5D).
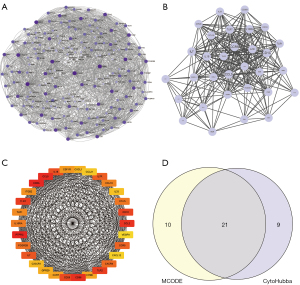
Construction of diagnostic marker prediction models
We used two different algorithms to screen potential biomarkers. Immune-related differential hub genes between control and DN sample groups were narrowed down by the LASSO regression algorithm, and consequently, 7 variables for early diagnosis were authenticated. Figure 6A,6B present the Lambda and minimum values of the LASSO logistic regression algorithm. Next, using the SVM-RFE algorithm, we obtained a subcollection of 7 features among the DEGs (Figure 6C). Finally, we superposed the 2 subsets and obtained 6 overlapping genes (encoding CD86, CCL19, CD1C, IL33, CXCR4, and IL7) as the diagnostic marker for DN (Figure 6D).
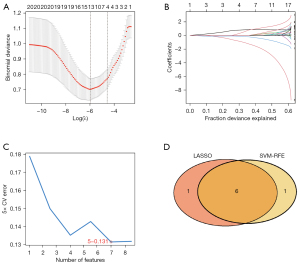
Diagnostic marker efficacy assessment
A nomogram drawn based on the above 6 genes showed the diagnostic efficacy of these predictive diagnostic marker genes for DN (Figure 7A), and the gene expression heat map showed the differences between DN and the control groups (Figure 7B). The calibration curve for the non-correlated nomogram prediction revealed that the performance of the nomogram was very close to the ideal model, indicating that the model has good predictive value (Figure 7C). Similarly, in the DCA analysis, the curves were higher than the 2 benefit threshold curves, indicating the efficacy of the model (Figure 7D). Finally, the diagnostic accuracy of the 6 diagnostic marker genes was evaluated by ROC curve analysis (Figure 7). The area under the curve (AUC) value, reflecting the diagnostic efficacy of each gene, was: 0.378, 0.445, 0.551, 0.553, 0.425, and 0.51 for CCL19, CD1C, CD86, CXCR4, IL33, and IL7, respectively (Figure 7E). Although their respective AUC values did not exceed 0.7, the model should be considered as a whole, rather than as the predictive power of individual genes. The AUC obtained by the combination of the 6 genes was 0.921>0.7, suggesting high diagnostic efficiency of the diagnostic marker gene model (Figure 7F).
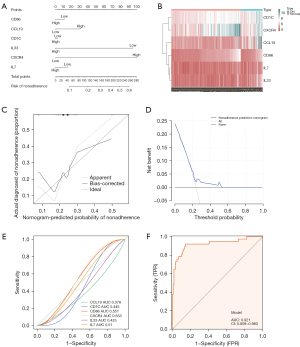
Differential analysis of immune cell infiltration
We derived an immune cell infiltration matrix by deconvolution using the gene expression matrix through the CIBERSORT algorithm. The immune cell percentage was calculated using the par function, and used to plot a stacked histogram (Figure 8A) and a heat map (Figure 8B). A correlation heat map was plotted to visualize the relationships among 22 immune cell infiltrations (Figure 8C). A violin plot was drawn to show differences among 22 immune cell infiltrations (Figure 8D), and the data were displayed as a series of significant differences between groups for various immune cells, including B cells, T cells, natural killer (NK) cells, plasma cells, dendritic cells (DC), mast cells, eosinophils, and neutrophils.
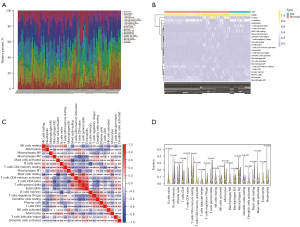
Differential expression of markers in DN
To confirm the accuracy of the results, the expression levels of CD86, CCL19, CD1C, IL33, CXCR4, and IL7 (Figure 9A-9F) were verified using the 3 datasets. However, only the expression of CCL19, CD1C, and IL33 (P=4.885e−07, P=0.016, P=8.914e−05) was significantly different in DN, with CCL19 being lowly expressed (Figure 9A) and IL33 (Figure 9B) and CD1C (Figure 9C) being highly expressed.
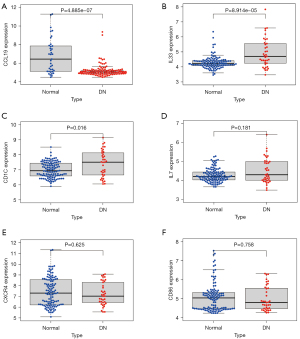
Correlation analysis of diagnostic markers with immune cells
By comparing immune cell infiltrates between the three diagnostic marker genes, the expression of CCL19, CD1C, and IL33 was found to be positively correlated with M1 and M2 macrophages and monocytes, and negatively correlated with M0 macrophages, memory-activated CD4T cells, and naïve CD4 T cells in DN. Notably, CCL19, CD1C, and IL33 strongly correlated with M2 macrophage infiltration (Figure 10).
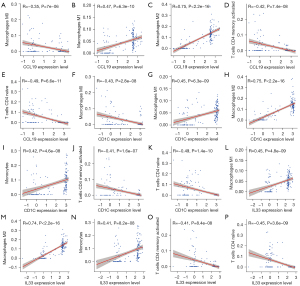
Discussion
The pathology of DN involves diabetes-associated chronic progressive kidney damage. Globally, there were 135 million DN patients and 0.5 million DN-related deaths in 2019, and diabetes is considered the primary contributor to new cases of chronic kidney disease (CKD) (34). Patients diagnosed with ESRD usually require renal replacement therapy, including dialysis (hemodialysis and peritoneal dialysis) and kidney transplantation. After receiving dialysis, patients may still experience anemia, bone disease, cardiovascular disease, and other complications. Similarly, after undergoing kidney transplantation, patients remain at risk for surgical failure and postoperative anti-rejection therapy. Notably, diabetes does not always progress to DN, and early treatment can delay disease progression. Therefore, a complete understanding of the pathological and molecular mechanisms underlying DN is important for early clinical diagnosis and treatment, and the identification of molecular markers that will help to diagnose DN early in the disease course. Microarray and bioinformatics analyses have enhanced our understanding of the molecular mechanisms underlying disease development and pathogenesis, which is essential for studying genetic changes and identifying potential diagnostic biomarkers. Here, we performed a comprehensive analysis of 3 mRNA microarray data sets (GSE30122, GSE47184, and GSE104948) using 118 control samples and 37 DN samples. Overall, we identified 191 immune-related DEGs. Furthermore, 21 immune-related DN hub genes were identified to have the highest scores in the PPI network. Next, we built and validated a prediction model and screened the key genes encoding CD86, CCL19, CD1C, IL33, CXCR4, and IL7. In addition, some immune cells, such as B cells, T cells, NK cells, eosinophils, and neutrophils, were significantly different in DN and the control group. Finally, we obtained 3 promising key genes (CCL19, CD1C, and IL33) in DN following differential expression analysis. Based on the correlation analysis, macrophages, T cells, CD4, and monocytes were found to be strongly related to the occurrence and development of DN. These results indicated that immune reactions exert an effect on gene expression in DN. In addition, the predicted key genes in these datasets may interact within network(s) and co-regulate DN pathogenesis.
The final 3 molecular markers screened were closely related to the initiation of inflammatory immune response. An immune homeostasis chemokine, CCL19, induces immune tolerance, T cell activation, and inflammatory response (35,36). A study has shown that CCL19 can promote the progression of DN by inhibiting the viability of HK-2 and HMC cells and promoting inflammation and fibrosis (37). As an antigen-presenting protein, CD1C can combine lipid and glycolipid antigens and submit them to T-cell receptors on NK-T cells to further activate the immune system (38). While CD1C is rarely expressed in normal kidneys, an increase in its expression has been significantly associated with renal interstitial immune cell infiltration and fibrosis (39,40). A member of the interleukin-1 family, IL33, can bind to IL1RL1/ST2 receptors to activate the NF-κB and MAPK signaling pathways in Th2 cells, and induce the secretion of type 2-related cytokines in T-helper cells (41). In addition, it targets regulatory T cells (Treg), T cells, B cells, and macrophages (42). Patients with diabetes, and those with DN, demonstrate a decrease in serum IL33 levels (43). Overexpression of IL33/ST2 promotes the production of TNF-ɑ and IL-6 in DN, and simultaneously promotes inflammation and fibrosis (44). Although the expression trend of CCL19 in this study was somewhat different from that observed in another study, the prediction model calculated by us emphasizes the integrity of a model as opposed to a single gene (37).
To ensure accuracy, 21 hub genes were obtained by intersecting the gene sets obtained by the two algorithms. In the PPI network, 21 DEGs with multiple interactions were revealed as the most significant hub genes. Further evaluations of these genes may help to elucidate the pathophysiology of DN. Moreover, CD1C, CD1D, CD28, CD86, and IL-7 were ranked as potential central regulatory proteins in DN, and are all involved in the activation and proliferation of T cells. A previous study demonstrated that 2 single nucleotide polymorphisms, CD28-rs3116494 and CD80-rs3850890, were associated with DN susceptibility (45). In addition, the number of CD4+ IL-17+ T cells was proportional to the degradation of eGFR in DN, as well as IL-17a, which is the key cytokine produced by this cell type (46). The IL-17a inhibitors reportedly improved renal dysfunction and disease progression in a murine model of DN by recovering the number of podocytes and inhibiting NF-κB/pro-inflammatory factors (47). Similarly, CD1C and CD1D have also been reported in DN. Thus, the above-mentioned results indicated that T cell activation and proliferation-related factors may be potential biomarkers for the early DN diagnosis. Renal fibrosis is the most typical manifestation of ESRD. Various pathogenic factors such as trauma, inflammation, blood circulation disorder, and immune response cause damage to kidney cells. In the late stage of DN, excessive collagen deposition and accumulation lead to hardening of the renal parenchyma, and ultimately, loss of renal function. Our GO analyses revealed that changes in DN were mainly associated with the positive regulation of cell adhesion, T cell activation, leukocyte proliferation and migration, urogenital system development, lymphocyte differentiation and proliferation, and mononuclear cell proliferation.
A study has demonstrated that the expression of vascular cell adhesion molecule-1 (VCAM-1) and intercellular adhesion molecule-1 (ICAM-1) increase significantly in DN (48). As an immunomodulator, ICAM-1 mediates contact and adhesion between different leukocyte subgroups through ligand interaction, and regulates the functional activity and immune response of leukocytes. The neutrophil-mediated phagocytosis, antigen recognition and T lymphocyte activation, killing of target cells, activation and differentiation of T lymphocytes to B lymphocytes, antibody formation, and other processes are related to ICAM-1 (49). Consistent with our predictions, microarray analysis of DN results from the mRNA datasets GSE30122, GSE47184, and GSE104948 identified cell adhesion as a main factor affecting the regulatory network in DN (50). In addition, more than 6 of 30 enriched signaling pathways were related to the progression of idiopathic pulmonary fibrosis (IPF), including the PI3K-Akt signaling pathway, MAPK signaling pathway, Rap1 signaling pathway, proteoglycan in cancer, cytokine-cytokine receptor interaction, and WNT signaling pathway. Notably, activation of PI3K-Akt signaling activates yes-associated protein to promote renal interstitial fibrosis (51). Moreover, preventing MAPK activation, which inhibits inflammation and apoptosis, can ameliorate renal fibrosis (52). Furthermore, inactivation of the WNT/β-catenin signaling pathway can inhibit podocyte apoptosis and DN progression (53). Overall, these findings are consistent with our data mining results.
We performed GSEA to study the biological function of immune-related DEGs associated with DN. Rheumatoid arthritis, Th1 and Th2 cell differentiation, and viral protein interaction with cytokine and cytokine receptor were the top 3 significantly enriched pathways. In this study, we found that the results of pathway enrichment were related to immune response and inflammation. Studies on murine models and patients with DN have demonstrated high levels of IL-6, IL-12, IL-4, IL-13, TNF-α, and interferon (IFN)-γ in DN (43,54). Similarly, a microarray analysis revealed that immune and inflammatory responses play a crucial role in the regulatory network of DN (55). Through data mining, we confirmed the importance of inflammation in DN progression.
While this research has improved our understanding of DN and immunity, it had certain limitations. First, our results have not been verified though in vivo/vitro experiments. Second, there is a lack of clinical data to validate our findings. Third, during analysis of multiple data sets, we could not mitigate batch-to-batch differences. In addition, although the model was validated internally, we lacked external data to validate the model. This will be one of subjects of our future research.
In conclusion, we comprehensively analyzed 3 data sets using bioinformatic tools and discussed the pathogenesis and potential molecular targets related to immune infiltration in DN. Moreover, we constructed an immune-related predictive model that facilitates the early diagnosis of DN and provides a basis for the assessment of DN prognosis. Of course, these should be validated in future molecular studies.
Acknowledgments
Funding: This work was supported by grants from the Guangxi Zhuang Autonomous Region Health Committee Program Project (No. Z20211334), Guangxi Zhuang Autonomous Region Traditional Chinese Medicine Administration Plan Project (No. GZZC2020252), and Baise Scientific Research and Technology Development Program Projects (No. BS20220920).
Footnote
Reporting Checklist: The authors have completed the TRIPOD reporting checklist. Available at https://atm.amegroups.com/article/view/10.21037/atm-22-1682/rc
Conflicts of Interest: All authors have completed the ICMJE uniform disclosure form (available at https://atm.amegroups.com/article/view/10.21037/atm-22-1682/coif). The authors have no conflicts of interest to declare.
Ethical Statement: The authors are accountable for all aspects of the work in ensuring that questions related to the accuracy or integrity of any part of the work are appropriately investigated and resolved. The study was conducted in accordance with the Declaration of Helsinki (as revised in 2013).
Open Access Statement: This is an Open Access article distributed in accordance with the Creative Commons Attribution-NonCommercial-NoDerivs 4.0 International License (CC BY-NC-ND 4.0), which permits the non-commercial replication and distribution of the article with the strict proviso that no changes or edits are made and the original work is properly cited (including links to both the formal publication through the relevant DOI and the license). See: https://creativecommons.org/licenses/by-nc-nd/4.0/.
References
- Umanath K, Lewis JB. Update on Diabetic Nephropathy: Core Curriculum 2018. Am J Kidney Dis 2018;71:884-95. [Crossref] [PubMed]
- Lim AKh. Diabetic nephropathy - complications and treatment. Int J Nephrol Renovasc Dis 2014;7:361-81. [Crossref] [PubMed]
- Cho NH, Shaw JE, Karuranga S, et al. IDF Diabetes Atlas: Global estimates of diabetes prevalence for 2017 and projections for 2045. Diabetes Res Clin Pract 2018;138:271-81. [Crossref] [PubMed]
- Chen Y, Lee K, Ni Z, et al. Diabetic Kidney Disease: Challenges, Advances, and Opportunities. Kidney Dis (Basel) 2020;6:215-25. [Crossref] [PubMed]
- Shao BY, Zhang SF, Li HD, et al. Epigenetics and Inflammation in Diabetic Nephropathy. Front Physiol 2021;12:649587. [Crossref] [PubMed]
- Pérez-Morales RE, Del Pino MD, Valdivielso JM, et al. Inflammation in Diabetic Kidney Disease. Nephron 2019;143:12-6. [Crossref] [PubMed]
- Perlman AS, Chevalier JM, Wilkinson P, et al. Serum Inflammatory and Immune Mediators Are Elevated in Early Stage Diabetic Nephropathy. Ann Clin Lab Sci 2015;45:256-63. [PubMed]
- Kwiendacz H, Nabrdalik K, Stompór T, et al. What do we know about biomarkers in diabetic kidney disease? Endokrynol Pol 2020;71:545-50. [Crossref] [PubMed]
- Pavkov ME, Weil EJ, Fufaa GD, et al. Tumor necrosis factor receptors 1 and 2 are associated with early glomerular lesions in type 2 diabetes. Kidney Int 2016;89:226-34. [Crossref] [PubMed]
- Bradley JR. TNF-mediated inflammatory disease. J Pathol 2008;214:149-60. [Crossref] [PubMed]
- Sabio G, Davis RJ. TNF and MAP kinase signalling pathways. Semin Immunol 2014;26:237-45. [Crossref] [PubMed]
- Yang Y, Kim SC, Yu T, et al. Functional roles of p38 mitogen-activated protein kinase in macrophage-mediated inflammatory responses. Mediators Inflamm 2014;2014:352371. [Crossref] [PubMed]
- Woroniecka KI, Park AS, Mohtat D, et al. Transcriptome analysis of human diabetic kidney disease. Diabetes 2011;60:2354-69. [Crossref] [PubMed]
- Na J, Sweetwyne MT, Park AS, et al. Diet-Induced Podocyte Dysfunction in Drosophila and Mammals. Cell Rep 2015;12:636-47. [Crossref] [PubMed]
- Ju W, Greene CS, Eichinger F, et al. Defining cell-type specificity at the transcriptional level in human disease. Genome Res 2013;23:1862-73. [Crossref] [PubMed]
- Grayson PC, Eddy S, Taroni JN, et al. Metabolic pathways and immunometabolism in rare kidney diseases. Ann Rheum Dis 2018;77:1226-33. [Crossref] [PubMed]
- Ritchie ME, Phipson B, Wu D, et al. limma powers differential expression analyses for RNA-sequencing and microarray studies. Nucleic Acids Res 2015;43:e47. [Crossref] [PubMed]
- Ito K, Murphy D. Application of ggplot2 to Pharmacometric Graphics. CPT Pharmacometrics Syst Pharmacol 2013;2:e79. [Crossref] [PubMed]
- Bhattacharya S, Dunn P, Thomas CG, et al. ImmPort, toward repurposing of open access immunological assay data for translational and clinical research. Sci Data 2018;5:180015. [Crossref] [PubMed]
- Gao CH, Yu G, Cai P. ggVennDiagram: An Intuitive, Easy-to-Use, and Highly Customizable R Package to Generate Venn Diagram. Front Genet 2021;12:706907. [Crossref] [PubMed]
- Kanehisa M, Goto S. KEGG: kyoto encyclopedia of genes and genomes. Nucleic Acids Res 2000;28:27-30. [Crossref] [PubMed]
- Yu G, Wang LG, Han Y, et al. clusterProfiler: an R package for comparing biological themes among gene clusters. OMICS 2012;16:284-7. [Crossref] [PubMed]
- Subramanian A, Kuehn H, Gould J, et al. GSEA-P: a desktop application for Gene Set Enrichment Analysis. Bioinformatics 2007;23:3251-3. [Crossref] [PubMed]
- Liberzon A, Birger C, Thorvaldsdóttir H, et al. The Molecular Signatures Database (MSigDB) hallmark gene set collection. Cell Syst 2015;1:417-25. [Crossref] [PubMed]
- Hänzelmann S, Castelo R, Guinney J. GSVA: gene set variation analysis for microarray and RNA-seq data. BMC Bioinformatics 2013;14:7. [Crossref] [PubMed]
- Szklarczyk D, Gable AL, Lyon D, et al. STRING v11: protein-protein association networks with increased coverage, supporting functional discovery in genome-wide experimental datasets. Nucleic Acids Res 2019;47:D607-13. [Crossref] [PubMed]
- Shannon P, Markiel A, Ozier O, et al. Cytoscape: a software environment for integrated models of biomolecular interaction networks. Genome Res 2003;13:2498-504. [Crossref] [PubMed]
- Osterman TJ, Terry M, Miller RS. Improving Cancer Data Interoperability: The Promise of the Minimal Common Oncology Data Elements (mCODE) Initiative. JCO Clin Cancer Inform 2020;4:993-1001. [Crossref] [PubMed]
- Chin CH, Chen SH, Wu HH, et al. cytoHubba: identifying hub objects and sub-networks from complex interactome. BMC Syst Biol 2014;8:S11. [Crossref] [PubMed]
- Chen B, Khodadoust MS, Liu CL, et al. Profiling Tumor Infiltrating Immune Cells with CIBERSORT. Methods Mol Biol 2018;1711:243-59. [Crossref] [PubMed]
- Friedman J, Hastie T, Tibshirani R. Regularization Paths for Generalized Linear Models via Coordinate Descent. J Stat Softw 2010;33:1-22. [Crossref] [PubMed]
- Sanz H, Valim C, Vegas E, et al. SVM-RFE: selection and visualization of the most relevant features through non-linear kernels. BMC Bioinformatics 2018;19:432. [Crossref] [PubMed]
- Van Calster B, Wynants L, Verbeek JFM, et al. Reporting and Interpreting Decision Curve Analysis: A Guide for Investigators. Eur Urol 2018;74:796-804. [Crossref] [PubMed]
- Deng Y, Li N, Wu Y, et al. Global, Regional, and National Burden of Diabetes-Related Chronic Kidney Disease From 1990 to 2019. Front Endocrinol (Lausanne) 2021;12:672350. [Crossref] [PubMed]
- Yan Y, Chen R, Wang X, et al. CCL19 and CCR7 Expression, Signaling Pathways, and Adjuvant Functions in Viral Infection and Prevention. Front Cell Dev Biol 2019;7:212. [Crossref] [PubMed]
- Westermann J, Nguyen-Hoai T, Baldenhofer G, et al. CCL19 (ELC) as an adjuvant for DNA vaccination: induction of a TH1-type T-cell response and enhancement of antitumor immunity. Cancer Gene Ther 2007;14:523-32. [Crossref] [PubMed]
- Sun J, Wang J, Lu W, et al. MiR-325-3p inhibits renal inflammation and fibrosis by targeting CCL19 in diabetic nephropathy. Clin Exp Pharmacol Physiol 2020;47:1850-60. [Crossref] [PubMed]
- Moody DB, Ulrichs T, Mühlecker W, et al. CD1c-mediated T-cell recognition of isoprenoid glycolipids in Mycobacterium tuberculosis infection. Nature 2000;404:884-8. [Crossref] [PubMed]
- Giuliani KTK, Kassianos AJ, Kildey K, et al. Role of inflammation and inflammasome activation in human bile cast nephropathy. Nephrology (Carlton) 2020;25:502-6. [Crossref] [PubMed]
- Kassianos AJ, Wang X, Sampangi S, et al. Increased tubulointerstitial recruitment of human CD141(hi) CLEC9A(+) and CD1c(+) myeloid dendritic cell subsets in renal fibrosis and chronic kidney disease. Am J Physiol Renal Physiol 2013;305:F1391-401. [Crossref] [PubMed]
- Schmitz J, Owyang A, Oldham E, et al. IL-33, an interleukin-1-like cytokine that signals via the IL-1 receptor-related protein ST2 and induces T helper type 2-associated cytokines. Immunity 2005;23:479-90. [Crossref] [PubMed]
- Cayrol C, Girard JP. Interleukin-33 (IL-33): A nuclear cytokine from the IL-1 family. Immunol Rev 2018;281:154-68. [Crossref] [PubMed]
- Anand G, Vasanthakumar R, Mohan V, et al. Increased IL-12 and decreased IL-33 serum levels are associated with increased Th1 and suppressed Th2 cytokine profile in patients with diabetic nephropathy (CURES-134). Int J Clin Exp Pathol 2014;7:8008-15. [PubMed]
- Elsherbiny NM, Said E, Atef H, et al. Renoprotective effect of calycosin in high fat diet-fed/STZ injected rats: Effect on IL-33/ST2 signaling, oxidative stress and fibrosis suppression. Chem Biol Interact 2020;315:108897. [Crossref] [PubMed]
- Li Y, Jin L, Yan J, et al. CD28 Genetic Variants Increase Susceptibility to Diabetic Kidney Disease in Chinese Patients with Type 2 Diabetes: A Cross-Sectional Case Control Study. Mediators Inflamm 2021;2021:5521050. [Crossref] [PubMed]
- Kuo HL, Huang CC, Lin TY, et al. IL-17 and CD40 ligand synergistically stimulate the chronicity of diabetic nephropathy. Nephrol Dial Transplant 2018;33:248-56. [Crossref] [PubMed]
- Lavoz C, Matus YS, Orejudo M, et al. Interleukin-17A blockade reduces albuminuria and kidney injury in an accelerated model of diabetic nephropathy. Kidney Int 2019;95:1418-32. [Crossref] [PubMed]
- Zhu Y, Ling Y, Wang X. Alantolactone mitigates renal injury induced by diabetes via inhibition of high glucose-mediated inflammatory response and macrophage infiltration. Immunopharmacol Immunotoxicol 2020;42:84-92. [Crossref] [PubMed]
- Lawson C, Wolf S. ICAM-1 signaling in endothelial cells. Pharmacol Rep 2009;61:22-32. [Crossref] [PubMed]
- Zhang Y, Li W, Zhou Y. Identification of hub genes in diabetic kidney disease via multiple-microarray analysis. Ann Transl Med 2020;8:997. [Crossref] [PubMed]
- Chen J, Wang X, He Q, et al. YAP Activation in Renal Proximal Tubule Cells Drives Diabetic Renal Interstitial Fibrogenesis. Diabetes 2020;69:2446-57. [Crossref] [PubMed]
- Malik S, Suchal K, Khan SI, et al. Apigenin ameliorates streptozotocin-induced diabetic nephropathy in rats via MAPK-NF-κB-TNF-α and TGF-β1-MAPK-fibronectin pathways. Am J Physiol Renal Physiol 2017;313:F414-22. [Crossref] [PubMed]
- Zhu H, Peng J, Li W. FOXA1 Suppresses SATB1 Transcription and Inactivates the Wnt/β-Catenin Pathway to Alleviate Diabetic Nephropathy in a Mouse Model. Diabetes Metab Syndr Obes 2021;14:3975-87. [Crossref] [PubMed]
- Li L, Zhang L, Chen D, et al. PEDF relieves kidney injury in type 2 diabetic nephropathy mice by reducing macrophage infiltration. Endokrynol Pol 2021;72:643-51. [Crossref] [PubMed]
- Fan XM, Huang CL, Wang YM, et al. Therapeutic Effects of Tangshen Formula on Diabetic Nephropathy in db/db Mice Using Cytokine Antibody Array. J Diabetes Res 2018;2018:8237590. [Crossref] [PubMed]