Benchmarking of 5 algorithms for high-resolution genotyping of human leukocyte antigen class I genes from blood and tissue samples
Introduction
Next-generation sequencing (NGS) is becoming an important tool for individualized therapeutic decision making in clinical oncology practice. The use of NGS could open up avenues for therapeutic options other than cytotoxic chemotherapy, which has had therapeutic success in treating some metastatic cancers, but has reached its therapeutic limit in treating others (1). Targeted NGS panels simultaneously interrogate 10s to 100s of genomic regions, and not only provide details of the actionable somatic mutation status in relation to a patient’s eligibility to receive targeted therapy, but also provide a genomic signature estimation, including the tumor mutation burden (TMB) and microsatellite instability (MSI), which can guide the use of immune checkpoint inhibitors (ICIs). TMB, MSI, deficient mismatch repair, and programmed cell death protein 1/programmed death ligand 1 status have been shown to be correlated with ICI response in some patients; however, clinical outcomes are still largely inconsistent with biomarker status (1-5). Thus, the search for other molecular biomarkers that accurately predict the ICI response continues.
Alterations in the human leukocyte antigen (HLA) are implicated in tumor invasion and development via the promotion of T lymphocyte-mediated immune escape mechanisms (6). Recent research has shown that specific alterations in HLA class I loci are associated with the clinical outcomes of ICIs (7-12), which increases the importance of accurate high-resolution HLA genotyping in immuno-oncology applications. The HLA gene complex encodes the major histocompatibility complex (MHC), which plays a major role in adaptive immunity by presenting pathogen-derived peptides to T lymphocytes (13,14). The HLA gene complex is located at the short arm of chromosome 6 (6p21.3), spans 3.6 megabase pairs, and can be divided into 3 regions (14,15). The class I region includes the classical, highly polymorphic HLA-A, HLA-B, and HLA-C genes, and the non-classical, more conserved, HLA-E, HLA-F, and HLA-G genes (13,14,16). Exons 2 and 3 of the HLA class I classical genes, which encode the binding sites for peptide antigens and lymphocyte receptors, are among the most polymorphic regions in the human genome (17). Currently, >21,000 alleles have been reported as classical HLA class I genes in the ImMunoGeneTics (IMGT) /HLA database (18).
According to the World Health Organization (WHO) HLA nomenclature, HLA allele names are composed of the HLA prefix and a letter indicating the specific gene locus separated by a hyphen, followed by an asterisk as a separator, followed by a series of unique numbers of up to 4 field levels assigned for each allele, which are separated by colons (e.g., HLA-A*02:101:01:02N) (19). The first 2 numbers after the asterisk, which are referred to as the first field, 2-digit, DNA-based, or low level of resolution, indicate the allele group and denote the serological antigen type of the allele (19). The next set of digits, which are referred to as the second field, 4-digit, or high level of resolution, indicate the specific HLA protein (19). The third and fourth set of numbers, which are also referred to as the 6- or 8-digit, respectively, the third or fourth field, respectively, or the allelic resolution genotype, indicate the synonymous DNA substitution within the coding region and DNA variations in the non-coding regions, respectively (19).
With its growing application, NGS allows higher throughput HLA genotyping, and has been instrumental in the discovery of novel point mutations in HLA class I genes (18,20-22). The earliest application of NGS for the high-resolution genotyping of HLA class I exons 2–3 had an accuracy of 96.4%, which indicated the reliability and efficiency of NGS (23). Over the years, various computational tools have been developed to classify HLA genotypes from NGS data in varying resolutions (22-28). NGS-based algorithms for HLA genotyping varies in their implementation of sequence alignment between the test and the reference sequences, which could affect their overall performance and accuracy in high resolution genotyping. For instance, algorithms such as HLA-HD, Polysolver, HLAscan, and OptiType use DNA-level sequence alignment, while some algorithms such as xHLA use protein-level sequence alignment (24-27,29). Despite the availability of numerous NGS-based algorithms, Sanger sequence-based typing (SBT) remains the gold-standard high-resolution HLA genotyping approach in clinical practice (30). Benchmarking studies against the gold standard are necessary to identify the NGS-based algorithm that could achieve comparable accuracy for high-resolution HLA genotyping as the gold standard. Moreover, the performance of the NGS-based algorithm should also be characterized using routine clinical samples such as tissue and blood samples. The best performing algorithm could then be incorporated into the NGS bioinformatics pipeline to enable simultaneous prediction of cancer-related somatic mutations and genomic signatures, including tumor mutation burden and HLA genotype for guiding targeted treatment decisions.
Our study aimed to identify the algorithm that enables the accurate, high-resolution genotyping of the classical HLA class I alleles sequenced by our targeted NGS panel. To achieve this aim, we retrieved the NGS data derived from paired white blood cell (WBC), plasma, and tissue DNA samples of 22 cancer patients with known HLA genotypes examined using Sanger SBT. The HLA genotypes from the NGS data were generated using 5 different computational tools at the first-field (low) and the second-field (high) levels of resolution. We then evaluated the concordance between the SBT-based and the NGS-based HLA genotype using the 5 computational tools. We present the following article in accordance with the STARD reporting checklist (available at https://atm.amegroups.com/article/view/10.21037/atm-22-875/rc).
Methods
Patient samples
The sample size was randomly selected based on the availability of SBT-based HLA genotype and paired WBC, plasma, and tissue samples submitted for sequencing using the 520 gene-panel. In total, 88 sequencing data were retrieved for 22 cancer patients from China-Japan Union Hospital of Jilin University with paired WBC, plasma, and tissue samples. The WBC samples were submitted for SBT-based HLA genotyping to serve as the reference, while paired plasma, WBC, and tissue samples were sequenced using a commercially available targeted panel covering 520 cancer-related genes (OncoScreen Plus, Burning Rock Biotech, Guangzhou, China) on a paired-end sequencing instrument (Nextseq 500, Illumina, CA, USA). The NGS-targeted panel covered all the exons of HLA-A, HLA-B, and HLA-C. The NGS processing and analysis were performed according to optimized protocols at the College of American Pathologists (CAP)-accredited and Clinical Laboratory Improvement Amendments (CLIA)-certified facility of Burning Rock Biotech. The study was conducted in accordance with the Declaration of Helsinki (as revised in 2013). This study was approved by the Institutional Ethics Committee of China-Japan Union Hospital of Jilin University (approval number: 2016-wjw015). Written informed consent was provided by all the patients before their inclusion in the study.
HLA typing algorithms
We selected and compared 5 publicly available computational tools, including HLA-HD (25), HLAscan (26), OptiType (29), Polysolver (24), and xHLA (27). All the algorithms were downloaded from their respective online sources, and implemented under default parameters. As some algorithms could only predict up to 4-digit resolution, all the HLA alleles generated by the 5 algorithms were normalized at the second-field level of resolution. Following the WHO HLA nomenclature (19), a 2-digit HLA classification was defined as low resolution, while a 4-digit HLA classification was defined as high resolution.
Statistical analysis
Accuracy was the performance metric used to evaluate the HLA typing algorithms, which represents the concordance between SBT-based and NGS-based HLA genotypes, and was computed as the fraction of all the alleles that were concordant with the reference (SBT-based genotype) over the total number of alleles for all alleles and all samples as shown by the formula below.
Results
Concordance of the 5 HLA typing algorithms with SBT for WBC samples
We first evaluated the concordance between the Sanger SBT-derived HLA genotypes and the NGS-based HLA genotypes classified by the 5 algorithms for the WBC samples from the 22 patients. As Figure 1 and Table 1 show, all 5 algorithms were highly accurate at classifying HLA class I alleles, HLA-A, HLA-B, and HLA-C at low resolution. However, despite having >90% overall accuracy, the accuracy of these algorithms was more varied at high resolution (see Figure 1A and Table 1). For high-resolution genotyping, HLA-HD had the highest accuracy at 99.2%, while xHLA had the lowest accuracy at 91.7% (see Figure 1A and Table 1). Of the classical HLA class I alleles, the high-resolution HLA-A genotyping had the most fluctuations, with HLA-HD achieving 100% accuracy, while xHLA was the least accurate at 79.6% (see Figure 1B and Table 1). For high-resolution HLA-B genotyping, HLA-HD consistently achieved 100% accuracy, while HLAscan was the least accurate at 93.2% (see Figure 1C and Table 1). For high-resolution HLA-C genotyping, xHLA achieved 100% accuracy, while Polysolver, OptiType, and HLAscan had a similar accuracy of 95.5% (see Figure 1D and Table 1).
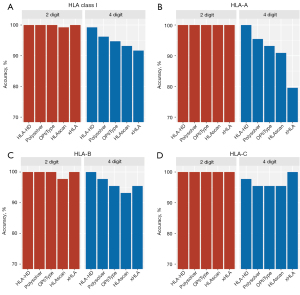
Table 1
Algorithm | Low resolution (2-digit/1st field) (%) | High resolution (4-digit/2nd field) (%) | |||||||
---|---|---|---|---|---|---|---|---|---|
HLA-A | HLA-B | HLA-C | HLA class I | HLA-A | HLA-B | HLA-C | HLA class I | ||
HLA-HD | 100.0 | 100.0 | 100.0 | 100.0 | 100.0 | 100.0 | 97.7 | 99.2 | |
Polysolver | 100.0 | 100.0 | 100.0 | 100.0 | 95.5 | 97.7 | 95.5 | 96.2 | |
OptiType | 100.0 | 100.0 | 100.0 | 100.0 | 93.1 | 95.5 | 95.5 | 94.7 | |
HLAscan | 100.0 | 97.7 | 100.0 | 99.2 | 90.9 | 93.2 | 95.5 | 93.2 | |
xHLA | 100.0 | 100.0 | 100.0 | 100.0 | 79.6 | 95.5 | 100.0 | 91.7 |
HLA, human leukocyte antigen; WBC, white blood cell.
Taken together, these data indicate that the performance of the 5 HLA typing algorithms was comparable at low resolution, but differed at high resolution, particularly in the classification of HLA-A alleles. Of the 5 algorithms, HLA-HD was the most accurate at the high-resolution HLA genotyping of the WBC samples.
HLA genotyping using different sample types
To examine the performance of the 5 algorithms for different sample types, we used NGS data derived from paired WBC, plasma, and tissue DNA samples from the same 22 patients with known HLA genotypes. Consistent with the WBC data, the 5 algorithms were similarly accurate at the low-resolution genotyping of HLA class I alleles from plasma samples, with HLA-HD, xHLA, and HLAscan achieving 100%, 100%, and 99.2% accuracy, respectively (see Figure 2). The genotyping of the HLA class I alleles from tissue samples was less accurate than that of the paired blood samples; however, the accuracy was within an acceptable range even at high resolution (range 87.9–96.2%; see Table S1). HLA-HD consistently achieved the highest accuracy for the high-resolution HLA genotyping of WBC, plasma, and tissue samples (see Figure 2). For tissue samples, HLA-HD achieved 96.2% accuracy and generated discordant calls for 5 alleles from 3 patients (see Tables S1,S2).
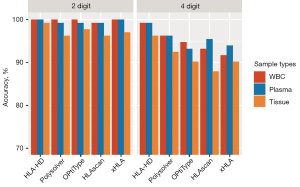
Taken together, these results indicate that the algorithms performed consistently well in both the low- and high-resolution genotyping of the plasma and WBC samples. Their performance in relation to tissue samples was less accurate, but had a similar pattern as that of the WBC samples. The performance of HLA-HD remained consistent in the high-resolution HLA genotyping of plasma and tissue samples.
Effect of sequencing depth on high-resolution HLA genotyping
We next explored the effect of different sequencing depths on the performance of the 5 algorithms in the high-resolution HLA genotyping of the three different sample types. The actual sequencing depth ranged between 6,488× and 8,766× for the WBC samples, between 6,102× and 20,231 for the plasma samples, and between 1,007× and 1,863× for the tissue samples. For this in-silico analysis, the sequencing data from the paired WBC, plasma, and tissue samples were down-sampled to simulate lower sequencing depths at a range of 50× to 6,000× for the WBC and plasma samples, and a range of 50× to 1,000× for the tissue samples. Among the 5 algorithms, HLA-HD consistently achieved 99.2% accuracy for the WBC samples sequenced at 6,000× to 300× (see Figure 3A). At 100×, all the algorithms were approximately 90% accurate. At 50×, HLA-HD still achieved 93.9% accuracy, while xHLA was the most accurate at 97.7%, and HLAscan was the least accurate at 85.6% (see Figure 3A). In the plasma samples, HLA-HD achieved >97% accuracy, and was the most accurate at sequencing depths ranging from 6,000× to 700× (see Figure 3B). All 5 algorithms demonstrated approximately 90% accuracy at 700×. Consistent with the WBC samples, xHLA was the most accurate (93.2%) and HLAscan was the least accurate (73.4%) at 50× for plasma samples (see Figure 3B). For the tissue samples, all 5 algorithms also demonstrated approximately 90% accuracy from 1,000× to 100× (see Figure 3C). Among them, the accuracy of HLA-HD was consistently high, and achieved up to 94.2% accuracy even at 50×. At 50× for the tissue samples, xHLA was the most accurate (94.7%) and HLAscan was the least accurate (84.9%) (see Figure 3C).
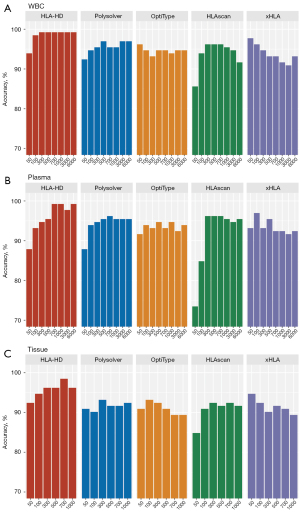
Taken together, these data indicate the accuracy and robustness of all the algorithms in the high-resolution HLA genotyping of all the sample types at a sequencing depth of at least 300×. HLA-HD was consistently accurate even at a sequencing depth of 50× for all sample types, but showed a direct association with the sequencing depth, achieving its highest accuracy at sequencing depths of ≥300×. Notably, the performance of xHLA was not affected by sequencing depths and even yielded better accuracy at lower depths (≤100×) than higher depths (≥300×).
Identifying the discordant HLA genotypes among the 5 algorithms
To further understand the limitations of each algorithm, we also analyzed the specific HLA alleles from various sample types that were discordantly classified by the 5 algorithms. Figure 4 summarizes the genotyping results, which revealed the discordant HLA genotypes in the WBC samples. Of the HLA class I genes, HLA-A had the most discordant results. Among the 5 algorithms, xHLA was the least accurate in HLA-A where it misclassified 4 patients with A*02:06 as A*02:01, and 2 patients with A*02:07 as A*02:474. Only xHLA misclassified both allele pairs from 3 patients (HLA-A1 and HLA-A2; see Figure 4). All the other discordant calls from the other 4 algorithms only occurred in 1 allele. HLA-HD accurately classified all the alleles except HLA-C1, whereby the HLA-C*04:82 of patient P14 was misclassified as C*04:01. Notably, only xHLA correctly classified this HLA-C1 allele. The 3 algorithms, Polysolver, OptiType, and HLAscan, similarly misclassified HLA-B*15:19 as B*15:12 for patient P18. HLA-C*07:18 for patient P20 was misclassified as C*07:01 by OptiType and HLAscan, and as C*07:06 by Polysolver. HLA-A*02:346, a rare HLA-A allele detected in patient P09, was misclassified as the most common A*02:01 allele by Polysolver and OptiType, and as A*02:09 by HLAscan but was correctly classified by both HLA-HD and xHLA.
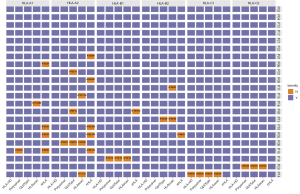
Consistent with the WBC data, HLA-C*04:82 was the most discordant allele in plasma samples, which was misclassified as C*04:01 by 4 algorithms except xHLA. Polysolver, OptiType, and HLAscan were consistent in the misclassification of HLA-A*02:346 as either A*02:01 or A*02:09 for patient P09, HLA-B*15:19 as B*15:12 for patient P18, and HLA-C*07:18 as either C*07:01 or C*07:06 for patient P20 (see Figure S1A).
For the tissue samples, HLA-C*04:82 from patient P14 remained the most discordant allele, and was misclassified as C*04:01 by all 5 algorithms (see Figure S1B). Consistent with the misclassification of HLA-A*02:346, HLA-B*15:19, and HLA-C*07:18 in the WBC and plasma samples of 3 patients, Polysolver, OptiType, HLAscan, and xHLA all misclassified HLA-B*39:01 as B*51:01 for patient P22 (see Figure S1B). Among the tissue samples, tissue samples from patient P22 had the most discordant results due to the poor sequencing quality, and below average library complexity.
We further investigated the reason for the discordant results for HLA-C*04:82 from patient P14. As Figure 5A shows, a 6-nucleotide sequence TGT CCT was repeatedly interspersed in the DNA sequence of the C*04:01 and C*04:82 alleles. In addition to the similarity between the 2 alleles, C*04:82 had an additional 9-nucleotide repeated sequence (TGT CCT AGC) in positions 957-965 and 966-974 of exon 5, which was only present once in C*04:01 (see Figure 5A). The SBT results showed heterozygous HLA-C*04:82 and C*08:01 alleles for P14 (see Figure 5B and Table S2). This highly repetitive region was correctly genotyped by xHLA in the plasma and WBC samples, but not in the tissue samples. All the other 4 algorithms consistently misclassified this allele in all sample types.
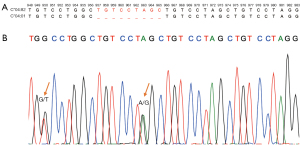
We also investigated the discordant results for HLA-A*02:346 from patient P09. As Figure S2A shows, the position 268 for A*02:01, A*02:346, and A*02:09 only differed in 1 nucleotide. The sequencing electropherogram also demonstrated overlapping A and C base calls for this position, indicating the heterozygous status of the 2 HLA-A alleles (see Figure S2B). The heterozygosity of this patient was correctly genotyped by HLA-HD and xHLA, but was misclassified as A*02:01 by Polysolver and OptiType, and as A*02:09 by HLAscan (see Figure S2C).
Taken together, these results indicate the robust performance of HLA-HD in the high-resolution HLA genotyping of various sample types.
Discussion
NGS typically generates short read lengths (between 150–250 bases for single-end sequencing and up to 400–500 bases for paired-end sequencing), and is highly dependent on bioinformatics tools to assemble the random sequencing reads into contiguous data (22). This poses a computational challenge to the accurate analysis of classical HLA class I loci from NGS sequencing data due to the extensive sequence similarity among different alleles. Computational tools for HLA typing use varied statistical approaches to align the sequence reads/read pairs to reference sequences from databases, such as the IMGT/HLA, for allele classification (24-27,31,32). Routine HLA typing only sequences the core exons 2 and 3 of classic HLA class I genes, which are reflected by the missing or unverified sequence information for intronic or other exonic regions other than the core exons in >90% of the entries on the IMGT/HLA database (29,32). For more precise genotyping from shorter read fragments, some computational tools can reconstruct the missing parts from the reference sequence by imputing from the partial sequences of other alleles within a phylogenetic region (26,27,29,32).
Kawaguchi et al. classified HLA typing algorithms into two categories (i.e., restricted and unrestricted) based on how they use the HLA information (25). HLA-HD and Polysolver are considered unrestricted, and scan for variations throughout the entire HLA gene, while OptiType is considered restricted, as its use is limited to exons, primers, and probes only (24,25,29). HLAscan performs alignment for exons 2, 3, 4, and 5 for HLA class I genes (26). Most algorithms use the DNA-level alignment method, but xHLA uses the protein-level alignment method (27). This varied approach for sequence alignment could introduce ambiguities and affect the classification accuracy of major and minor HLA genotypes. Another advantage of the NGS approach over the SBT approach is the phase resolution for heterozygous alleles. NGS-based computational tools assemble the paired-sequence reads into separate alleles corresponding to the haplotype, which provides a more accurate genotyping of heterozygous alleles than the ambiguous allele phasing of the Sanger sequencing (21). Numerous efforts have been made to develop and benchmark various HLA typing algorithms (24-27,29,31-35); however, most studies evaluate genotyping performance using NGS data from publicly available whole genome/whole exome datasets or WBCs. Indeed, few studies have comprehensively evaluated the performance of these algorithms using various sample types, particularly plasma and tissue samples that are routinely submitted for NGS-based analysis in clinical oncology practice. Thus, we set out to benchmark 5 algorithms against the gold standard to identify the most accurate algorithm for HLA genotyping of paired WBC, plasma, and tissue samples.
We found that the 5 algorithms were comparably accurate in the low-resolution HLA genotyping of blood and tissue samples; however, their performance varied for high-resolution genotyping. All 5 algorithms achieved >90% accuracy in blood-based high-resolution HLA genotyping; however, their performance for tissue-based genotyping was inferior. For the tissue-based high-resolution HLA genotyping, the accuracy was >90% for HLA-HD and Polysolver, ~90% for Optitype and xHLA, and <90% for HLAscan. The lower accuracy for the tissue samples was due to the use of formalin-fixed, paraffin-embedded (FFPE) tissue samples in the sequencing, which was exemplified by the 2 discordant alleles from each of the 2 tissue samples from P15 and P22 by HLA-HD (see Table S2). Notably, HLA-HD was able to report the correct genotype for 1 of the polymorphic allele pair and only misclassified the other allele. This pattern was also observed in other algorithms except that of xHLA, which appeared to have a bias for major alleles.
As at times, HLA alleles only vary at the single-nucleotide level, some changes brought about by FFPE processing, such as DNA fragmentation or the presence of nucleotide changes, might have contributed to the lower sequencing accuracy in this sample type. A previous study demonstrated the amplification of exons 2, 3, and 4 of HLA-A in only 88% (14/16) from DNA isolated from 10-year-old FFPE tissue samples (36). Among the 5 algorithms included in our study, the performance of HLA-HD was consistent for the WBC, plasma, and tissue samples, which were highly concordant with the SBT-based HLA genotype. HLA-HD was highly accurate at the sequencing depth of 50×; however, its accuracy showed a pattern of linear association with sequencing depth, such that its peak performance occurred at ≥300× with a corresponding drop in accuracy as the sequencing depth decreased. Conversely, the performance of xHLA was stable across a range of sequencing depths and even achieved better accuracy at lower depths (≤100×) than higher sequencing depths (≥700×). At sequencing depths of ≥300×, all the algorithms were ≥90% accurate in high-resolution HLA typing. Our observations on the effect of sequencing depth on the accuracy of the algorithms were consistent with those of previous reports (26,32-34). Notably, the actual sequencing depths during routine targeted sequencing runs for WBC and plasma samples are within 10,000× and do not reach below 300×. Conversely, in this study, the actual sequencing depth for the tissue samples was within 1,000×.
It should also be noted that our study used the gold-standard SBT-based HLA genotype to benchmark the 5 HLA typing algorithms. The gold-standard benchmarking of the performance of the algorithms indicates the stringency of our study and the accuracy of our findings. Benchmarking using the most concordant HLA genotype is a widely used method for comparing algorithm performance; however, the most concordant allele might not be the correct genotype. This scenario is best exemplified by HLA-C*04:82, the SBT-based genotype of P14, which was misclassified as HLA-C*04:01 by 4 algorithms for the paired WBC and plasma samples and by all 5 algorithms for the tissue samples. Genotyping of this particular allele was challenging due to the repetitive nature of this loci (see Figure 5). Figure 4 and Figure S1 provide more examples of concordant alleles among the algorithms that were discordant with the SBT-based genotype.
Consistent with our findings on HLA-HD, a recently published study examined the accuracy and robustness of the HLA-HD and HISAT genotype in high-resolution HLA typing among 7 algorithms (33). This study mentioned that both the HLA-HD and HISAT genotype had higher requirements for computer resources (33). The accuracy of HLA-HD compensated for the time the HLA typing took. Additionally, since our targeted panel included all exons of classical HLA class I genes, the inherent features of HLA-HD in scanning the full gene were enforced to ensure precise and robust HLA genotyping. Thus, we are of the view that HLA-HD is the most optimal algorithm for accurate high-resolution NGS-based HLA typing for our application.
Previous studies have established the role of HLA alterations in cytotoxic T lymphocyte-mediated immune escape mechanisms that promote cancer development (6,37). Homozygosity in the HLA class I gene even at a single HLA locus and the loss of heterozygosity (LOH) of the HLA class I gene, particularly in patients with a low mutation burden, was shown to be significantly associated with reduced overall survival (7). HLA LOH, which occurs in ~40% of non-small-cell lung cancers (NSCLC), was previously reported to be associated with an elevated neoantigen burden and to contribute to mediating the immune evasion mechanism in lung cancer development (38). Additionally, patients with advanced-stage melanoma harboring the germline HLA-B44 supertype who received anti-CTLA4 therapy had significantly better overall survival than those with the HLA-B62 supertype, who had inferior outcomes (7). Compared to HLA-B44 generally, specific HLA-B alleles that bring about radical glutamic acid substitutions in the anchor position of B44 were associated with a poor prognosis and better clinical outcomes with ICIs in NSCLC (11). Specific HLA-A alleles (A*02:01 and A*24:02) were also associated with the prognosis of metastatic hormone-sensitive prostate cancer (8). Studies on the implications of the HLA genotype in the immunotherapy response in other solid tumor types are also increasing (12,39). These reports raise the relevance of accurate high-resolution HLA genotyping in cancer immunotherapy applications. The inclusion of the most accurate algorithm into the variant calling pipelines for targeted NGS panels could enable high-resolution HLA genotyping at no additional cost.
Our study is limited by its retrospective nature, which limited the number of patients with available NGS data for paired blood and tissue samples and the information available on the SBT-based HLA genotype. The tissue samples that we analyzed were FFPE samples, which might have contributed to the lower accuracy of the HLA genotyping in the tissues compared to the blood samples. Our study did not include the benchmarking for HLA class II genes due to the selection of some algorithms that were only designed for the genotyping of classical HLA class I genes (i.e., OptiType). We speculate that the performance of these algorithms, particularly HLA-HD, can be applied to other targeted panels designed to interrogate HLA class I and class II loci at a sequencing depth higher than 300× for blood and tissue samples. Another limitation is the selection of the 5 HLA typing algorithms based on published reports, which introduced selection bias. The HLA-HD showed consistent performance across the sample types, but the optimization of the prediction parameters might further improve its accuracy.
Conclusions
Our study demonstrates the accuracy and robustness of HLA-HD in the high-resolution genotyping of classical HLA class I loci from WBC, plasma, and tissue samples sequenced by our targeted NGS panel. Our study contributes to the growing knowledge that sample types, NGS sequencing conditions, and the choice of computational tools are important factors for accurate high-resolution HLA genotyping. Our study also highlights the importance of targeted NGS in providing a comprehensive profile of somatic mutations and genomic signatures that are crucial in identifying cancer patients who would likely benefit from both targeted therapies and immunotherapy.
Acknowledgments
The authors would like to thank all the patients and their families for their cooperation and support. The authors would also like to thank the investigators, study coordinators, operation staff, and the whole project team who worked on this project, particularly Drs. Analyn Lizaso, Jian Wang, Jianxing Xiang, Bing Li, and Zhou Zhang of Burning Rock Biotech for their support.
Funding: This study was supported by grants from the Science and Technology Project of Xiamen City (No. 3502Z20199006 to JL), and the Medical Science Foundation of Guangdong Province (No. A2019347 to BY). The funders had no role in the conceptualization or design of the study, data collection, analysis, decision to publish, or preparation of the manuscript.
Footnote
Reporting Checklist: The authors have completed the STARD reporting checklist. Available at https://atm.amegroups.com/article/view/10.21037/atm-22-875/rc
Data Sharing Statement: Available at https://atm.amegroups.com/article/view/10.21037/atm-22-875/dss
Conflicts of Interest: All authors have completed the ICMJE uniform disclosure form (available at https://atm.amegroups.com/article/view/10.21037/atm-22-875/coif). JL reports that this study was supported by grants from the Science and Technology Project of Xiamen City (No. 3502Z20199006). BY reports that this study was supported by the Medical Science Foundation of Guangdong Province (No. A2019347). The other authors have no conflicts of interest to declare.
Ethical Statement: The authors are accountable for all aspects of the work in ensuring that questions related to the accuracy or integrity of any part of the work are appropriately investigated and resolved. The study was conducted in accordance with the Declaration of Helsinki (as revised in 2013). This study was approved by the Institutional Ethics Committee of China-Japan Union Hospital of Jilin University (approval number: 2016-wjw015). Written informed consent was obtained from all the participants included in the study.
Open Access Statement: This is an Open Access article distributed in accordance with the Creative Commons Attribution-NonCommercial-NoDerivs 4.0 International License (CC BY-NC-ND 4.0), which permits the non-commercial replication and distribution of the article with the strict proviso that no changes or edits are made and the original work is properly cited (including links to both the formal publication through the relevant DOI and the license). See: https://creativecommons.org/licenses/by-nc-nd/4.0/.
References
- Groisberg R, Subbiah V. Immunotherapy and next-generation sequencing guided therapy for precision oncology: What have we learnt and what does the future hold? Expert Rev Precis Med Drug Dev 2018;3:205-13. [Crossref] [PubMed]
- Apetoh L, Ladoire S, Coukos G, et al. Combining immunotherapy and anticancer agents: the right path to achieve cancer cure? Ann Oncol 2015;26:1813-23. [Crossref] [PubMed]
- Kruger S, Ilmer M, Kobold S, et al. Advances in cancer immunotherapy 2019 - latest trends. J Exp Clin Cancer Res 2019;38:268. [Crossref] [PubMed]
- Gong J, Chehrazi-Raffle A, Reddi S, et al. Development of PD-1 and PD-L1 inhibitors as a form of cancer immunotherapy: a comprehensive review of registration trials and future considerations. J Immunother Cancer 2018;6:8. [Crossref] [PubMed]
- Waldman AD, Fritz JM, Lenardo MJ. A guide to cancer immunotherapy: from T cell basic science to clinical practice. Nat Rev Immunol 2020;20:651-68. [Crossref] [PubMed]
- Garrido F, Ruiz-Cabello F, Cabrera T, et al. Implications for immunosurveillance of altered HLA class I phenotypes in human tumours. Immunol Today 1997;18:89-95. [Crossref] [PubMed]
- Chowell D, Morris LGT, Grigg CM, et al. Patient HLA class I genotype influences cancer response to checkpoint blockade immunotherapy. Science 2018;359:582-7. [Crossref] [PubMed]
- Stokidis S, Fortis SP, Kogionou P, et al. HLA Class I Allele Expression and Clinical Outcome in De Novo Metastatic Prostate Cancer. Cancers (Basel) 2020;12:1623. [Crossref] [PubMed]
- Wang C, Xiong C, Hsu YC, et al. Human leukocyte antigen (HLA) and cancer immunotherapy: HLA-dependent and -independent adoptive immunotherapies. Ann Blood 2020;5:14. [Crossref]
- Kim CJ, Parkinson DR, Marincola F. Immunodominance across HLA polymorphism: implications for cancer immunotherapy. J Immunother 1998;21:1-16. [Crossref] [PubMed]
- Cummings AL, Gukasyan J, Lu HY, et al. Mutational landscape influences immunotherapy outcomes among patients with non-small-cell lung cancer with human leukocyte antigen supertype B44. Nat Cancer 2020;1:1167-75. [Crossref] [PubMed]
- Schaafsma E, Fugle CM, Wang X, et al. Pan-cancer association of HLA gene expression with cancer prognosis and immunotherapy efficacy. Br J Cancer 2021;125:422-32. [Crossref] [PubMed]
- Klein J, Sato A. The HLA system. First of two parts. N Engl J Med 2000;343:702-9. [Crossref] [PubMed]
- Horton R, Wilming L, Rand V, et al. Gene map of the extended human MHC. Nat Rev Genet 2004;5:889-99. [Crossref] [PubMed]
- Complete sequence and gene map of a human major histocompatibility complex. The MHC sequencing consortium. Nature 1999;401:921-3. [Crossref] [PubMed]
- Bjorkman PJ, Saper MA, Samraoui B, et al. The foreign antigen binding site and T cell recognition regions of class I histocompatibility antigens. Nature 1987;329:512-8. [Crossref] [PubMed]
- Robinson J, Guethlein LA, Cereb N, et al. Distinguishing functional polymorphism from random variation in the sequences of >10,000 HLA-A, -B and -C alleles. PLoS Genet 2017;13:e1006862. [Crossref] [PubMed]
- Robinson J, Barker DJ, Georgiou X, et al. IPD-IMGT/HLA Database. Nucleic Acids Res 2020;48:D948-55. [PubMed]
- Nunes E, Heslop H, Fernandez-Vina M, et al. Definitions of histocompatibility typing terms. Blood 2011;118:e180-3. [Crossref] [PubMed]
- Smith AG, Pereira S, Jaramillo A, et al. Comparison of sequence-specific oligonucleotide probe vs next generation sequencing for HLA-A, B, C, DRB1, DRB3/B4/B5, DQA1, DQB1, DPA1, and DPB1 typing: Toward single-pass high-resolution HLA typing in support of solid organ and hematopoietic cell transplant programs. HLA 2019;94:296-306. [Crossref] [PubMed]
- Klasberg S, Surendranath V, Lange V, et al. Bioinformatics Strategies, Challenges, and Opportunities for Next Generation Sequencing-Based HLA Genotyping. Transfus Med Hemother 2019;46:312-25. [Crossref] [PubMed]
- Wang C, Krishnakumar S, Wilhelmy J, et al. High-throughput, high-fidelity HLA genotyping with deep sequencing. Proc Natl Acad Sci U S A 2012;109:8676-81. [Crossref] [PubMed]
- Erlich RL, Jia X, Anderson S, et al. Next-generation sequencing for HLA typing of class I loci. BMC Genomics 2011;12:42. [Crossref] [PubMed]
- Shukla SA, Rooney MS, Rajasagi M, et al. Comprehensive analysis of cancer-associated somatic mutations in class I HLA genes. Nat Biotechnol 2015;33:1152-8. [Crossref] [PubMed]
- Kawaguchi S, Higasa K, Shimizu M, et al. HLA-HD: An accurate HLA typing algorithm for next-generation sequencing data. Hum Mutat 2017;38:788-97. [Crossref] [PubMed]
- Ka S, Lee S, Hong J, et al. HLAscan: genotyping of the HLA region using next-generation sequencing data. BMC Bioinformatics 2017;18:258. [Crossref] [PubMed]
- Xie C, Yeo ZX, Wong M, et al. Fast and accurate HLA typing from short-read next-generation sequence data with xHLA. Proc Natl Acad Sci U S A 2017;114:8059-64. [Crossref] [PubMed]
- Hosomichi K, Shiina T, Tajima A, et al. The impact of next-generation sequencing technologies on HLA research. J Hum Genet 2015;60:665-73. [Crossref] [PubMed]
- Szolek A, Schubert B, Mohr C, et al. OptiType: precision HLA typing from next-generation sequencing data. Bioinformatics 2014;30:3310-6. [Crossref] [PubMed]
- Adams SD, Barracchini KC, Simonis TB, et al. High throughput HLA sequence-based typing (SBT) utilizing the ABI Prism 3700 DNA Analyzer. Tumori 2001;87:S40-3. [Crossref] [PubMed]
- Matey-Hernandez MLDanish Pan Genome Consortium. Benchmarking the HLA typing performance of Polysolver and Optitype in 50 Danish parental trios. BMC Bioinformatics 2018;19:239. [Crossref] [PubMed]
- Bauer DC, Zadoorian A, Wilson LOW, et al. Evaluation of computational programs to predict HLA genotypes from genomic sequencing data. Brief Bioinform 2018;19:179-87. [PubMed]
- Liu P, Yao M, Gong Y, et al. Benchmarking the Human Leukocyte Antigen Typing Performance of Three Assays and Seven Next-Generation Sequencing-Based Algorithms. Front Immunol 2021;12:652258. [Crossref] [PubMed]
- Yi J, Chen L, Xiao Y, et al. Investigations of sequencing data and sample type on HLA class Ia typing with different computational tools. Brief Bioinform 2021;22:bbaa143.
- Li X, Zhou C, Chen K, et al. Benchmarking HLA genotyping and clarifying HLA impact on survival in tumor immunotherapy. Mol Oncol 2021;15:1764-82. [Crossref] [PubMed]
- Villabona L, Leon Rodriguez DA, Andersson EK, et al. A novel approach for HLA-A typing in formalin-fixed paraffin-embedded-derived DNA. Mod Pathol 2014;27:1296-305. [Crossref] [PubMed]
- Jiménez P, Cantón J, Collado A, et al. Chromosome loss is the most frequent mechanism contributing to HLA haplotype loss in human tumors. Int J Cancer 1999;83:91-7. [Crossref] [PubMed]
- McGranahan N, Rosenthal R, Hiley CT, et al. Allele-Specific HLA Loss and Immune Escape in Lung Cancer Evolution. Cell 2017;171:1259-1271.e11. [Crossref] [PubMed]
- Anderson P, Aptsiauri N, Ruiz-Cabello F, et al. HLA class I loss in colorectal cancer: implications for immune escape and immunotherapy. Cell Mol Immunol 2021;18:556-65. [Crossref] [PubMed]
(English Language Editor: L. Huleatt)