Development and validation of a nomogram based on the hospital information system for quantitative assessment of the risk of cardiocerebrovascular complications of diabetes
Introduction
Diabetes mellitus is an independent risk factor for cardiocerebrovascular disease (CCVD): 50–80% of patients with type 2 DM (T2DM) are often complicated with macrovascular disease, and >70% of diabetic patients eventually die of cardiocerebrovascular complications (CCVCs) (1,2). Therefore, the prevention and treatment of CCVCs has generated much interest and researchers have identified risk factors such as obesity, hypertension, dyslipidemia, smoking, and family history (3). The guidelines for diabetes prevention and treatment formulated in China and those formulated by the American Diabetes Association (ADA) both recommend adopting a comprehensive plan to prevent and treat the CCVCs of T2DM based on lifestyle interventions, and comprehensive assessment and management of the multiple risk factors for these CCVCs. Lifestyle interventions for diabetic patients include self-management education, nutritional therapy, exercise therapy, smoking cessation, and psychosocial interventions. Managing the clinical risk factors of T2DM includes managing blood pressure (BP) and blood lipids, antiplatelet therapy, and individualized treatment of cardiovascular disease (CVD) (4,5).
Although preventive and treatment measures for CCVCs of T2DM have been established, these complications still have a high incidence and low control rates (6). Diabetes is a lifelong chronic disease. As patients’ physical functions decline due to their increased age, the management of diabetes is challenging (7). In addition, as long-term lifestyle interventions in diabetic patients continue, patients’ perception of risk factors can decrease due to a lack of tools to objectively measure these factors, resulting in decreased motivation and compliance (8). These two problems interact synergistically, significantly affecting the management of CCVCs of T2DM.
Therefore, a tool that quantitatively evaluates the risk of the CCVCs of T2DM will dynamically assess changes in the risk of complications to guide clinical treatment, and improve patient compliance through intuitive and visual risk evaluation. Nomograms have been used to predict the risks of developing T2DM and the complications of diabetic nephropathy (9,10). Hochster and Niedzwiecki confirmed that big-data accumulation effectively improves the accuracy of nomograms (11). However, despite the large number of studies analyzing the influencing factors of CCVC of diabetes, only few studies have proposed models to predict CCVD risk in T2DM patients (12,13).
In this study, we conducted a retrospective analysis based on data accumulated in a hospital information system with the aim of constructing a nomogram to quantitatively assess the risk of CCVCs in patients with T2DM. This quantitative prediction model will provide a reference for individualized clinical treatment and mobilize patients with T2DM to adhere to lifestyle interventions. We present the following article in accordance with the TRIPOD reporting checklist (available at https://atm.amegroups.com/article/view/10.21037/atm-22-2439/rc).
Methods
Data source
We obtained data for 1,864 inpatients diagnosed with T2DM in Minhang Hospital, Fudan University (Shanghai, China). The data were collected from January 2014 to December 2018 from the hospital’s information system database and were used as the training cohort. In addition, we obtained data from the same database for 727 inpatients diagnosed with diabetes at the same hospital, from January 2019 to December 2020, to be used as the validation cohort. Diagnostic criteria for T2DM were in accordance with the Chinese guidelines for the diagnosis of T2DM (4). Structured Query Language was used to exclude data with missing information.
CCVCs of diabetes include coronary heart disease, cerebral ischemia, and intracerebral hemorrhage (14). The CCVCs of the study patients with T2DM were extracted based on medical history and clinical diagnoses in the medical records. Inclusion criteria were patients who: (I) received treatment according to the guideline criteria and (II) were >18 years of age. Exclusion criteria were patients with: (I) information missing from physician’s orders, missing clinical data, or unclear medical history in the records; (II) gestational diabetes; (III) severe liver and renal insufficiency; (IV) history of malignancies; and (V) other endocrine disorders. A total of 1,145 patients were included in the training cohort and were further divided into subgroups with and without CCVCs according to their medical records: 309 patients with T2DM plus CCVCs (case group) and 836 as the control group. We included 411 patients in the validation cohort, 106 of whom had CCVCs (Figure 1). This retrospective cross-sectional study was approved by the Ethics Committee of Minhang Hospital, Fudan University (No. 2021-14). Informed consent was given by all participants. The study was conducted in accordance with the Declaration of Helsinki (as revised in 2013).
Research methods
After importing patient data from the hospital information system into the study database, we classified and analyzed demographic information and laboratory test data. The demographic information of the patients included age, sex, duration of T2DM, body mass index (BMI; weight/height2), BP, heart rate (HR), hypertension history, and smoking history. The other indicators collected from patient’s fasting blood test in the morning on admission included glycated hemoglobin A1c (HbA1c), fasting plasma glucose (FPG), 2-h postprandial plasma glucose (2hPG; venous-blood glucose 2 h after oral administration of 75 g glucose), triglycerides (TG), total cholesterol (TC), high-density lipoprotein cholesterol (HDL-C), low-density lipoprotein cholesterol (LDL-C), uric acid (UA), blood urea nitrogen (BUN), serum creatinine (sCr), alanine aminotransferase (ALT), aspartate aminotransferase (AST), and gamma-glutamyl transferase (GGT).
Construction of the nomogram
In the training cohort, we used univariate and multivariate logistic-regression analyses to screen the independent risk factors of CCVCs in patients with T2DM. A nomogram was constructed based on each independent factor to predict the risk of CCVCs, after which we tested the prediction performance of the nomogram in the training and validation cohorts.
Statistical analysis
We used the Kolmogorov-Smirnov test to confirm the normality of the measurement data. The Mann-Whitney U test was utilized to compare differences in non-normally distributed data. Count data were compared using the chi-square (χ2) test. In the training cohort, univariate and multivariate logistic-regression analyses were used to screen independent risk factors of CCVCs in patients with T2DM and to calculate the odds ratios (ORs) and 95% confidence intervals (CIs). We constructed a nomogram to predict the risk of CCVCs in T2DM patients based on each independent factor that was statistically significant in multivariate Logistic regression. The nomogram was validated internally and externally based on data from the training and validation cohorts. For internal validation, we used bootstrap resampling (×1,000) to evaluate the discrimination of the nomogram by the area under the curve (AUC) of the receiver operating characteristic (ROC) curve. The calibration of nomogram was evaluated using the calibration curve combined with the Hosmer-Lemeshow (HL) test. Decision curve analysis (DCA) was used to evaluate the clinical applicability of the nomogram. We performed these analyses using SPSS version 22.0 (IBM Corp., Armonk, NY, USA), MedCalc version 11.0 (MedCalc Software, Ostend, Belgium), and R language version 3.6.2 (R Foundation for Statistical Computing, Vienna, Austria) software packages. P<0.05 (two-sided) was considered statistically significant.
Results
Clinical data of patients
This study included 309 T2DM patients with CCVCs in the training cohort (26.99%, 309/1,145) and 106 with CCVCs in the validation cohort (25.79%, 106/411). Table 1 shows the clinical data, including age, sex, disease duration, HR, BP, hypertension history, smoking history, and biochemical indicators, of the patients in both cohorts. No significant differences in clinical data were found between them (all P>0.05).
Table 1
Factor | Training cohort (n=1,145) | Validation cohort (n=411) | P |
---|---|---|---|
Age (years) | 59.00 (45.00, 76.00) | 60.00 (50.00, 69.00) | 0.594# |
Sex | |||
Male [n (%)] | 378 (33.01) | 144 (35.04) | 0.456* |
Female [n (%)] | 767 (66.99) | 267 (64.96) | |
Disease duration (years) | 7.00 (4.00, 11.00) | 7.24 (3.37, 10.33) | 0.239# |
BMI (kg/m2) | 25.12 (21.44, 28.86) | 24.52 (21.05, 28.02) | 0.007# |
BP | |||
Systolic (mmHg) | 139.00 (117.00, 158.50) | 135.00 (114.00, 153.00) | 0.012# |
Diastolic (mmHg) | 76.93 (66.20, 88.64) | 76.23 (67.79, 79.23) | 0.435# |
Heart rate (beats/min) | 74.80 (65.89, 84.91) | 73.62 (64.65, 84.48) | 0.269# |
Hypertension [cases (%)] | 450 (39.30) | 157 (38.20) | 0.672* |
Smoking [cases (%)] | 299 (26.11) | 111 (27.01) | 0.724* |
HbA1c (%) | 7.51 (5.66, 9.45) | 7.63 (5.70, 9.37) | 0.952# |
FBG (mmol) | 7.94 (5.97, 9.90) | 8.03 (5.85, 10.17) | 0.854# |
2hPG (mmol) | 11.79 (9.81, 13.77) | 11.61 (9.48, 13.64) | 0.259# |
TC (mmol) | 5.48 (3.57, 7.75) | 5.93 (3.76, 8.04) | 0.081# |
TG (mmol) | 2.13 (1.23, 2.94) | 2.06 (1.10, 2.96) | 0.194# |
HDL-C (mmol) | 1.77 (0.98, 2.49) | 1.64 (0.92, 2.39) | 0.072# |
LDL-C (mmol) | 3.70 (2.40, 4.90) | 3.54 (2.35, 4.89) | 0.964# |
UA (µmol) | 295.55 (224.32, 367.46) | 286.10 (214.84, 368.23) | 0.132# |
BUN (mmol) | 5.20 (3.75, 6.70) | 5.30 (3.80, 7.00) | 0.291# |
sCr (mmol) | 71.92 (48.78, 93.31) | 70.85 (48.61, 92.71) | 0.664# |
ALT (U/L) | 12.98 (10.45, 15.72) | 13.10 (10.15, 15.85) | 0.583# |
AST (U/L) | 16.74 (12.31, 21.30) | 16.88 (12.51, 21.24) | 0.069# |
GGT (U/L) | 20.06 (13.53, 27.47) | 20.25 (12.76, 28.24) | 0.901# |
Data are shown as mean (range). *, χ2 test; #, Mann-Whitney U test. 2hPG, 2-h postprandial plasma glucose; ALT, alanine aminotransferase; AST, aspartate aminotransferase; BMI, body mass index; BP, blood pressure; BUN, blood urea nitrogen; FPG, fasting plasma glucose; GGT, gamma-glutamyl transferase; HbA1c, glycated hemoglobin A1c; HDL-C, high-density lipoprotein cholesterol; LDL-C, low-density lipoprotein cholesterol; sCr, serum creatinine; T2DM, type 2 diabetes mellitus; TC, total cholesterol; TG, triglycerides; UA, uric acid.
Univariate analysis of risk factors for CCVCs in diabetic patients
In the training cohort, patients in the case group had longer disease duration and higher BMI, systolic BP (SBP), HbA1c, UA, and BUN than those in the control group. Univariate logistic-regression analysis showed that disease duration, BMI, SBP, HbA1c, UA, and BUN were the risk factors for CCVCs (all P<0.05). Multivariate logistic-regression analysis further showed that disease duration (OR =1.051), BMI (OR =1.129), SBP (OR =1.015), HbA1c (OR =1.106), and UA (OR =1.003) were independent risk factors of CCVCs in T2DM patients (P<0.05; Table 2).
Table 2
Factor | Univariate logistic regression | Multivariate logistic regression | |||||
---|---|---|---|---|---|---|---|
P | OR | 95% CI | P | OR | 95% CI | ||
Age | 0.080 | 1.010 | 1.003–1.017 | ||||
Disease duration | 0.011 | 1.043 | 1.010–1.077 | 0.005 | 1.051 | 1.015–1.088 | |
BMI | <0.001 | 1.129 | 1.093–1.166 | <0.001 | 1.129 | 1.092–1.167 | |
Systolic BP | <0.001 | 1.015 | 1.010–1.021 | <0.001 | 1.015 | 1.009–1.021 | |
HbA1c | 0.005 | 1.091 | 1.026–1.159 | 0.002 | 1.106 | 1.038–1.179 | |
BUN | <0.001 | 1.153 | 1.035–1.137 | 0.214 | 1.053 | 0.971–1.142 | |
UA | <0.001 | 1.009 | 1.007–1.010 | <0.001 | 1.003 | 1.001–1.005 |
BMI, body mass index; BP, blood pressure; BUN, blood urea nitrogen; CCVC, cardiocerebrovascular complication; CI, confidence interval; HbA1c, glycated hemoglobin A1c; OR, odds ratio; T2DM, type 2 diabetes mellitus.
Nomogram for predicting CCVCs in patients with T2DM
Based on the five independent risk factors, we constructed a nomogram to predict the probability of CCVCs in patients with T2DM (Figure 2). Each predictor was proportionally assigned a score according to its OR in the multivariate logistic-regression analyses. We used the total score to determine the risk of CCVCs in patients with T2DM.
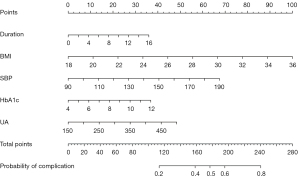
Validation of the nomogram
In the internal validation, the nomogram achieved an AUC of 0.85 (95% CI: 0.828–0.870) after 1,000 bootstrap resampling, indicating good discrimination (AUC >0.75; Figure 3). The calibration plot and HL test showed that the probability predicted by the nomogram did not deviate significantly from actual incidence (χ2=12.596, P=0.127), indicating good calibration (Figure 3B). In the external validation using the validation cohort, the nomogram also showed good discrimination (AUC =0.825; 95% CI: 0.795–0.852; Figure 3D), and the calibration plot and HL test also showed good agreement (χ2=4.654, P=0.096; Figure 3E). DCA results indicated that the nomogram produced net benefits in both cohorts, indicating good application value in clinical decision making (Figure 3C,3F).
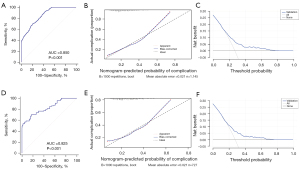
Discussion
CCVD is one of the most common complications of diabetes. Several studies have reported the risk factors of CCVCs of diabetes and corresponding preventive and therapeutic measures, but these complications are often not effectively controlled due to factors such as poor patient compliance (15-17). Because changes in objective indicators can effectively improve patients’ perception of disease risk, thereby improving compliance, having an objective evaluation of the risk factors for CCVCs of diabetes will boost patients’ enthusiasm for participating in long-term treatment (18,19). In this study, we analyzed the relevant data of T2DM patients from a hospital information system and constructed a nomogram for the quantitative assessment of CCVC risk based on the independent risk factors of T2DM patients with CCVCs. By analyzing individual situations and calculating the probability of CCVCs based on the nomogram, clinicians can adjust patients’ treatment plans according to the indicators and provide them with objective, easy-to-understand consultation indicators to encourage treatment adherence. Our nomogram generated net benefits in both the training and validation cohorts, suggesting it has a role in engaging patients’ adherence and motivation.
Diabetes is a chronic disease that requires lifelong treatment. As the disease persists, skeletal muscle mass gradually diminishes, and patients are prone to reduced insulin secretion (20). In addition, muscle mitochondrial function declines with age (21,22), challenging the control of blood glucose and increasing the risk of CCVCs. The basis of treating the CCVCs of diabetes is adherence to lifestyle interventions, so actively encouraging patients to adhere to these interventions is particularly important. Objective and visual risk assessment tools effectively improve patient compliance. Duan et al. (23) proposed that health management tools using goal-oriented designs improved self-management compliance in hypertensive patients, so to simply and quantitatively assess the risk of CCVCs in diabetic patients, we developed a nomogram to convert risk factors into quantifiable risk value indicators. Dynamic assessment of their own risk values in diabetic patients with CCVCs will help mobilize these patients’ enthusiasm for complying with long-term lifestyle interventions and medical treatment.
The components of the nomogram were the independent risk factors identified from the multivariate logistic-regression analysis. This study identified disease duration, BMI, SBP, HbA1c, and UA as independent risk factors of CCVCs in diabetic patients, and all of them except UA are also common risk factors for CCVD. This result was similar to those of McGurnaghan et al. (24), Ilkun et al. (25), and Li et al. (26). As an independent risk factor for CCVD, UA has been receiving increasing attention. Studies have concluded that hyperuricemia affects lipid metabolism (27,28), and high levels of UA affect the secretory function of vascular endothelial cells (ECs) (29,30). Precipitation of UA salt on the walls of blood vessels causes local changes such as inflammation (31,32), triggering the onset and progression of atherosclerosis, and thereby causing cerebrovascular diseases. Hu et al. (33) proposed that elevated plasma UA was associated with an increased risk of insulin resistance. Keerman et al. (34) also reported that UA levels were significantly associated with an increased risk of diabetes. We also found that elevated UA was an independent risk factor for CCVCs.
Nomograms integrate multiple risk factors and quantify and visualize them by displaying the risk weight of each in a digital form (35-37). The strength of this study was using a nomogram to convert probabilities of CCVCs in diabetic patients into quantifiable risk factors. The nomogram can predict the risk of CCVCs and help clinicians communicate with patients using easy-to-understand indicators. The nomogram in this study had good discrimination and calibration after internal and external validation. The probability predicted by the nomogram in both the training and validation cohorts generated net benefits, suggesting that it had good application value in predicting CCVCs in diabetic patients. Clinicians can use the nomogram to calculate the probability of CCVCs in diabetic patients; for example, the total score of a patient with T2DM duration of 10 years, BMI of 25, SBP of 150 mmHg, HbA1c of 9%, and UA of 450 µmol would be calculated as 220, suggesting that the probability of CCVCs for this patient would be 70%. A personalized clinical treatment plan could be formulated, and the risk value calculation repeated according to different clinical states to dynamically evaluate improvements in the risk of CCVCs. This would effectively engage the patient to adhere to lifestyle interventions and medical treatments.
This study had several limitations. The retrospective and cross-sectional design of this study limited our access to longitudinal data and outcomes in T2DM patients. As a result, our model was unable to predict when T2DM patients would progress to CVCC. Besides, only patients with complete clinical data and in-hospital treatments were included, resulting in selection bias. Moreover, this study analyzed only common clinical factors, such as medical history and blood biochemical indicators, and did not include many clinical risk factors or the pathogeneses of CCVCs. Therefore, the clinical applicability of the current model should be further verified. Further multi-center prospective investigations are required in the future. We plan to include patients from the community, and to add data on microvascular complications and indicators for monitoring vascular EC functions. These additions should further improve the clinical applicability of our nomogram.
Conclusions
T2DM patients with longer disease duration and higher BMI, SBP, HbA1c, and UA levels had higher probability of developing CCVCs. The nomogram based on these five independent risk factors quantitatively assessed the risk of CCVCs in T2DM patients. Our findings may help clinicians formulate clinical treatment plans and encourage patients to adhere to them.
Acknowledgments
Funding: None.
Footnote
Reporting Checklist: The authors have completed the TRIPOD reporting checklist. Available at https://atm.amegroups.com/article/view/10.21037/atm-22-2439/rc
Data Sharing Statement: Available at https://atm.amegroups.com/article/view/10.21037/atm-22-2439/dss
Conflicts of Interest: All authors have completed the ICMJE uniform disclosure form (available at https://atm.amegroups.com/article/view/10.21037/atm-22-2439/coif). The authors have no conflicts of interest to declare.
Ethical Statement:
Open Access Statement: This is an Open Access article distributed in accordance with the Creative Commons Attribution-NonCommercial-NoDerivs 4.0 International License (CC BY-NC-ND 4.0), which permits the non-commercial replication and distribution of the article with the strict proviso that no changes or edits are made and the original work is properly cited (including links to both the formal publication through the relevant DOI and the license). See: https://creativecommons.org/licenses/by-nc-nd/4.0/.
References
- Gong Q, Zhang P, Wang J, et al. Morbidity and mortality after lifestyle intervention for people with impaired glucose tolerance: 30-year results of the Da Qing Diabetes Prevention Outcome Study. Lancet Diabetes Endocrinol 2019;7:452-61. [Crossref] [PubMed]
- Yang P, Feng J, Peng Q, et al. Advanced Glycation End Products: Potential Mechanism and Therapeutic Target in Cardiovascular Complications under Diabetes. Oxid Med Cell Longev 2019;2019:9570616. [Crossref] [PubMed]
- Birukov A, Eichelmann F, Kuxhaus O, et al. Opposing Associations of NT-proBNP With Risks of Diabetes and Diabetes-Related Complications. Diabetes Care 2020;43:2930-7. [Crossref] [PubMed]
- Jia W, Weng J, Zhu D, et al. Standards of medical care for type 2 diabetes in China 2019. Diabetes Metab Res Rev 2019;35:e3158. [Crossref] [PubMed]
- American Diabetes Association. Cardiovascular Disease and Risk Management: Standards of Medical Care in Diabetes-2021. Diabetes Care 2021;44:S125-50. [Crossref] [PubMed]
- Ma RCW. Epidemiology of diabetes and diabetic complications in China. Diabetologia 2018;61:1249-60. [Crossref] [PubMed]
- Chew BH, Ghazali SS, Ismail M, et al. Age ≥ 60 years was an independent risk factor for diabetes-related complications despite good control of cardiovascular risk factors in patients with type 2 diabetes mellitus. Exp Gerontol 2013;48:485-91. [Crossref] [PubMed]
- Koipuram A, Carroll S, Punthakee Z, et al. Diabetes knowledge, risk perception, and quality of life among South Asian caregivers in young adulthood. BMJ Open Diabetes Res Care 2020;8:e001268. [Crossref] [PubMed]
- Wu Y, Hu H, Cai J, et al. A prediction nomogram for the 3-year risk of incident diabetes among Chinese adults. Sci Rep 2020;10:21716. [Crossref] [PubMed]
- Dong W, Wan EYF, Fong DYT, et al. Prediction models and nomograms for 10-year risk of end-stage renal disease in Chinese type 2 diabetes mellitus patients in primary care. Diabetes Obes Metab 2021;23:897-909. [Crossref] [PubMed]
- Hochster HS, Niedzwiecki D. Big Data, Small Effects. J Clin Oncol 2016;34:1170-1. [Crossref] [PubMed]
- Ikeda S, Shinohara K, Enzan N, et al. Serial measurement of B-type natriuretic peptide and future cardiovascular events in patients with type 2 diabetes mellitus without known cardiovascular disease. Int J Cardiol 2022;356:98-104. [Crossref] [PubMed]
- Razaghizad A, Oulousian E, Randhawa VK, et al. Clinical Prediction Models for Heart Failure Hospitalization in Type 2 Diabetes: A Systematic Review and Meta-Analysis. J Am Heart Assoc 2022;11:e024833. [Crossref] [PubMed]
- Fox CS, Golden SH, Anderson C, et al. Update on Prevention of Cardiovascular Disease in Adults With Type 2 Diabetes Mellitus in Light of Recent Evidence: A Scientific Statement From the American Heart Association and the American Diabetes Association. Diabetes Care 2015;38:1777-803. [Crossref] [PubMed]
- Nie R, Han Y, Xu J, et al. Illness perception, risk perception and health promotion self-care behaviors among Chinese patient with type 2 diabetes: A cross-sectional survey. Appl Nurs Res 2018;39:89-96. [Crossref] [PubMed]
- Ha Dinh TT, Bonner A, Clark R, et al. The effectiveness of the teach-back method on adherence and self-management in health education for people with chronic disease: a systematic review. JBI Database System Rev Implement Rep 2016;14:210-47. [Crossref] [PubMed]
- Cho NH, Shaw JE, Karuranga S, et al. IDF Diabetes Atlas: Global estimates of diabetes prevalence for 2017 and projections for 2045. Diabetes Res Clin Pract 2018;138:271-81. [Crossref] [PubMed]
- Jamthikar AD, Puvvula A, Gupta D, et al. Cardiovascular disease and stroke risk assessment in patients with chronic kidney disease using integration of estimated glomerular filtration rate, ultrasonic image phenotypes, and artificial intelligence: a narrative review. Int Angiol 2021;40:150-64. [Crossref] [PubMed]
- Nevins TE, Nickerson PW, Dew MA. Understanding Medication Nonadherence after Kidney Transplant. J Am Soc Nephrol 2017;28:2290-301. [Crossref] [PubMed]
- Dela F, Helge JW. Insulin resistance and mitochondrial function in skeletal muscle. Int J Biochem Cell Biol 2013;45:11-5. [Crossref] [PubMed]
- Zhang HH, Ma XJ, Wu LN, et al. SIRT1 attenuates high glucose-induced insulin resistance via reducing mitochondrial dysfunction in skeletal muscle cells. Exp Biol Med (Maywood) 2015;240:557-65. [Crossref] [PubMed]
- Kaliszewska A, Allison J, Martini M, et al. Improving Age-Related Cognitive Decline through Dietary Interventions Targeting Mitochondrial Dysfunction. Int J Mol Sci 2021;22:3574. [Crossref] [PubMed]
- Duan H, Wang Z, Ji Y, et al. Using Goal-Directed Design to Create a Mobile Health App to Improve Patient Compliance With Hypertension Self-Management: Development and Deployment. JMIR Mhealth Uhealth 2020;8:e14466. [Crossref] [PubMed]
- McGurnaghan S, Blackbourn LAK, Mocevic E, et al. Cardiovascular disease prevalence and risk factor prevalence in Type 2 diabetes: a contemporary analysis. Diabet Med 2019;36:718-25. [Crossref] [PubMed]
- Ilkun OL, Greene T, Cheung AK, et al. The Influence of Baseline Diastolic Blood Pressure on the Effects of Intensive Blood Pressure Lowering on Cardiovascular Outcomes and All-Cause Mortality in Type 2 Diabetes. Diabetes Care 2020;43:1878-84. [Crossref] [PubMed]
- Li S, Nemeth I, Donnelly L, et al. Visit-to-Visit HbA1c Variability Is Associated With Cardiovascular Disease and Microvascular Complications in Patients With Newly Diagnosed Type 2 Diabetes. Diabetes Care 2020;43:426-32. [Crossref] [PubMed]
- Wu AH, Gladden JD, Ahmed M, et al. Relation of serum uric acid to cardiovascular disease. Int J Cardiol 2016;213:4-7. [Crossref] [PubMed]
- Katsiki N, Dimitriadis GD, Mikhailidis DP. Serum Uric Acid and Diabetes: From Pathophysiology to Cardiovascular Disease. Curr Pharm Des 2021;27:1941-51. [Crossref] [PubMed]
- Hisatome I, Li P, Miake J, et al. Uric Acid as a Risk Factor for Chronic Kidney Disease and Cardiovascular Disease- Japanese Guideline on the Management of Asymptomatic Hyperuricemia. Circ J 2021;85:130-8. [Crossref] [PubMed]
- Otani N, Toyoda S, Sakuma M, et al. Effects of uric acid on vascular endothelial function from bedside to bench. Hypertens Res 2018;41:923-31. [Crossref] [PubMed]
- Cho YS, Bae JH, Moon SH, et al. Serum uric acid in asymptomatic adults is weakly associated with carotid artery FDG uptake but not intima-media thickness. J Nucl Cardiol 2020;27:1537-46. [Crossref] [PubMed]
- Kleber ME, Delgado G, Grammer TB, et al. Uric Acid and Cardiovascular Events: A Mendelian Randomization Study. J Am Soc Nephrol 2015;26:2831-8. [Crossref] [PubMed]
- Hu X, Rong S, Wang Q, et al. Association between plasma uric acid and insulin resistance in type 2 diabetes: A Mendelian randomization analysis. Diabetes Res Clin Pract 2021;171:108542. [Crossref] [PubMed]
- Keerman M, Yang F, Hu H, et al. Mendelian randomization study of serum uric acid levels and diabetes risk: evidence from the Dongfeng-Tongji cohort. BMJ Open Diabetes Res Care 2020;8:e000834. [Crossref] [PubMed]
- Zheng RR, Huang XW, Liu WY, et al. Nomogram Predicting Overall Survival in Operable Cervical Cancer Patients. Int J Gynecol Cancer 2017;27:987-93. [Crossref] [PubMed]
- Wu M, Hu Y, Ren A, et al. Nomogram Based on Ultrasonography and Clinical Features for Predicting Malignancy in Soft Tissue Tumors. Cancer Manag Res 2021;13:2143-52. [Crossref] [PubMed]
- Zeng T, Yao L, Zhao K, et al. Identification and validation of a five apoptosis-related genes signature for prediction of disease-free survival for testicular germ cell tumors. Transl Androl Urol 2021;10:1250-72. [Crossref] [PubMed]
(English Language Editor: K. Brown)