Diagnostic study on clinical feasibility of an AI-based diagnostic system as a second reader on mobile CT images: a preliminary result
Introduction
Lung cancer is the most commonly diagnosed cancer and the leading cause of cancer death (18% of total cancer deaths) (1). Early-stage diagnosis helps achieve timely treatment and improves survival (2). Appropriate screening tests and accurate interpretation of results are crucial for detecting early-stage disease and thus reducing the burden of lung cancer. Low-dose computed tomography (LDCT) is an ideal testing tool and its implementation has improved cancer detection worldwide (3). However, LDCT carries the risk of unnecessary follow-up CT scans if the results are overinterpreted (4,5). Therefore, a new focus of research has been the development of techniques that can diagnose nodules with expertise, ensuring identification of early-stage disease and avoidance of additional radiation exposure (6).
Over the past decade, artificial intelligence (AI) has breathed new life into the medical field due to its high efficacy in data postprocessing and disease diagnosis (7,8). Although concerns still exist in regard to its reproducibility and robustness, AI’s potential in optimizing clinical medicine practice has been widely acknowledged (9,10). Radiologists expect AI to act as an alternative for experts in diagnosing lung cancer or aiding in the diagnosis of abnormal tumor in the CT imaging. Thus, one of the most promising applications of AI algorithms is as a second reader for radiologists or imaging assistants, especially in regions where experts are scarce. A deep learning-based automatic detection algorithm (DLAD) for lung segmentation and nodule detection (threshold-, region-, and clustering-based methods, etc.) has been built but has demonstrated varied accuracy [area under the curve (AUC) of currently reported validation tests ranged from 61.3% to 92.0%] (11,12). Moreover, whether the output information from AI will translate into benefits for clinical workflow or patient outcomes in a real-world setting remains unknown. These questions have driven our research to facilitate implementation of this evolving technique (13,14).
InferRead CT Lung (https://us.infervision.com/product/5/) is an AI platform developed by Infervision Technology Co., Ltd. (15). It is designed to support concurrent reading and aid radiologists in pulmonary nodule detection during chest CT scan reviews. It has received Food and Drug Administration (FDA) approval and Conformité Européenne (CE) certification and is integrated into the clinical practice of more than 280 hospitals worldwide (https://www.fda.gov/medical-devices/510k-clearances/july-2020-510k-clearances). Here we prospectively examined cases collected through a mobile CT traveling to nearby medium-sized cities. All cases were sent to our center and diagnosed by radiologists using AI as a second reader. We sought to demonstrate the efficacy of a developed lung nodule detection and diagnosis AI algorithm and examine its effects on radiologists as a second reader in screening patients for pulmonary abnormalities in a real-world setting. We present the following article in accordance with the STARD reporting checklist (available at https://atm.amegroups.com/article/view/10.21037/atm-22-2157/rc).
Methods
Population
This prospective study cohort was selected from a lung cancer screening program in Sichuan Province, China (ChiCTR2200056422, http://www.chictr.org.cn). This program aimed to conduct a cross-sectional screening of lung cancer in adults aged between 40–69 years in Sichuan Province using a mobile CT platform (NeuViz, Neusoft Medical, China). The mobile CT is a miniature advanced CT diagnosis room composed of CT inspection system, air suspension platform, radiation protection cargo, intelligent imaging cloud system, 5G communication module, and automatic power supply system. It was transported by car to nearby medium cities (Figure S1). Patients whose images were successfully transferred back to our center, diagnosable, and available for AI analysis were included. The exclusion criteria included: (I) patients aged <40 or >69 years, (II) patients with no electronic health records, and (III) patients whose images were not transferrable. The study was conducted in accordance with the Declaration of Helsinki (as revised in 2013). The study was approved by ethics committee of West China Hospital of Sichuan University [No. 2020(145)]. Informed consent was taken from all the patients. Demographic data, smoking history, and history of pulmonary or other neoplastic diseases were recorded and stored in a secure electronic data capture system (EDC). In total, 4,858 participants received mobile LDCT screening between July 10th, 2020 and September 10th, 2020 (Figure 1).
AI intervention workflow for radiologists
Images of all included patients were sent to the Department of Radiology of a single center for interpretation and diagnosis. A total of 7 radiologists with similar expertise (5-year work experience, trained and worked at our center) interpreted the images. All participating radiologists were blinded to patients’ clinical information.
For each case, images were initially sent to a radiology resident (junior reader) and AI server for first reading. The radiologist (senior reader) assigned to the case then performed a second reading and final report. During the second reading, the senior reader reviewed the image alone and made an initial diagnosis, and then reviewed the report from the junior reader, corrected description errors, and made a final diagnosis. When making the final diagnosis, the senior reader would also consult the AI algorithm results and decide whether to accept the AI findings.
During this workflow, the senior reader was required to give a high-risk (HR) label when a HR lesion was suspected as raised by the junior reader, AI, or senior reader. An additional label (HR-junior, HR-AI, HR-senior, or HR-final) was also included to designate who provided the HR diagnosis. The length of time for each case report was automatically traced and recorded by the imaging workstation. Radiologists made a note of any delays, for example, a phone call. Those reports labeled as “delayed” were excluded when calculating average report duration. A backstage management system was used to record whether the results from the AI algorithm were reviewed (Figure 2).
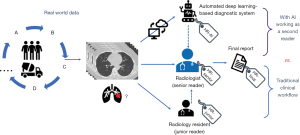
Clinical and imaging data collection
The following demographic data were collected: (I) clinical features (age, gender, BMI, smoking history, and respiratory system related symptoms) and (II) imaging diagnostic work-flow (HR labels, diagnostic duration, and AI use) from the EDC and online imaging system. Next, CT imaging characteristics, including location, dimension (average of long and short axes at the maximum axial area), maximum axial area, volume, nodule type [ground glass nodule (GGN), partial-solid nodule (PSN), or solid nodule (SN)], and signs of malignancy (spiculation, lobulation, irregular shape, and pleural involvement) of each lesion were acquired from the AI workstation. These characteristics were automatically described and calculated by the AI algorithm and manually revised by a blinded investigator (Appendix 1). Additionally, for each lesion, an AI-based risk score was calculated and recorded. For patients with multiple lesions, only the lesion with the highest risk score or highest suspicion of malignancy was selected and used for analysis (16).
Study outcomes
The primary outcome was defined as the diagnostic accuracy of AI when compared with the results from senior readers. In addition, cases were divided into 2 groups based on whether agreement on HR was reached between AI and the senior readers, and the CT imaging characteristics of the 2 groups were compared. The secondary outcome was subjective evaluation of AI performance by the participating radiologists. At the end of the study, participating radiologists were required to complete a questionnaire concerning the performance of AI in the clinical workflow.
Statistical analysis
Descriptive analysis was performed to describe the characteristics of the demographic data. Continuous variables are presented as mean ± standard deviation (SD) or median with interquartile range (IQR), and categorical variables are presented as frequency and percentage. The baseline variables and CT features of patients for whom there was agreement between AI and radiologists regarding the HR label were compared with those of patients for whom there was disagreement between AI and radiologists. Student’s t-test or Mann-Whitney U test was used for continuous variables, and Chi-square test or Fisher’s exact test was used for categorical variables. Heatmaps showing the contribution weight of pixels of a CT image to the AI prediction results are presented graphically for featured cases. A P value less than 0.05 in two-sided was considered statistically significant. Statistical analysis was performed with R project (v. 3.3.1, R Foundation for Statistical Computing, Vienna, Austria).
Results
Patient demographics
In total, 251/3,872 patients (6.48%) were labeled as HR. The final group comprised 91 males and 160 females (the median age for all patients was 66 years, ranging from 60–71 years). Baseline clinical characteristics were collected from 238 patients. Of these patients, 6 had a history of malignant diseases, including 2 with lung cancer, 3 with gastrointestinal cancer, and 1 with breast cancer. A total of 39 (15.5%) patients were current smokers and 19 used to smoke (Table 1).
Table 1
Characteristic | Data |
---|---|
No. of patients | 238 |
Age*, years | 66 [60–71] |
Gender | |
Male | 91 (36.3) |
Female | 160 (63.7) |
BMI, kg/m2, mean ± SD | 24.0±3.3 |
Smoking history | |
Never | 180 (75.6) |
Quit | 19 (8.0) |
Current smoker | 39 (16.4) |
History of COPD | 1 (0.4) |
History of malignancy | 6 (2.5) |
Symptoms | |
Short of breath | 21 (8.8) |
Hoarseness | 8 (3.4) |
Chest pain | 18 (7.6) |
*, data are expressed as medians with interquartile range in parentheses. Categorical data are expressed as numbers with percentages in parentheses. BMI, body mass index; COPD, chronic obstructive pulmonary disease.
During the workflow, patients received a total of 587 subclass HR labels, including 120 HR-junior labels, 101 HR-senior labels, 234 HR-AI labels, and 132 HR-final labels from senior readers. By referring to the senior readers’ final results as the gold standard, positive predictive value (PPV) of the AI platform was 55.6% [95% confidence interval (CI): 49.0–62.0%], while the negative predictive value (NPV) came to 88.2% (95% CI: 62.2–98.0%). The time spent by senior readers on finalizing a single radiology report was 234±137.3 s. The time spent finalizing a report was significantly shorter when AI was deployed as a second reader (with AI: 223±145.6 s vs. without AI: 270±143.17 s, P<0.001) (Figure 3).
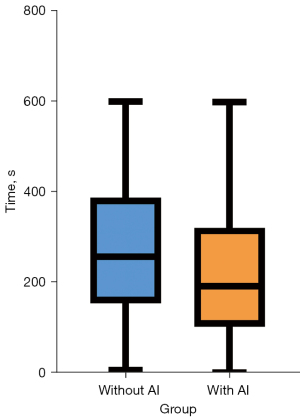
Comparison of CT image characteristics between AI- and radiologist-labeled HR nodules
In summary, radiologists and AI agreed on the HR label for 95 (37.8%) patients, junior radiologists and AI were in agreement for 106 (42.2%) patients, and among junior and senior radiologists, agreement was reached for 61 (24.3%) patients. Overall, agreement on HR labeling among the junior radiologists, senior radiologists, and AI was reached for only 58 (23.1%) patients.
Patients with a HR label that AI and radiologists had agreed on had a significantly higher percentage of lesions with a dimension over 6 mm [90 (94.7%) vs. 115 (79.3%), P=0.002], a larger maximum axial area [107.8 (IQR, 65.3–267.2) vs. 55.7 (IQR, 30.9–100.5) mm2, P<0.001], and a higher volume [773.9 (IQR, 300.1–2,805.8) vs. 191.0 (IQR, 90.7–445.6) mm3, P<0.001] compared to patients who received an inconsistent opinion from AI and radiologists (Table 2).
Table 2
Characteristics | Agreed HR labels (n=95) | Disagreed HR labels (n=145) | P value |
---|---|---|---|
Age, years | 66 [63–72] | 66 [58–70] | 0.091 |
Gender (male/female) | 38/57 | 49/96 | 0.400 |
Location of the nodule | 0.797 | ||
Left upper lobe | 15 (15.8) | 25 (17.2) | |
Left lower lobe | 21 (22.1) | 36 (24.8) | |
Right upper lobe | 32 (33.7) | 38 (26.2) | |
Right middle lobe | 5 (5.3) | 10 (6.9) | |
Right lower lobe | 22 (23.2) | 36 (24.8) | |
Dimension | 0.002* | ||
<6 mm | 5 (5.3) | 30 (20.7) | |
≥6 mm | 90 (94.7) | 115 (79.3) | |
Maximum axial area (mm2) | 107.8 (65.3–267.2) | 55.7 (30.9–100.5) | <0.001* |
Volume (mm3) | 773.9 (300.1–2,805.8) | 191.0 (90.7–445.6) | <0.001* |
Average CT number | −331.0 (−507.0–21.0) | −255.0 (−517.0–23.0) | 0.933 |
Malignant signs | |||
Spiculated | 37 (38.9) | 18 (12.4) | <0.001* |
Lobulated | 41 (43.2) | 22 (15.2) | <0.001* |
Irregular shape | 3 (3.2) | 3 (2.1) | 0.733 |
Pleural involved | 27 (28.4) | 10 (6.9) | <0.001* |
Type of nodule | 0.013* | ||
SN | 29 (30.5) | 57 (39.3) | |
PSN | 48 (50.5) | 46 (31.7) | |
pGGN | 18 (18.9) | 42 (29.0) | |
AI-risk score | 0.93 (0.87–0.96) | 0.86 (0.77–0.91) | <0.001* |
Dimensions are average of long and short axes, rounded to the nearest millimeter. Non-parametric data are expressed as medians with IQR in parentheses. Categorical data are expressed as numbers with percentages in parentheses. *, statistical significance. HR, high risk; AI, artificial intelligence; SN, solid nodule; PSN, partial-solid nodule; pGGN, pure ground glass nodule; IQR, interquartile range.
In addition, the AI risk score and percentage of presence of HR CT characteristics, including spiculation [37 (38.9%) vs. 18 (12.4%), P<0.001], lobulation [41 (43.2%) vs. 22 (15.2%), P<0.001], and pleural involvement [27 (28.4%) vs. 10 (6.9%), P<0.001] were higher in patients with a unanimous HR label. Cases in which AI and radiologists differed in their opinions are presented in Figure 4.
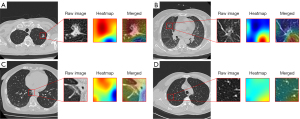
CT characteristics of nodules with a revised HR classification
Among the 145 patients who were given disparate labels from the radiologists and AI, 35 (24.1%) were reclassified as HR and 4 (3.0%) HR patients were reclassified as non-HR after referring to the AI opinion (Table 3).
Table 3
Characteristics | Reclassified (n=35) | Non-reclassified (n=104) | P value |
---|---|---|---|
Age, years | 65 [59–69] | 66 [60–71] | 0.438 |
Gender (male/female) | 13/22 | 36/68 | 0.947 |
Location of the nodule | 0.013* | ||
Left upper lobe | 11 (31.4) | 13 (12.5) | |
Left lower lobe | 5 (14.3) | 30 (28.8) | |
Right upper lobe | 11 (31.4) | 26 (25.0) | |
Right middle lobe | 4 (11.4) | 4 (3.8) | |
Right lower lobe | 4 (11.4) | 31 (29.8) | |
Dimension | 0.054 | ||
<6 mm | 3 (8.6) | 27 (26.0) | |
≥6 mm | 32 (91.4) | 77 (74.0) | |
Maximum axial area (mm2) | 69.1 (51.9–118.1) | 50.0 (27.8–97.8) | 0.014* |
Volume (mm3) | 309.9 (214.9–732.5) | 141.3 (79.3–380.8) | <0.001* |
Average CT number | −511.0 (−576.5 to −100.5) | −191.5 (−487.3–22.5) | 0.008* |
Malignant signs | |||
Spiculated | 5 (14.3) | 13 (12.5) | 0.776 |
Lobulated | 6 (17.1) | 16 (15.4) | 0.793 |
Irregular shape | 2 (5.7) | 1 (1.0) | 0.156 |
Pleural involved | 9 (25.7) | 9 (8.7) | 0.451 |
Type of the nodule | 0.010* | ||
SN | 9 (25.7) | 47 (45.2) | |
PSN | 9 (25.7) | 34 (32.7) | |
pGGN | 17 (48.6) | 23 (22.1) | |
AI-risk score | 0.90 (0.86–0.94) | 0.83 (0.76–0.89) | <0.001* |
Dimensions are average of long and short axes, rounded to the nearest millimeter. Non-parametric data are expressed as medians with interquartile range in parentheses. Category data are expressed as numbers with percentages in parentheses. *, statistical significance. HR, high risk; AI, artificial intelligence; SN, solid nodule; PSN, partial-solid nodule; pGGN, pure ground glass nodule.
The lesions in patients with a revised HR label had a higher volume [309.9 (IQR, 214.9–732.5) vs. 141.3 (IQR, 79.3–380.8) mm3, P<0.001] and a larger maximum axial area [69.1 (IQR, 51.9–118.1) vs. 50.0 (IQR, 27.8–97.8) mm2, P=0.014] when compared to those that were unchanged. Further, radiologists tended to revise the label if a nodule with an AI-labeled HR was pure GGN (pGGN) rather than PSN or SN (P=0.010). Similarly, the lesions in the revised group had a significantly lower average CT number [−511.0 (IQR, −576.5 to −100.5) vs. −191.5 (IQR, −487.3 to 22.5), P=0.008) than the those in the non-revised group.
Self-evaluation results
Self-evaluation results from the radiologists showed that the median score of nodule detection and nodule classification was 8 (IQR, 8–8.5) and 7 (IQR, 5.5–8), respectively. The majority (5/7, 71.4%) of participants indicated that the AI algorithm was superior to radiologists in nodule detection, while only 14.2% (1/7) felt AI was superior to radiologists in nodule classification. Additionally, with the AI algorithm as a second reader, 85.71% (6/7) and 42.8% (3/7) of participants indicated that nodule detection accuracy and nodule classification, respectively, could be improved. In contrast, no participant thought the diagnostic result from a radiology resident would increase accuracy, in either nodule detection or classification (Table S1).
Discussion
This study demonstrated that the validated lung cancer AI software had a relatively high NPV and low PPV in diagnosing HR lung nodules in the general population, and thus it is feasible to use lung cancer AI as a second reader for clinical evaluation. The major advantage of the AI algorithm was a reduction of 47 s in average diagnosis time. Additionally, the diagnosis provided by the AI algorithm was most useful when the target nodule had a larger volume, ground glass opacity (GGO), or recognized signs of malignancy. However, continued learning for AI using selected case training may be warranted to improve its specificity and thus better realize its potential, further enhancing its role in clinical settings.
The performance of the mature AI product in this patient population was inferior to a previous report in a simulated study environment (17). Technically, the sensitivity and specificity of a designed AI algorithm, which is a diagnostic tool, could be adjusted based on the needs of the medical center (18). Thus, it is not surprising that the NPV would be higher with a lower PPV. This results from demand for high sensitivity in a screening-test scenario to avoid missing any potential lung cancer nodules (19). Although a false positive diagnosis would lead to unnecessary CT examination, a second consideration from a radiologist could avoid such a problem. Previous AI studies have suggested that AI might be optimal for underdeveloped areas or those without medical resources (20,21). Our results further supported this by demonstrating AI performance for data collected using a mobile CT. The images collected in this study had comparable image quality and equivalent radiation doses to images acquired from in-hospital CT scanners (Appendix 2). Our data supported the use of an AI platform for screening tests at locations with a shortage of thoracic imaging experts. Patients could be triaged faster and referred to experts if there was suspicion of a HR lesion.
Another significant finding from our study was that diagnostic duration when AI was used as a second reader was shorter than without AI. This result is consistent with previous reports, although the difference in time reduction was smaller. For instance, Annarumma et al. reported that the average reporting delay could be reduced from 11.2 to 2.7 days by using a radiograph AI diagnostic system (22). Nevertheless, the workload at our center is extremely large (over 100 reports per day for each radiologist), and the average diagnostic duration of each case had to be shortened to <5 min, which may explain the difference. We also found that the reduction in diagnosis time mostly occurred in cases with a negative finding. For such cases, it is possible that an agreed finding of “negative” from AI would increase confidence in the radiologist’s diagnosis, which would encourage them to finalize the report in a shorter time.
The AI opinion as a second reader affected radiologist decision-making and led to a change in the final report in approximately 1/5 of cases, mainly driven by a larger lesion volume and a GGO rather than solid imaging presentation. Interestingly, dimensions did not impact radiologists’ decisions. Understandably, 3-dimensional (3D) volume is preferred to 2D for diagnosis of malignant lung nodules (23,24). Nevertheless, 2D-axial view was still the first choice for taking a quick look at a case as quantifying 3D volume by hand is not convenient (25). As the AI algorithm has been proven to have high accuracy in multiple quantification tasks, it does provide an ideal assistant for radiologists (20,26). GGO is another key sign of early-stage lung cancer but is more likely to be missed even by an experienced radiologist, especially when handling a large volume of cases (27). Our results are supported by reports stating that AI has significantly increased the detection of GGO in hospitals (28,29).
The disagreement between radiologists and the AI algorithm regarding HR nodule diagnosis implied that the AI algorithm “thinks” in a different way from human beings. It remains unknown how to fill this gap and better facilitate the implementation of AI. As expected, the performance of an AI platform in a real-world setting is lower than that in a simulated setting (30,31). Continued learning by additional data feeding could partially help maintain AI performance (32). However, the clinical thinking during the diagnosis is not necessarily “learned” by the computer (33,34). Cao et al. developed a step-by-step aorta dissection AI segmentation model which realistically reflected how doctors would handle the task, and this step-by-step model was superior to the traditional model developed by simple data feeding (35). In our study, the heat map of a missed case by AI showed that the focus of AI algorithm deviates, which cannot simply be solved by adding another training case. Thus, we propose that future AI design open the “black box” and follow the steps that a doctor would take when determining diagnoses.
Limitations
Our study had several limitations. First, we did not acquire the pathologic diagnosis or follow-up data for these nodules. Thus, if AI had a different opinion for the nodules, we could not judge them to be incorrect. Nevertheless, the purpose of this study was to report AI performance in a real-world clinical workflow, as well as to investigate the impact of AI participation on radiologists. Results concerning the final diagnosis need to be further analyzed in a future study. Second, although we collected data from multiple centers using the mobile CT, all participating radiologists were from a single center. While the diagnostic expertise of all radiologists was kept consistent, validation from more centers is still warranted.
Conclusions
A validated lung cancer detection AI algorithm could be deployed as a second reader for routine clinical practice, especially in regions where resources are scarce and fast, safe triage is expected. Continued learning is needed to maintain or improve the performance of AI in the clinical setting.
Acknowledgments
We would like to thank Xiaodi Zhang (Philips Healthcare) who assisted with revising this paper. The authors thank Mr. Wen Tang and Dr. Hongkun Yin from the Institute of Advanced Research, Infervision Medical Technology Co., Ltd., Beijing, China for their support in data analysis.
Funding: This study was supported by the Research Grant of National Nature Science Foundation of China (No. 81971571) and 1.3.5 Project for Disciplines of Excellence, West China Hospital, Sichuan University (No. ZYYC21004).
Footnote
Reporting Checklist: The authors have completed the STARD reporting checklist. Available at https://atm.amegroups.com/article/view/10.21037/atm-22-2157/rc
Data Sharing Statement: Available at https://atm.amegroups.com/article/view/10.21037/atm-22-2157/dss
Conflicts of Interest: All authors have completed the ICMJE uniform disclosure form (available at https://atm.amegroups.com/article/view/10.21037/atm-22-2157/coif). BS serves as an unpaid editorial board member of Annals of Translational Medicine from September 2020 to August 2022. The other authors have no conflicts of interest to declare.
Ethical Statement: The authors are accountable for all aspects of the work in ensuring that questions related to the accuracy or integrity of any part of the work are appropriately investigated and resolved. The study was conducted in accordance with the Declaration of Helsinki (as revised in 2013). The study was approved by ethics committee of West China Hospital of Sichuan University [No. 2020 (145)]. Informed consent was taken from all the patients.
Open Access Statement: This is an Open Access article distributed in accordance with the Creative Commons Attribution-NonCommercial-NoDerivs 4.0 International License (CC BY-NC-ND 4.0), which permits the non-commercial replication and distribution of the article with the strict proviso that no changes or edits are made and the original work is properly cited (including links to both the formal publication through the relevant DOI and the license). See: https://creativecommons.org/licenses/by-nc-nd/4.0/.
References
- Sung H, Ferlay J, Siegel RL, et al. Global Cancer Statistics 2020: GLOBOCAN Estimates of Incidence and Mortality Worldwide for 36 Cancers in 185 Countries. CA Cancer J Clin 2021;71:209-49. [Crossref] [PubMed]
- Sands J, Tammemägi MC, Couraud S, et al. Lung Screening Benefits and Challenges: A Review of The Data and Outline for Implementation. J Thorac Oncol 2021;16:37-53. [Crossref] [PubMed]
- Yankelevitz DF, Yip R, Smith JP, et al. CT Screening for Lung Cancer: Nonsolid Nodules in Baseline and Annual Repeat Rounds. Radiology 2015;277:555-64. [Crossref] [PubMed]
- Silva M, Milanese G, Sestini S, et al. Lung cancer screening by nodule volume in Lung-RADS v1.1: negative baseline CT yields potential for increased screening interval. Eur Radiol 2021;31:1956-68. [Crossref] [PubMed]
- MacMahon H, Naidich DP, Goo JM, et al. Guidelines for Management of Incidental Pulmonary Nodules Detected on CT Images: From the Fleischner Society 2017. Radiology 2017;284:228-43. [Crossref] [PubMed]
- Oudkerk M, Liu S, Heuvelmans MA, et al. Lung cancer LDCT screening and mortality reduction - evidence, pitfalls and future perspectives. Nat Rev Clin Oncol 2021;18:135-51. [Crossref] [PubMed]
- Fadil H, Totman JJ, Hausenloy DJ, et al. A deep learning pipeline for automatic analysis of multi-scan cardiovascular magnetic resonance. J Cardiovasc Magn Reson 2021;23:47. [Crossref] [PubMed]
- Kotter E, Ranschaert E. Challenges and solutions for introducing artificial intelligence (AI) in daily clinical workflow. Eur Radiol 2021;31:5-7. [Crossref] [PubMed]
- Yamashita R, Long J, Longacre T, et al. Deep learning model for the prediction of microsatellite instability in colorectal cancer: a diagnostic study. Lancet Oncol 2021;22:132-41. [Crossref] [PubMed]
- Raya-Povedano JL, Romero-Martín S, Elías-Cabot E, et al. AI-based Strategies to Reduce Workload in Breast Cancer Screening with Mammography and Tomosynthesis: A Retrospective Evaluation. Radiology 2021;300:57-65. [Crossref] [PubMed]
- Lachance CC, Walter M. Artificial Intelligence for Classification of Lung Nodules: A Review of Clinical Utility, Diagnostic Accuracy, Cost-Effectiveness, and Guidelines. Ottawa (ON): Canadian Agency for Drugs and Technologies in Health; 2020 Jan 22.
- Halder A, Dey D, Sadhu AK. Lung Nodule Detection from Feature Engineering to Deep Learning in Thoracic CT Images: a Comprehensive Review. J Digit Imaging 2020;33:655-77. [Crossref] [PubMed]
- Cruz Rivera S, Liu X, Chan AW, et al. Guidelines for clinical trial protocols for interventions involving artificial intelligence: the SPIRIT-AI extension. Nat Med 2020;26:1351-63. [Crossref] [PubMed]
- Setting guidelines to report the use of AI in clinical trials. Nat Med 2020;26:1311. [Crossref] [PubMed]
- Liu K, Li Q, Ma J, et al. Evaluating a Fully Automated Pulmonary Nodule Detection Approach and Its Impact on Radiologist Performance. Radiol Artif Intell 2019;1:e180084. [Crossref] [PubMed]
- Yang K, Liu J, Tang W, et al. Identification of benign and malignant pulmonary nodules on chest CT using improved 3D U-Net deep learning framework. Eur J Radiol 2020;129:109013. [Crossref] [PubMed]
- Jacobs C, Setio AAA, Scholten ET, et al. Deep Learning for Lung Cancer Detection on Screening CT Scans: Results of a Large-Scale Public Competition and an Observer Study with 11 Radiologists. Radiol Artif Intell 2021;3:e210027. [Crossref] [PubMed]
- Guo J, Wang C, Xu X, et al. DeepLN: an artificial intelligence-based automated system for lung cancer screening. Ann Transl Med 2020;8:1126. [Crossref] [PubMed]
- Walker SP. The ROC Curve Redefined - Optimizing Sensitivity (and Specificity) to the Lived Reality of Cancer. N Engl J Med 2019;380:1594-5. [Crossref] [PubMed]
- Luchini C, Pea A, Scarpa A. Artificial intelligence in oncology: current applications and future perspectives. Br J Cancer 2022;126:4-9. [Crossref] [PubMed]
- Ueda T, Ohno Y, Yamamoto K, et al. Deep Learning Reconstruction of Diffusion-weighted MRI Improves Image Quality for Prostatic Imaging. Radiology 2022;303:373-81. [Crossref] [PubMed]
- Annarumma M, Withey SJ, Bakewell RJ, et al. Automated Triaging of Adult Chest Radiographs with Deep Artificial Neural Networks. Radiology 2019;291:196-202. [Crossref] [PubMed]
- Han D, Heuvelmans MA, Vliegenthart R, et al. Influence of lung nodule margin on volume- and diameter-based reader variability in CT lung cancer screening. Br J Radiol 2018;91:20170405. [Crossref] [PubMed]
- Heuvelmans MA, Walter JE, Vliegenthart R, et al. Disagreement of diameter and volume measurements for pulmonary nodule size estimation in CT lung cancer screening. Thorax 2018;73:779-81. [Crossref] [PubMed]
- Hein PA, Romano VC, Rogalla P, et al. Linear and volume measurements of pulmonary nodules at different CT dose levels - intrascan and interscan analysis. Rofo 2009;181:24-31. [Crossref] [PubMed]
- Sung J, Park S, Lee SM, et al. Added Value of Deep Learning-based Detection System for Multiple Major Findings on Chest Radiographs: A Randomized Crossover Study. Radiology 2021;299:450-9. [Crossref] [PubMed]
- Donald JJ, Barnard SA. Common patterns in 558 diagnostic radiology errors. J Med Imaging Radiat Oncol 2012;56:173-8. [Crossref] [PubMed]
- Cho J, Kim J, Lee KJ, et al. Incidence Lung Cancer after a Negative CT Screening in the National Lung Screening Trial: Deep Learning-Based Detection of Missed Lung Cancers. J Clin Med 2020;9:3908. [Crossref] [PubMed]
- Yen A, Pfeffer Y, Blumenfeld A, et al. Use of a Dual Artificial Intelligence Platform to Detect Unreported Lung Nodules. J Comput Assist Tomogr 2021;45:318-22. [Crossref] [PubMed]
- Korfiatis P, Denic A, Edwards ME, et al. Automated Segmentation of Kidney Cortex and Medulla in CT Images: A Multisite Evaluation Study. J Am Soc Nephrol 2022;33:420-30. [Crossref] [PubMed]
- Lin H, Li R, Liu Z, et al. Diagnostic Efficacy and Therapeutic Decision-making Capacity of an Artificial Intelligence Platform for Childhood Cataracts in Eye Clinics: A Multicentre Randomized Controlled Trial. EClinicalMedicine 2019;9:52-9. [Crossref] [PubMed]
- Pianykh OS, Langs G, Dewey M, et al. Continuous Learning AI in Radiology: Implementation Principles and Early Applications. Radiology 2020;297:6-14. [Crossref] [PubMed]
- Steiger P. Radiomics and Artificial Intelligence: From Academia to Clinical Practice. J Physician Assist Educ 2015;26:109-10. [Crossref]
- Bratt A. Why Radiologists Have Nothing to Fear From Deep Learning. J Am Coll Radiol 2019;16:1190-2. [Crossref] [PubMed]
- Cao L, Shi R, Ge Y, et al. Fully automatic segmentation of type B aortic dissection from CTA images enabled by deep learning. Eur J Radiol 2019;121:108713. [Crossref] [PubMed]
(English Language Editor: A. Muijlwijk)