A prediction model with measured sentiment scores for the risk of in-hospital mortality in acute pancreatitis: a retrospective cohort study
Introduction
Acute pancreatitis (AP) is an inflammatory disease of the pancreas and is the cause of substantial mortality and morbidity worldwide (1). In the general population, the incidence of AP is 34 per 100,000 people annually and continues to increase (2). As one of the most frequent gastrointestinal causes for hospital admissions, AP leads to $9.3 billion in costs to the healthcare system in the United States each year (3,4). Evidence shows that approximately 20–30% of AP patients can go on to develop severe acute pancreatitis (SAP), which has high mortality and morbidity because of the development of extra-pancreatic and pancreatic necrosis, subsequent infection, and multiple organ failure (5,6). Despite a decreased overall mortality due to improvements in the care of critically ill patients and in accurate and prompt diagnosis, the mortality and long-term sequelae of AP are still considerable (7,8). To implement more effective management, it is of great importance for clinicians to accurately assess the severity and prognosis of AP patients in a timely manner.
In the last few years, scoring systems, such as sequential organ failure assessment (SOFA) and Ranson scores, and laboratory indicators, such as glucose-to-lymphocyte ratio, have been used to assess the prognosis of AP (9-12). However, the predictive performance of the single laboratory indicator is likely to be affected by its fluctuation in accuracy. In addition, despite involvement of about 10 variables, SOFA and Ranson scores both need to be dynamically recorded and their application is limited in early prediction (13). Therefore, it is essential to establish a predictive model that has better accuracy when assessing the prognosis of AP.
Previous studies showed that clinicians were able to predict the mortality in the intensive care unit (ICU), and their notes were valuable to the health status of patients (14-16). The sentiments in clinical notes can reflect the attitude or impression of clinicians to patients, which can be measured through sentiment analysis. These sentiments are found to change with time, clinical characteristics, and outcomes, and are correlated with readmission and mortality (17). In contrast to structured data, the use of unstructured data such as nursing notes showed greater potential to predict mortality (15). To the best of our knowledge, the value of nursing notes in predicting in-hospital mortality of AP is unclear. Herein, we developed a predictive model combined with sentiment scores in nursing notes for in-hospital mortality in AP patients and validated its performance in the prediction of in-hospital mortality. We present the following article in accordance with the TRIPOD reporting checklist (available at https://atm.amegroups.com/article/view/10.21037/atm-22-1613/rc).
Methods
Study design and population
The data used in this retrospective cohort study were accessed from Medical Information Mart for Intensive Care III (MIMIC-III), a large, freely available, public database. MIMIC-III encompasses deidentified health-associated data related to 53,423 hospital admissions for the patients aged ≥16 years who stayed in critical care units at a large tertiary care hospital (Beth Israel Deaconess Medical Center, BIDMC) between 2001 and 2012 (18). The project was initiated by collaborating research groups and researchers from the Massachusetts Institute of Technology (MIT) Laboratory for Computational Physiology and was approved by the institutional review boards of the BIDMC and MIT, with a waiver of informed consent. The study was conducted in accordance with the Declaration of Helsinki (as revised in 2013).
Data from patients diagnosed with AP were collected from the MIMIC-III through the computer code of International Classification of Diseases. AP was diagnosed based on the following conditions: (I) abdominal pain related to AP; (II) imaging evidence of AP through computed tomography (CT) scanning and/or ultrasonography; and (III) at least 3-fold increase of lipase and/or amylase levels compared with the normal threshold (13). Patients aged <18 years and those without clinical notes were excluded.
Study variables
Sentiments in the clinical notes were assessed through sentiment analysis, which is an approach of classifying or quantifying the subjective properties of the written text (19). For each individual clinical note, sentiment polarity scores and sentiment subjectivity scores were assigned. In this study, Python’s TextBlob library was used to analyze the sentiment in clinical notes via natural language processing and text analysis (20). The AFINN sentiment lexicon from the polarity scale of −1 (most negative) to 1 (most positive) was used. In addition, subjectivity analysis for each individual clinical note was performed using Python’s TextBlob library and was labeled, with a range of 0 (objective) to 1 (subjective). Higher scores represented more positive and more subjective sentiments, respectively.
Covariates
The health-related data were all extracted from the MIMIC-III, including demographics (age, gender, marital status, ethnicity, etc.), vital signs (temperature, respiratory rate, heart rate and blood pressure), laboratory test indicators [oxygen saturation (SpO2), oxygen partial pressure (PO2), partial pressure of carbon dioxide (PCO2), white blood cells (WBC), red blood cells (RBC), platelets (PLT), RDW, international normalized ratio (INR), mean corpuscular volume (MCV), glucose, hematocrit, hemoglobin, total bilirubin (TBIL), aspartate aminotransferase (AST), alanine aminotransferase (ALT), creatinine, blood urea nitrogen (BUN), albumin, etc.], fluid balance (sodium, potassium, phosphate, calcium, pH value, etc.), first and last care unit [coronary care unit (CCU), cardiac surgery recovery unit (CSRU), medical/surgical/trauma or surgical intensive care unit (MICU/SICU/TSICU)], ICU length of stay (LOS), hospital LOS, medical history [chronic obstructive pulmonary disease (COPD), lung cancer, liver cirrhosis, etc.], subjective mean, subjective minimum, polarity mean and polarity minimum. Moreover, SOFA score and simplified acute physiology score-II (SAPS-II) were used to evaluate the severity of AP according to the MIMIC-III data.
Outcomes
The in-hospital mortality of AP patients was the outcome in this cohort. The follow-up period was the entire hospital stay. Follow-up was terminated if the patient died while hospitalized. A total of 631 patients were included in the study for analysis. Of these, 88 cases died during hospitalization.
Statistical analysis
In this study, a normality test for measurement data was performed with the Kolmogorov-Smirnov test. The measurement data with a normal distribution were compared by t-test and described as the mean ± standard deviation (), while those with an abnormal distribution were expressed as the median and interquartile ranges [M (Q1, Q3)] and compared with the Mann-Whitney U rank-sum test. Categorical variables were compared with the χ2 test or Fisher’s exact test, with results presented as cases and percentages [n (%)]. Five randomized imputations were performed on the missing data using the mice package. For continuous variables, the mean value was taken based on the results of randomized imputations, while for categorical variables the mode was adopted to fill the missing values and to generate the final dataset.
Absence or presence of in-hospital mortality was the outcome and sentiment scores were the major research factor. Comparison among groups was conducted to identify the potential confounding factors, which were then included into the multivariate model through stepwise regression. Additionally, the association between sentiment scores and in-hospital mortality was also analyzed in multivariate logistic models, especially when the remaining variables screened by the stepwise regression were adjusted.
All patients were randomly assigned into the training group (n=410) and the testing group (n=221) with a proportion of 6.5:3.5. A predictive model for in-hospital mortality was developed in the training group using logistic regression analysis and then validated in the testing group. Various indicators, including the area under the curve (AUC), sensitivity and specificity, were used to assess the performance of this predictive model. Delong test was utilized to compare the predictive performance of this model, this model without sentiment scores, SOFA score, and SAPS-II score. The AUC value was over 0.8, indicating the performance of the model was good. Moreover, the clinical value of the predictive model was also examined using decision curves. The statistical power was calculated by PASS software (NCSS, Kaysville, Utah, USA; version 15), and the sample sizes of the training and testing samples were adequate for assessing the predictive validity. A two-sided P value less than 0.05 was considered statistically significant. All the data were managed by SAS software (SAS Institute Inc., Cary, NC, USA; version 9.4) and R package (version 3.6.3).
Results
Baseline information of AP patients
A total of 904 AP patients were identified in the MIMIC-III database. After excluding 2 cases aged <18 years and 271 cases that did not have clinical notes, 631 patients were finally included into the study for analysis. Among them, 543 cases (86.1%) survived, while 88 (13.9%) died during hospitalization. The baseline information of the surviving and dead patients is compared in Table 1.
Table 1
Variables | Total (n=631) | Survivors (n=543) | Non-survivors (n=88) | χ2/Z/t | P |
---|---|---|---|---|---|
Gender | 1.596 | 0.206 | |||
Female | 283 (44.85) | 249 (45.86) | 34 (38.64) | ||
Male | 348 (55.15) | 294 (54.14) | 54 (61.36) | ||
Age, years | 60.38 (47.08, 72.54) | 59.40 (46.26, 71.61) | 66.45 (52.19, 81.58) | 3.339 | <0.001 |
Marital status | 1.166 | 0.761 | |||
Married | 312 (49.45) | 265 (48.80) | 47 (53.41) | ||
Separated/divorced | 54 (8.56) | 47 (8.66) | 7 (7.95) | ||
Single | 186 (29.48) | 164 (30.20) | 22 (25.00) | ||
Widowed | 79 (12.52) | 67 (12.34) | 12 (13.64) | ||
Ethnicity | – | 0.965 | |||
White | 516 (81.77) | 441 (81.22) | 75 (85.23) | ||
Asian | 15 (2.38) | 14 (2.58) | 1 (1.14) | ||
Black | 62 (9.83) | 54 (9.94) | 8 (9.09) | ||
Hispanic | 21 (3.33) | 19 (3.50) | 2 (2.27) | ||
Others | 17 (2.69) | 15 (2.76) | 2 (2.27) | ||
First care unit | 6.293 | 0.178 | |||
CCU | 43 (6.81) | 34 (6.26) | 9 (10.23) | ||
CSRU | 32 (5.07) | 30 (5.52) | 2 (2.27) | ||
MICU | 367 (58.16) | 310 (57.09) | 57 (64.77) | ||
SICU | 130 (20.60) | 117 (21.55) | 13 (14.77) | ||
TSICU | 59 (9.35) | 52 (9.58) | 7 (7.95) | ||
Last care unit | 3.567 | 0.468 | |||
CCU | 31 (4.91) | 24 (4.42) | 7 (7.95) | ||
CSRU | 32 (5.07) | 29 (5.34) | 3 (3.41) | ||
MICU | 358 (56.74) | 305 (56.17) | 53 (60.23) | ||
SICU | 150 (23.77) | 131 (24.13) | 19 (21.59) | ||
TSICU | 60 (9.51) | 54 (9.94) | 6 (6.82) | ||
ICU LOS, days | 4.27 (2.02, 11.92) | 3.87 (1.89, 10.72) | 9.19 (4.65, 17.85) | 5.239 | <0.001 |
RR, insp/min | 20.80±6.53 | 20.53±6.56 | 22.49±6.06 | −2.63 | 0.009 |
Temperature, ℃ | 36.94±1.09 | 37.00±1.06 | 36.62±1.17 | 3.04 | 0.002 |
Heart rate, bpm | 98.37±21.89 | 98.31±22.03 | 98.77±21.14 | −0.19 | 0.853 |
SBP, mmHg | 128.96±27.46 | 129.88±27.71 | 123.27±25.33 | 2.10 | 0.036 |
DBP, mmHg | 67.21±17.41 | 67.92±17.43 | 62.82±16.74 | 2.56 | 0.011 |
MAP, mmHg | 85.24±17.90 | 85.93±17.99 | 81.01±16.81 | 2.40 | 0.017 |
SpO2, % | 96.41±4.18 | 96.55±3.75 | 95.53±6.15 | 1.51 | 0.134 |
White blood cells, ×109/L | 12.70 (8.80, 17.60) | 12.60 (8.80, 17.60) | 13.35 (8.90, 17.60) | 0.428 | 0.668 |
Red blood cells, ×109/L | 3.93±0.86 | 3.97±0.85 | 3.69±0.84 | 2.95 | 0.003 |
Sodium, mEq/L | 138.05±5.78 | 138.15±5.72 | 137.42±6.14 | 1.10 | 0.273 |
Potassium, mEq/L | 4.19±0.89 | 4.18±0.88 | 4.28±0.96 | −1.02 | 0.307 |
Phosphate, mg/dL | 3.30 (2.50, 4.30) | 3.20 (2.40, 4.20) | 3.80 (2.85, 5.40) | 3.421 | <0.001 |
Calcium, mg/dL | 8.31±1.37 | 8.35±1.39 | 8.11±1.23 | 1.49 | 0.136 |
Platelets, ×109/L | 224.00 (159.00, 302.00) | 226.00 (165.00, 308.00) | 207.50 (118.00, 286.50) | −2.391 | 0.017 |
pH | 7.35±0.11 | 7.36±0.11 | 7.34±0.15 | 1.13 | 0.263 |
Lactate, mmol/L | 1.80 (1.30, 2.90) | 1.80 (1.30, 2.80) | 1.90 (1.30, 3.65) | 1.449 | 0.147 |
INR | 1.20 (1.10, 1.50) | 1.20 (1.10, 1.50) | 1.30 (1.10, 1.80) | 2.694 | 0.007 |
MCV, fL | 91.35±8.05 | 91.02±7.62 | 93.41±10.12 | −2.12 | 0.036 |
Magnesium, mg/dL | 1.90±0.44 | 1.89±0.44 | 1.95±0.44 | −1.18 | 0.238 |
Glucose, mg/dL | 129.00 (103.00, 173.00) | 129.00 (103.00, 168.00) | 129.50 (107.00, 187.50) | 1.157 | 0.247 |
Creatinine, mg/dL | 1.10 (0.80, 1.90) | 1.10 (0.80, 1.80) | 1.30 (0.80, 2.30) | 1.998 | 0.046 |
BUN, mg/dL | 22.00 (14.00, 40.00) | 21.00 (13.00, 36.00) | 31.00 (17.50, 51.50) | 3.949 | <0.001 |
Bicarbonate, mEq/L | 22.31±5.86 | 22.34±5.83 | 22.09±6.06 | 0.37 | 0.711 |
Neutrophil, % | 77.55±15.26 | 77.63±14.65 | 77.11±18.66 | 0.25 | 0.803 |
Lymphocytes, % | 8.70 (5.00, 14.90) | 9.00 (5.20, 15.00) | 7.00 (4.00, 11.00) | −2.900 | 0.004 |
Albumin, g/dL | 3.15±0.71 | 3.20±0.71 | 2.85±0.67 | 4.45 | <0.001 |
TBIL, mg/dL | 0.90 (0.50, 2.20) | 0.80 (0.50, 2.00) | 1.25 (0.60, 4.70) | 3.251 | 0.001 |
Hematocrit, % | 35.68±7.08 | 35.94±7.02 | 34.12±7.24 | 2.25 | 0.025 |
PO2, mmHg | 97.00 (71.00, 158.00) | 99.80 (71.00, 159.80) | 92.50 (73.50, 138.80) | −1.057 | 0.291 |
Hemoglobin, g/dL | 12.02±2.48 | 12.13±2.46 | 11.32±2.49 | 2.89 | 0.004 |
MCHC, % | 33.71±1.63 | 33.80±1.60 | 33.13±1.67 | 3.60 | <0.001 |
ALP, IU/L | 105.00 (70.00, 175.00) | 104.00 (69.00, 171.00) | 119.50 (75.50, 225.00) | 2.133 | 0.033 |
PCO2, mmHg | 38.80 (33.00, 46.00) | 39.00 (33.20, 46.00) | 37.00 (31.50, 48.00) | 0.918 | 0.359 |
RDW, % | 14.92±2.02 | 14.76±1.87 | 15.89±2.57 | −3.96 | <0.001 |
ALT, IU/L | 42.00 (23.00, 129.00) | 42.00 (21.00, 129.00) | 45.00 (25.50, 145.00) | 1.275 | 0.202 |
AST, IU/L | 57.00 (28.00, 138.00) | 56.00 (28.00, 138.00) | 73.50 (32.00, 162.00) | 1.548 | 0.122 |
Amylase, IU/L | 180.00 (74.00, 583.00) | 185.00 (77.00, 590.00) | 161.50 (69.00, 532.00) | −0.859 | 0.391 |
Lipase, IU/L | 188.00 (53.00, 945.00) | 193.00 (58.00, 1,027.00) | 169.00 (37.50, 740.50) | −1.638 | 0.101 |
COPD | 61 (9.67) | 56 (10.31) | 5 (5.68) | 1.860 | 0.173 |
Lung cancer | 7 (1.11) | 3 (0.55) | 4 (4.55) | – | 0.009 |
Atrial fibrillation | 143 (22.66) | 114 (20.99) | 29 (32.95) | 6.180 | 0.013 |
Liver cirrhosis | 46 (7.29) | 34 (6.26) | 12 (13.64) | 6.094 | 0.014 |
Congestive heart failure | 188 (29.79) | 155 (28.55) | 33 (37.50) | 2.903 | 0.088 |
Heart disease | 28 (4.44) | 26 (4.79) | 2 (2.27) | – | 0.407 |
Diabetes mellitus | 167 (26.47) | 137 (25.23) | 30 (34.09) | 3.055 | 0.080 |
Respiratory failure | 246 (38.99) | 193 (35.54) | 53 (60.23) | 19.398 | <0.001 |
Hyperlipidemia | 159 (25.20) | 153 (28.18) | 6 (6.82) | 18.328 | <0.001 |
Renal failure | 295 (46.75) | 233 (42.91) | 62 (70.45) | 23.080 | <0.001 |
Malignant cancer | 92 (14.58) | 76 (14.00) | 16 (18.18) | 1.065 | 0.302 |
SAPS-II | 36.00 (27.00, 45.00) | 34.00 (25.00, 44.00) | 47.00 (37.50, 62.50) | 7.554 | <0.001 |
SOFA score | 5.00 (3.00, 8.00) | 5.00 (3.00, 8.00) | 8.00 (5.00, 10.50) | 5.621 | <0.001 |
LOS, day | 13.72 (7.27, 24.01) | 12.92 (7.15, 23.37) | 15.56 (7.95, 30.78) | 1.577 | 0.115 |
Subjective mean | 5.16±1.28 | 5.13±1.26 | 5.34±1.39 | −1.44 | 0.150 |
Subjective minimum | 2.40 (1.00, 3.13) | 2.50 (1.00, 3.18) | 2.01 (0.00, 2.83) | −2.392 | 0.017 |
Polarity mean | 0.58 (0.36, 0.89) | 0.62 (0.37, 0.95) | 0.47 (0.18, 0.64) | −4.320 | <0.001 |
Polarity minimum | −0.42 (−1.06, 0.03) | −0.38 (−1.00, 0.05) | −0.75 (−1.41, −0.24) | −3.797 | <0.001 |
“–” represents Fisher’s exact test. ICU, intensive care unit; CCU, coronary care unit; CSRU, cardiac surgery recovery unit; MICU/SICU/TSICU, medical/surgical/trauma or surgical intensive care unit, LOS, length of stay; RR, respiratory rate; SBP, systolic blood pressure; DBP, diastolic blood pressure; MAP, mean atrial pressure; SpO2, oxygen saturation; INR, international normalized ratio; MCV, mean corpuscular volume; BUN, blood urea nitrogen; TBIL, total bilirubin; PO2, oxygen partial pressure; MCHC, mean corpuscular hemoglobin concentration; ALP, alkaline phosphatase; PCO2, partial pressure of carbon dioxide; RDW, red cell distribution width; ALT, alanine aminotransferase; AST, aspartate aminotransferase; COPD, chronic obstructive pulmonary disease; SAPS-II, Simplified Acute Physiology Score-II; SOFA, sequential organ failure assessment.
Results showed that there were significant differences in multiple variables, such as age (P<0.001), ICU LOS (P<0.001), respiratory rate (P=0.009), temperature (P=0.002), systolic blood pressure (P=0.036), diastolic blood pressure (P=0.011), mean arterial pressure (P=0.017), red blood cells (P=0.003), phosphate (P<0.001), INR (P=0.007), MCV (P=0.036), creatinine (P=0.046), BUN (P<0.001), lymphocytes (P=0.004), albumin (P<0.001), TBIL (P=0.001), hematocrit (P=0.025), hemoglobin (P=0.004), MCHC (P<0.001), ALP (P=0.033), RDW (P<0.001), history of lung cancer (P=0.009), atrial fibrillation (P=0.013), liver cirrhosis (P=0.014), respiratory failure (P<0.001), hyperlipidemia (P<0.001) and renal failure (P<0.001), SAPS-II (P<0.001), SOFA scores (P<0.001), subjective minimum (P=0.017), polarity mean (P<0.001), and polarity minimum (P<0.001).
Association between sentiment scores and in-hospital morality
As shown in Table 2, the correlation between sentiment scores and in-hospital mortality was analyzed in each of the three models. In the original model (model 1), subjective minimum [odds ratio (OR): 0.819; 95% confidence interval (CI): 0.694–0.966; P=0.018], polarity mean (OR: 0.353; 95% CI: 0.210–0.577; P<0.001), and polarity minimum (OR: 0.756; 95% CI: 0.651–0.888; P<0.001) were all shown to be protective factors for in-hospital mortality in AP patients. After the confounding factors including age, gender, ethnicity, and ICU LOS were corrected, polarity mean (OR: 0.391; 95% CI: 0.226–0.656; P<0.001; model 2) was found to be associated with a reduced risk of in-hospital mortality. After adjusting for confounding factors in model 2 and the remaining covariates screened by stepwise regression, such as respiratory rate, TBIL, hemoglobin, lung cancer, respiratory failure, hyperlipidemia and renal failure, polarity mean (OR: 0.448; 95% CI: 0.233–0.833; P=0.014; model 3) remained an independent protective factor for in-hospital mortality in AP patients, which was consistent with the results before data imputation (Table 3).
Table 2
Variables | Model 1 | Model 2 | Model 3 | |||||
---|---|---|---|---|---|---|---|---|
OR (95% CI) | P | OR (95% CI) | P | OR (95% CI) | P | |||
Subjective mean | 1.129 (0.953–1.326) | 0.15 | 0.998 (0.815–1.206) | 0.982 | 0.974 (0.785–1.195) | 0.807 | ||
Subjective minimum | 0.819 (0.694–0.966) | 0.018 | 0.932 (0.772–1.129) | 0.471 | 0.967 (0.785–1.195) | 0.757 | ||
Polarity mean | 0.353 (0.210–0.577) | <0.001 | 0.391 (0.226–0.656) | <0.001 | 0.448 (0.233–0.833) | 0.014 | ||
Polarity minimum | 0.756 (0.651–0.888) | <0.001 | 0.867 (0.715–1.055) | 0.148 | 0.896 (0.723–1.117) | 0.319 |
Model 1, the original model; Model 2, the model after adjusting for the variables age, gender, ethnicity, and ICU length of stay; Model 3, the model after adjusting for the variables in model 2 and the remaining variables (respiratory rate, total bilirubin, hemoglobin, lung cancer, respiratory failure, hyperlipidemia, and renal failure) screened by stepwise regression. OR, odds ratio; CI, confidence interval.
Table 3
Variables | Model 1 | Model 2 | Model 3 | |||||
---|---|---|---|---|---|---|---|---|
OR (95% CI) | P | OR (95% CI) | P | OR (95% CI) | P | |||
Subjective mean | 1.129 (0.953–1.326) | 0.150 | 0.997 (0.788–1.234) | 0.979 | 0.971 (0.757–1.223) | 0.810 | ||
Subjective minimum | 0.819 (0.694–0.966) | 0.018 | 0.906 (0.727–1.132) | 0.383 | 0.956 (0.752–1.217) | 0.712 | ||
Polarity mean | 0.353 (0.210–0.577) | <0.001 | 0.409 (0.234–0.713) | 0.002 | 0.481 (0.231–0.971) | 0.046 | ||
Polarity minimum | 0.756 (0.651–0.888) | <0.001 | 0.871 (0.714–1.063) | 0.173 | 0.936 (0.719–1.246) | 0.637 |
Model 1, the original model; Model 2, the model after adjusting for the variables age, gender, ethnicity, and ICU length of stay; Model 3, the model after adjusting for the variables in model 2 and the remaining variables (respiratory rate, total bilirubin, hemoglobin, lung cancer, respiratory failure, hyperlipidemia, and renal failure) screened by stepwise regression. OR, odds ratio; CI, confidence interval.
Establishment of a predictive model for in-hospital mortality
According to the proportion of 6.5:3.5, the patients were divided into the training group (n=410) and the testing group (n=221). In comparison to the baseline characteristics, no significant differences were identified between the training group and the testing group (all P>0.05; Table 4), which suggested balanced and comparable data between these two groups.
Table 4
Variables | Training (n=410) | Testing (n=221) | χ2/Z/t | P |
---|---|---|---|---|
Gender | 0.035 | 0.851 | ||
Female | 185 (45.12) | 98 (44.34) | ||
Male | 225 (54.88) | 123 (55.66) | ||
Marital status | 2.992 | 0.393 | ||
Married | 193 (47.07) | 119 (53.85) | ||
Separated/divorced | 36 (8.78) | 18 (8.14) | ||
Single | 129 (31.46) | 57 (25.79) | ||
Widowed | 52 (12.68) | 27 (12.22) | ||
Ethnicity | 1.501 | 0.827 | ||
White | 334 (81.46) | 182 (82.35) | ||
Asian | 8 (1.95) | 7 (3.17) | ||
Black | 42 (10.24) | 20 (9.05) | ||
Hispanic | 15 (3.66) | 6 (2.71) | ||
Others | 11 (2.68) | 6 (2.71) | ||
ICU LOS, days | 4.49 (2.06, 12.70) | 4.04 (1.89, 11.55) | −0.755 | 0.450 |
Age, years | 59.81 (46.93, 71.61) | 61.20 (47.41, 74.30) | 1.135 | 0.256 |
RR, insp/min | 20.94±6.77 | 20.54±6.06 | −0.72 | 0.471 |
TBIL, mg/dL | 0.80 (0.50, 2.10) | 0.90 (0.50, 2.24) | 0.873 | 0.383 |
Hemoglobin, g/dL | 12.11±2.49 | 11.85±2.46 | −1.25 | 0.212 |
Lung cancer | – | 0.431 | ||
No | 404 (98.54) | 220 (99.55) | ||
Yes | 6 (1.46) | 1 (0.45) | ||
Respiratory failure | 3.645 | 0.056 | ||
No | 239 (58.29) | 146 (66.06) | ||
Yes | 171 (41.71) | 75 (33.94) | ||
Hyperlipidemia | 1.652 | 0.199 | ||
No | 300 (73.17) | 172 (77.83) | ||
Yes | 110 (26.83) | 49 (22.17) | ||
Renal failure | 0.792 | 0.374 | ||
No | 213 (51.95) | 123 (55.66) | ||
Yes | 197 (48.05) | 98 (44.34) | ||
SAPS-II | 36.00 (27.00, 46.00) | 36.00 (26.00, 44.00) | −0.271 | 0.786 |
SOFA score | 5.00 (3.00, 9.00) | 6.00 (3.00, 8.00) | 0.073 | 0.942 |
Subjective mean | 5.19±1.31 | 5.10±1.23 | −0.85 | 0.395 |
Subjective minimum | 2.34 (0.67, 3.09) | 2.50 (1.20, 3.21) | 1.631 | 0.103 |
Polarity mean | 0.57 (0.36, 0.86) | 0.62 (0.35, 0.98) | 1.253 | 0.210 |
Polarity minimum | −0.43 (−1.06, 0.00) | −0.41 (−1.06, 0.05) | 0.648 | 0.517 |
In-hospital mortality | 0.081 | 0.776 | ||
Survivors | 354 (86.34) | 189 (85.52) | ||
Non-survivors | 56 (13.66) | 32 (14.48) |
“–” represents Fisher’s exact test. ICU, intensive care unit; LOS, length of stay; RR, respiratory rate; TBIL, total bilirubin; SAPS-II, Simplified Acute Physiology Score-II; SOFA, sequential organ failure assessment.
Using all variables in model 3 as the predictors, a predictive model for in-hospital mortality was established in the training group via logistic regression analysis, namely ln(p/1-p) = −4.61 − 1.06005 × polarity mean + 0.04 × age + 0.54 × gender (male) −1.116 × Asian + 0.71 × Black − 0.33 × Hispanic-14.88 × others + 0.02 × ICU LOS + 0.05 × respiratory rate + 0.07 × TBIL-0.11 × hemoglobin + 3.92 × lung cancer + 0.55 × respiratory failure − 1.56 × hyperlipidemia + 0.69 × renal failure. When this model was validated in the testing group, it showed a sensitivity of 0.656, a specificity of 0.815, and an AUC of 0.812 (Table 5).
Table 5
Variables | Selected model | Selected model without sentiments | SOFA score | SAPS-II |
---|---|---|---|---|
Cut-off value | 0.153 | 0.150 | 0.126 | 0.160 |
Training group | ||||
Sensitivity (95% CI) | 0.786 (0.678–0.893) | 0.696 (0.576–0.817) | 0.696 (0.576–0.817) | 0.607 (0.479–0.735)* |
Specificity (95% CI) | 0.760 (0.715–0.804) | 0.732 (0.685–0.778) | 0.540 (0.488–0.591)* | 0.749 (0.703–0.794) |
PPV (95% CI) | 0.341 (0.259–0.423) | 0.291 (0.214–0.368) | 0.193 (0.139–0.248)* | 0.276 (0.197–0.355) |
NPV (95% CI) | 0.957 (0.934–0.981) | 0.938 (0.910–0.967) | 0.918 (0.881–0.956) | 0.923 (0.893–0.954) |
AUC (95% CI) | 0.840 (0.838–0.842) | 0.759 (0.692–0.826)* | 0.661 (0.659–0.663)* | 0.725 (0.723–0.728)* |
Accuracy (95% CI) | 0.763 (0.722–0.805) | 0.727 (0.684–0.770) | 0.561 (0.513–0.609)* | 0.729 (0.686–0.772) |
Testing group | ||||
Sensitivity (95% CI) | 0.656 (0.492–0.821) | 0.719 (0.563–0.875) | 0.812 (0.677–0.948) | 0.594 (0.424–0.764) |
Specificity (95% CI) | 0.815 (0.759–0.870) | 0.720 (0.656–0.784) | 0.545 (0.474–0.616)* | 0.831 (0.777–0.884) |
PPV (95% CI) | 0.375 (0.248–0.502) | 0.303 (0.199–0.406) | 0.232 (0.154–0.310) | 0.373 (0.240–0.505) |
NPV (95% CI) | 0.933 (0.895–0.971) | 0.938 (0.899–0.977) | 0.945 (0.902–0.988) | 0.924 (0.884–0.963) |
AUC (95% CI) | 0.812 (0.809–0.815) | 0.793 (0.708–0.879)* | 0.732 (0.729–0.735)* | 0.792 (0.790–0.795)* |
Accuracy (95% CI) | 0.792 (0.738–0.845) | 0.719 (0.660–0.779) | 0.584 (0.519–0.649)* | 0.796 (0.743–0.849) |
“*” represents the value of P less than 0.05 by comparison to the model established. CI, confidence interval; PPV, positive predictive value; NPV, negative predictive value; AUC, area under the curve; SAPS-II, Simplified Acute Physiology Score-II; SOFA, sequential organ failure assessment.
Performance and clinical value of the predictive model
The assessment indicators of sensitivity, specificity, positive predictive value (PPV), negative predictive value (NPV), AUC, and accuracy were used to compare the performance of this predictive model, this predictive model without sentiment scores, SOFA score and SAPS-II score in the prediction of in-hospital mortality. As shown in Table 5, the AUC of this model was up to 0.840 in the training group and 0.812 in the testing group, which was significantly higher than those of this predictive model without sentiment scores (0.759 and 0.793; P<0.05), SOFA (0.661 and 0.584; P<0.05) and SAPS-II (0.725 and 0.792; P<0.05). Additionally, the specificity and accuracy of the predictive model were both superior to SOFA (training group, specificity: 0.760 vs. 0.540, accuracy: 0.763 vs. 0.561, P<0.05; testing group; specificity: 0.760 vs. 0.540, accuracy: 0.763 vs. 0.561, P<0.05). The receiver operating characteristic curves of the different models in the prediction of in-hospital mortality is shown in Figure 1.
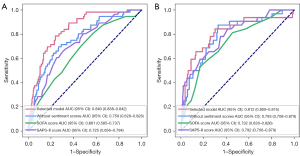
Decision-making curves were used to compare the clinical value of different models. Results showed that, under the same level and types of risk, the clinical benefits of the predictive model were the highest compared with SOFA and SAPS-II (Figure 2).
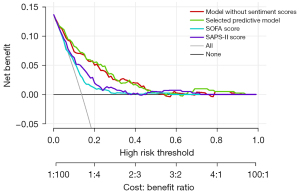
A predictive nomogram for in-hospital mortality
A nomogram was constructed based on the predictors included in the predictive model (Figure 3). The Hosmer-Lemeshow test of goodness of fit was performed on the predictive model. The P value of goodness of fit was 0.807, larger than 0.05, which suggested a good fit ability of the predictive model.
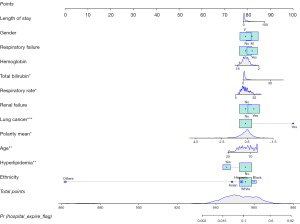
For example, the 15th patient from the dataset was a Hispanic female aged 24 years, with ICU LOS of 1 day and respiratory rate of 24 times/min. The levels of hemoglobin and TBIL were 9.8 g/dL and 0.6 mg/dL, respectively. She had renal failure, hyperlipidemia, and respiratory failure, but no lung cancer. The final predictive scores were 945, with the probability of in-hospital mortality of 0.0185 (Figure 4). This patient survived, which suggested that the nomogram in predicting in-hospital mortality was accurate.
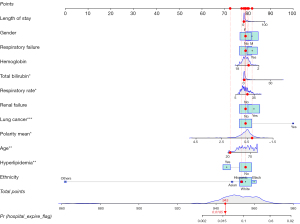
Discussion
In this study, a total of 631 AP patients were eligible for analysis, with the in-hospital mortality rate of 13.9%. Analysis of the association between sentiment scores and in-hospital mortality indicated by the sentiment polarity mean was correlated with a lowered risk of in-hospital mortality. Through multivariate logistic regression analysis, 12 independent variables were screened out to establish a predictive model for in-hospital mortality, which represented better performance and clinical value than other models. Additionally, a nomogram constructed based on the predictors further demonstrated the accuracy of the predictive model for in-hospital mortality of AP patients.
Severity of disease scoring systems, such as SOFA and SAPS II, are usually used to predict mortality in the ICU. These scoring systems are computed through the coded data of laboratory test results, demographics, and vital signs accessed from the electronic health record (EHR) of patients, but they have poor generalizability across diseases and countries (15,21,22). A total of 80% of all EHRs contain unstructured data, such as clinician-written notes with crucial information on the physical condition and trajectory of patients (23,24). Although the unstructured form of clinical notes enables clinicians to document rich, accurate, and domain-specific information, they are difficult to quantify and are easy to neglect in the structured fields of the EHR (15,25).
Previous studies indicated that the sentiment in clinical notes can be measured using natural language processing tools and is associated with the likelihood of mortality and readmission to ICU (17). Quantitative assessment of the sentiment in clinical notes related to the 30-day mortality of patients in the ICU, and there was a positive association between the sentiment polarity mean and patients’ survival (19). In this study, Python’s TextBlob library was applied to measure the sentiment in clinical notes via natural language processing and text analysis, and sentiment polarity mean was found to be associated with a reduced risk of in-hospital mortality in AP, similar to the results of Waudby-Smith et al. (19). Based on this, a predictive model for in-hospital mortality in AP was developed via multivariate logistic regression analysis. In the prediction of in-hospital mortality, this predictive model showed the largest AUC and the highest clinical benefits compared to the model without sentiment scores, SOFA and SAPS-II. SOFA score is mainly used to assess organ failure, which enables it to predict clinical outcomes in critically ill patients (26). However, the AUC of SOFA in this study was less than 0.7, which indicated a poor predictive performance for in-hospital mortality. This might be partially explained by the fact that the organ dysfunction was often easy to detect and treat in the early phase of AP, but deterioration of organ dysfunction was correlated with mortality and poor clinical outcomes (27). Based on a large international sample of patients, SAPS-II was developed to estimate the mortality risk without having to specify the primary diagnosis (28). As the most common tool in ICU, SAPS-II consisted of 12 immediate variables that should be used the first 24 hours of admission to the ICU; age and comorbidities obtained prior to admission were also taken into consideration (29). However, it is probable to misdiagnose the severity in the early stage of AP, thereby restricting the application of SAPS-II in the early prognostic assessment of AP (30,31).
To the best of our knowledge, this was the first study to develop a predictive model with well performance and clinical value for in-hospital mortality in AP using the sentiment in clinical notes, which highlighted the potential of unstructured data to improve mortality prediction. Moreover, the visualized nomogram performed well, which further demonstrated the accuracy of this predictive model to assess the prognosis of AP patients. Nevertheless, several limitations mean our findings should be interpreted with caution. First, the data used in our study were obtained from MIMIC-III database where most patients were Americans, therefore this predictive model may have limitations when applied to other ethnicities. Second, some important factors related to poor prognosis of AP patients were missing in MIMIC-III, such as interlukin-6 (32). Third, no external validation was used to further validate the performance of the model. Fourth, despite having a relatively large sample size it was a database-based retrospective study. In the future, more prospective studies with larger samples should be performed to validate our results and to enhance the clinical application of this predictive model for in-hospital mortality in AP.
Conclusions
Our findings demonstrated that sentiment polarity mean was a significant protective factor for in-hospital mortality in AP. Based on this, a predictive model was developed. This model showed well performance and clinical value in the prediction of in-hospital mortality in AP patients.
Acknowledgments
Funding: None.
Footnote
Reporting Checklist: The authors have completed the TRIPOD reporting checklist. Available at https://atm.amegroups.com/article/view/10.21037/atm-22-1613/rc
Conflicts of Interest: All authors have completed the ICMJE uniform disclosure form (available at https://atm.amegroups.com/article/view/10.21037/atm-22-1613/coif). The authors have no conflicts of interest to declare.
Ethical Statement: The authors are accountable for all aspects of the work in ensuring that questions related to the accuracy or integrity of any part of the work are appropriately investigated and resolved. The study was conducted in accordance with the Declaration of Helsinki (as revised in 2013).
Open Access Statement: This is an Open Access article distributed in accordance with the Creative Commons Attribution-NonCommercial-NoDerivs 4.0 International License (CC BY-NC-ND 4.0), which permits the non-commercial replication and distribution of the article with the strict proviso that no changes or edits are made and the original work is properly cited (including links to both the formal publication through the relevant DOI and the license). See: https://creativecommons.org/licenses/by-nc-nd/4.0/.
References
- Shi N, Sun GD, Ji YY, et al. Effects of acute kidney injury on acute pancreatitis patients' survival rate in intensive care unit: A retrospective study. World J Gastroenterol 2021;27:6453-64. [Crossref] [PubMed]
- Lee PJ, Papachristou GI. New insights into acute pancreatitis. Nat Rev Gastroenterol Hepatol 2019;16:479-96. [Crossref] [PubMed]
- Peery AF, Crockett SD, Barritt AS, et al. Burden of Gastrointestinal, Liver, and Pancreatic Diseases in the United States. Gastroenterology 2015;149:1731-1741.e3. [Crossref] [PubMed]
- Wadhwa V, Patwardhan S, Garg SK, et al. Health Care Utilization and Costs Associated With Acute Pancreatitis. Pancreas 2017;46:410-5. [Crossref] [PubMed]
- Banks PA, Bollen TL, Dervenis C, et al. Classification of acute pancreatitis--2012: revision of the Atlanta classification and definitions by international consensus. Gut 2013;62:102-11. [Crossref] [PubMed]
- Zerem E. Treatment of severe acute pancreatitis and its complications. World J Gastroenterol 2014;20:13879-92. [Crossref] [PubMed]
- Yasuda H, Horibe M, Sanui M, et al. Etiology and mortality in severe acute pancreatitis: A multicenter study in Japan. Pancreatology 2020;20:307-17. [Crossref] [PubMed]
- Machicado JD, Gougol A, Stello K, et al. Acute Pancreatitis Has a Long-term Deleterious Effect on Physical Health Related Quality of Life. Clin Gastroenterol Hepatol 2017;15:1435-1443.e2. [Crossref] [PubMed]
- Zhou H, Mei X, He X, et al. Severity stratification and prognostic prediction of patients with acute pancreatitis at early phase: A retrospective study. Medicine (Baltimore) 2019;98:e15275. [Crossref] [PubMed]
- Vasudevan S, Goswami P, Sonika U, et al. Comparison of Various Scoring Systems and Biochemical Markers in Predicting the Outcome in Acute Pancreatitis. Pancreas 2018;47:65-71. [Crossref] [PubMed]
- Chen Y, Tang S, Wang Y. Prognostic Value of Glucose-to-Lymphocyte Ratio in Critically Ill Patients with Acute Pancreatitis. Int J Gen Med 2021;14:5449-60. [Crossref] [PubMed]
- Han T, Cheng T, Liao Y, et al. Development and Validation of a Novel Prognostic Score Based on Thrombotic and Inflammatory Biomarkers for Predicting 28-Day Adverse Outcomes in Patients with Acute Pancreatitis. J Inflamm Res 2022;15:395-408. [Crossref] [PubMed]
- Ding N, Guo C, Li C, et al. An Artificial Neural Networks Model for Early Predicting In-Hospital Mortality in Acute Pancreatitis in MIMIC-III. Biomed Res Int 2021;2021:6638919. [Crossref] [PubMed]
- Zou Y, Wang J, Lei Z, et al. Sentiment analysis for necessary preview of 30-day mortality in sepsis patients and the control strategies. J Healthc Eng 2021;2021:1713363. [Crossref] [PubMed]
- Hashir M, Sawhney R. Towards unstructured mortality prediction with free-text clinical notes. J Biomed Inform 2020;108:103489. [Crossref] [PubMed]
- Gao Q, Wang D, Sun P, et al. Sentiment Analysis Based on the Nursing Notes on In-Hospital 28-Day Mortality of Sepsis Patients Utilizing the MIMIC-III Database. Comput Math Methods Med 2021;2021:3440778. [Crossref] [PubMed]
- McCoy TH, Castro VM, Cagan A, et al. Sentiment Measured in Hospital Discharge Notes Is Associated with Readmission and Mortality Risk: An Electronic Health Record Study. PLoS One 2015;10:e0136341. [Crossref] [PubMed]
- Johnson AE, Pollard TJ, Shen L, et al. MIMIC-III, a freely accessible critical care database. Sci Data 2016;3:160035. [Crossref] [PubMed]
- Waudby-Smith IER, Tran N, Dubin JA, et al. Sentiment in nursing notes as an indicator of out-of-hospital mortality in intensive care patients. PLoS One 2018;13:e0198687. [Crossref] [PubMed]
- Saleh SN, Lehmann CU, McDonald SA, et al. Understanding public perception of coronavirus disease 2019 (COVID-19) social distancing on Twitter. Infect Control Hosp Epidemiol 2021;42:131-8. [Crossref] [PubMed]
- Haniffa R, Isaam I, De Silva AP, et al. Performance of critical care prognostic scoring systems in low and middle-income countries: a systematic review. Crit Care 2018;22:18. [Crossref] [PubMed]
- Cheng JY. Mortality prediction in status epilepticus with the APACHE II score. J Intensive Care Soc 2017;18:310-7. [Crossref] [PubMed]
- Murdoch TB, Detsky AS. The inevitable application of big data to health care. JAMA 2013;309:1351-2. [Crossref] [PubMed]
- Boag Willie, Doss Dustin, Naumann Tristan, et al. What’s in a note? unpacking predictive value in clinical note representations. AMIA Jt Summits Transl Sci Proc 2017;26-34. [PubMed]
- Aylor M, Campbell EM, Winter C, et al. Resident Notes in an Electronic Health Record. Clin Pediatr (Phila) 2017;56:257-62. [Crossref] [PubMed]
- Lambden S, Laterre PF, Levy MM, et al. The SOFA score-development, utility and challenges of accurate assessment in clinical trials. Crit Care 2019;23:374. [Crossref] [PubMed]
- Yadav J, Yadav SK, Kumar S, et al. Predicting morbidity and mortality in acute pancreatitis in an Indian population: a comparative study of the BISAP score, Ranson's score and CT severity index. Gastroenterol Rep (Oxf) 2016;4:216-20. [Crossref] [PubMed]
- Haq A, Patil S, Parcells AL, et al. The Simplified Acute Physiology Score III Is Superior to the Simplified Acute Physiology Score II and Acute Physiology and Chronic Health Evaluation II in Predicting Surgical and ICU Mortality in the "Oldest Old". Curr Gerontol Geriatr Res 2014;2014:934852. [Crossref] [PubMed]
- Ferreira Ade F, Bartelega JA, Urbano HC, et al. Acute pancreatitis gravity predictive factors: which and when to use them? Arq Bras Cir Dig 2015;28:207-11. [Crossref] [PubMed]
- Domínguez-Muñoz JE, Carballo F, García MJ, et al. Evaluation of the clinical usefulness of APACHE II and SAPS systems in the initial prognostic classification of acute pancreatitis: a multicenter study. Pancreas 1993;8:682-6. [Crossref] [PubMed]
- Capuzzo M, Moreno RP, Le Gall JR. Outcome prediction in critical care: the Simplified Acute Physiology Score models. Curr Opin Crit Care 2008;14:485-90. [Crossref] [PubMed]
- Arutla M, Raghunath M, Deepika G, et al. Efficacy of enteral glutamine supplementation in patients with severe and predicted severe acute pancreatitis- A randomized controlled trial. Indian J Gastroenterol 2019;38:338-47. [Crossref] [PubMed]
(English Language Editor: C. Mullens)