Developing a prediction model for estimating adrenocorticotropic hormone changes in patients undergoing scheduled open hepatectomy
Introduction
Unlike other components of general anesthesia, such as hypnosis and muscle relaxation (1), currently, there is no gold standard for monitoring a patient’s response to noxious stimuli during intraoperative analgesia (2). Due to the interpatient variability in response to nociceptive input, optimal opioid titration remains a challenge for anesthetists.
Traditionally, analgesic administration is based on the changes in blood pressure (BP) and heart rate (HR), as well as surgical stimuli according to the clinical experience of individual anesthetists (3). Over the past two decades, several monitoring systems have been developed and marketed that assess the balance between nociception and analgesia using measures of the patient’s autonomic nervous system (ANS). Examples of such measures include the nociception level (NOL) index, the analgesia-nociception index (ANI), the surgical plethysmographic index (SPI), and pupillometry (4). All these techniques have been beneficial in the intraoperative administration of opioids. Recently, Funcke et al. compared the performance of SPI, pupillary pain index (PPI), and NOL. They demonstrated a difference in the ability of these monitoring methods to reflect nociception and clinical symptoms compared to the control group. A higher opioid dose was associated with a lower serum ACTH concentration (5). In addition, although the use of PPI reduced intraoperative opioid consumption, there was a higher incidence of unwanted movement and elevated stress hormones (6). These results suggested that further optimization of these monitoring methods is warranted in the clinical setting.
Stress stimuli, including surgical stimuli, lead to rapid activation of the sympathetic-adreno-medullar (SAM) axis and the hypothalamus-pituitary-adrenal (HPA) axis (7,8). Stress hormones such as ACTH have been used to evaluate surgical stress response (9), but it needs blood samples drawn from patients to detect their concentrations in the laboratories and it costs time. Therefore, it’s inconvenient and impossible to get simultaneously when the intraoperative stimulation comes. Since the above monitors/indices do not correlate well with the levels of stress hormones, this study was conducted to develop a prediction model for surgical stress based on ACTH fluctuations. Routine hemodynamic parameters and other baseline characteristics that correlate with the levels of blood ACTH fluctuation during surgery were used to develop the logistic regression model. Internal validation was performed using the bootstrapping technique to evaluate the performance of the prediction model. We present the following article in accordance with the TRIPOD reporting checklist (available at https://atm.amegroups.com/article/view/10.21037/atm-22-2276/rc).
Methods
This single-site prospective study was conducted in the Zhongshan Hospital, affiliated with Fudan University and approved by its Ethics Committee (No. B2018-288R). The study was conducted in accordance with the Declaration of Helsinki (as revised in 2013). Informed consent was taken from all the patients and all study procedures were approved prior to enrollment of patients. This trial was registered retrospectively with ClinicalTrials (registration number: NCT03892538).
A total of 205 patients undergoing scheduled open hepatectomy from October 2018 to March 2020 were enrolled in this study. The inclusion criteria were as follows: (I) patients with American Society of Anesthesiologist (ASA) I–III classification; (II) patients aged 18–80 years old; and (III) patients who underwent open hepatectomy. Patients presenting with adrenal gland-related diseases, immune system-related diseases, severe cardiovascular or cerebrovascular diseases, or those with severe liver and kidney dysfunction were excluded. Patients who refused to participate in this study were also excluded.
Data collection
The systolic blood pressure (SBP), diastolic blood pressure (DBP), mean arterial pressure (MAP), and HR were collected at baseline and then again at 1-minute intervals for 10 minutes after skin incision. Plasma ACTH concentrations were measured using the Roche electrical chemiluminescence immunoassay at baseline and 10 minutes after skin incision. The selection of the data collection timepoint was based on the study by Donald et al. (10), which demonstrated that in a conventional cholecystectomy, ACTH levels peak at 10 minutes after incision. Hemodynamic variables were also collected before the surgery. Other baseline patient characteristics, including age, body mass index (BMI), liver function, and renal function, were also collected.
Anesthesia protocol
A standard ASA monitor was fitted, and basic vital signs were recorded when the patient arrived in the induction room. All patients received a premedication intramuscular (IM) injection of midazolam (Enhua Pharmaceutical Co., Jiangsu, China) at 0.05 mg/kg. All patients underwent right internal jugular vein catheterization and left radial artery catheterization, and the first blood sample was collected.
General anesthesia was induced with 4 µg/mL propofol (B. Braun Melsungen AG, Berlin, Germany, with the Marsh model) and 4 ng/mL remifentanil (Renfu Pharmaceutical Co., Beijing, China) by computer-assisted target-controlled infusion (TCI; Braun Space) at the target plasma concentrations, and 0.6 mg/kg rocuronium (Xianju Pharmaceutical Co., Zhejiang, China) was administered intravenously when loss of consciousness (LOC) was achieved. When propofol effect-site concentration ≥2.3 mg/mL and remifentanil concentration ≥3 ng/mL, tracheal intubation was performed and patient were connected to a Drager Fabius anesthesia machine (Dragerwerk AG, Lubeck, Germany) for the operation.
After successful intubation, propofol infusion was terminated, and remifentanil infusion was continued at a plasma concentration 1 ng/mL. Anesthesia was maintained with sevoflurane (Drager AbbVie, MAC 1.0) and cisatracurium infusion at 1 mg/kg/min.
Patients who presented with the following were excluded from the final analysis. If the patient’s MAP was under 60 mmHg for more than 1 minute, 0.1 mg phenylephrine was used intravenously. If the MAP was over 130% baseline or the HR was over 140 bpm, then remifentanil plasma concentration was increased by 1 ng/mL. If the patient’s HR was under 45 bpm, 0.5 mg atropine was administered.
Sample size calculation
According to a previous study (11), the coefficient of correlation between the HPA-related hormone change and the SBP is 0.27. We aimed to achieve a power of 0.9 at an α level of 0.05. The following linear regression formula was used: N = 4{(µα + µβ)/ln[(1 + ρ)/(1 − ρ)]}2 + 3 (12) , with a 20% missing data consideration. The sample size of the study was calculated to be at least 168.
Model design
Dependent variable
The dependent variable considered in this study is the relative change in the levels of blood ACTH release before and during surgery, defined as the “ACTH change” = (ACTH 10 min after incision – ACTH at baseline)/ACTH at baseline. As there is no well-accepted ACTH threshold for specific surgical stress, “unacceptable ACTH change” was defined as patients whose changes are in the last quarter (>75%) of the whole ACTH change distribution. Therefore, patients were divided into 2 groups, where group 1 are patients with acceptable ACTH change (n=153) and group 2 are patients with unacceptable ACTH change (n=52).
Independent variable
The primary objective of this study was to investigate the association between BP changes, HR changes, and relative ACTH changes after balancing out other factors that could be associated with the outcome. The MAP, defined as 1/3 of systolic pressure and 2/3 of diastolic pressure, was used to study the association. The changes in MAP and HR were captured using three measures: 20% + increase indicator and 20% + decrease indicator, and maximum increase. The 20% + increase indicator was calculated to determine whether any MAP/HR measurements during surgery increased more than 20% compared to the baseline MAP/HR measurements. The 20% + decrease indicator was calculated to determine whether any MAP/HR measurements during surgery decreased more than 20% compared to the baseline measurements. The maximum increase was calculated as the difference between the highest MAP/HR during surgery and the baseline MAP/HR.
Control variables
Several control variables were included in the analysis, including age, sex, BMI, baseline ACTH and hemodynamic variables, for example, total bilirubin, hemoglobin. The summary statistics of all critical variables are provided in Table 1.
Table 1
Covariates | Mean (SD) or n (%) | P value | ||
---|---|---|---|---|
All (n=205) | Group 1 (n=153) | Group 2 (n=52) | ||
Preoperative baseline data | ||||
Age (years) | 54.6 (10.5) | 54.8 (10.0) | 53.9 (12.1) | 0.599 |
Gender: male | 146 (71.2) | 109 (71.2) | 37 (71.1) | 0.471 |
BMI (kg/m2) | 24.0 (3.2) | 23.8 (3.2) | 24.4 (3.1) | 0.222 |
Hypertension | 52 (25.4) | 41 (26.8) | 11 (21.2) | 0.419 |
Hemoglobin (g/L) | 138.5 (18.3) | 138.6 (19.3) | 138.1 (15.3) | 0.865 |
Platelet (×109/L) | 178.2 (74.9) | 174.8 (75.5) | 188.2 (73.1) | 0.267 |
Total bilirubin (μmol/L) | 15.5 (16.5) | 15.0 (15.5) | 17.0 (19.5) | 0.464 |
Direct bilirubin (μmol/L) | 6.1 (13.8) | 5.9 (13.0) | 6.5 (16.0) | 0.816 |
Albumin (g/L) | 43.9 (4.3) | 43.9 (4.4) | 44.0 (4.1) | 0.837 |
ALT (U/L) | 32.5 (54.4) | 34.2 (38.3) | 26.9 (16.7) | 0.181 |
AST (U/L) | 32.5 (54.4) | 34.6 (62.5) | 26.4 (11.0) | 0.349 |
Creatinine (μmol/L) | 72.5 (15.3) | 72.1 (14.9) | 73.7 (16.3) | 0.523 |
Baseline ACTH (pg/mL) | 27.3 (38.2) | 31.4 (43.3) | 15.1 (8.0) | 0.007* |
Baseline ACTH >30 pg/mL | 53 (25.9) | 50 (32.7) | 3 (5.8) | <0.0001* |
Intraoperative variables | ||||
MAP, maximum increase (mmHg) | 15.7 (19.2) | 14.7 (19.3) | 18.8 (18.9) | 0.190 |
MAP 20% + increase indicator | 71 (34.6) | 47 (30.7) | 24 (46.2) | 0.043* |
MAP 20% + decrease indicator | 109 (53.2) | 83 (54.2) | 26 (50.0) | 0.596 |
HR, maximum increase (bpm) | 4.4 (11.2) | 5.1 (11.1) | 2.4 (11.3) | 0.129 |
HR 20% + increase indicator | 39 (19.0) | 31 (20.3) | 8 (15.4) | 0.439 |
HR 20% + decrease indicator | 53 (25.9) | 35 (22.9) | 18 (34.6) | 0.095 |
Postoperative pathology result | ||||
Malignant tumor, n (%) | 181 (88.3) | 136 (88.9) | 45 (86.5) | 0.649 |
Type of cancer | – | |||
Primary liver cancer | 140 | 104 | 36 | |
Colorectal liver metastases | 34 | 27 | 7 | |
Others | 7 | 5 | 2 |
Group 1: patients with acceptable ACTH change; group 2: patients with unacceptable ACTH change. *, P<0.05. ACTH, adrenocorticotropic hormone; ALT, alanine aminotransferase; AST, aspartate transaminase; BMI, body mass index; HR, heart rate; MAP, mean arterial pressure; SD, standard deviation.
Statistical analysis
The model was built based on the bootstrapping approach with replacement, and 10,000 simulations were run to average the results. In each simulation, patients who were not sampled were treated as validation data. A logistic regression model was built with the train data (selected patients) and detailed receiver operating characteristic (ROC) analyses were performed on the validation data (unselected patients). The confusion matrix was generated to calculate the sensitivity and specificity as the probability cut-off of predicting 1 varies from 0 to 1. A final ROC curve was plotted and the area under the curve (AUC) was calculated. The optimal probability cut-off was extracted to maximize the product of sensitivity and specificity (13). Two criteria in model acceptance are proposed before analyses: AUC should be at least 0.700 and the correct prediction rate for abnormal ACTH patients should be at least 70%. Continuous baseline variables are expressed as the mean ± standard deviation (SD) and were analyzed with Student’s t-test. Categorical variables are expressed as category counts and percentages and were analyzed with a chi-square test. Statistical analyses are performed in R studio (version 1.2.1335) and SPSS 26.0 software (SPSS, Chicago, IL, United States). P values were computed based on two-sided t-tests, and a P value less than 0.05 was considered statistically significant.
Results
Sample characteristics
As shown in Figure 1, 280 consenting patients were screened and enrolled in the study. Of these, 75 patients were excluded, including 16 patients with incomplete data and 59 patients who experienced hemodynamic fluctuations that required medical intervention. After exclusions, a total of 205 patients were finally analyzed.
The 205 analyzed patients were stratified into 2 groups according to changes in their ACTH levels. Group 1 patients had acceptable levels of ACTH change (n=153) and group 2 patients presented with unacceptable ACTH changes (n=52).
As shown in Table 1 and Figure 2, the average age of patients in group 1 was 54.8±10.0 years, with an average BMI of 23.8±3.2 kg/m2, and 71.2% were males. Patients in group 2 were 53.9±12.1 years old, with an average BMI of 24.4±3.1 kg/m2, and 71.1% were males. There was no significant difference in liver and renal function between the two groups. Patients in group 2 had a significantly lower baseline ACTH compared to patients in group 1 (15.1 vs. 31.4 pg/mL). Only 5.8% of patients in group 2 had baseline ACTH levels greater 30 pg/mL, compared to 32.7% of patients in group 1. The maximum MAP increase was 14.7±19.3 and 18.8±18.9 mmHg for group 1 and 2, respectively. In group 2, 46.2% of patients showed an increase in MAP by over 20%, compared to 30.7% of group 1 patients. The maximum HR change for group 1 patients was 5.1±11.1 bpm, compared to a decreased of 2.4±11.3 bpm for group 2 patients. Around 22.9% of patients in group 1 experienced a decrease in HR by over 20% during surgery, compared to 34.6% of group 2 patients.
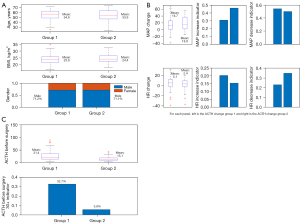
Variable selections
Demographic information including age, gender, BMI, and baseline BP, HR, and ACTH before surgery was collected for each patient. The patient’s vital statistics were monitored every minute and blood samples were collected to measure the ACTH levels during surgery. A total of 205 patients were studied in this research, 25% (n=52) were determined to have unacceptable ACTH changes (group 2).
Bivariate analysis was performed to study the association between the ACTH groups and each covariate to revise and introduce new variables in the model. Age and BMI were evenly distributed between the two groups, and were therefore unchanged. For baseline ACTH, due to outliers, this variable was categorized into two groups: >30 and <30 pg/mL. Changes in MAP and HR were calculated as the maximum increase between baseline values and values collected during surgery. In addition to raw changes, two other indicators were introduced, namely, the increase indicator which was defined as a maximum increase greater than 20% of the baseline value, and the decrease indicator defined as a maximum decrease greater than 20% of the baseline value. These indicators were included because in clinical practice, further medical interventions will be applied if a patient experiences such significant changes in their vital statistics.
Table 2 compares the logistic regression model with covariates in raw formats, the model with revised covariates, and the model with selected significant covariates. The BMI became insignificant when revised covariates were used. This is because the associations between BMI and ACTH group labels can be partially explained by the MAP and HR change indicators. The model performance metrics are included in Table 2. The reduction in deviance and the Akaike information criterion (AIC) drop was larger with revised covariates. The AUC from the ROC chart also increased in the model with revised covariates. The results remained similar when further trimmed, with only significant variables included (model 3). The reduction in deviance and the AUC was close to model 2, while the reduction in AIC was much larger than that in model 2.
Table 2
Covariates | Coefficients | P value |
---|---|---|
Model 1: raw format | ||
Age | −0.006 | 0.7133 |
Gender | 0.555 | 0.1871 |
BMI | 0.133 | 0.0341* |
Baseline ACTH | −0.111 | <0.001*** |
MAP change | −0.005 | 0.6344 |
HR change | −0.033 | 0.0741^ |
Model performance | ||
Reduction in AIC | 9.10 | |
Reduction in deviance | 31.10 | |
ROC area | 0.72 | |
Model 2: revised covariates | ||
Age | −0.016 | 0.364 |
Gender | 0.439 | 0.305 |
BMI | 0.096 | 0.109 |
Baseline ACTH 30+ indicator | −2.557 | <0.001*** |
MAP change | 0.002 | 0.921 |
MAP increase indicator | 1.467 | 0.084^ |
MAP decrease indicator | 1.128 | 0.120 |
HR change | −0.001 | 0.972 |
HR increase indicator | 0.112 | 0.853 |
HR decrease indicator | 1.422 | 0.011* |
Model performance | ||
Reduction in AIC | 14.60 | |
Reduction in deviance | 34.60 | |
ROC area | 0.75 | |
Model 3: selected covariates | ||
Baseline ACTH 30+ indicator | −2.294 | <0.001*** |
MAP increase indicator | 0.702 | 0.087^ |
HR decrease indicator | 1.321 | <0.01** |
Model performance | ||
Reduction in AIC | 21.54 | |
Reduction in deviance | 32.50 | |
ROC area | 0.73 |
*, P<0.05; **, P<0.01; ***, P<0.001; ^, P value close to significance. ACTH, adrenocorticotropic hormone; AIC, Akaike information criterion; BMI, body mass index; HR, heart rate; MAP, mean arterial pressure; ROC, receiver operating characteristic.
Bootstrapping
Instead of building a logistic regression model with full data, the bootstrapping approach was applied to this study. For each simulation, we applied a stratified sampling approach to select a sample from our population with replacement. In particular, our new sample consisted of 75% of patients from group 1 and 25% of patients in group 2. Due to the replacement, some patients could be selected more than once, and some may not be selected at all. After sampling, a logistic regression model was built based on this sample and the model details were extracted, including coefficients, standard errors, and the P values. Patients who were not sampled were treated as validation data to perform the ROC analyses.
Table 3 shows the average results of two logistic regression models: the model with all revised covariates included and the model with only significant covariates included. The coefficients were very close to the models built with full data, and again the baseline ACTH change >30 pg/mL indicator and the HR decrease indicator were significant. The MAP increase indicator was close to significance.
Table 3
Covariates | Coefficient | P value | 95% confidence interval |
---|---|---|---|
Model 2: revised covariates | |||
Age | −0.016 | 0.400 | −0.052, 0.021 |
Gender | 0.507 | 0.271 | −0.395, 1.409 |
BMI | 0.098 | 0.131 | −0.029, 0.225 |
Baseline ACTH >30 pg/mL indicator | −2.797 | <0.001*** | −4.361, −1.234 |
MAP change | 0.001 | 0.943 | −0.034, 0.037 |
MAP increase indicator | 1.622 | 0.085^ | −0.043, 3.287 |
MAP decrease indicator | 0.969 | 0.259 | −0.896, 2.834 |
HR change | −0.0003 | 0.991 | −0.055, 0.054 |
HR increase indicator | 0.090 | 0.890 | −1.182, 1.362 |
HR decrease indicator | 1.577 | <0.01* | 0.387, 2.767 |
Model 3: selected covariates | |||
Baseline ACTH >30 pg/mL indicator | −2.294 | <0.001*** | −3.633, −0.890 |
MAP increase indicator | 0.702 | 0.08^ | −0.082, 1.493 |
HR decrease indicator | 1.321 | <0.01** | 0.454, 2.210 |
*, P<0.05; **, P<0.01; ***, P<0.001; ^, P value close to significance. ACTH, adrenocorticotropic hormone; BMI, body mass index; MAP, mean arterial pressure; HR, heart rate.
Figure 3 compares the model performance metrics for all 10,000 simulations between model 2 (the model with all revised covariates) and model 3 (only significant covariates included). The AUC ranged from 0.663 to 0.902, with a mean of 0.778 (95% CI: 0.714–0.842): for model 2. For model 3, the AUC ranged from 0.614 to 0.824, with a mean of 0.724 (95% CI: 0.657–0.791). The predicted correct classification rate based on test data with model 2 for group 1 ranged from 42.6% to 75.5%, with a mean of 62.7%. For group 2, the range was from 53.1% to 94.3%, with a mean of 74.9%. The numbers were similar for model 3, however, with only three variables included in the model, there were more outliers, suggesting that there was instability for some samples. In this study, we decided to adopt model 3 even its AUC is much lower that of model 2 because first, a parsimony model is preferred for the purpose of prediction; second, the main goal is to identify patients with abnormal ACTH change, while model 2 has a better AUC value. However, the power of detecting abnormal patients is similar to that of model 3. The optimal probability cut-off of predicting abnormal ACTH patients is 0.712.
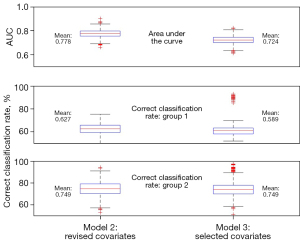
The relationship between MAP, HR, and relative ACTH change
Based on our regression models, baseline ACTH level >30 pg/mL was significantly negatively associated with large ACTH changes, indicating that the probability of patients with baseline ACTH level >30 pg/mL is, on average, only 11.1% as likely to experience large ACTH increases as patients with baseline ACTH level below 30 pg/mL. The MAP increase indicator was positively associated with large ACTH changes, suggesting that the probability of patients with at least 20% MAP increase during surgery experiencing very large ACTH increases is about 2.0 times greater than that of patients with no more than 20% MAP increase. The last significant variable is the HR 20% + decrease indicator which indicated that patients with a HR rate decrease greater 20% are less likely to experience a large ACTH increase compared to patients with a HR rate decrease less than 20% during surgery.
Numerical index to predict ACTH change group
Based on the regression models constructed herein, the following mathematical formula was derived: A = −1.39 − 2.29 × (baseline ACTH ≥30) + 0.70 × MAP 20% increase indicator + 1.32 × HR 20% decrease indicator.
The probability of experiencing abnormal .
Correct classification rate
Since patients in group 2 account for 25% of the sample, when the above-calculated probability is greater than 25%, the patient will be classified into the unacceptable ACTH change group. Table 4 shows the correct classification rate. Using the above numeric expression, we were able to detect 74.9% of patients with unacceptable ACTH change. However, 41.1% of patients in group 1 were misclassified, suggesting that our model overreacted to the ACTH changes. Therefore, we recommend using the numerical expression as the first pass of detecting large and unacceptable ACTH changes.
Table 4
Group | Classified acceptable ACTH change group | Classified unacceptable ACTH change group |
---|---|---|
Group 1 | 90 (58.8%) | 63 |
Group 2 | 13 | 39 (75.0%) |
Group 1: patients with acceptable ACTH change; group 2: patients with unacceptable ACTH change. ACTH, adrenocorticotropic hormone.
Discussion
This present study investigated the correlation between basic physiological parameters (MAP and HR), that are routinely monitored during anesthesia, and the stress hormone ACTH during general anesthesia under noxious stimuli of skin incision. A logistic regression model was set up for intraoperative stress levels under certain stimuli and demonstrated specific correlations of hemodynamic changes with ACTH fluctuations. The model built may have potential in predicting an unacceptable ACTH change with high sensitivity and specificity.
Prolonged surgical stress may lead to sympathetic hyperfunction, leading to tachycardia, hypertension, myocardial ischemia, arrhythmia, and other pathological changes and risks (14), resulting in higher morbidity and delayed recovery after surgery (15). Stress-reducing anesthesia improves postoperative recovery [enhanced recovery after surgery (ERAS)] through measured control of analgesia and hypnosis. The fundamental triggers of the rapid stress response are sympathetic activation and increased adrenal production of stress hormones in the body (16). Different methods or indices derived from sympathetic tone (17) provide conflicting information and can result in ACTH, cortisol, and catecholamines fluctuations. Circulating catecholamines are unlikely to be perfect indicators of intraoperative stress levels (18), as Furuya et al. found that plasma epinephrine and norepinephrine concentrations maintained within the normal range when ACTH and cortisol levels increased after incision (19). ACTH and cortisol have been shown to be correlated with the levels of stimulation during surgical stress. At the same time, they showed variations with different levels of analgesia (5,18,20). In this study, 10 minutes after skin incision was taken as the main timepoint to measure stress hormone levels. The patient’s maximum hemodynamic changes during these 10 minutes were also obtained. Considering that cortisol is secondary to a preceding increase in ACTH secretion, ACTH was selected as the stress indicator. In addition, due to patient-to-patient differences in serum ACTH, the change in ACTH levels was used as an objective index of stress.
Since no previous studies have indicated an upper threshold value for either ACTH nor its fluctuations, we artificially stratified patients into two groups with acceptable ACTH change (0–75%) and unacceptable ACTH change (>75%). Bivariate logistic regression was used to analyze the significant variables that correlate with ACTH changes. The results identified two significant variables, namely, baseline ACTH and HR 20% + change. Another variable, MAP 20% + change achieved close to significance. Based on the regression model, it is evident that baseline ACTH level is an essential variable and showed a negative association with dramatic ACTH change. In addition, patients who experienced MAP increase greater than 20% were more likely to experience dramatic ACTH changes. In contrast, patients whose HR decreased more than 20% were less likely to experience a dramatic ACTH change. The prediction model had an AUC of 0.723, and sensitivity and specificity of 75.0% and 58.8%, respectively. The positive and negative predictive values for unacceptable ACTH change were 38.2% and 87.4%, respectively. Therefore, the model showed a moderate performance in predicting ACTH changes, suggesting that intraoperative stress levels may be predicted based on physiological parameters from routine patient monitoring.
In a previous study (21), researchers examined the correlation between SPI and stress hormones in 80 patients undergoing propofol-remifentanil anesthesia. The results showed that SPI reflected a “specific level” of ACTH (defined as the average of the data at baseline) with an AUC of 0.85, an optimal threshold value of SPI ≥46 to predict an ACTH of ≥15 pg/mL with a sensitivity of 81% and a specificity of 73%. However, their result was based on a small sample size. Also, SPI is an assessment based on HR variability. To date, there has been little evidence to link heart rate variability (HRV)-based monitors with intraoperative stress hormone levels (20), and SPI itself can be affected by artefacts such as vasoconstriction, hypovolemia, and hypothermia (1,22).
Traditionally, general anesthetic and analgesic administration are often determined by experienced anesthesiologists based on hemodynamic parameters and different surgical stimuli (4). Nevertheless, these are imperfect surrogate markers and are not accurate for anesthetic management (23). Anesthetic administration guided by clinical signs has been shown to be insensitive and unspecific, as they are easily affected by confounders and may differ individually. Unlike other components of anesthesia, such as hypnosis and neuromuscular relaxation, there is currently no gold standard for assessing nociceptive-antinociceptive balance (NAN) (1). Various monitoring devices have been developed to evaluate the NAN balance in anesthetized patients using sympathetic or parasympathetic nervous system measurements, such as ANI (24,25), NOL index (26), SPI, PPI (25,27), the CARdiovascular Depth of Analgesia (CARDEAN) index (28), the number of fluctuations in skin conductance (NFSC), etc. These indices have been applied in many clinical studies and compared to HR or MAP in detecting painful stimulations and analgesic administrations (3).
However, none of the above-mentioned indices have been widely utilized due to their deficiencies and inadequate data demonstrating their clinical significance. To verify their effect on opioid administration and stress control, numerous randomized clinical trials have been designed to evaluate the NAN balance by comparing it to a group guided by clinical signs or the experience of anesthetists. These studies showed mixed and conflicting results in both opioid consumption and perioperative adverse events. Most of these studies revealed no significant effect of nociception monitors on opioid use nor postoperative recovery (5,6,29-31). In a recent study, Ledowski et al. examined the associations between SPI, NFSC, and stress hormones in a small sample of 20 patients. Their results showed poor and unreliable correlations between two NAN monitors and stress hormone levels (noradrenaline, adrenaline, ACTH, and cortisol), suggesting that neither SPI nor the NFSC is a good predictor of changes in stress hormone levels with satisfactory accuracy (32). In another study, Funcke et al. compared SPI, PPI, and NOL with a control group. Opioids consumption in patients receiving SPI monitor was higher than that in the control group and showed a minor increase in ACTH and cortisol. However, in the PPI and NOL groups, where fewer opioids were used, more intraoperative movements occurred, and patients showed higher stress hormone levels (6). Due to these contradictory results in nociception-monitoring devices with opioid administration, stress hormone levels, and intraoperative unwanted incidents, more clinical studies should focus on the relationship between the patient’s intraoperative stress hormone levels and NAN balances.
There were some limitations to this study. First, the prediction model was based on a critical variable, that is, baseline ACTH. The patient’s baseline ACTH levels must be measured before surgery. Second, the study subjects were mainly male patients, as only patients undergoing hepatectomy were selected to avoid surgery-related confounders. Most of the patients in the study presented with hepatocellular carcinoma (HCC), which occurs mainly in men (with a 4:1 male to female ratio) (33). Therefore, this study may not be representative of the whole population, and there may be certain limitations to the model. Third, there may be other unmeasured and confounding variables that were overlooked which may influence fluctuations in ACTH levels and hemodynamic parameters. Moreover, the prediction model was validated using an internal sample, which may bring down the accuracy of the model. Well-designed clinical trials should be designed to provide external validation to validate the actual predictive accuracy.
Conclusions
This research is the preliminary step in modeling intraoperative surgical stress based on simple physiological parameters. Further carefully designed, validation studies are still warranted, targeting different patient groups, different types of surgical stress, as well as varying anesthetic strategies.
Acknowledgments
The authors would like to thank Yuguang Gao and Qiang Yang for their helpful discussions on the statistical analyses of ACTH responses and hemodynamic parameters.
Funding: This research was supported by the Shanghai Municipal Key Clinical Specialty grant (Grant No. shslczdzk03603) and Zhongshan Smart Healthcare Project (Grant No. 2020ZHZS23).
Footnote
Reporting Checklist: The authors have completed the TRIPOD reporting checklist. Available at https://atm.amegroups.com/article/view/10.21037/atm-22-2276/rc
Data Sharing Statement: Available at https://atm.amegroups.com/article/view/10.21037/atm-22-2276/dss
Conflicts of Interest: All authors have completed the ICMJE uniform disclosure form (available at https://atm.amegroups.com/article/view/10.21037/atm-22-2276/coif). All authors report this research was supported by the Shanghai Municipal Key Clinical Specialty grant (Grant No. shslczdzk03603) and the Zhongshan Smart Healthcare Project (Grant No. 2020ZHZS23). The authors have no other conflicts of interest to declare.
Ethical Statement: The authors are accountable for all aspects of the work in ensuring that questions related to the accuracy or integrity of any part of the work are appropriately investigated and resolved. The study was conducted in accordance with the Declaration of Helsinki (as revised in 2013). The study was approved by the institutional ethics committee board of Zhongshan Hospital (No. B2018-288R). Informed consent was taken from all the patients.
Open Access Statement: This is an Open Access article distributed in accordance with the Creative Commons Attribution-NonCommercial-NoDerivs 4.0 International License (CC BY-NC-ND 4.0), which permits the non-commercial replication and distribution of the article with the strict proviso that no changes or edits are made and the original work is properly cited (including links to both the formal publication through the relevant DOI and the license). See: https://creativecommons.org/licenses/by-nc-nd/4.0/.
References
- De Jonckheere J, Bonhomme V, Jeanne M, et al. Physiological Signal Processing for Individualized Anti-nociception Management During General Anesthesia: a Review. Yearb Med Inform 2015;10:95-101. [PubMed]
- Rogobete AF, Bedreag OH, Papurica M, et al. Multiparametric Monitoring of Hypnosis and Nociception-Antinociception Balance during General Anesthesia-A New Era in Patient Safety Standards and Healthcare Management. Medicina (Kaunas) 2021;57:132. [Crossref] [PubMed]
- Funcke S, Sauerlaender S, Pinnschmidt HO, et al. Validation of Innovative Techniques for Monitoring Nociception during General Anesthesia: A Clinical Study Using Tetanic and Intracutaneous Electrical Stimulation. Anesthesiology 2017;127:272-83. [Crossref] [PubMed]
- Anderson TA. Intraoperative Analgesia-Nociception Monitors: Where We Are and Where We Want To Be. Anesth Analg 2020;130:1261-3. [Crossref] [PubMed]
- Funcke S, Pinnschmidt HO, Wesseler S, et al. Guiding Opioid Administration by 3 Different Analgesia Nociception Monitoring Indices During General Anesthesia Alters Intraoperative Sufentanil Consumption and Stress Hormone Release: A Randomized Controlled Pilot Study. Anesth Analg 2020;130:1264-73. [Crossref] [PubMed]
- Funcke S, Pinnschmidt HO, Brinkmann C, et al. Nociception level-guided opioid administration in radical retropubic prostatectomy: a randomised controlled trial. Br J Anaesth 2021;126:516-24. [Crossref] [PubMed]
- Lucassen PJ, Pruessner J, Sousa N, et al. Neuropathology of stress. Acta Neuropathol 2014;127:109-35. [Crossref] [PubMed]
- Besnier E, Clavier T, Compere V. The Hypothalamic-Pituitary-Adrenal Axis and Anesthetics: A Review. Anesth Analg 2017;124:1181-9. [Crossref] [PubMed]
- Matovic E, Delibegovic S. Adrenocorticotropic Hormone (ACTH) and Cortisol Monitoring as Stress Markers During Laparoscopic Cholecystectomy: Standard and Low Intraabdominal Pressure and Open Cholecystectomy. Med Arch 2019;73:257-61. [Crossref] [PubMed]
- Donald RA, Perry EG, Wittert GA, et al. The plasma ACTH, AVP, CRH and catecholamine responses to conventional and laparoscopic cholecystectomy. Clin Endocrinol (Oxf) 1993;38:609-15. [Crossref] [PubMed]
- Ewart CK, Elder GJ, Jorgensen RS, et al. The Role of Agonistic Striving in the Association Between Cortisol and High Blood Pressure. Psychosom Med 2017;79:416-25. [Crossref] [PubMed]
- Graybill FA. An Introduction to Linear Statistical Models. New York (State): McGraw-Hill; 1961.
- Liu X. Classification accuracy and cut point selection. Stat Med 2012;31:2676-86. [Crossref] [PubMed]
- Pan YS, Hu YF, Tian FB, et al. Effects of epidural preemptive analgesia on stress reaction in retroperitoneal laparoscopic adrenalectomy surgery: a randomized controlled study. Int J Clin Exp Med 2015;8:9862-8. [PubMed]
- Gibbison B, Spiga F, Walker JJ, et al. Dynamic pituitary-adrenal interactions in response to cardiac surgery. Crit Care Med 2015;43:791-800. [Crossref] [PubMed]
- Kohl BA, Deutschman CS. The inflammatory response to surgery and trauma. Curr Opin Crit Care 2006;12:325-32. [Crossref] [PubMed]
- Defresne A, Harrison M, Clement F, et al. Two different methods to assess sympathetic tone during general anesthesia lead to different findings. J Clin Monit Comput 2019;33:463-9. [Crossref] [PubMed]
- Traynor C, Hall GM. Endocrine and metabolic changes during surgery: anaesthetic implications. Br J Anaesth 1981;53:153-60. [Crossref] [PubMed]
- Furuya K, Shimizu R, Hirabayashi Y, et al. Stress hormone responses to major intra-abdominal surgery during and immediately after sevoflurane-nitrous oxide anaesthesia in elderly patients. Can J Anaesth 1993;40:435-9. [Crossref] [PubMed]
- Ledowski T, Bein B, Hanss R, et al. Neuroendocrine stress response and heart rate variability: a comparison of total intravenous versus balanced anesthesia. Anesth Analg 2005;101:1700-5. [Crossref] [PubMed]
- Chen X, Thee C, Gruenewald M, et al. Correlation of surgical pleth index with stress hormones during propofol-remifentanil anaesthesia. ScientificWorldJournal 2012;2012:879158. [Crossref] [PubMed]
- Huiku M, Uutela K, van Gils M, et al. Assessment of surgical stress during general anaesthesia. Br J Anaesth 2007;98:447-55. [Crossref] [PubMed]
- Gélinas C, Arbour C. Behavioral and physiologic indicators during a nociceptive procedure in conscious and unconscious mechanically ventilated adults: similar or different? J Crit Care 2009;24:628.e7-17. [Crossref] [PubMed]
- Ledowski T, Averhoff L, Tiong WS, et al. Analgesia Nociception Index (ANI) to predict intraoperative haemodynamic changes: results of a pilot investigation. Acta Anaesthesiol Scand 2014;58:74-9. [Crossref] [PubMed]
- Cowen R, Stasiowska MK, Laycock H, et al. Assessing pain objectively: the use of physiological markers. Anaesthesia 2015;70:828-47. [Crossref] [PubMed]
- Ben-Israel N, Kliger M, Zuckerman G, et al. Monitoring the nociception level: a multi-parameter approach. J Clin Monit Comput 2013;27:659-68. [Crossref] [PubMed]
- Chapman CR, Oka S, Bradshaw DH, et al. Phasic pupil dilation response to noxious stimulation in normal volunteers: relationship to brain evoked potentials and pain report. Psychophysiology 1999;36:44-52. [Crossref] [PubMed]
- Martinez JY, Wey PF, Lions C, et al. A beat-by-beat cardiovascular index, CARDEAN: a prospective randomized assessment of its utility for the reduction of movement during colonoscopy. Anesth Analg 2010;110:765-72. [Crossref] [PubMed]
- Gruenewald M, Willms S, Broch O, et al. Sufentanil administration guided by surgical pleth index vs standard practice during sevoflurane anaesthesia: a randomized controlled pilot study. Br J Anaesth 2014;112:898-905. [Crossref] [PubMed]
- Jain N, Gera A, Sharma B, et al. Comparison of Surgical Pleth Index-guided analgesia using fentanyl versus conventional analgesia technique in laparoscopic cholecystectomy. Minerva Anestesiol 2019;85:358-65. [Crossref] [PubMed]
- Colombo R, Raimondi F, Rech R, et al. Surgical Pleth Index guided analgesia blunts the intraoperative sympathetic response to laparoscopic cholecystectomy. Minerva Anestesiol 2015;81:837-45. [PubMed]
- Ledowski T, Pascoe E, Ang B, et al. Monitoring of intra-operative nociception: skin conductance and surgical stress index versus stress hormone plasma levels. Anaesthesia 2010;65:1001-6. [Crossref] [PubMed]
- Zhang X, El-Serag HB, Thrift AP. Sex and Race Disparities in the Incidence of Hepatocellular Carcinoma in the United States Examined through Age-Period-Cohort Analysis. Cancer Epidemiol Biomarkers Prev 2020;29:88-94. [Crossref] [PubMed]
(English Language Editor: J. Teoh)