Novel prognostic nomograms to assess survival in high-grade serous ovarian carcinoma after surgery and chemotherapy: a retrospective cohort study from SEER database
Introduction
In 2018, over 295,000 women were diagnosed with ovarian cancer and nearly 185,000 died from the disease worldwide (1). For patients and their families, decisions about active medical treatment versus palliative care inherently depend on survival expectations (2). Yet, accurate prediction of the prognosis of actively-treated patients remain a significant challenge for gynecologic oncologists.
It is well known that ovarian carcinoma consists of a heterogeneous group of neoplasms with multiple histologic subtypes, including epithelial and non-epithelial ovarian cancers (3,4). High-grade serous ovarian carcinoma (HGSOC) is the most common histological type with distinct biological features compared to other ovarian carcinomas, accounting for approximately 70–80% of all malignant ovarian neoplasms (5,6). Most HGSOC patients are diagnosed at stage III/IV (advanced stage) with a poor overall prognosis (7). Comprehensive staging surgery, primary cytoreductive surgery, or neoadjuvant chemotherapy combined with interval debulking surgery followed by adjuvant chemotherapy is the treatment used for most patients (8).
There are already some prognostic models for epithelial ovarian cancer that have been tested and externally validated; however, most are not specific to histologic subtypes (9). Although the International Federation of Obstetrics and Gynecology (FIGO) staging system has been incorporated in the existing prognostic models for HGSOC, some demographic and socioeconomic factors, such as younger age, have not been considered (10,11). Despite their previous omission, these easily assessed factors profiling the distinct specificities of functional status and social support may be prognostic indicators. Currently used prognostic models for HGSOC mainly focus on biomarkers (12-15), or clinical features with radiology (16,17). They reported the range of models’ c-index of about 0.6–0.7. However, the associated expenses restrict access to these prognostic tools for decision making. And clinical data are still the major source of information regarding prognosis in large part because many biological factors have not yet been validated or are not readily measurable. Prediction nomograms based on easily accessible clinical information for patients with HGSOC need to be further studied.
Integration of the pivotal factors into a model to build a nomogram requires a statistic-based tool to evaluate the prognosis and quantify the risks in many cancer types (18-20). Accurate prognostic prediction is important for providing individualized therapy for patients. Therefore, we aimed to use the Surveillance, Epidemiology, and End Results (SEER) database to establish a novel, easy-to-use, and accurate prognostic nomogram for HGSOC patients. We present the following article in accordance with the TRIPOD reporting checklist (available at https://atm.amegroups.com/article/view/10.21037/atm-21-4383/rc).
Methods
Participants
This study is a retrospective cohort study. For the development model, samples used for this study were obtained from the SEER database, encompassing approximately 35% of the total population in the United States (21). The study was conducted in accordance with the Declaration of Helsinki (as revised in 2013). No personally identifying information was used in the study, which eliminated the requirement for Institutional Review Board approval or informed patient consent.
Clinical data extraction and measurement
Patients diagnosed with ovarian serous cancer between 2010 to 2016 were selected using the International Classification of Diseases for Oncology, third edition (ICD-O-3) primary site code of C56.9 (ovary) and morphology codes of 8441/3, 8442/3, 8460/3, and 8461/3. Patients with a diagnosis not confirmed by histology, ovarian serous carcinoma not as the primary tumor, survival time shorter than 1 month or unknown, and unknown staging information [according to the American Joint Committee on Cancer (AJCC), 7th edition], marital status, race, tumor grade, tumor laterality, tumor size, lymph node (LN), residual disease, or organ metastasis were excluded from the study cohort. Cases with poorly differentiated or undifferentiated carcinoma were classified as HGSOC. The detailed flow chart for patient inclusion and exclusion is shown in Figure 1. The study outcomes included overall survival (OS) and cancer-specific survival (CSS). The survival time was measured in months. OS was calculated from diagnosis to death of all causes or to date of last follow-up in November 2016. CSS was calculated from diagnosis to death of cancer-specific cause or to date of last follow-up in November 2016.
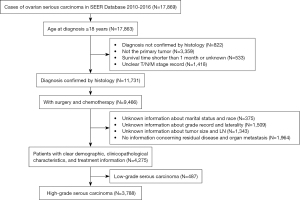
Marital status was classified into three types: currently married, never married, and separated/ divorced/widowed (22). The FIGO stage was re-evaluated based on information from the database, which included the tumor-node-metastasis (TNM) stage (according to the AJCC, 6th and 7th editions), tumor size, LN status and distant metastasis, tumor size (cm), tumor laterality (unilateral versus bilateral), performance of regional lymphadenectomy [no, adequate, and inadequate; ≥10 excised LNs was defined as adequate lymphadenectomy, according to the Gynecologic Oncology Group (GOG) criteria (23)]. The tumor size was divided into the following five categories, based on maximum diameter: <2, ≥2 and <4, ≥4 and <10, ≥10 and <16, and ≥16 cm. The type of resection performed was classified as: R0 no residual disease, R1 microscopic residual disease (0–1 cm), and R2 macroscopic residual disease (>1 cm). The different age categories at diagnosis were specified as: <50, ≥50 and <60, ≥60 and <70, ≥70 and <80, and ≥80 years.
Variable screening and nomogram model building
All cases in this study were randomly dichotomized into two groups for training and testing at a ratio of 7:3. The primary endpoints were OS and CSS. The comparison of clinicopathological characteristics between the two cohorts was performed using the t-test or chi-square test. Firstly, the most valuable predictive features of HGSOC among 11 parameters were primarily selected via the least absolute shrinkage and selection operator (LASSO) method. The partial likelihood deviance (binomial deviance) curve was plotted versus log (lambda). The two vertical dashed lines represented the minimum value and one standard deviation from the minimum value. The one standard deviation from the minimum value of lambda values, 0.030 and 0.036, were chosen for OS and CSS, respectively. Then, the nomograms were built on the final risk factors identified in the multivariate analysis from the Cox proportional hazards model in the training cohort.
Assessment of nomogram performance and clinical use
The discriminative performance of the nomograms was evaluated using Harrell’s concordance index (C-index). The maximum value of the Harrell’s C-index, 1, indicates a perfectly discriminating model while a value of 0.5 indicates that discrimination is not better than chance (24). Calibration curves (1,000 bootstrap resamples) were generated to assess the consistency between the actual and predicted survival. Decision curve analysis (DCA) was applied to evaluate the clinical latent value of the nomograms (25). Risk stratification of HGSOC patients were evaluated. Firstly, the median risk score from the training cohort was deemed as the threshold for OS and CSS respectively. Secondly, we used the thresholds to distinguish high risk and low risk in both training cohort and test cohort.
Statistical analysis
All statistical analyses were performed using R software (version 4.0.4; http://www.r-project.org/) and SPSS (version 24.0; SPSS, Chicago, IL, USA). Categorical variables were shown as numbers and proportions. Kaplan-Meier analysis and log-rank test were used to explore the survival difference between subgroups. Scaled Schoenfeld residuals was used to check the proportional hazards assumption. There are two endpoints including OS and CSS in our study, and we did not perform multiple test correction. A two-sided P value of <0.05 was considered statistically significant.
Results
Clinicopathological characteristics and survival
From the SEER database, a total of 3,788 individuals diagnosed with primary HGSOC were identified and included in the final analysis. Most of the cases (83.2%) had reached an advanced stage of cancer (stages III and IV) at their initial diagnosis, and more than half of the patients (53.9%) were older than 59 years. A total of 2,329 (61.5%) cases underwent LN dissection and 2,500 cases (66.0%) received R0 resection. The comparison of clinicopathological characteristics in the training cohort (n=2,591) and test cohort (n=1,197) is provided in Table 1. For all cases, the 3-year OS and CSS rates were 68.5%, and 69.5%, respectively. The 5-year OS and CSS rates were 49.0% and 50.5 %, respectively.
Table 1
Variables | Training set, n (%) | Testing set, n (%) | All patients, n (%) | P |
---|---|---|---|---|
Total | 2,591 (70.0) | 1,197 (30.0) | 3,788 (100.0) | |
Marital status | 0.810 | |||
un-SDW | 2,007 (77.5) | 923 (77.1) | 2,930 (77.3) | |
SDW | 584 (22.5) | 274 (22.9) | 858 (22.3) | |
Race | 0.482 | |||
White | 2,201 (84.9) | 999 (83.5) | 3,200 (84.5) | |
Black | 159 (6.1) | 83 (6.9) | 242 (6.4) | |
Other | 231 (8.9) | 115 (9.6) | 346 (9.1) | |
Age, years | 1.000 | |||
<50 | 407 (15.7) | 189 (15.8) | 596 (15.7) | |
50–59 | 787 (30.4) | 363 (30.3) | 1,150 (30.4) | |
60–69 | 825 (31.8) | 382 (31.9) | 1,207 (31.9) | |
70–79 | 461 (17.8) | 211 (17.6) | 672 (17.7) | |
≥80 | 111 (4.3) | 52 (4.3) | 163 (4.3) | |
Grade | 0.537 | |||
Grade III | 1,247 (48.1) | 589 (49.2) | 1,836 (48.5) | |
Grade IV | 1,344 (51.9) | 608 (50.8) | 1,952 (51.5) | |
Laterality | 0.479 | |||
Unilateral | 1,064 (41.1) | 477 (39.8) | 1,541 (40.7) | |
Bilateral | 1,527 (58.9) | 720 (60.2) | 2,247 (59.3) | |
Radiation | 0.115 | |||
No/unknown | 2,562 (98.9) | 1,190 (99.4) | 3,752 (99.9) | |
Yes | 29 (1.1) | 7 (0.6) | 36 (1.0) | |
LN dissected | 0.996 | |||
No or examined | 997 (38.5) | 462 (38.6) | 1,459 (38.5) | |
1–10 | 770 (29.7) | 354 (29.6) | 1,124 (29.7) | |
≥11 | 824 (31.8) | 381 (31.8) | 1,205 (31.8) | |
Organ metastasis | 0.453 | |||
No | 2,371 (91.5) | 1,104 (92.2) | 3,475 (91.7) | |
Yes | 220 (8.5) | 93 (7.8) | 313 (8.3) | |
Tumor size, cm | 0.924 | |||
<2 | 181 (7.0) | 84 (7.0) | 265 (7.0) | |
≥2 and <4 | 291 (11.2) | 143 (11.9) | 434 (11.5) | |
≥4 and <10 | 1,110 (42.8) | 508 (42.4) | 1,618 (42.7) | |
≥10 and <16 | 725 (28.0) | 340 (28.4) | 1,065 (28.1) | |
≥16 | 284 (11.0) | 122 (10.2) | 406 (10.7) | |
Residual disease | 0.087 | |||
R0 | 1,739 (67.1) | 761 (63.6) | 2,500 (66.0) | |
R1 | 566 (21.8) | 283 (23.6) | 849 (22.4) | |
R2 | 286 (11.0) | 153 (12.8) | 439 (11.6) | |
FIGO stage | 0.706 | |||
I | 184 (7.1) | 94 (7.9) | 278 (7.3) | |
II | 252 (9.7) | 107 (8.9) | 359 (9.5) | |
III | 1,601 (61.8) | 748 (62.5) | 2,349 (62.0) | |
IV | 554 (21.4) | 248 (20.7) | 802 (21.2) | |
T stage | 0.698 | |||
T1 | 227 (8.8) | 113 (9.4) | 340 (9.0) | |
T2 | 367 (14.2) | 161 (13.5) | 528 (13.9) | |
T3 | 1,997 (77.1) | 923 (77.1) | 2,920 (77.1) | |
N stage | 0.907 | |||
N0 | 1,692 (65.3) | 784 (65.5) | 2,476 (65.4) | |
N1 | 899 (34.7) | 413 (34.5) | 1,312 (34.6) | |
M stage | 0.642 | |||
M0 | 2,037 (78.6) | 949 (79.3) | 2,986 (78.8) | |
M1 | 554 (21.4) | 248 (20.7) | 802 (21.2) |
HGSOC, high-grade serous ovarian carcinoma; SDW, separated, divorced, and widowed; LN, lymph node; FIGO, International Federation of Gynecology and Obstetrics.
Identification of prognostic variables and construction of the nomograms
A total of 11 variables were included in the LASSO Cox regression analysis. We found that marital status, age, LN dissection, tumor size, residual disease, and FIGO stage were risk factors for OS and age, and LN dissection, tumor size, residual disease, and FIGO stage were risk factors for CSS, respectively (Figure 2). On multivariate Cox regression analysis, the factors independently associated with OS were marital status, age, LN dissection, tumor size, residual disease, and FIGO stage, whereas marital status was not significantly associated with CSS (Table 2). The nomograms were constructed to predict 3- and 5-year OS and CSS by combining the above prognostic factors (Figure 3).
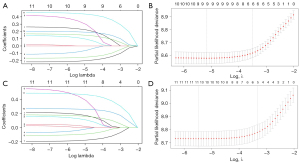
Table 2
Variables | OS | CSS | |||
---|---|---|---|---|---|
HR (95% CI) | P | HR (95% CI) | P | ||
Marital status | |||||
un-SDW | Reference | ||||
SDW | 1.313 (1.120–1.541) | 0.001 | |||
Age, years | |||||
<50 | Reference | Reference | |||
50–59 | 1.110 (0.879–1.402) | 0.382 | 1.422 (1.109–1.823) | 0.006 | |
60–69 | 1.418 (1.128–1.782) | 0.003 | 1.683 (1.321–2.144) | <0.001 | |
70–79 | 1.522 (1.188–1.949) | 0.001 | 1.830 (1.410–2.376) | <0.001 | |
≥80 | 1.914 (1.333–2.747) | <0.001 | 2.506 (1.702–3.691) | <0.001 | |
LN dissected | |||||
No or examined | Reference | Reference | |||
1–10 | 0.895 (0.761–1.052) | 0.178 | 0.877 (0.740–1.038) | 0.126 | |
≥11 | 0.647 (0.538–0.777) | <0.001 | 0.677 (0560–0818) | <0.001 | |
Tumor size, cm | |||||
<2 | Reference | Reference | |||
≥2 and <4 | 0.795 (0.594–1.063) | 0.021 | 0.853 (0615–1.182) | 0.339 | |
≥4 and <10 | 0.710 (0.554–0.908) | 0.006 | 0.835 (0.631–1.106) | 0.209 | |
≥10 and <16 | 0.555 (0.425–0.726) | <0.001 | 0.671 (0.498–0.904) | 0.009 | |
≥16 | 0.523 (0.376–0.726) | <0.001 | 0.697 (0.489–0.995) | 0.047 | |
Residual | |||||
R0 | Reference | Reference | |||
R1 | 1.422 (1.207–1.676) | <0.001 | 1.602 (1.351–1.899) | <0.001 | |
R2 | 1.409 (10155–1.719) | 0.001 | 1.601 (1.301–1.970) | <0.001 | |
FIGO stage | |||||
Stage I | Reference | Reference | |||
Stage II | 1.936 (1.047–3.579) | 0.035 | 1.820 (0.934–3.547) | 0.078 | |
Stage III | 4.371 (2.604–7.339) | <0.001 | 4.413 (2.529–7.701) | <0.001 | |
Stage IV | 5.900 (3.476–10.014) | <0.001 | 6.281 (3.556–11.094) | <0.001 |
OS, overall survival; CSS, cancer-special survival; HR, hazard ratio; CI, confidence interval; SDW, separated, divorced, and widowed; LN, lymph node; R0, no residual disease; R1, microscopic residual disease (0–1 cm); R2, macroscopic residual disease (>1 cm); FIGO, International Federation of Gynecology and Obstetrics; OS, overall survival; CSS, cancer-special survival.
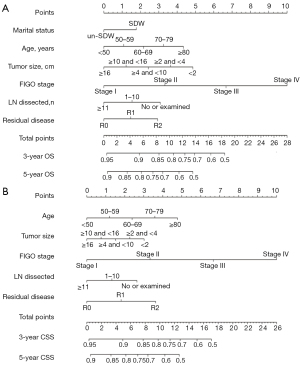
Testing of this novel nomogram and comparison with current models
The C-indices for the nomograms in the training cohort were 0.679 [95% confidence interval (CI): 0.660 to 0.699] and 0.678 (95% CI: 0.658 to 0.698) for OS and CSS, respectively, both of which were higher than that of the commonly accepted FIGO staging system [OS: 0.609 (95% CI: 0.591 to 0.627); CSS: 0.612 (95% CI: 0.593 to 0.630)] and the AJCC staging system [OS: 0.615 (95% CI: 0.595 to 0.636); CSS: 0.619 (95% CI: 0.598 to 0.640)]. The C-indices for the new models of OS and CSS [0.662 (95% CI: 0.633 to 0.690) and 0.680 (95% CI: 0.653 to 0.707), respectively] were also well presented in the test cohort compared with the FIGO [OS: 0.599 (95% CI: 0.573 to 0.624); CSS: 0.605 (95% CI: 0.579 to 0.630)] and AJCC [OS: 0.605 (95% CI: 0.576 to 0.634); CSS: 0.613 (95% CI: 0.585 to 0.642)] staging systems. In comparison with models including only the FIGO or the AJCC staging system, C-statistics using the nomogram were increased by 4.5–7.0% for the development test and by 6.7–7.9% for the validation test. The calibration curves showed sufficient agreement between the predicted and actual observed survival in both the training and test cohorts (Figure 4). The DCA curves indicated that our novel nomogram models achieved more accurate clinical prognosis predictions compared to survival predicted by the AJCC staging system and the FIGO staging system (Figure 5).
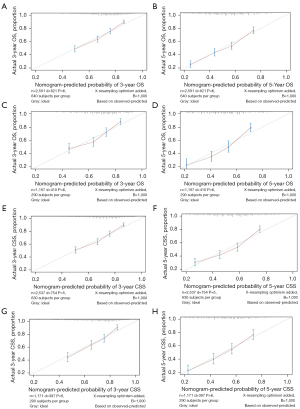
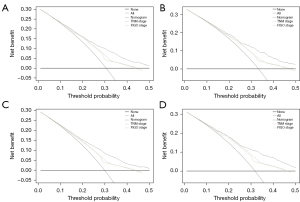
Risk stratification of HGSOC patients
The risk score of each feature was assigned by the nomogram and a total score was calculated for each individual case. The median risk score was 15 (range: 2 to 25) and 12 (range: 0 to 23) for OS and CSS in the training cohort, respectively. Based on the above scores, cases were divided into low- and high-risk groups, with significant different prognoses for both OS (P<0.001) and CSS (P<0.001) in the training and test cohorts (Figure 6), showing the nomogram’s good ability for prognostic and risk stratification.
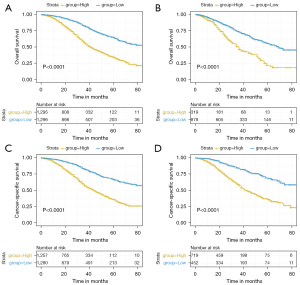
Discussion
Current European guidelines published in 2019 recommend BRCA genetic testing for all patients with non-mucinous ovarian cancer. In addition, other genes in homologous recombination pathways have been recognized, and biomarkers are now popular in ovarian cancer diagnosis and treatment. However, their real effect on the assessment of epithelial ovarian cancer risk is still uncertain (26) and due to their expense, not everybody has access to or can acquire these prognostic tools for decision making. For patients with HGSOC, there is still a lack of widely available, cost-effective methods for predicting survival after surgery and chemotherapy. In our study, we identified prognostic risk factors from clinicopathological, demographic, and socioeconomic factors. Then, we developed novel nomograms using these easily accessible factors, with the aim of assessing the 3- and 5-year OS and CSS. The bigger range of threshold probabilities in DCA curves, higher C-index, and consistent calibration curve observed in both the training and test sets indicated better performances of the models compared with the FIGO staging system and the AJCC staging system. Additionally, the nomograms conveniently and successfully stratified patients with HGSOC according to their risk scores.
In our study, 6 independent prognostic factors were identified for OS: marital status, age, LN dissection, tumor size, residual disease, and FIGO stage. These factors also significantly played the same role in CSS, except for marital status. According to research, generally, younger patients are more likely to have a favorable prognosis because they are more likely to have tumors of less aggressive histology, lower grade, and better baseline performance status (11,27). We observed that the older the age, the less favorable the prognosis.
As for separated, divorced, and widowed (SDW) patients, their social support networks may reflect a lack of personal and social support and they appear to have more psychological distress and financial problems compared with non-SDW women (28). Among women at increased ovarian cancer risk, perceived threat was a unique predictor of cancer risk which influenced early performance behavior. This emphasized the close relationship between psychological state and the incidence of ovarian cancer. Our study found that compared with other patients, SDW patients had less favorable survival outcomes in HGSOC (29).
For patients with epithelial ovarian cancer (EOC), systematic lymphadenectomy is an important part of surgical treatment, because LN status is regarded as a significant prognostic factor (30). The GOG defined a 10-lymph-node cutoff as adequate lymphadenectomy criteria (23). In our study, patients with more than 10 LNs removed had better survival. In contrast, in another study, there was no significant survival improvement in advanced ovarian cancer patients with systematic retroperitoneal lymphadenectomy (31). Therefore, the role of lymphadenectomy in EOC patients, including HGSOC patients, is still controversial and needs further research.
The extent of metastatic disease and metastasis size has important significance for the postoperative pathological staging and prognostic evaluation of patients with ovarian cancer. However, there is no definite consensus on the prognostic influence of tumor size. Another potential reason for the inconformity is the histologic type. In ovarian malignant tumors, the significance of the size of the primary tumor has been demonstrated in sex cord-stromal tumors as follows: the smaller the tumor, the better the prognosis (28). However, in our study, we found that the larger the diameter of the primary tumor, the better the prognosis. We considered whether patients with larger sized tumors might be more prone to earlier diagnosis and treatment.
Residual disease is one of the most significant independent predictors of the prognosis of patients with ovarian cancer (10). Among the previously developed prognostic models or nomograms for ovarian cancer, the assessment of residual disease has not been sufficiently detailed (32,33). The difference was significant among the 3 classifications of residual disease in the nomogram.
Nomogram modelling has performed better than conventional staging systems, and researchers propose the nomogram as a promising tool for prognostic prediction (18,34). The nomogram area under the curve (AUC) used to predict the 5-year OS (0.72, 95% CI: 0.68 to 0.76) for all patients with extra-nodal, nasal-type NK/T-cell lymphoma was significantly higher than the AUC of other prognostic tools (34). Another nomogram was generated to predict the 2-year progression-free survival (PFS), 5-year OS, and pelvic recurrence for locally advanced cervical cancer limited to the pelvis, and achieved C-indices of 0.62, 0.64, and 0.73, respectively, which were well calibrated (18). The nomograms mentioned above demonstrated better performance in prediction than did the staging systems. The nomograms also showed better prediction capacity compared with the FIGO staging system (9) and the AJCC (7th edition) staging system. Via our models, individual treatment plans and follow-up schedules can be made by integrating risk stratification in patient assessment. It may be feasible to use a prediction model to formulate and perform a realistic follow-up plan.
There were several limitations to this study. Firstly, detailed information on chemotherapy was not provided in the SEER database. The SEER data were also lacking details of tumor recurrence, reoperation, and molecular genetic testing. Secondly, because of the nature of the SEER data, some well-known prognostic factors, such as performance status, presence of ascites, and etc. were not included in our study. This might lead to the relative low C-index. Nomogram with a C-index less than 0.8 was not so good and we still need to build a more accurate model to predict survival in the future. Finally, in the present condition of only achieving internal validation, our models need external validation to confirm their performance.
Conclusions
We have set up novel and accurate prognostic prediction models based on the most common histologic type of epithelial ovarian cancer (HGSOC). On the basis of the FIGO staging system, incorporating easy-to-obtain clinicopathological features makes our model low-cost and convenient to use. Our models will help to guide clinical decision-making about individual treatment plans and follow-up schedules using accurate assessments.
Acknowledgments
We thank Xiaofei Ye, senior statistician, for advice.
Funding: None.
Footnote
Reporting Checklist: The authors have completed the TRIPOD reporting checklist. Available at https://atm.amegroups.com/article/view/10.21037/atm-21-4383/rc
Conflicts of Interest: All authors have completed the ICMJE uniform disclosure form (available at https://atm.amegroups.com/article/view/10.21037/atm-21-4383/coif). The authors have no conflicts of interest to declare.
Ethical Statement: The authors are accountable for all aspects of the work in ensuring that questions related to the accuracy or integrity of any part of the work are appropriately investigated and resolved. The study was conducted in accordance with the Declaration of Helsinki (as revised in 2013). No personally identifying information was used in the study, which eliminated the requirement for Institutional Review Board approval or informed patient consent.
Open Access Statement: This is an Open Access article distributed in accordance with the Creative Commons Attribution-NonCommercial-NoDerivs 4.0 International License (CC BY-NC-ND 4.0), which permits the non-commercial replication and distribution of the article with the strict proviso that no changes or edits are made and the original work is properly cited (including links to both the formal publication through the relevant DOI and the license). See: https://creativecommons.org/licenses/by-nc-nd/4.0/.
References
- Bray F, Ferlay J, Soerjomataram I, et al. Global cancer statistics 2018: GLOBOCAN estimates of incidence and mortality worldwide for 36 cancers in 185 countries. CA Cancer J Clin 2018;68:394-424. [Crossref] [PubMed]
- Vickers AJ. Prediction models in cancer care. CA Cancer J Clin 2011;61:315-26. [PubMed]
- Lee K, Tavassoli FA, Prat J, et al. Tumors of the ovary and peritoneum. World Health Organization Classification of Tumours: Pathology and Genetics of Tumours of the Breast and Female Genital Organs. France: Lyon, 2003:117.
- Boussios S, Moschetta M, Zarkavelis G, et al. Ovarian sex-cord stromal tumours and small cell tumours: Pathological, genetic and management aspects. Crit Rev Oncol Hematol 2017;120:43-51. [Crossref] [PubMed]
- Köbel M, Kalloger SE, Huntsman DG, et al. Differences in tumor type in low-stage versus high-stage ovarian carcinomas. Int J Gynecol Pathol 2010;29:203-11. [Crossref] [PubMed]
- Machida H, Matsuo K, Yamagami W, et al. Trends and characteristics of epithelial ovarian cancer in Japan between 2002 and 2015: A JSGO-JSOG joint study. Gynecol Oncol 2019;153:589-96. [Crossref] [PubMed]
- Kurman RJ. Origin and molecular pathogenesis of ovarian high-grade serous carcinoma. Ann Oncol 2013;24:x16-21. [Crossref] [PubMed]
- Moschetta M, Boussios S, Rassy E, et al. Neoadjuvant treatment for newly diagnosed advanced ovarian cancer: where do we stand and where are we going? Ann Transl Med 2020;8:1710. [Crossref] [PubMed]
- van de Laar R. External validation of three prognostic models for overall survival in patients with advanced-stage epithelial ovarian cancer. Br J Cancer 2014;110:42-8. [Crossref] [PubMed]
- Winter WE 3rd, Maxwell GL, Tian C, et al. Prognostic factors for stage III epithelial ovarian cancer: a Gynecologic Oncology Group Study. J Clin Oncol 2007;25:3621-7. [Crossref] [PubMed]
- Klar M, Hasenburg A, Hasanov M, et al. Prognostic factors in young ovarian cancer patients: An analysis of four prospective phase III intergroup trials of the AGO Study Group, GINECO and NSGO. Eur J Cancer 2016;66:114-24. [Crossref] [PubMed]
- Zhu T, Chen R, Wang J, et al. The prognostic value of ITGA and ITGB superfamily members in patients with high grade serous ovarian cancer. Cancer Cell Int 2020;20:257. [Crossref] [PubMed]
- Xu L, Wu Y, Che X, et al. Cox-LASSO Analysis Reveals a Ten-lncRNA Signature to Predict Outcomes in Patients with High-Grade Serous Ovarian Cancer. DNA Cell Biol 2019;38:1519-28. [Crossref] [PubMed]
- Feng C, Xu Y, Liu Y, et al. Gene Expression Subtyping Reveals Immune alterations:TCGA Database for Prognosis in Ovarian Serous Cystadenocarcinoma. Front Mol Biosci 2021;8:619027. [Crossref] [PubMed]
- An Y, Bi F, You Y, et al. Development of a Novel Autophagy-related Prognostic Signature for Serous Ovarian Cancer. J Cancer 2018;9:4058-71. [Crossref] [PubMed]
- Wang X, Lu Z. Radiomics Analysis of PET and CT Components of 18F-FDG PET/CT Imaging for Prediction of Progression-Free Survival in Advanced High-Grade Serous Ovarian Cancer. Front Oncol 2021;11:638124. [Crossref] [PubMed]
- Hong Y, Liu Z, Lin D, et al. Development of a radiomic-clinical nomogram for prediction of survival in patients with serous ovarian cancer. Clin Radiol 2022;77:352-9. [Crossref] [PubMed]
- Rose PG, Java J, Whitney CW, et al. Nomograms Predicting Progression-Free Survival, Overall Survival, and Pelvic Recurrence in Locally Advanced Cervical Cancer Developed From an Analysis of Identifiable Prognostic Factors in Patients From NRG Oncology/Gynecologic Oncology Group Randomized Trials of Chemoradiotherapy. J Clin Oncol 2015;33:2136-42. [Crossref] [PubMed]
- Guo Y, Jiang T, Ouyang L, et al. A novel diagnostic nomogram based on serological and ultrasound findings for preoperative prediction of malignancy in patients with ovarian masses. Gynecol Oncol 2021;160:704-12. [Crossref] [PubMed]
- Barlin JN, Yu C, Hill EK, et al. Nomogram for predicting 5-year disease-specific mortality after primary surgery for epithelial ovarian cancer. Gynecol Oncol 2012;125:25-30. [Crossref] [PubMed]
- Surveillance, Epidemiology, and End Results (SEER) Program: Incidence-SEER 18 Regs Custom Data (with additional treatment fields), Nov 2018 Sub (1975–2016 varying) National Cancer Institute, DCCPS, Surveillance Research Program, released April 2019, based on the November 2018 submission.
- Luo P, Zhou JG, Jin SH, et al. Influence of marital status on overall survival in patients with ovarian serous carcinoma: finding from the surveillance epidemiology and end results (SEER) database. J Ovarian Res 2019;12:126. [Crossref] [PubMed]
- Mahdi H, Moslemi-Kebria M, Levinson KL, et al. Prevalence and prognostic impact of lymphadenectomy and lymph node metastasis in clinically early-stage ovarian clear cell carcinoma. Int J Gynecol Cancer 2013;23:1226-30. [Crossref] [PubMed]
- Harrell FE Jr, Lee KL, Mark DB. Multivariable prognostic models: issues in developing models, evaluating assumptions and adequacy, and measuring and reducing errors. Stat Med 1996;15:361-87. [Crossref] [PubMed]
- Vickers AJ, Elkin EB. Decision curve analysis: a novel method for evaluating prediction models. Med Decis Making 2006;26:565-74. [Crossref] [PubMed]
- Boussios S, Karathanasi A, Cooke D, et al. PARP Inhibitors in Ovarian Cancer: The Route to "Ithaca". Diagnostics (Basel) 2019;9:55. [Crossref] [PubMed]
- Berman ML. Future directions in the surgical management of ovarian cancer. Gynecol Oncol 2003;90:S33-9. [Crossref] [PubMed]
- Lindström M. Marital status, social capital, material conditions and self-rated health: a population-based study. Health Policy 2009;93:172-9. [Crossref] [PubMed]
- Smits S, Boivin J, Menon U, et al. Influences on anticipated time to ovarian cancer symptom presentation in women at increased risk compared to population risk of ovarian cancer. BMC Cancer 2017;17:814. [Crossref] [PubMed]
- Prat J. Ovarian, fallopian tube and peritoneal cancer staging: Rationale and explanation of new FIGO staging 2013. Best Pract Res Clin Obstet Gynaecol 2015;29:858-69. [Crossref] [PubMed]
- Harter P, Sehouli J, Lorusso D, et al. A Randomized Trial of Lymphadenectomy in Patients with Advanced Ovarian Neoplasms. N Engl J Med 2019;380:822-32. [Crossref] [PubMed]
- Kim SI, Song M, Hwangbo S, et al. Development of Web-Based Nomograms to Predict Treatment Response and Prognosis of Epithelial Ovarian Cancer. Cancer Res Treat 2019;51:1144-55. [Crossref] [PubMed]
- Gerestein CG, Eijkemans MJ, de Jong D, et al. The prediction of progression-free and overall survival in women with an advanced stage of epithelial ovarian carcinoma. BJOG 2009;116:372-80. [Crossref] [PubMed]
- Chen SY, Yang Y, Qi SN, et al. Validation of nomogram-revised risk index and comparison with other models for extranodal nasal-type NK/T-cell lymphoma in the modern chemotherapy era: indication for prognostication and clinical decision-making. Leukemia 2021;35:130-42. [Crossref] [PubMed]