Bioinformatic analysis of cancer-associated fibroblast related gene signature as a predictive model in clinical outcomes and immune characteristics of gastric cancer
Introduction
Gastric cancer (GC) is one of the most common malignant tumors globally, ranking fifth for incidence and fourth for mortality in the world (1). However, tools to predict the prognosis of GC currently are inadequate, and there is currently a lack of biomarkers for predicting prognosis as well as the responsiveness to therapy in patients. For example, traditional gastrointestinal tumor biomarkers including carcinoembryonic antigen (CEA), carbohydrate antigen 19-9 (CA19-9), and carbohydrate antigen 72-4 (CA72-4) have limited sensitivity and specificity in the diagnosis of gastric cancer. TNM stage is the primary tool used to predict the prognosis of patients with GC. However, GC patients of the same TNM stage often show different clinical outcomes, suggesting that there are other factors that influence long-term outcomes. Thus, the development of novel biomarkers for GC which could predict prognosis and immunotherapy response with excellent predictive performance would be significant.
The tumor microenvironment (TME) harbors malignant cancer cells, immune cells, stromal cells, and other components, such as blood vessels, extracellular matrix, and cytokines, all of which participate in tumorigenesis and cancer progression (2). Among cells in the TME, cancer-associated fibroblasts (CAFs) are the most prevalent and essential stromal cells correlated with an unfavorable prognosis in multiple cancers. It was reported that CAFs could promote cancer progression by secreting growth factors, cytokines, and chemokines (3). Furthermore, CAFs contribute to immune evasion by upregulating immunosuppressive cytokines and immune checkpoint ligands, preventing anti-tumor CD8+ T cell infiltration, and effecting an anti-tumor response via crosstalk with other immune cells (4). Consequently, CAFs usually contribute to an unfavorable prognosis. Recently, Herrera et al. [2021] developed a CAF-derived gene signature to predict the prognosis of colon cancer patients. They identified 596 CAF-associated genes, which were used to develop a “CAF signature” and showed great predictive robustness (5). They demonstrated that CAF-associated genes may act as biomarkers to reveal the clinical outcome of colon cancer patients. GC and colon cancer are both gastrointestinal tumors and share similarities in pathological characteristics. Consequently, using CAF-associated genes to forecast the prognosis of patients with GC could be a promising strategy.
In this study, in order to screen out CAF genes related to overall survival, we performed univariate COX proportional hazards regression based on the 596 CAF-associated genes identified by Herrera et al. [2021] (5) and then construct a CAF gene signature (CAFGS) to estimate prognosis in GC patients. Screening of 43 prognostic genes and construction of CAFGS (10 candidate genes signature) were achieved using Least Absolute Shrinkage and Selection Operator (LASSO) regression. Furthermore, we indicated the significant role of CAFGS in immune cell infiltration as well as in immune checkpoint inhibitor (ICI) responses in GC patients. We present the following article in accordance with the TRIPOD reporting checklist (available at https://atm.amegroups.com/article/view/10.21037/atm-22-2810/rc).
Methods
Public datasets and sample collection
We collected gene expression profiles along with the relevant clinical data from The Cancer Genome Atlas Stomach Adenocarcinoma (TCGA-STAD), GSE62254, and GSE84437 datasets. The training set contained RNA-seq data from 353 patients [Illumina HiSeq 2000, log2(x+1) transformed RSEM normalized read count] and corresponding clinical information from the TCGA-STAD, which were retrieved from the Cancer Genomics Browser of the University of California Santa Cruz (UCSC). The validation datasets included two microarray gene expression matrixes with processed series matrix files collected from the Gene Expression Omnibus (GEO) database, including GSE62254 [n=300, log2(x+1) transformed RMA normalized read count], GSE84437 [n=433, log2(x+1) transformed RMA normalized read count]. We utilized the average expression value for the genes that had several probes. Furthermore, we collected 48 surgically resected GC tissues from the Xijing Hospital of Digestive Disease between July 2015 and September 2015. Table S1 provides a summary of the relevant clinicopathological information. A further 596 CAF-associated genes were obtained from the study of Herrera (5). This study was a bioinformatics analysis. Figure 1A depicts the flowchart for the present research. Firstly, we performed univariate COX proportional hazards regression to identified CAF genes related with OS. Then we deceloped and validated a CAF related predictive model in four GC cohorts. Finally, we indicated the significant role of CAFGS in immune cell infiltration as well as in immune checkpoint inhibitor (ICI) responses in GC patients.
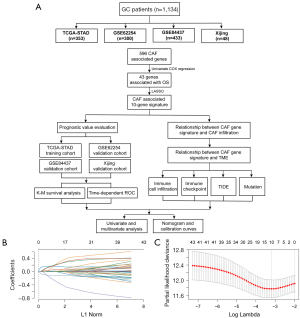
Selection of prognostic genes
Univariate Cox regression analysis was performed according to the expression of 596 CAF-associated genes to examine the correlation between CAF genes and overall survival (OS) in the TCGA-STAD dataset. CAF genes with P value <0.05 were recognized as significant prognostic genes.
Construction and evaluation of CAFGS
We performed LASSO regression analysis on the basis of the abovementioned prognostic CAF genes with 10-fold cross-validation (6). Based on the LASSO regression coefficients and gene expression, the CAFGS score was calculated utilizing the equation as follows: CAFGS score = coefficient 1 × expression of gene 1 + … + coefficient n × expression of gene n. Participants in the TCGA-STAD and GSE62254 datasets were classified into low- and high-risk groups based on their median CAFGS score, which served as a cut-off value for the classification. Patients in GSE84437 and Xijing validation cohorts were classified into low- and high-risk groups based on the best threshold value, which had been computed utilizing the “survminer” R package. The CAFGS prognostic performance was assessed by time-dependent receiver operating characteristic (ROC) curves and Kaplan-Meier survival analysis. The Wilcoxon rank-sum test was utilized to examine the differences between the two groups for a variety of clinicopathological factors.
Differentially expressed genes (DEGs) identification and functional enrichment analysis
DEGs (fold change >1.5, adjusted P value <0.05) between high- and low-risk groups were determined utilizing “limma” R package. Subsequently, we utilized the R package “clusterProfiler” to conduct Gene Ontology (GO) and the Kyoto Encyclopedia of Genes and Genomes (KEGG) analyses. Gene Set Enrichment Analysis (GSEA) was also carried out to illustrate the differences of signaling pathways between high- and low-risk groups. Gene sets with P value <0.05 and false discovery rates (FDRs) <0.25 were deemed significant.
Estimation of CAF infiltration and correlation analysis
CAF infiltration in the TME was evaluated by three algorithms: EPIC (7), xCell (8), and MCPcounter (9), which was achieved by the “immunedeconv” R package. Spearman correlation estimated the relationship between CAFGS and the infiltration of CAF predicted by these algorithms.
Estimation of immune cell infiltration
We utilized CIBERSORT, TIMER, and ssGSEA to approximate immune cell infiltration, and 22 kinds of immune cells were evaluated by CIBERSORT for their relative percentage in the TME (10). The gene signature of the 22 immune cell types was termed LM22, which contained 547 genes capable of distinguishing between distinct immune cell types. TIMER was used to examine the correlation between CAFGS and major immune cells (11). Single sample gene set enrichment analysis (ssGSEA) was also utilized to define the immune infiltration status of GC samples by calculating the normalized enrichment score (NES). The immune cell gene set was derived from TISIDB, an integrated database for tumor and immune system interaction.
Estimation of immune and stromal scores
ESTIMATE is an algorithm estimating the levels of infiltrating immune and stromal cells in the TME utilizing transcriptional gene expression data (12). Here, we used this algorithm to calculate the percentage of immune and stromal cells in GC.
Tumor immune dysfunction and exclusion (TIDE) analysis
TIDE is a technique established to assess the potential of immune evasion based on transcriptional profiles (13) which employs a collection of markers to model two primary processes of immune evasion: the dysfunction of tumor-infiltrating cytotoxic T lymphocytes (CTL) and the exclusion of CTL by immunosuppressive factors. The elevated TIDE score reflects the poorer effectiveness of ICI therapy and shortened survival time after receiving ICI therapy. We downloaded the TIDE estimation results of the TCGA-STAD from the TIDE website (http://tide.dfci.harvard.edu/).
Acquisition of gene mutation information
The somatic mutation information of the TCGA-STAD dataset was obtained via the “TCGAbiolinks” R package, and the “maftools” R package was utilized to visualize the top 20 genes with the highest mutation frequency in both groups. Microsatellite instability (MSI) and tumor mutation burden (TMB) scores of the TCGA-STAD were downloaded from the website (Assistant for clinical bioinformatics, http://www.aclbi.com/). Spearman correlation analyses examined the correlation between CAFGS score and TMB/MSI score, and the TMB/MSI scores between the two groups were subjected to Wilcoxon rank-sum test for comparison.
Identification of independent prognostic variables and construction of nomogram
Univariate and multivariate Cox regression analyses were conducted to determine if CAFGS independently functioned as a significant prognostic marker. Nomograms were then developed for the GSE62254 and TCGA-STAD cohorts. Calibration curves and ROC curves were employed to evaluate predictive performance of these nomograms. Furthermore, decision curve analysis (DCA) was used to quantify their clinical utility.
Patients and tissue specimens
From July 2015 to September 2015, we collected a total of 48 surgically excised gastric adenocarcinoma tissues from patients enrolled at the Xijing Hospital of Digestive Disease. Isolation of total RNA from these samples was then performed utilizing an RNA extracting kit (Qiagen, Germany; #74106). To synthesize cDNA from 1000 ng of total RNA, we utilized the PrimeScript™ RT Master Mix kit (Takara, Japan; #PR036A-1), and quantitative real-time PCR (qPCR) determined the relative mRNA amount of genes. β-actin was the internal control. Table S2 presents the primer sequences utilized. The relative expression of prognostic genes was determined by 2−ΔΔCT, then log2 transformed for signature validation. Subjects who participated signed a formal informed consent document, and the research was performed in conformity with the Declaration of Helsinki (as revised in 2013). This study was approved by the Ethical Committee of Xijing Hospital (No. KY20192088-F-1).
Statistical analysis
Univariate Cox regression analysis and Kaplan-Meier survival analysis were employed in identifying prognostic CAF-associated genes. Spearman correlation analysis was adopted to examine the correlation between CAFGS scores and other variables, and the Wilcoxon rank-sum test was conducted to probe into significant differences between two groups. Two-tailed P values were interpreted statistically significant when they were less than 0.05. To conduct statistical analysis, we utilized the tools GraphPad Prism (version: 8.0), SPSS (version: 24.0), and R (version: 3.6.1).
Results
Identification of CAF genes correlated with OS
To screen out CAF genes related to OS, we performed univariate COX proportional hazards regression based on 596 CAF-associated genes. We then dichotomously divided patients in the TCGA-STAD by the median expression value of each prognostic gene, and the low- and high-expression groups were subjected to Kaplan-Meier survival analyses to compare the survival difference between the two groups. Finally, we demonstrated that 43 CAF genes were substantially associated with OS (Figure S1), among which 31 were determined as “high-risk” genes, with hazard ratios (HR) greater than 1, and 12 were discovered to be protective genes with HR less than 1.
Development and confirmation of CAFGS
To construct a gene signature for prognosis prediction, the remaining 43 genes were subject to LASSO regression analysis (Figure 1B,1C), and ten (ANGPTL4, CPNE8, CST2, HTR1F, IL1RAP, NR1D1, NTAN1, OLFML2B, TMEM259, VTN) were revealed and utilized to establish a gene signature. The risk score of CAFGS based on the LASSO coefficients and gene expression level were calculated as follows: CFAGS score = (0.0019) × ANGPTL4 + (0.2069) × CPNE8 + (0.0054) × CST2 + (0.1564) × HTR1F + (0.0019) × IL1RAP + (0.1624) × NR1D1 + (0.1771) × NTAN1 + (0.0123) × OLFML2B + (−0.2786) × TMEM259 + (0.0609) × VTN. Patients in the TCGA-STAD cohort were classified into low-risk and high-risk groups based on the median CAFGS score. Figure 2A shows that, with the increase of CAFGS, the mortality rates of GC patients also increased, and the CAFGS scores of the deceased patients were also considerably higher than those of the survivors (P<0.001) (Figure 2B). High-risk group patients had a substantially lower OS in contrast with those in the low-risk group (HR =2.90, 95% CI: 2.08–4.03, P<0.001) (Figure 2C). Time-dependent ROC curves illustrated that the 5-year area under the curve (AUC) of CAFGS reached 0.713 in the TCGA-STAD training cohort (Figure 2D). Moreover, the CAFGS score was positively correlated with the TNM stage in GC patients, and a higher CAFGS score was found in advanced TNM stages (Figure 2E).
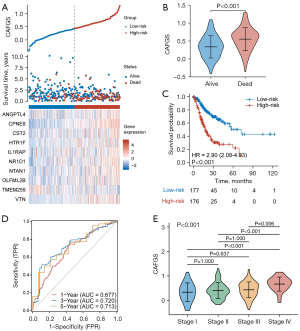
To validate the results, we used GSE62254 and GSE84437 cohorts as validation datasets. With the same formula, GC patients were classified into high-risk and low-risk groups according to the optimal cutoff value, and the results showed patients having lower CAFGS scores exhibited considerably extended OS times as opposed to those with elevated scores (GSE62254: HR =1.58, 95% CI: 1.15–2.17, P=0.005; GSE84437: HR =1.36, 95% CI: 1.04–1.79, P=0.025) (Figure 3A,3B). Furthermore, we collected 48 GC tissues from Xijing Hospital of Digestive Disease, and after RNA extraction and qPCR, obtained the relative expression of 10 genes in GC patients. CAFGS were then calculated as described above, and their distribution, survival status, and expression of the ten genes are shown in Figure 3C. Similarly, patients with high CAFGS showed worse outcome and deceased patients had higher CAFGS scores than those still surviving (HR =2.67, 95% CI: 0.95–7.52, P=0.022) (Figure 3D-3E), implying a higher CAFGS score may indicate worse outcomes in GC patients.
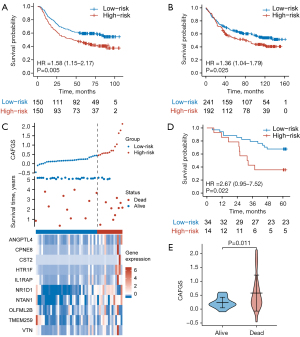
CAFGS correlated with CAF infiltration in GC
To test whether CAFGS could precisely represent the infiltration of CAF, we performed correlation analysis between CAFGS and classical CAF markers, such as vimentin (VIM), platelet-derived growth factor receptors (PDGFR), fibroblast activation protein (FAP), α-smooth muscle actin (α-SMA), and S100 Calcium Binding Protein A4 (S100A4). The findings illustrated that CAFGS exhibited a positive correlation with the expression of CAF marker genes, such as ACTA2 (R=0.50), FAP (R=0.41), VIM (R=0.46), PDGFRA (R=0.40), and PDGFRB (R=0.51) (Figure 4A) in the TCGA-STAD dataset. Moreover, to verify the robustness of CAFGS as an indicator in estimating CAF infiltration, we used three algorithms (EPIC, xCell, and MCP-counter) to estimate the infiltration of CAFs in the TME. The findings demonstrated a positive correlation between CAFGS score and CAF infiltration in the TCGA-STAD dataset (R=0.280, R=0.330, R=0.350) (Figure 4B-4D). In addition, we verified the results in the GSE62254 dataset. CAFGS score was also positively correlated with the expression of CAF marker genes (Figure S2A), and CAFGS score was positively correlated with CAF infiltration estimated by the three algorithms (R=0.470, R=0.230, R=0.510) (Figure S2B-S2D). We also validated the similar results in the GSE84437 dataset (Figure S2E-S2H). All above data demonstrated CAFGS could well represent the infiltration of CAF in the TME.
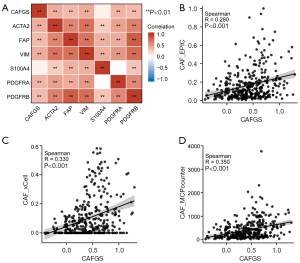
Identification of CAFGS associated biological mechanisms
In view of the strong stratification ability of CAFGS, we investigated CAF-related biological alterations in both low- and high-risk groups by firstly identifying DEGs between low- and high-risk groups in the TCGA-STAD cohort (n=353). In total, 177 DEGs (fold change >1.5, adjusted P value <0.05) were identified and subsequently subjected to GO/KEGG analysis, and the results of GO demonstrated that extracellular matrix-related terms were enriched substantially in high-risk groups. Similarly, the top five enriched terms of KEGG analysis were cell adhesion molecules, protein digestion, PI3K-Akt signaling pathway, focal adhesion, ECM-receptor interaction, and absorption, which are highly correlated with the biological functions of CAFs (Figure S3A). The results in GSE62254 (n=300) and GSE84437 (n=433) validation sets showed a similar trend (Figure S3B,S3C).
Furthermore, we conducted GSEA between low- and high-risk groups, and from the three datasets, epithelial-mesenchymal transition (EMT) was shown to be enriched substantially in the high-risk group, which is in compliance with the biological behavior and oncogenic role of CAFs in aggressive GC. Moreover, a substantial enrichment was discovered in hedgehog signaling, TGF-β signaling, KRAS signaling, and angiogenesis in the high-risk group. The findings indicated CAFs could perform a cancer-promoting role in GC by activating these pathways (Figure 5A-5C).
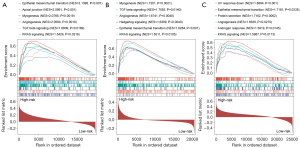
Relationship between CAFGS and TME
Subsequently, using ssGSEA and CIBERSORT algorithms, we examined the correlation between CAFGS and immune cells in GC. Results of ssGSEA in the TCGA-STAD dataset illustrated T follicular helper cells, immature dendritic cells, natural killer cells, macrophages, plasmacytoid dendritic cells, myeloid-derived suppressor cells (MDSCs), mast cells, natural killer T cells, effector memory CD4+ T cells, regulatory T cells (Tregs), central memory CD4+ T cells, and type 1 T helper cells were significantly enriched in the high-risk group, while type 17 T helper cells and activated CD4+ T cells were enriched in the low-risk group (Figure 6A). In addition, to approximate the levels of infiltrating immune cells, we employed the CIBERSORT algorithm, and the results showed plasma B cells, resting mast cells, M2 macrophages, and monocytes exhibited a greater abundance in the high-risk group. However, activated mast cells and follicular helper T cells were enriched in the low-risk group (Figure 6B). We also performed ssGSEA and CIBERSORT in the GSE62254 (Figure S4) and GSE84437 (Figure S5) validation datasets. Among the three datasets, T follicular helper cells, macrophages, immature dendritic cells, plasmacytoid dendritic cells, mast cells, MDSCs, effector memory CD4+ T cells, Tregs, central memory CD4+ T cells, type 1 T helper cells, and MDSCs, were all substantially enriched in the high-risk groups, suggesting CAF infiltration in GC may contribute to poor prognosis by recruiting these immunosuppressive cells in the TME. Notably, despite the results of CIBERSORT varying in three datasets, the high-risk group in each exhibited more resting mast cells as opposed to the low-risk group, illustrating that CAFs and mast cells may have reciprocal interaction, and that CAFs may inhibit the activation of mast cells.
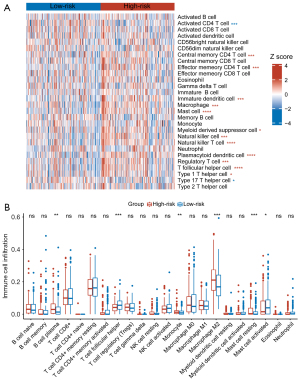
As CAFs were reported to regulate the polarization of macrophages in the TME, we investigated their relationship with macrophages, and the results of TIMER showed macrophages were strongly correlated with CAFGS in the three datasets (Figure S6A). As shown by CIBERSORT, the M2 macrophage infiltration level was higher in high CAFGS patients. Combining the above results, we illustrated that CAFs may promote the M2 polarization of macrophages.
Moreover, we used ESTIMATE to explore the composition of the TME in low- and high-risk groups. The findings illustrated that stromal and estimate scores were significantly elevated in the high-risk group, while immune scores showed no difference in the TCGA-STAD cohort (Figure S6B-S6D), whereas tumor purity was reduced (Figure S6E), and similar results were seen in the validation datasets of GSE62254 and GSE84437 (Figure S6F-S6M). These aforementioned results suggested the high-risk group was characterised by an elevation in stromal cell infiltration, such as CAFs, and the characteristics of the low-risk group included a stromal fibroblast-low microenvironment.
Relationship between CAFGS and ICI responsiveness
ICI therapy is an important aspect of GC treatment. As mentioned above, CAFs could influence the responsiveness of ICI therapy by regulating the TME. Therefore, we explored the relationship between CAFGS and responsiveness to ICI by analyzing their correlation with a variety of extensively utilized ICI biomarkers. The mRNA expression of immune checkpoint genes was examined first. Higher expression of PD-L2, TIM-3, and CD276 was observed in the high-risk group. Nevertheless, CTLA4, PD-1, and PD-L1 expression in the two groups seemed to make no difference (Figure 7A). Moreover, we used TIDE, an algorithm calculating T cell dysfunction and an exclusion criterion to predict ICI responsiveness and found TIDE scores were higher in the high-risk group than the low-risk group (Figure 7B). The T cell exclusion and T cell dysfunction scores were also elevated in the high-risk group (Figure 7C,7D). These results implied patients with higher CAFGS scores may have poorer ICI efficacy, and that their survival time is shorter after receiving ICI treatment, suggesting CAFGS may function as a prognosis biomarker for immunotherapy in GC.
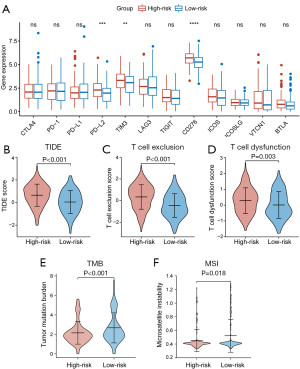
We then investigated the correlation between CAFGS and tumor mutation load. It is widely acknowledged that tumor mutation burden (TMB) and microsatellite instability (MSI) are reliable biomarkers for immunotherapy (14), and we demonstrated an inverse association between CAFGS score and TMB/MSI. Moreover, lower levels of TMB and MSI scores were observed in the high-risk group (Figure 7E,7F), suggesting patients with high CAFGS scores may have a poor response for immunotherapy due to the low tumor mutation burden.
Relationship between CAFGS and somatic variation
The 20 most commonly mutated genes in the high- (Figure 8A) and low- (Figure 8B) risk groups were displayed as waterfall plots, among which some genes existed in both two groups, including TP53, TTN, MUC16, LRP1B, SYNE1, CSMD3, FLG, ARID1A, CSMD1, RYR2, FAT4, PCLO, and FAT3. Mutations of SPTA1, DNAH5, AHNAK2, DNAH11, PCDH15, and ZFHX4 genes were prevalent in the high-risk group, whereas mutations of KMT2D, OBSCN, PIK3CA, HMCN1, LAMA1, PLEC, and SACS were uniquely seen in the low-risk group.
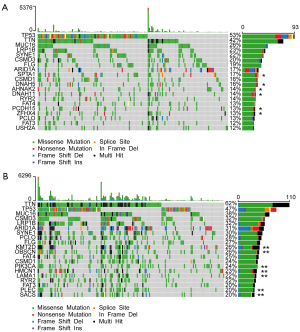
CAFGS independently served as a prognostic indicator for GC patients
The above results illustrated that CAFGS could serve as a promising prognosis biomarker for GC patients. Consequently, to determine if CAFGS independently served as an independent prognostic indicator, we conducted univariate and multivariable Cox regression analyses in the GSE62254 and TCGA cohorts. The findings demonstrated CAFGS (HR =5.52, 95% CI: 3.24–9.41, P<0.001), T stage (HR =1.35, 95% CI: 1.07–1.71, P=0.011), N stage (HR =1.21, 95% CI: 1.04–1.41, P=0.016), and age (HR =1.03, 95% CI: 1.01–1.05, P=0.001) were independently correlated with OS in the TCGA-STAD cohort (Figure 9A), and CAFGS (HR =5.75, 95% CI: 2.05–16.12, P=0.001) was consistently validated as an independent prognostic indicator in the GSE62254 cohort (Figure S7A).
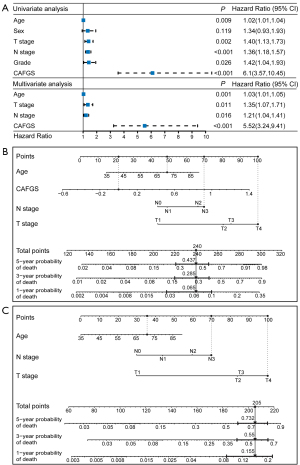
Development of CAFGS nomogram and clinicopathological nomogram
A nomogram integrating independent prognostic variables, including CAFGS, was developed for predicting the mortality rate of GC patients in the TCGA-STAD (Figure 9B) and GSE62254 (Figure S7B) cohorts, respectively. A clinicopathological nomogram without CAFGS was also developed (Figure 9C, Figure S7C). The predictive performance of the CAFGS nomogram was then compared with the clinicopathological nomogram, and the results showed the CAFGS model had better predictive performance. At the time point of 3 years, the calibration curves of the CAFGS nomogram exhibited greater concordance with the actual survival rate in contrast with the calibration curves of the clinicopathological nomogram (Figure 10A,10B), and DCA curves showed that the CAFGS model had a better net benefit than the clinicopathological model (Figure 10C,10D). Finally, time-dependent ROC curves showed the CAFGS nomogram had preferable predictive performance compared with the clinicopathological nomogram in both datasets of TCGA-STAD and GSE62254 cohorts. The 5-year AUC of CAFGS nomogram reached 0.806 in the TCGA-STAD dataset, while the 5-year AUC of CAFGS nomogram was 0.781 in the GSE62254 validation dataset (Figure 10E,10F).
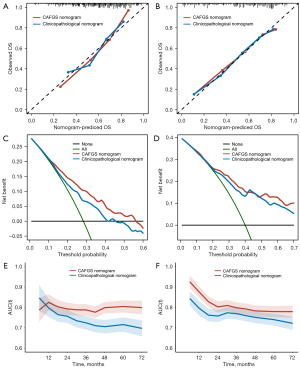
Discussion
CAFs have been shown to perform critical functions in tumor initiation, progression, and therapeutic resistance. Furthermore, they could promote immune evasion of cancer by interacting with cancer cells and immune cells in the TME (15). Recently, Herrera et al. [2021] developed a CAF-derived gene signature which contained 596 genes and showed great prognostic significance in colon cancer patients (5). GC, especially undifferentiated carcinoma of the stomach, often manifests massive fibrosis accompanied by increased infiltration of CAFs. Consequently, using 596 CAF-associated genes identified by Herrera et al. [2021] (5), we established a CAF gene signature (CAFGS) to evaluate the prognosis of GC, and the results showed CAFGS had great value in prognosis evaluation of the disease. Moreover, CAFGS had a close relationship with immunotherapy efficacy and immune cell infiltration status in GC patients. These findings are essential as GC was usually associated with poor prognosis and invalid therapeutic response to immunotherapy.
In this study, 10 CAF-associated genes (ANGPTL4, CPNE8, CST2, HTR1F, IL1RAP, NR1D1, NTAN1, OLFML2B, TMEM259, VTN) were finally identified and used to establish a CAFGS. Among these biomarkers, VTN, ANGPTL4, and IL1RAP were most closely related to CAF. For instance, VTN is a protein-coding gene encoding vitronectin, which is an adhesive glycoprotein promoting cell adhesion in the extracellular matrix (ECM) through binding to different types of integrins (16). There is a close relationship between CAFs and VTN, as CAFs are involved in extracellular matrix remodeling as well and trigger invasion mainly via integrin. CAFs highly express VTN to shape the fibrotic TME (17). Moreover, serum VTN was reported to be diagnostic and a prognostic biomarker in breast cancer (18), melanoma (19), prostate cancer (20), and hepatitis B-related liver cancer (21). Overexpression of ANGPTL4 was correlated with poor prognosis of cancer patients also. In GC cells in virto, knockdown of ANGPTL4 inhibits progression of the disease (22), suggesting CAF-derived ANGPTL4 may play an oncogenic role in TME. Analogously, CAF was reported to secrete ANGPTL4, MMP13, and STC1, which could promote proliferation of breast cancer cells (23). In gallbladder cancer, the expression of ANGPTL4 was also upregulated in CAFs, and in the stroma of xenograft tumors in nude mice and in human gallbladder cancer, ANGPTL4 was significantly upregulated (24). In addition, IL1RAP was identified as a CAF-associated gene in our study. IL1RAP encodes an accessory protein for the IL-1 receptor complex, contributing to the activation of the IL-1 signaling pathway. The soluble form of IL1RAP could also be detected in serum (25). In GC, IL1RAP was shown to regulate inflammation and apoptosis, and its knockdown could inhibit tumor progression (26). IL1RAP and its ligand IL-33, were highly expressed in myofibroblasts in the pancreas (27). Moreover, IL1RAP is essential for the activation of T lymphocytes and mast cells induced by IL-33 (28), and could also upregulate CD47 to inhibit macrophage phagocytosis (29). Recently the potential role of IL1RAP on TME-related inflammatory factors in stomach carcinoma has been reported (26). It is interesting to note that VTN, ANGPTL4, and IL1RAP can be detected in serum, which lays the foundation for the development of tumor biomarkers in human peripheral blood.
As for the remaining genes, CST2 encodes a type 2 cysteine protease inhibitor, which exists in various body fluids, especially saliva, tears, and semen. Existing research showed the expression of CST2 has a certain value for the diagnosis of prostate cancer, breast cancer, and GC (30,31). Another recent report has demonstrated that CST2 overexpression was closely connected with the cell proliferation and migration as well as TGF-β signalling pathway (32), which coincided with our GSEA results in three datasets (Figure 5A-5C). NR1D1 encodes a transcription factor that negatively regulates the expression of core clock proteins, which is associated with immune cell infiltration in stomach adenocarcinoma (33), and OLFML2B was reported to be correlated with poor prognosis of GC (32,34). However, the functions of the remaining four genes, CPNE8, HTR1F, NTAN1, and TMEM259, necessitate further study.
Our results showed CAFGS had excellent performance in prognosis evaluation of GC patients, and a high CAFGS score is characterized by activation of extracellular matrix remodeling. GSEA also confirmed that CAFGS is closely associated with activation of EMT and the TGF-β signaling pathway, which are both typical features of fibrogenesis (35). However, the most interesting finding of this study lies in the relationship between CAFGS and the immune evasion mechanism of GC. A previous study reported that CAFs could promote the immune evasion of cancer cells to influence immunotherapy efficacy (36), and in the present research, we explored the possibility that CAFGS might serve as a biomarker for immunotherapeutic responsiveness. We hypothesized that patients with high CAFGS scores may have poor ICI therapy efficacy, and the reasons may lie in the immunosuppressive microenvironment shaped by CAFs. Results of ssGSEA showed macrophages, MDSCs, Tregs, and other immunosuppressive cells were considerably enriched in the high CAF infiltration group, which may interact with CAFs to regulate the TME. For example, in our study, we showed that CAFs were associated with macrophages in three datasets. Specifically, CAFs were positively correlated with M2, but not M1 macrophage infiltration in GC (37). Interestingly, macrophage co-culture with GC cells converted M1 phenotype into M2 phenotype, and the contribution of the M2 phenotype in tumor progression has been evidenced in clinical studies of intraperitoneal tumor-associated macrophage in GC patients with peritoneal dissemination (38). Furthermore, previous studies showed that CAFs could recruit macrophages into the TME and promote M2 polarization, and our study is consistent with previous studies (39). CAFs could secrete SDF-1, IL-6, IL-8, IL-10, and TGF-β to promote the differentiation of M2 macrophages, which in turn could accelerate tumor growth, angiogenesis, EMT, and immune evasion (40). Moreover, M2 macrophages can release TGF-β, which in turn promotes the formation and maintenance of CAFs (41). CAFs and M2 macrophages can also produce MMPs, TGF-β, cyclooxygenase-2, and IL-6 to create a tumor-promoting microenvironment (42). In addition, CAF associated genes identified by this study were involved in immune evasion. For example, ANGPTL4 promotes the recruitment of Treg cell and M2 macrophage in the TME (43), and cancer cells could upregulate the expression of CD47 in the cell membrane via the IL1-RAP dependent pathway to avoid macrophage phagocytosis (29). Thus, we speculate that CAFs contribute to immune evasion and unfavorable prognosis of GC patients by creating an immunosuppressive microenvironment, which is partially dependent on these CAF-associated genes.
Although CAFGS could act as an effective predictive tool to evaluate the prognosis of GC patients, some questions remain. Firstly, the expression pattern and biological function of these CAF genes in the TME is still unclear, as is whether these genes are ubiquitously expressed in all types of cells in the TME or specifically expressed in CAFs. Also, are CAFs in different tumor types different? The question of how these genes regulate the biological function of CAFs is also unanswered. Understanding the underlying molecular and cellular mechanisms governing the dynamic interactions of cancer cells with their microenvironment can be used as a novel strategy to disrupt cancer cell interplay and contribute to the development of efficient and safe therapeutic strategies to fight cancer. Secondly, while we showed that high CAFGS score group had higher expression level of immune checkpoint genes, such as TIM-3, CD276, and PD-L2, the classical immune checkpoint molecules, such as CTLA4, PD-1, and PD-L1, had no significant difference between high-risk and low-risk group. The underlying mechanism should be explored further. In addition, the relationship between 10 CAF genes and immune checkpoint molecules should be explored at the protein level. Finally, our study is a bioinformatic analysis based on three public datasets and one qPCR validation cohort. The real prognostic values of the CAFGS should be validated prospectively in independent and multicenter GC cohorts with larger sample sizes in the future.
Conclusions
In conclusion, we used CAF-derived genes to establish a CAF gene signature, which shows great performance in the prognosis estimation of GC patients. CAF associated genes identified in this study could provide valuable insight and a useful resource for studying the role of CAFs and targeting therapy during cancer progression in GC.
Acknowledgments
The authors appreciate the academic support from the AME Gastric Cancer Collaborative Group.
Funding: None.
Footnote
Reporting Checklist: The authors have completed the TRIPOD reporting checklist. Available at https://atm.amegroups.com/article/view/10.21037/atm-22-2810/rc
Data Sharing Statement: Available at https://atm.amegroups.com/article/view/10.21037/atm-22-2810/dss
Conflicts of Interest: All authors have completed the ICMJE uniform disclosure form (available at https://atm.amegroups.com/article/view/10.21037/atm-22-2810/coif). The authors have no conflicts of interest to declare.
Ethical Statement: The authors are accountable for all aspects of the work in ensuring that questions related to the accuracy or integrity of any part of the work are appropriately investigated and resolved. This study was conducted in accordance with the Declaration of Helsinki (as revised in 2013) and was approved by the Ethical Committee of Xijing Hospital (No. KY20192088-F-1). Written informed consent was taken from all the patients.
Open Access Statement: This is an Open Access article distributed in accordance with the Creative Commons Attribution-NonCommercial-NoDerivs 4.0 International License (CC BY-NC-ND 4.0), which permits the non-commercial replication and distribution of the article with the strict proviso that no changes or edits are made and the original work is properly cited (including links to both the formal publication through the relevant DOI and the license). See: https://creativecommons.org/licenses/by-nc-nd/4.0/.
References
- Sung H, Ferlay J, Siegel RL, et al. Global Cancer Statistics 2020: GLOBOCAN Estimates of Incidence and Mortality Worldwide for 36 Cancers in 185 Countries. CA Cancer J Clin 2021;71:209-49. [Crossref] [PubMed]
- Junttila MR, de Sauvage FJ. Influence of tumour micro-environment heterogeneity on therapeutic response. Nature 2013;501:346-54. [Crossref] [PubMed]
- Chen X, Song E. Turning foes to friends: targeting cancer-associated fibroblasts. Nat Rev Drug Discov 2019;18:99-115. [Crossref] [PubMed]
- Desbois M, Wang Y. Cancer-associated fibroblasts: Key players in shaping the tumor immune microenvironment. Immunol Rev 2021;302:241-58. [Crossref] [PubMed]
- Herrera M, Berral-González A, López-Cade I, et al. Cancer-associated fibroblast-derived gene signatures determine prognosis in colon cancer patients. Mol Cancer 2021;20:73. [Crossref] [PubMed]
- Gao J, Kwan PW, Shi D. Sparse kernel learning with LASSO and Bayesian inference algorithm. Neural Netw 2010;23:257-64. [Crossref] [PubMed]
- Racle J, de Jonge K, Baumgaertner P, et al. Simultaneous enumeration of cancer and immune cell types from bulk tumor gene expression data. Elife 2017;6:26476. [Crossref] [PubMed]
- Aran D, Hu Z, Butte AJ. xCell: digitally portraying the tissue cellular heterogeneity landscape. Genome Biol 2017;18:220. [Crossref] [PubMed]
- Becht E, Giraldo NA, Lacroix L, et al. Estimating the population abundance of tissue-infiltrating immune and stromal cell populations using gene expression. Genome Biol 2016;17:218. [Crossref] [PubMed]
- Newman AM, Liu CL, Green MR, et al. Robust enumeration of cell subsets from tissue expression profiles. Nat Methods 2015;12:453-7. [Crossref] [PubMed]
- Li T, Fan J, Wang B, et al. TIMER: A Web Server for Comprehensive Analysis of Tumor-Infiltrating Immune Cells. Cancer Res 2017;77:e108-10. [Crossref] [PubMed]
- Yoshihara K, Shahmoradgoli M, Martínez E, et al. Inferring tumour purity and stromal and immune cell admixture from expression data. Nat Commun 2013;4:2612. [Crossref] [PubMed]
- Jiang P, Gu S, Pan D, et al. Signatures of T cell dysfunction and exclusion predict cancer immunotherapy response. Nat Med 2018;24:1550-8. [Crossref] [PubMed]
- Shum B, Larkin J, Turajlic S. Predictive biomarkers for response to immune checkpoint inhibition. Semin Cancer Biol 2022;79:4-17. [Crossref] [PubMed]
- Mhaidly R, Mechta-Grigoriou F. Role of cancer-associated fibroblast subpopulations in immune infiltration, as a new means of treatment in cancer. Immunol Rev 2021;302:259-72. [Crossref] [PubMed]
- Felding-Habermann B, Cheresh DA. Vitronectin and its receptors. Curr Opin Cell Biol 1993;5:864-8. [Crossref] [PubMed]
- Chao C, Carmical JR, Ives KL, et al. CD133+ colon cancer cells are more interactive with the tumor microenvironment than CD133- cells. Lab Invest 2012;92:420-36. [Crossref] [PubMed]
- Kadowaki M, Sangai T, Nagashima T, et al. Identification of vitronectin as a novel serum marker for early breast cancer detection using a new proteomic approach. J Cancer Res Clin Oncol 2011;137:1105-15. [Crossref] [PubMed]
- Ortega-Martínez I, Gardeazabal J, Erramuzpe A, et al. Vitronectin and dermcidin serum levels predict the metastatic progression of AJCC I-II early-stage melanoma. Int J Cancer 2016;139:1598-607. [Crossref] [PubMed]
- Niu Y, Zhang L, Bi X, et al. Evaluation of Vitronectin Expression in Prostate Cancer and the Clinical Significance of the Association of Vitronectin Expression with Prostate Specific Antigen in Detecting Prostate Cancer. Urol J 2016;13:2527-32. [PubMed]
- Yang XP, Zhou LX, Yang QJ, et al. Diagnostic and prognostic roles of serum vitronectin in hepatitis B-related hepatocellular carcinoma. Cancer Biomark 2016;17:271-9. [Crossref] [PubMed]
- Chen JW, Luo YJ, Yang ZF, et al. Knockdown of angiopoietin-like 4 inhibits the development of human gastric cancer. Oncol Rep 2018;39:1739-46. [Crossref] [PubMed]
- Avalle L, Raggi L, Monteleone E, et al. STAT3 induces breast cancer growth via ANGPTL4, MMP13 and STC1 secretion by cancer associated fibroblasts. Oncogene 2022;41:1456-67. [Crossref] [PubMed]
- Wang FT, Li XP, Pan MS, et al. Identification of the prognostic value of elevated ANGPTL4 expression in gallbladder cancer-associated fibroblasts. Cancer Med 2021;10:6035-47. [Crossref] [PubMed]
- Smith DE, Hanna R. The soluble form of IL-1 receptor accessory protein enhances the ability of soluble type II IL-1 receptor to inhibit IL-1 action. Immunity 2003;18:87-96. [Crossref] [PubMed]
- Lv Q, Xia Q, Li A, et al. The Potential Role of IL1RAP on Tumor Microenvironment-Related Inflammatory Factors in Stomach Adenocarcinoma. Technol Cancer Res Treat 2021;20:1533033821995282. [Crossref] [PubMed]
- Nishida A, Andoh A, Imaeda H, et al. Expression of interleukin 1-like cytokine interleukin 33 and its receptor complex (ST2L and IL1RAcP) in human pancreatic myofibroblasts. Gut 2010;59:531-41. [Crossref] [PubMed]
- Ali S, Huber M, Kollewe C, et al. IL-1 receptor accessory protein is essential for IL-33-induced activation of T lymphocytes and mast cells. Proc Natl Acad Sci U S A 2007;104:18660-5. [Crossref] [PubMed]
- Liu F, Dai M, Xu Q, et al. SRSF10-mediated IL1RAP alternative splicing regulates cervical cancer oncogenesis via mIL1RAP-NF-κB-CD47 axis. Oncogene 2018;37:2394-409. [Crossref] [PubMed]
- Cheng A, Zhao S, FitzGerald LM, et al. A four-gene transcript score to predict metastatic-lethal progression in men treated for localized prostate cancer: Development and validation studies. Prostate 2019;79:1589-96. [Crossref] [PubMed]
- Liu X, Wu J, Zhang D, et al. Identification of Potential Key Genes Associated With the Pathogenesis and Prognosis of Gastric Cancer Based on Integrated Bioinformatics Analysis. Front Genet 2018;9:265. [Crossref] [PubMed]
- Zhang WP, Wang Y, Tan D, et al. Cystatin 2 leads to a worse prognosis in patients with gastric cancer. J Biol Regul Homeost Agents 2020;34:2059-67. [PubMed]
- Huang Z, He A, Wang J, et al. The circadian clock is associated with prognosis and immune infiltration in stomach adenocarcinoma. Aging (Albany NY) 2021;13:16637-55. [Crossref] [PubMed]
- Liu J, Liu Z, Zhang X, et al. Bioinformatic exploration of OLFML2B overexpression in gastric cancer base on multiple analyzing tools. BMC Cancer 2019;19:227. Erratum in: BMC Cancer 2019;19:759. [Crossref] [PubMed]
- Wu F, Yang J, Liu J, et al. Signaling pathways in cancer-associated fibroblasts and targeted therapy for cancer. Signal Transduct Target Ther 2021;6:218. [Crossref] [PubMed]
- Harper J, Sainson RC. Regulation of the anti-tumour immune response by cancer-associated fibroblasts. Semin Cancer Biol 2014;25:69-77. [Crossref] [PubMed]
- De Palma M, Lewis CE. Macrophage regulation of tumor responses to anticancer therapies. Cancer Cell 2013;23:277-86. [Crossref] [PubMed]
- Yamaguchi T, Fushida S, Yamamoto Y, et al. Tumor-associated macrophages of the M2 phenotype contribute to progression in gastric cancer with peritoneal dissemination. Gastric Cancer 2016;19:1052-65. [Crossref] [PubMed]
- Ziani L, Chouaib S, Thiery J. Alteration of the Antitumor Immune Response by Cancer-Associated Fibroblasts. Front Immunol 2018;9:414. [Crossref] [PubMed]
- Cassetta L, Pollard JW. Targeting macrophages: therapeutic approaches in cancer. Nat Rev Drug Discov 2018;17:887-904. [Crossref] [PubMed]
- Braga TT, Agudelo JS, Camara NO. Macrophages During the Fibrotic Process: M2 as Friend and Foe. Front Immunol 2015;6:602. [Crossref] [PubMed]
- Kalluri R. The biology and function of fibroblasts in cancer. Nat Rev Cancer 2016;16:582-98. [Crossref] [PubMed]
- Zhou S, Tu J, Ding S, et al. High Expression of Angiopoietin-like Protein 4 in Advanced Colorectal Cancer and its Association with Regulatory T Cells and M2 Macrophages. Pathol Oncol Res 2020;26:1269-78. [Crossref] [PubMed]
(English Language Editor: B. Draper)