Development of the prediction model based on clinical-imaging omics: molecular typing and sentinel lymph node metastasis of breast cancer
Introduction
Breast cancer has the highest mortality and morbidity of all tumors and cancers among women (1). Breast cancer can be divided into 4 molecular types based on gene expression profiles, and biomarkers, and the differences between the molecular types results in different clinical treatment plans and prognoses (2). Clinically, tumor molecular typing is mainly based on immunohistochemical results. If the molecular typing of tumors can be accurately predicted using non-invasive procedures at an early stage of the disease, it would be of great help in late clinical treatment. If axillary lymph node metastasis occurs in breast cancer patients, the survival rate is significantly reduced (3).
Sentinel lymph node (SLN) is the vanguard of axillary lymph node metastasis; however, SLN biopsy, which is commonly used in clinical settings, is not suitable for all patients (4). Gillies first proposed the concept of using image omics to extract features via high-throughput radiographic images and to establish a database to provide scientific guidance for individual disease diagnosis and treatment (5). Imaging omics is a common non-invasive method used to predict tumor classification and prognosis in clinical practice (6-8). The application of imaging in clinical medicine has been paid more and more attention. Breast cancer, as a hot spot of imaging research, has carried out many scientific experiments and clinical studies, among which a breakthrough has been made in molecular typing of breast cancer. Magnetic resonance imaging (MRI) is usually used for breast examination. Multi-parameter MRI with different functions can visualize and quantify the functional changes of cancer development, provide specific information about cancer markers, and intuitively observe the general features of the lesions and the surrounding tissues. Imaging features based on the growth microenvironment of the tumor may be the key biomarker to distinguish between benign and malignant tumors, and features extracted from the surrounding areas of the tumor can be used to distinguish triple-negative breast cancer from other molecular subtypes. At present, the research on the imaging features of dynamic contrast-enhanced (DCE) and diffusion-weighted imaging (DWI) to predict the molecular typing value has achieved results, but the imaging model based on DWI and DCE-MRI is not enough to predict the molecular subtypes, and the prediction value of the prediction model based on the three-dimensional volume of interest of the lesion to the four molecular subtypes of breast cancer has not been fully studied. In this paper, features are extracted from multi-parameter MRI images, and a prediction model of molecular typing and SLN metastasis of breast cancer is established. Suggestions are made for noninvasive prediction of different molecular typing and SLN metastasis of breast cancer, and additional value of treatment plan selection and prognosis evaluation is provided without increasing the economic burden of patients. We present the following article in accordance with the STARD reporting checklist (available at https://atm.amegroups.com/article/view/10.21037/atm-22-2844/rc).
Methods
Clinical data
The clinical data of 202 patients diagnosed with breast cancer at the Department of General Surgery, The First Medical Center, Chinese PLA General Hospital, from October 2020 to November 2021 who underwent preoperative multi-parameter MRI examinations [i.e., T2-weighted imaging (T2WI), T1-weighted imaging + C (T1WI+C), and diffusion-weighted imaging (DWI)] were retrospectively analyzed. The patients were divided into the following 2 groups according to the time of MRI examination: (I) the development group (n=110 cases); and (II) the validation group (n=92 cases). There were no significant differences in the basic clinical information and pathological tissue information between the 2 groups (P>0.05), which indicated that the study was feasible. The study was conducted in accordance with the Declaration of Helsinki (as revised in 2013). The study was approved by the Ethics Committee of the Chinese PLA General Hospital (No. 2021-692-01) and individual consent for this retrospective analysis was waived.
Patients were included in the study if they met the following inclusion criteria: (I) had postoperative pathological examination confirmed breast cancer with unilateral breast cancer and no distant metastasis; (II) had undergone a SLN biopsy, and had complete biopsy results; (III) had undergone multi-parameter (T2WI, T1WI+C, or DWI) MRI examinations before surgery for which the images were clear; and (IV) had complete clinical data.
Patients were excluded from the study if they met any of the following exclusion criteria: (I) had incomplete MRI items or unclear images; (II) had undergone chemotherapy, radiotherapy, or other treatments before MRI examination; (III) had undergone a biopsy before MRI; and/or (IV) had undergone a MRI examination after surgery.
MRI examinations
Equipment
MRI examinations were performed in both groups using the same 3.0T GE Discovery MR 750W breast MRI machine.
MRI examination method
Each patient was placed in the supine position with their body parallel to the scanning bed. The patient’s arms were raised above their head with both breasts hanging naturally in the coil. The head was first entered into the machine, and the following parameters were used for scanning after conventional positioning scanning:
- T2WI sequence: echo time (TE): 70 ms, repeat time (TR): 4,000 ms, thickness: 4 mm, layer interval: 6 mm, and field of vision (FOV): 340×340 mm.
- DWI sequence: TR: 6,000 ms, layer thickness: 4 mm, TE: 84 ms, FOV: 320×320 mm, layer interval: 5 mm, matrix: 902×220, diffusion sensitivity coefficient B: 0, 800 s/mm2.
- Axial contrast enhancement T1 weighting (T1WI+C): 0.3 mL/kg of gadolinium diethyl triamine pentaacetic was injected into the cubital vein at a rate of 2.8 mL/s, followed by an injection of normal saline in the same amount. The single scanning time was 16 s, and a total of 30 scanning stages were performed. TR/TE: 5.2/2.3 ms, matrix: 300×320 mm, field of vision: 300×320 mm, voxel: 1.00×1.00×1.00, layer thickness: 2 mm, reverse angle: 15°.
Outlining the ROI
Multi-parameter MRI (T1WI+C, T2WI, and DWI) images were used to segment the lesions of the original breast cancer images collected. Region of interest (ROI) mapping was performed on a 3-dimensional (3D) Slicer (V4.10.2; https://www.slicer.org/), which is capable of semi-automatic segmentation. The ROI was manually mapped by 2 radiologists with >8 years of experience in clinical breast MRI. It was not necessary to avoid the sites of cystic lesions and necrosis, but all the tumor structures and tissues were manually delineated as much as possible. Neither of the radiologists who delineated them were aware of the pathologic findings of any of the patients.
Feature extraction and dimension reduction
Feature extraction
There are 14 gray-level run-matrix features, 17 first-order statistical features, 22 gray-level co-occurrence matrix (GLCM) features, 8 shape features, 5 neighborhood gray-level difference matrix features, and 13 gray-level regional matrix features. Then 3D Coiflet wavelet transform was used to decompose each original image into 8 new images with different signal frequency scales. The 2 images before and after the conversion were the same size. The 3D feature was re-calculated for the transformed image.
Feature extraction was performed using AMni-Kinetics software. The ROIs, which had been delineated from the T2WI fat compression, T1WI+C, and DWI images, were imported into the software. The step sizes ranged from 1–9, and automatic texture feature extraction was performed using the software. The following texture features were selected:
- First-order features, such as energy, entropy, interquartile range, kurtosis, maximum, mean absolute deviation, mean, median, minimum, range, robust mean absolute deviation, root mean squared, skewness, total energy, and uniformity, variance;
- Shape, which describes the size and shape of the ROI, including elongation, flatness, least axis length, major axis length, maximum 2-dimensional (2D) diameter column, maximum 2D diameter row, maximum 2D diameter slice, maximum 3D diameter, mesh volume, minor axis length, sphericity surface area, surface volume ratio, and voxel volume;
- Texture features, including: (I) the gray co-occurrence matrix; (II) the characteristics of homogeneous regions, including short region emphasis and long region emphasis based on the gray-scale connected region matrix; (III) the gray length matrix; (IV) 5 neighborhood gray color difference matrices, which were used to describe the differences between each voxel and roughness (measured edge density), contrast (any local changes in the image), and adjacent voxel busyness (measured spatial speed of gray value change; and (V) the filter characteristics.
Characteristics of tumor and peritumoral radiation
The development group comprised 110 targeted lesions and the validation group comprised 92 targeted lesions. First, in all the extracted features inside delete a missing value loss, ensure the quality of characteristics, the remaining features of standardized treatment (Z score). Finally, least absolute shrinkage and selection operator (LASSO) algorithm omics feature dimension reduction was used to get the final image based on the important feature of the ROI peritumoral and tumor. A total of 20 features were extracted from the T2WI, T1+C, and DWI images, including the maximum 2D diameter slice, wavelet-HLL GLCM Maximal Correlation Coefficient (MCC), long run low gray-level emphasis, skewness, wavelet-lhh GLCM MCC, Inverse Difference Moment Normalized (IDMN), large area high gray-level emphasis, median, small area emphasis, mean, cluster prominence, HHL GLCM cluster shade, large area low gray-level emphasis, contrast, wavelet-lll GLCM cluster shade, difference entropy, Haralick correlation quantile 25, wavelet-upp, sphericity, and maximum 3D diameter.
Analysis of pathological results
Pathology and immunohistochemistry
The pathologic diagnosis of all the patients was breast cancer. The selected pathological specimens had a thickness of 4 um and were stained using the Streptavidin Peroxidase Con-jugated (SP) method to detect the expressions of biological markers progesterone receptor (PR), recombinant Ki-67 protein (Ki-67), human epidermal growth factor receptor-2 (HER-2) and estrogen receptor (ER).
Analysis of pathological results
In relation to the diagnosis of HER-2, it should be noted that HER-2+ is an uncertain condition, and the status of HER-2 gene amplification needs to be tested by fluorescence immunohybridization. When the gene is amplified, it is positive, and when the gene is not amplified, it is negative. Specifically, 0 and 1+ are negative, while 3 plus is positive.
The diagnostic criteria for ER and PR were as follows: >1% indicates a positive result.
For Ki-67, a cut-off value of 14% was used to judge high and low, and the threshold value for PR expression was 20%.
Molecular typing and pathological grading of breast cancer
Breast cancer was divided into the following 4 molecular subtypes based on the immunohistochemical Ki-67, ER, HER-2, and PR results (9):
- Luminal A type: both PR and ER were positive with a high expression of PR (>20%), a low expression of Ki-67 (<14%), and negative HER-2;
- Luminal B (HER-2+) type: positive PR or ER, positive PR and ER, positive HER-2, and Ki-67positive or negative; or luminal B (HER-2–) type: positive for PR or ER or positive for PR and ER, with a low expression of Ki-67 (<14%), and negative for HER-2;
- HER-2 overexpression type: ER and PR negative, HER-2 positive, and Ki-67 positive or negative;
- Triple negative (TN) type: HER-2, PR, and ER negative, and Ki-67 positive or negative.
The semi-quantitative analysis method of Ellis and Elston was used to grade the pathology, and there were 3 grades; that is, Grade I, Grade II, and Grade III.
Statistical method
SPSS25.0 software was used to conduct a statistical analysis of the general characteristics between the 2 groups of breast cancer patients using a t-test or an analysis of variance. Intra-group correlation coefficient (ICC) was used to evaluate the consistency of the two physicians, and ICC >0.75 was considered as a good reliability. The receiver operating characteristic (ROC) curve was used to evaluate the predictive efficacy of radiation characteristics based on T1+C, T2WI and DWI images for molecular typing of breast cancer and SLN metastasis. In the training set, the generalized linear regression model (Logistic regression model) of imageology and clinical factors is constructed by using the features selected by LASSO and the meaningful clinical factors analyzed by univariate Logistic regression, and the joint prediction model is established by further screening variables. ROC was used to evaluate the discrimination and calibration of each model, and P>0.05 indicated that the fitting effect was better. The goodness-of-fit of the model was demonstrated by the calibration curve, and the area under the curve (AUC), diagnostic accuracy, sensitivity, specificity, positive predictive value (PPV) and negative predictive value (NPV) were calculated. The results of 10 stratified random sampling were averaged. The smaller the difference between AUC and 1, the higher the diagnostic efficiency. The diagnostic efficiency was considered significant when the AUC was >0.9, moderate when the AUC ranged from 0.7–0.9 and low when the AUC ranged from 0.5–0.7. A LASSO logistic regression analysis was performed using R statistical software. The ROC curves were drawn using the “pROC” package.
Results
ROI sketch result
It is generally believed that the main part of the tumor is the ROI of the tumor, while the ROI around the tumor describes the tissue around the tumor (the edge of expansion). In this study, the tumor and the tissue around the tumor were reconstructed with an expansion algorithm of 2 mm each. If the longest distance from the breast surface to the tumor surface is less than the expansion distance, the region with the longest distance from the superficial lesions is considered the ROI around the tumor (10). According to the performance of the validation group and the validation group, the ROI around the tumor (extended edge) was further plotted according to the expansion distance in the model with the best performance. Finally, the tumor ROI (main part) was drawn (see Figure 1).
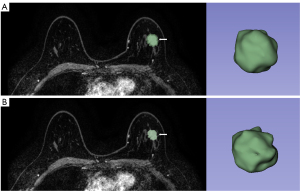
Demographic analysis
The data of 202 breast cancer patients admitted to our Department of General Surgery from October 2020 to November 2021 were collected. Among the 202 patients, 63 had luminal A type, 28 had triple negative (TN) type, 32 had HER-2 overexpression type, 79 had luminal B type, 103 had SLN (+), and 109 had SLN (−). The patients in the development group (n=140) had a mean age of 55.73±8.6 years, and included 36 premenopausal patients and 75 SLN positive patients. The patients in the validation group (n=52) had a mean age of 53.64±8.9 years and included 23 premenopausal patients and 28 SLN positive patients. A comparison of the 2 groups of breast cancer patients revealed that there were no statistically significant differences in terms of the general information of patients, including age and menopausal status (P>0.05).
Consistency evaluation of imaging features
ICC: the intra-observer results were 0.781–0.879, and the inter-observer results were 0.779–0.889. The ICC results were higher than 0.75, which indicated high consistency.
Radiological analysis
In this study, a total of 851 imaging omics features were extracted, and different models were established according to the corresponding features. The ROC curves were plotted using LASSO for AUC comparisons. The results showed that the constructed imaging features had the best predictive performance when the tumor + peritumoral 2 mm was used (see Table 1 and Figure 2).
Table 1
Variables | Group | Image model group | AUC (95% confidence interval) | Sensitivity | Specificity | Accuracy |
---|---|---|---|---|---|---|
Tumor | Development group | Luminal type A | 0.801 | 0.765 | 0.774 | 0.772 |
Luminal type B | 0.739 | 0.727 | 0.708 | 0.713 | ||
HER-2 overexpression | 0.724 | 0.704 | 0.618 | 0.656 | ||
TN type | 0.745 | 0.765 | 0.629 | 0.648 | ||
SLN | 0.734 | 0.715 | 0.666 | 0.713 | ||
Validation group | Luminal type A | 0.740 | 0.833 | 0.639 | 0.667 | |
Luminal type B | 0.808 | 0.735 | 0.539 | 0.631 | ||
HER-2 overexpression | 0.655 | 0.556 | 0.706 | 0.639 | ||
TN type | 0.703 | 0.765 | 0.581 | 0.607 | ||
SLN | 0.708 | 0.794 | 0.285 | 0.707 | ||
Tumor + peritumoral (2 mm) | Development group | Luminal type A | 0.810 | 0.848 | 0.640 | 0.697 |
Luminal type B | 0.808 | 0.835 | 0.539 | 0.631 | ||
HER-2 overexpression | 0.900 | 0.734 | 0.732 | 0.816 | ||
TN type | 0.801 | 0.772 | 0.765 | 0.774 | ||
SLN | 0.838 | 0.819 | 0.714 | 0.795 | ||
Validation group | Luminal type A | 0.831 | 0.856 | 0.891 | 0.819 | |
Luminal type B | 0.832 | 0.660 | 0.877 | 0.776 | ||
HER-2 overexpression | 0.801 | 0.772 | 0.765 | 0.774 | ||
TN type | 0.904 | 0.769 | 0.873 | 0.822 | ||
SLN | 0.819 | 0.810 | 0.500 | 0.780 |
AUC, area under the receiver operator characteristic curve; HER-2, human epidermal growth factor receptor 2; TN, triple negative; SLN, sentinel lymph node.
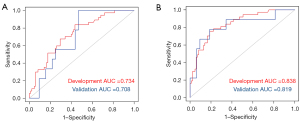
Discussion
The incidence of breast cancer has been increasing in recent years. However, under the current guidelines, treatment can only reduce mortality and improve survival rates. The clinical characteristics and tumor types of each patient differ. If all tumors are simply treated, the treatment effect of some patients will be poor, and even excessive treatment of some patients. Clinically, to maximize the treatment effect, targeted treatment measures should be adopted according to individual differences to provide precise treatment and unnecessary treatment should be avoided.
Breast cancer is a heterogeneous disease with extensive clinical and pathological features. Common pathological information includes histological features, Ki-67 expression level, tumor size, lymph node metastasis, and other indicators. Such pathological information can be used to predict the prognosis of patients. Indeed, lymph node metastasis and histological grade are very important prognostic indicators for breast cancer patients. Thus, extending our understandings of these indicators will have great significance for the treatment plans and prognosis assessments of patients.
Determining the molecular classification of breast cancer is very important to determine treatment plans and predict the prognosis of patients. The use of immunohistochemical methods to determine molecular typing has many disadvantages, including that it is a lengthy process and damages the patient’s body (11). Image omics, which is a new technology in the field of medical image analysis, can improve the accuracy of disease diagnosis and prediction by extracting and screening information features related to the microscopic structure and biological characteristics of tumor tissues (12,13). At present, many scholars have used radiometrics to perform molecular typing, efficacy evaluations, non-invasive identification, histological grading, prognosis evaluations, and predict the metastasis of benign and malignant breast tumors.
SLN status is a major factor affecting the prognosis of breast cancer patients, and the evaluation of SNL status is very important in predicting the prognosis of patients. Currently, biopsy and axillary lymph node dissection are commonly used to determine axillary lymph node metastasis in breast cancer patients. However, this method may lead to excessive treatments in some patients and result in unnecessary injuries; thus, the non-invasive prediction of SLN metastasis is very necessary.
This study sought to predict the molecular typing and SLN metastasis of breast cancer based on the tumor + peritumoral application of combined fat suppression on T2WI, T1WI+C, and DWI combined sequence imaging features. For the clinical selection of breast cancer treatments and prognosis evaluation of patients to put forward suggestions.
Mammography, ultrasound, and MRI are currently available for breast cancer patients. Among these, MRI is very important in the diagnosis of breast cancer. MRI has many advantages, including that it is non-invasive, does not involve exposure to radiation, has high resolution, and can intuitively display the morphological and functional information of breast cancer, which will increase the accuracy of breast cancer diagnosis (14-16). Numerous studies (17-20) have found that imaging groups can be used to predict the diagnosis, type, and lymph node metastasis of breast cancer. Breast MRI (21,22) has achieved satisfactory clinical results in this area. Currently, DCE-MRI, T1WI+C, T2WI sequence and diffusion addition imaging are commonly used in clinical breast MRI examinations, and the latter 3 were mainly used in this study.
In clinical practice, breast MRI has been simplified in recent years, such that only T1WI (with or without enhancement) is used. Kuhl and Baltzer (23) and Petrillo et al. (24) both showed that the application of T1+C imaging alone has significance in the diagnosis of breast cancer.
In T2WI, images are obtained after a long repetition time and a long echo scan, which is mainly composed of images of intracellular, extracellular and extravascular space, and clearly show the morphology of the tumor (25). T2WI images are helpful for the diagnosis of breast cancer and molecular typing.
DWI quantifies and visualizes the Brownian motion of water molecules in human tissues by calculating the apparent diffusion coefficient (ADC), and side evaluates the microstructure of tissues (26). In biological tissue, the integrity of the cell membranes and the cell density of tissues are negatively correlated with the degree of water diffusion. As breast tumors have intact cell membranes and high cell density, the movement of water molecules is restricted; thus, the tumors present high signals in DWI images and low signals in ADC images (27,28). The changes of vascular distribution and lesion size were later than the changes of ADC value, and useful information about early breast cancer can be obtained from ADC images.
Clinical-imaging omics refers to the application of medical images for feature extraction and screening, and the acquisition of key information via diversified data processing. It is a multistep and extremely complex process. Its main steps include obtaining high-quality images, segmenting the images, extracting and quantifying the ROI features, screening the key features, constructing prediction models, verifying effectiveness, and finally clinical application (29). In recent years, some scholars have contended that the imaging omics method used in breast cancer could be used to conduct a comprehensive quantitative analysis of tumors, check the growth status of tumors in different periods, and monitor the metastasis and related characteristics of tumors (30,31). Further, imaging features and clinical information could be integrated to improve the clinical diagnosis, treatment efficacy, and prognosis prediction of breast cancer, and thus to improve the treatment effect (32). Most scholars only study the correlation of single parameter MRI. Different parameters have their own limitations and advantages. The combined detection of multi-parameter images can further improve the accuracy of breast cancer diagnosis through the similarity and specificity of images with different parameters. Research should seek to improve the predictive power of molecular typing of breast cancer by using 2 kinds of MRI. The combination of multi-parameter MRI imaging has gradually become a research trend in predicting the pathological information of breast cancer.
This study used different parameters of image information between complementary relationship, joint multi-parameter magnetic resonance images (i.e., T1WI+C, T2WI, and DWI) characteristics, molecular classification of breast cancer, were used to predict the SLN metastasis and improve the accuracy of the single parameter imaging features of pathological information forecasting and provide the basis for early treatment of breast cancer and prognosis evaluation. The results of this study showed that when comparing the consistency between observation groups based on the characteristics of breast multi-parameter MRI in breast cancer patients with different molecular types and SLN metastasis, the ICC of the 2 observers was 0.781–0.879, and the ICC between the 2 observers was >0.75, indicating high consistency.
In addition, among the models for identifying luminal A, luminal B, TN, HER2 overexpression, and SLN based on multi-parameter imaging features of tumor and tumor + intratumoral, the AUC (sensitivity, specificity) of the validation group were 0.831 (0.856, 0.891), 0.832 (0.660, 0.877), 0.801 (0.772, 0.765), 0.904 (0.769, 0.873), and 0.819 (0.810, 0.500) respectively when the tumor was 2 mm around the tumor. In conclusion, the imaging features of multi-parameter breast MRI extraction based on tumor + intratumoral 2 mm have certain value in differentiating between different molecular types of breast cancer and SLN metastasis, which is expected to make the non-invasive prediction of molecular type and SLN metastasis of breast cancer at an early stage and to better guide clinical decision making. Zhong et al. (33) extracted the characteristics of ADC images and DCE-MRI images of patients, and found that imaging features based on DCE-MRI and DWI were conducive to the identification of molecular subtypes of breast cancer, and had great significance and clinical value in the identification of TN breast cancer. The AUCs of the best model for the differential diagnosis between luminal B and non-luminal B, luminal A and non-luminal A, basal-like type, and non-basal-like type were 0.786, 0.733, and 0.941, respectively, which was consistent with previous study (34).
In conclusion, the imaging features of multi-parameter breast MRI extraction based on tumor + intratumoral 2 mm have certain value in differentiating between different molecular types of breast cancer and SLN metastasis, and can be used for the non-invasive prediction of molecular type and SLN metastasis of breast cancer in the early stage of the disease and to better guide clinical decision making.
Acknowledgments
Funding: None.
Footnote
Reporting Checklist: The authors have completed the STARD reporting checklist. Available at https://atm.amegroups.com/article/view/10.21037/atm-22-2844/rc
Data Sharing Statement: Available at https://atm.amegroups.com/article/view/10.21037/atm-22-2844/dss
Conflicts of Interest: All authors have completed the ICMJE uniform disclosure form (available at https://atm.amegroups.com/article/view/10.21037/atm-22-2844/coif). The authors have no conflicts of interest to declare.
Ethical Statement: The authors are accountable for all aspects of the work in ensuring that questions related to the accuracy or integrity of any part of the work are appropriately investigated and resolved. The study was conducted in accordance with the Declaration of Helsinki (as revised in 2013). The study was approved by the Ethics Committee of the Chinese PLA General Hospital (No. 2021-692-01) and individual consent for this retrospective analysis was waived.
Open Access Statement: This is an Open Access article distributed in accordance with the Creative Commons Attribution-NonCommercial-NoDerivs 4.0 International License (CC BY-NC-ND 4.0), which permits the non-commercial replication and distribution of the article with the strict proviso that no changes or edits are made and the original work is properly cited (including links to both the formal publication through the relevant DOI and the license). See: https://creativecommons.org/licenses/by-nc-nd/4.0/.
References
- Pekmezi D, Fontaine K, Rogers LQ, et al. Adapting MultiPLe behavior Interventions that eFfectively Improve (AMPLIFI) cancer survivor health: program project protocols for remote lifestyle intervention and assessment in 3 inter-related randomized controlled trials among survivors of obesity-related cancers. BMC Cancer 2022;22:471. [Crossref] [PubMed]
- Luo C, Lu L, Zhang W, et al. The Value of Shear Wave Elastography in the Diagnosis of Breast Cancer Axillary Lymph Node Metastasis and Its Correlation With Molecular Classification of Breast Masses. Front Oncol 2022;12:846568. [Crossref] [PubMed]
- Serrano Del Moral Á, Pérez Viejo E, Castaño Pascual Á, et al. Usefulness of histological superstudy of sentinel lymph nodes detected with radioisotopes in colon cancer. Rev Esp Med Nucl Imagen Mol 2021;40:358-66. (Engl Ed). [Crossref] [PubMed]
- Omilian AR, Sheng H, Hong CC, et al. Multiplexed digital spatial profiling of invasive breast tumors from Black and White women. Mol Oncol 2022;16:54-68. [Crossref] [PubMed]
- Li Z, Zhong Q, Zhang L, et al. Computed Tomography-Based Radiomics Model to Preoperatively Predict Microsatellite Instability Status in Colorectal Cancer: A Multicenter Study. Front Oncol 2021;11:666786. [Crossref] [PubMed]
- Gilard V, Derrey S, Marret S, et al. Precision Neurosurgery: A Path Forward. J Pers Med 2021;11:1019. [Crossref] [PubMed]
- Xie Y, Zhao H, Guo Y, et al. A PET/CT nomogram incorporating SUVmax and CT radiomics for preoperative nodal staging in non-small cell lung cancer. Eur Radiol 2021;31:6030-8. [Crossref] [PubMed]
- Ab Mumin N, Ramli Hamid MT, Wong JHD, et al. Magnetic Resonance Imaging Phenotypes of Breast Cancer Molecular Subtypes: A Systematic Review. Acad Radiol 2022;29:S89-S106. [Crossref] [PubMed]
- Chronaiou I, Giskeødegård GF, Goa PE, et al. Feasibility of contrast-enhanced MRI derived textural features to predict overall survival in locally advanced breast cancer. Acta Radiol 2020;61:875-84. [Crossref] [PubMed]
- Chen M, Cao J, Hu J, et al. Clinical-Radiomic Analysis for Pretreatment Prediction of Objective Response to First Transarterial Chemoembolization in Hepatocellular Carcinoma. Liver Cancer 2021;10:38-51. [Crossref] [PubMed]
- Zhang G, Ren C, Li C, et al. Distinct clinical and somatic mutational features of breast tumors with high-, low-, or non-expressing human epidermal growth factor receptor 2 status. BMC Med 2022;20:142. [Crossref] [PubMed]
- Huang T, Shan R, Zhang M, et al. Steroidogenic acute regulatory protein-related lipid transfer 4 (StarD4) promotes breast cancer cell proliferation and its mechanism. Sheng Wu Yi Xue Gong Cheng Xue Za Zhi 2021;38:1118-25. [PubMed]
- Liu G, Li Y, Chen SL, et al. Non-mass enhancement breast lesions: MRI findings and associations with malignancy. Ann Transl Med 2022;10:357. [Crossref] [PubMed]
- Neeter LMFH, Raat HPJF, Alcantara R, et al. Contrast-enhanced mammography: what the radiologist needs to know. BJR Open 2021;3:20210034. [Crossref] [PubMed]
- Heck L, Herzen J. Recent advances in X-ray imaging of breast tissue: From two- to three-dimensional imaging. Phys Med 2020;79:69-79. [Crossref] [PubMed]
- Savaridas SL, Tennant SL. Quantifying lesion enhancement on contrast-enhanced mammography: a review of published data. Clin Radiol 2022;77:e313-20. [Crossref] [PubMed]
- Expert Panel on Breast Imaging. ACR Appropriateness Criteria® Supplemental Breast Cancer Screening Based on Breast Density. J Am Coll Radiol 2021;18:S456-73. [Crossref] [PubMed]
- Wang Y, Zhang L, Qi L, et al. Machine Learning: Applications and Advanced Progresses of Radiomics in Endocrine Neoplasms. J Oncol 2021;2021:8615450. [Crossref] [PubMed]
- Bruno F, Granata V, Cobianchi Bellisari F, et al. Advanced Magnetic Resonance Imaging (MRI) Techniques: Technical Principles and Applications in Nanomedicine. Cancers (Basel) 2022;14:1626. [Crossref] [PubMed]
- Feng Q, Yu X, Wang Y, et al. Synthesis and functional studies of self-adjuvanting multicomponent anti-HER2 cancer vaccines. RSC Adv 2021;11:33814-22. [Crossref] [PubMed]
- Jing L, Tian Y, Ren G, et al. Epidemiological features of hypertension among ischemic survivors in Northeast China: insights from a population-based study, 2017-2019. BMC Public Health 2021;21:1648. [Crossref] [PubMed]
- Xiaowen M, Lingxi C, Song L, et al. Rare Fetus-in-Fetu: Experience From a Large Tertiary Pediatric Referral Center. Front Pediatr 2021;9:678479. [Crossref] [PubMed]
- Kuhl CK, Baltzer P. You Get What You Pay For: Breast MRI Screening of Women With Dense Breasts Is Cost-effective. J Natl Cancer Inst 2021;113:1439-41. [Crossref] [PubMed]
- Petrillo A, Fusco R, Vallone P, et al. Digital breast tomosynthesis and contrast-enhanced dual-energy digital mammography alone and in combination compared to 2D digital synthetized mammography and MR imaging in breast cancer detection and classification. Breast J 2020;26:860-72. [Crossref] [PubMed]
- Jin TY, Park KS, Nam SE, et al. BRCA1/2 Serves as a Biomarker for Poor Prognosis in Breast Carcinoma. Int J Mol Sci 2022;23:3754. [Crossref] [PubMed]
- Hesse LS, Kuling G, Veta M, et al. Intensity Augmentation to Improve Generalizability of Breast Segmentation Across Different MRI Scan Protocols. IEEE Trans Biomed Eng 2021;68:759-70. [Crossref] [PubMed]
- Li X, Guo H, Cong C, et al. The Potential Value of Texture Analysis Based on Dynamic Contrast-Enhanced MR Images in the Grading of Breast Phyllode Tumors. Front Oncol 2021;11:745242. [Crossref] [PubMed]
- Xue F, Jiang J. Dynamic Enhanced Magnetic Resonance Imaging versus Ultrasonic Diffused Optical Tomography in Early Diagnosis of Breast Cancer. J Healthc Eng 2022;2022:4834594. [Crossref] [PubMed]
- Brown KH, Ghita M, Dubois LJ, et al. A scoping review of small animal image-guided radiotherapy research: Advances, impact and future opportunities in translational radiobiology. Clin Transl Radiat Oncol 2022;34:112-9. [Crossref] [PubMed]
- Hu X, Li J, Sun Y, et al. Percentage of Tumor Invasion at Pretreatment High-Resolution Magnetic Resonance Imaging: Associating With Aggressive and Tumor Response in Chinese T3 Rectal Cancer-Preliminary Results. Front Oncol 2022;12:616310. [Crossref] [PubMed]
- Chan KS, Cheung SM, Senn N, et al. Peri-tumoural spatial distribution of lipid composition and tubule formation in breast cancer. BMC Cancer 2022;22:285. [Crossref] [PubMed]
- Wang L, Shen L, Shi J, et al. Application of multiple empirical kernel mapping ensemble classifier based on self-paced learning in ultrasound-based computer-aided diagnosis for breast cancer. Sheng Wu Yi Xue Gong Cheng Xue Za Zhi 2021;38:30-8. [PubMed]
- Zhong B, Zhang J, Wu J, et al. Efficacy and safety of palbociclib plus endocrine therapy for patients with HR +/HER2 - advanced breast cancer in real-world clinical practice. Ann Transl Med 2022;10:362. [Crossref] [PubMed]
- Wang L, Yang W, Xie X, et al. Application of digital mammography-based radiomics in the differentiation of benign and malignant round-like breast tumors and the prediction of molecular subtypes. Gland Surg 2020;9:2005-16. [Crossref] [PubMed]
(English Language Editor: L. Huleatt)