Differential expression of microRNA in the serum of patients with polycystic ovary syndrome with insulin resistance
Introduction
Polycystic ovary syndrome (PCOS) is a diverse disease with different phenotypes. The clinical manifestations of PCOS are hyperandrogenemia, irregular menstruation, and polycystic ovary morphology (PCOM) (1,2). The 2018 PCOS Evaluation and Management Guidelines update states that at least 2 of the following items must be met for a diagnosis to be made: clinical or biochemical hyperandrogen manifestations, oligoovulation or anovulation with irregular menstruation, and PCOM (3). The metabolic diseases of PCOS include insulin resistance, atherosclerotic dyslipidemia, systemic inflammation, obesity, oxidative stress, type 2 diabetes mellitus (T2DM), and cardiovascular disease (4,5). It has been reported that about 70% of patients are insulin resistant (6,7). Studies have found insulin resistance in the follicular environment of obese patients with PCOS, even when there were no clinical symptoms (8). PCOS with insulin resistance is selective and affects metabolism but does not affect the signaling pathway of mitosis (9).
Because of the heterogeneity of insulin resistance, the degree of insulin resistance varies in different tissues. The liver insulin uptake in patients with PCOS is reduced, leading to hyperinsulinemia. Abnormal insulin action has also been observed in the adipose tissue and fat cells of women with PCOS (6). Obesity-related insulin resistance seems to be mainly regulated through the PI3K pathway (10-13). In addition, hyperinsulinemia caused by insulin resistance increases the risk of obesity, leading to a vicious circle of worsening insulin resistance and its metabolic sequelae (14). Elevated insulin and glucose levels are considered hallmarks of the development of type 2 diabetes, and PCOS plays a key role in the development and progression of diabetes (15). Di et al. have found that glucose metabolism, lipid accumulation, and insulin resistance are closely related (16). In addition, the glucose metabolism of ovarian granulosa cells not only affects the proliferation and apoptosis of cells, but it also affects the quality of oocytes in the ovulation of PCOS ovaries (17,18). However, the mechanism of PCOS with insulin resistance on glucose metabolism is not clear, and there is a lack of literature on this topic. Although many mechanisms and molecular pathways leading to insulin resistance have been extensively studied, the molecular mechanisms of insulin resistance still need to be studied in depth.
MicroRNA (miRNA) is a small 21–22 nt noncoding RNA that regulates important biological processes in animals and plants. MiRNA plays a regulatory role in target gene expression and transcription (19), including the regulation of multiple gene expression, and 1 miRNA can simultaneously target several genes located in the same cell signaling pathway. A significant amount of evidence has shown that miRNA regulates various key regulatory biological functions, including cell growth and development, apoptosis, metabolism, stress response, and immune regulation (20). MiRNA promotes or inhibits miRNA signaling through a regulatory feedback mechanism. This can cause significant changes in miRNA expression, which can lead to the development of different diseases, including cardiovascular diseases and diabetes (21,22). Evidence has increasingly shown the influence of miRNAs in the pathogenesis of diabetes, and miRNAs may be a new biomarker of diabetes. Nanda and his colleagues found that miRNA-24 is associated with insulin resistance and changes in sex hormone levels, and miRNA-24 may be used as a new biomarker for the diagnosis of PCOS (23). However, no studies have focused on whole blood miRNA profiles in PCOS patients with insulin resistance.
Our research on the exact relationship between differentially expressed miRNA and PCOS is still preliminary. The role of miRNA in the diagnosis and predicted therapeutic targets of PCOS with insulin resistance needs further exploration. We present the following article in accordance with the MDAR reporting checklist (available at https://atm.amegroups.com/article/view/10.21037/atm-22-2941/rc).
Methods
General information
A total of 106 healthy women and patients with PCOS were selected from April 2021 to October 2021 in the gynecological clinic of the Second Hospital of Tianjin Medical University. The women were 17 to 45 years old, with an average age of 28 years. According to the homeostatic model assessment for insulin resistance (HOMA-IR) index {HOMA-IR = [fasting blood glucose (FBG) (mmol/L) × fasting insulin (INS) (mU/L)]/22.5}, the patients with PCOS were divided into a PCOS with insulin resistance group (n=46) and a PCOS without insulin resistance group (n=30). A group of 30 normal healthy women served as the control. The sample size meets the statistical requirements. The study was conducted in accordance with the Declaration of Helsinki (as revised in 2013). This study was approved by the Ethics Committee of the Second Hospital of Tianjin Medical University (No. KY2017K002). The informed consent of the patients under 18 years old was obtained from their legal guardians. The other patients and their family members gave informed consent and signed an informed consent form.
Selection standards
According to the 2018 PCOS Evaluation and Management Guidelines, at least 2 of the following three criteria must be met to be diagnosed: (I) sparse ovulation or anovulation or irregular uterine bleeding; (II) clinical or biochemical high androgen manifestations [hirsutism, acne, and alopecia; hyperandrogenemia is based on the determination of total testosterone (T)]; (III) ovarian polycystic changes, with ≥12 follicles of 2–9 mm in diameter on 1 or both ovaries, and/or ovarian volume (OV) ≥10 mL (OV =0.5× long diameter × transverse diameter × front and back diameter). A diagnosis of PCOS can be made if the patient meets 2 of the above items.
Observation indicators
Detailed records of the patients’ menstrual history, marriage and childbirth history, family history, other related medical history, and height and weight measurements were collected. Participants collected 2 mL of peripheral venous blood on days 2 to 5 of their menstrual period. Samples from patients with oligomenorrhea and amenorrhea were collected after ultrasound examination of nondominant follicles. Serum follicle-stimulating hormone (FSH), luteinizing hormone (LH), estradiol (E2), prolactin (PRL), T, thyroid stimulating hormone (TSH), FBG, and INS levels were detected in each group, and a whole blood cell analysis was conducted. After centrifugation, 1 mL of the upper serum was absorbed and marked for storage at −20 ℃.
RNA sequencing and bioinformatics analysis
Three patient serum samples were randomly selected from each group for RNA sequencing analysis. RNA isolation and RNA sequencing were performed. In short, a TRIzol reagent was used to extract total RNA from the specimen according to the manufacturer’s instructions. The concentration of RNA was measured with a NanoDrop 2000 spectrophotometer (Thermo Fisher Scientific, Waltham, MA, USA). An RNA library was generated from total RNA using Illumina’s NEBNext Step Superdirected RNA Library Preparation Kit (NEB, Ipswich, MA, USA). The quality and quantity of the RNA library were evaluated using an Agilent 2100 bioanalyzer (Santa Clara, CA, USA) and an ABI real-time polymerase chain reaction system (Applied Biosystems, Waltham, MA, USA), respectively. A hierarchical clustering heat map generated by R version 1.0.8 (The R Foundation for Statistical Computing, Vienna, Austria) was used to show the differentially expressed miRNA patterns between the two groups. Expression differences were characterized by log2FC (|log2fold change| >1) and P values (P<0.05). Gene Ontology (GO) analysis and Kyoto Encyclopedia of Genes and Genomes (KEGG) pathway analysis were used to explore the molecular functions, cellular components, and biological processes of the differentially expressed miRNAs (http://geneontology.org/).
Prediction, bioinformatics analysis, and network generation of miRNA target genes
We used GO terms, KEGG pathway, and Cytoscape analysis (https://cytoscape.org/) to determine the role of differentially expressed miRNAs. The target genes of the differentially expressed miRNAs were predicted using the miRTarBase, Targentscan, and miRDB databases. GO enrichment analysis was used to identify the main biological functions of the differentially expressed genes. KEGG pathway enrichment was used to study the cellular and biological functions of the test genes.
Statistical analysis
SPSS 22.0 (IBM Corp., Armonk, NY, USA) statistical software was used to process the data. The measurement data were expressed as mean ± standard deviation (SD). The two groups were compared using a Student’s t-test and a one-way analysis of variance, and a logistic multiple linear regression analysis was carried out using GraphPad Prism 8.0.1 (GraphPad Software Inc., San Diego, CA, USA). A P value of <0.05 was considered statistically significant.
Results
Analysis of relevant indicators of clinical data
Analysis of the data found that there was no significant difference in age between the three groups. The body mass index (BMI), T, PRL, FBG, and INS of the PCOS group were significantly higher than those of the healthy control group, while the E2 and FSH were significantly lower than those of the control group (Table 1).
Table 1
Variables | NC (n=30) | PCOS (n=76) | Total | P value# |
---|---|---|---|---|
Patient number (%) | 30 (28.3) | 76 (71.7) | 106 | – |
Age (years), mean (SD) | 28.83 (3.50) | 26.75 (6.16) | 27.35 (5.57) | 0.085 |
BMI (kg/m2), mean (SD) | 22.30 (2.63) | 23.68 (3.93) | 23.28 (3.63) | 0.042* |
FSH (IU/L), mean (SD) | 6.72 (1.51) | 5.10 (1.94) | 5.56 (1.95) | 0.000** |
LH (mIU/mL), mean (SD) | 6.81 (2.85) | 6.35 (5.40) | 6.51 (4.81) | 0.602 |
PRL (ng/mL), mean (SD) | 4.69 (7.03) | 16.72 (8.99) | 13.50 (10.17) | 0.000** |
E2 (pg/mL), mean (SD) | 137.75 (81.13) | 48.98 (47.21) | 72.82 (71.41) | 0.000** |
T (nmol/L), mean (SD) | 0.78 (0.29) | 1.29 (0.43) | 1.15 (0.45) | 0.000** |
TSH (μIU/mL), mean (SD) | 2.16 (1.11) | 2.16 (1.24) | 2.14 (1.21) | 0.505 |
FBG (mmol/L), mean (SD) | 5.05 (0.46) | 5.58 (0.80) | 5.43 (0.76) | 0.001** |
INS (μIU/mL), mean (SD) | 7.57 (1.45) | 12.30 (11.63) | 10.94 (10.05) | 0.001** |
#, the characteristics of the PCOS and NC groups were compared using a Student’s t-test for continuous variables. P signifies the difference between the two groups. *, P<0.05; **, P<0.01. PCOS, polycystic ovary syndrome; SD, standard deviation; BMI, body mass index; FSH, follicle-stimulating hormone; LH, luteinizing hormone; PRL, prolactin; E2, estradiol; T, testosterone; TSH, thyroid-stimulating hormone; FBG, fasting blood glucose; INS, fasting insulin; NC, normal control.
When the PCOS groups were compared, the results showed that BMI and INS were significantly higher in the PCOS with insulin resistance group than in the PCOS without insulin resistance group, while FSH was lower in the PCOS with insulin resistance group than in the PCOS without insulin resistance group, with statistically significant differences (Table 2).
Table 2
Variables | PCOS-NIR | PCOS-IR | Total | P value# |
---|---|---|---|---|
Patient number (%) | 46 (60.5) | 30 (39.5) | 76 | – |
Age (years), mean (SD) | 26.61 (6.00) | 26.97 (7.03) | 26.77 (6.12) | 0.820 |
BMI (kg/m2), mean (SD) | 22.06 (2.99) | 26.17(3.92) | 23.66 (3.91) | 0.000** |
FSH (IU/L), mean (SD) | 5.49 (1.80) | 4.52 (2.04) | 5.11 (1.93) | 0.032* |
LH (mIU/mL), mean (SD) | 7.21 (5.94) | 5.03 (4.28) | 6.93 (5.40) | 0.077 |
PRL (ng/mL), mean (SD) | 15.09 (7.38) | 19.25 (10.68) | 16.93 (9.11) | 0.074 |
E2 (pg/mL), mean (SD) | 47.25 (39.49) | 57.24 (57.06) | 47.19 (47.36) | 0.392 |
T (nmol/L), mean (SD) | 1.23 (0.42) | 1.40 (0.42) | 1.29 (0.42) | 0.089 |
TSH (μIU/mL), mean (SD) | 2.22 (1.38) | 2.06 (1.02) | 2.14 (1.26) | 0.483 |
FBG (mmol/L), mean (SD) | 5.30 (0.51) | 6.01 (1.00) | 5.57 (0.80) | 0.001** |
INS (μIU/mL), mean (SD) | 7.29 (2.20) | 19.98 (15.55) | 12.26 (11.56) | 0.000** |
#, the characteristics of the PCOS with insulin resistance and without insulin resistance groups were compared using a Student’s t-test for continuous variables. P signifies the difference between the two groups. *, P<0.05; **, P<0.01. PCOS, polycystic ovary syndrome; SD, standard deviation; BMI, body mass index; FSH, follicle-stimulating hormone; LH, luteinizing hormone; PRL, prolactin; E2, estradiol; T, testosterone; TSH, thyroid-stimulating hormone; FBG, fasting blood glucose; INS, fasting insulin; PCOS-NIR, PCOS without insulin resistance; PCOS-IR, PCOS with insulin resistance.
Analysis of factors affecting insulin resistance in patients with PCOS
Multivariate logistic regression analysis was carried out with insulin resistance as the dependent variable and whether there was a statistically different index of insulin resistance as the independent variable. The logistic regression analysis found that only insulin levels were significantly correlated with insulin resistance (r=0.9649; R squared =0.93; P<0.0001) (Figure 1). However, we found no significant correlation between sex hormone levels, BMI, FBG and INS levels. Clinically, there are indeed some normal-weight or thin patients who also have insulin resistance. Although serum FBG levels are within the normal reference range in such patients, their INS levels remain high. We need to further explore the effects of insulin resistance on granulosa cells and oocytes in ovarian tissue. How the regulation of insulin levels affects the normal development of ovarian follicles needs further verification.
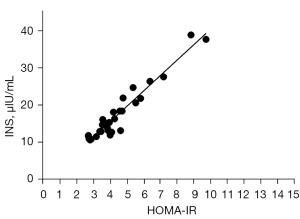
Differential expression of miRNA in serum
The “limma” package in R was used to compare the different expression profiles of miRNA among the PCOS groups and the healthy control group. In the serum, 46 differential miRNAs were identified among the three groups (Figure 2A). Enrichment analysis of differentially expressed miRNAs was carried out using GO terms (Figure 2B). The analysis of biological processes showed that most of the targeted miRNAs were related to sensory system development, positive regulation of cellular protein localization, osteoblast differentiation, and insulin response. Other targeted genes were related to lung development and respiratory tube development. The analysis of cellular components showed that the target genes of the differentially expressed miRNAs were mainly located in circulating endosomes, clathrin-coated vesicle membranes, protein kinase complexes, cell-cell adhesion junctions, and the synthesis of nuclear matrix and endosomal membranes. Others were located in microvillus, actin filament bundle, contraction of actin tows and stress fibers. The analysis of molecular functions showed that the target genes of the differentially expressed miRNAs were mainly related to enhancers, growth factor binding, regulation of oxidoreductase activity, positive regulation of oxidoreductase activity, and phosphoprotein binding. Others were related to transition metal ion transmembrane transporter activity, protein phosphorylated amino acid binding, receptor tyrosine kinase binding, messenger RNA (mRNA) 3'-untranslated region (3'-UTR) binding, and protein tyrosine kinase binding. The KEGG pathway analysis showed that the targets of these differentially expressed miRNAs were involved in a variety of pathways, including glycosphingolipid biosynthesis and mucin-type O-glycan biosynthesis. Other pathways included citrate cycle (TCA cycle) and AGE-RAGE signaling pathway in diabetic complications, p53 signaling pathway, carbohydrate digestion and absorption, thyroid hormone synthesis, cysteine and methionine metabolism and inflammatory bowel disease (Figure 2C). To verify the function of specific miRNAs in patients with insulin resistance, miRDB, TargetScan, and miRTarBase were used to predict their target genes. STRING was utilized for the Protein-Protein Interaction Network construct. Four miRNAs and 260 target genes were identified (Figure 2D). The target gene network diagram illustrated the key regulatory functions of the identified miRNA and its target genes. In short, all 4 miRNAs had specific prediction targets. At the same time, different miRNAs had common target genes. For example, among these predicted target genes, ABHD178, MAP3K7, and KIAAO225 were the common target genes of 2 miRNAs (miR-486-5p and miR-223-3p). These results indicated that differential miRNAs might have unique regulatory functions, or they might share some similar regulatory functions.
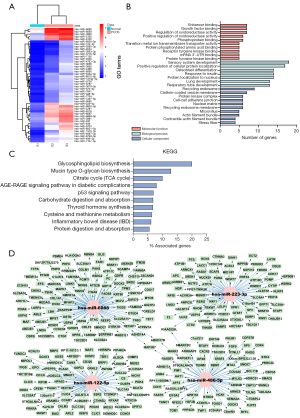
The miRNA analysis of the PCOS with insulin resistance group (S3), the PCOS without insulin resistance group (S2), and the healthy control group found that the expression of miR-223-3p was significantly different among the three groups (Figure 3A). We used GO terms and KEGG gene analysis to determine the pathway of action of differentially expressed miR-223-3p. The target genes of miR-223-3p were predicted using miRDB, TargetScan, and microRNA.org. GO analysis of the target genes indicated that miRNA-223-3p was involved in the regulation of different biological processes (Figure 3B). The analysis of biological processes showed that miRNA-223-3p was mainly related to mitotic cytokinesis and cell communication involved in cardiac conduction (Figure 3C). The analysis of cellular components showed that miRNA-223-3p was related to the perinuclear region of cytoplasm and adherens junctions. The analysis of molecular functions showed that miRNA-223-3p was mainly related to protein phosphorylated amino acid binding. The KEGG pathway analysis showed that the targets of the differentially expressed miRNAs were involved in the regulation of a variety of pathways, including proximal tubule bicarbonate reclamation and mucin type O-glycan biosynthesis (Figure 3D). We used a protein interaction Cytoscape diagram of miRNA target genes to analyze the correlation among the related target gene proteins (Figure 3E).
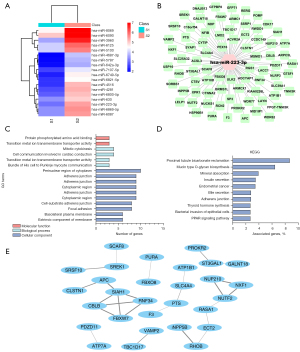
Analysis of differentially expressed miRNAs between the two PCOS groups
The miRNA analysis of the PCOS groups showed that the expression of miR-122-5p was significantly different between the two groups. The target genes of miR-122-5p were predicted using miRDB, TargetScan, and microRNA.org (Figure 4A). To gain insight into the underlying mechanism of PCOS insulin resistance, we used GO terms and KEGG pathway analysis to determine the pathway of action of differentially expressed miRNAs. The biological functions and pathways of differentially expressed miRNAs were closely related to the development of PCOS insulin resistance. GO analysis of target genes indicated that miR-122-5p was involved in the regulation of different biological processes. The analysis of biological processes showed that miR-122-5p was mainly related to the negative regulation of GTPase activity, tightly connected tissues, tightly connected components, two-cell tightly connected assembly, maintenance of protein localization in cells, and epithelial cell morphogenesis. The analysis of cellular components showed that miR-122-5p was related to chloride channel complex, ESCRT complex, and ESCRT 1 complex. The analysis of molecular functions showed that miR-122-5p was mainly related to the negative regulation of GTPase activity, transferase activity, transfer of nitrogen-containing groups, and transaminase activity (Figure 4B).
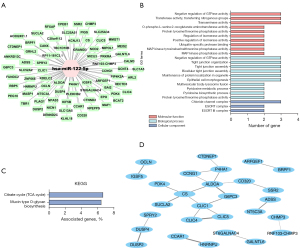
The KEGG pathway analysis showed that the targets of these differentially expressed miRNAs were involved in two pathways: the citrate cycle (TCA) and the biosynthesis of mucin-type O-glycans (Figure 4C). We conducted a Cytoscape protein interaction analysis of miRNA target genes and found that G6PC3 and other proteins participated in the mechanism of regulating insulin resistance (Figure 4D). In summary, these results indicated that miR-122-5p plays an important regulatory role in the regulation of insulin resistance in PCOS.
Discussion
PCOS is a common gynecological endocrine disease characterized by hyperandrogenemia and insulin resistance (9). Insulin resistance is a common feature in women with PCOS, with approximately 60–80% of patients developing insulin resistance (24). This suggests a strong link between PCOS and insulin resistance. There are many ways to improve the mechanism of insulin resistance, such as changing the intestinal microbiota (25), reducing oxidative stress (26,27), controlling weight (28), changing lifestyles (29), and balancing nutrients (30). However, the underlying mechanism of PCOS with insulin resistance remains unclear.
The common pathogenesis of PCOS includes insulin resistance, obesity, chronic inflammation, and mitochondrial dysfunction. Insulin resistance can lead to increased androgen levels and infertility, thereby exacerbating PCOS. While many factors contribute to insulin resistance in PCOS patients, the main factor is obesity. Approximately 30–60% of patients with PCOS exhibit symptoms of obesity. Obesity has a great influence on different PCOS phenotypes and affects the management of symptoms and reproductive outcomes (31). In our clinical case statistics, we found that high BMI (>25 kg/m2), hyperglycemia, and hyperinsulinemia were strongly correlated with PCOS and insulin resistance. Obesity and insulin resistance may interfere with the signal transduction of the action of insulin by inhibiting insulin, causing inflammatory changes, and leading to oxidative stress. Some scholars have found that obesity can activate the toll-like receptor 2 (TLR2), toll-like receptor 4 (TLR4) (32), DNA-PKCs (PKCs), and nuclear factor-κB (NF-κB) pathways. In addition, the expression of tumor necrosis factor-α (TNF-α), interleukin-6 (IL-6) (33), interleukin-8 (IL-8) (34), and C-reactive protein (CRP) induces insulin resistance. One study found that although a large number of PCOS patients in the obese group did not show obvious clinical symptoms of insulin resistance, for instance BCL2L1, BRAF and CBL were mainly responsible for the proliferation and differentiation of cumulus cells, insulin resistance, and apoptosis during oocyte maturation (8). These changes may be related to the poor prognosis of follicular development and oocyte maturation in obese women with PCOS.
There is a correlation between insulin resistance and miRNA. miR-33b-5p is overexpressed in the ovarian tissue of insulin-resistant PCOS rats and therefore may play an important role in the development of insulin resistance in patients with PCOS (35). Another study found that miR-3585-5p and miR-30-5p were significantly upregulated and miR-146-5p was downregulated in the ovaries of rats with PCOS and insulin resistance (36). There are many changes in the expression of miRNA in the follicular fluid of patients with PCOS, and miR-127-3p has been found to be associated with insulin resistance (37). One study found that in PCOS ovarian granulosa cells, inhibition of long noncoding RNA (lncRNA) plasmacytoma variant translocation 1 (PVT1) and overexpression of miR-17-5p resulted in the downregulation of phosphatase and tensin homolog (PTEN) and promoted cell proliferation, thereby inhibiting the apoptosis of ovarian granulosa cells (38). In addition, the downregulation of miR-99a expression in patients with PCOS may be closely related to insulin resistance and hyperinsulinemia (39). miR-99a can inhibit the proliferation of human glomerular basement membrane cells and promote their apoptosis by targeting insulin-like growth factor 1 receptor (IGF-1R), which may be part of the cause of abnormal PCOS follicles (40). Research has increasingly shown that miRNAs are closely related to insulin resistance, and they may play an important role in PCOS with insulin resistance (40,41).
Our results showed that miR-486-5p, miR-223-3p, miR-6088, and miR-122-5p had significant differences between the PCOS group and the control group. Comparative analysis between the control group and the PCOS without insulin resistance group found that the expression of miR-223-3p was significantly different. The expression of miR-122-5p was significantly high in the PCOS group, especially in PCOS patients with insulin resistance. Expression of miR-122-5p has been implicated in the development of multiple cancers and insulin resistance. A study by Badacz and other scholars (42) found that miR-122-5p may be related to the occurrence of cardiovascular disease. Compared with PCOS patients without insulin resistance, the expression of miR-122-5p in PCOS patients with insulin resistance was significantly increased. In recent years, the effect of the mitochondrial biosynthetic pathway on the follicular development and glucose and lipid metabolism mechanism of PCOS has become a research hotspot. Using KEGG pathway analysis, our study found that miR-122-5p plays an important role in the TCA cycle and may participate in the regulation of glucose and lipid metabolism pathways and thus affect the PCOS insulin signal transduction.
In summary, our statistical clinical data found that the levels of T, BMI, FBG, and INS in patients with PCOS were generally higher than those in healthy controls. Among patients with PCOS, high BMI, high blood sugar, and hyperinsulinemia were more significant in the insulin resistance group. In PCOS, obesity and insulin resistance lead to glucose and lipid metabolism disorders. The degree of insulin resistance can be assessed by BMI and other obesity-related indicators such as waist circumference, waist height ratio (WHtR), waist circumference/height0.5 (WHT.5R), and lipid accumulation products (LAP), which can increase the cardiovascular and metabolic risk of patients with PCOS (43,44). Studies have shown that women with PCOS have different epigenetic regulation, which may be caused by an unfavorable intrauterine environment or post-natal environmental factors such as diet and/or obesity (45-47). Both obesity and high androgen can aggravate insulin resistance. Mu and Li found that the expression of miR-103 in obese women with PCOS showed a slight downward trend after fat loss (45). In our study, microarray analysis was used to detect the blood samples of each group of cases, and differentially expressed miRNAs were found in the PCOS group compared with the control group. In addition, 4 significantly differentially expressed miRNAs were found in PCOS patients with insulin resistance, namely miR-486-5p, miR-223-3p, miR-6088, and miR-122-5p. In the PCOS group, it was found that miR-122-5p had the largest difference in the group with insulin resistance. A research found that the overexpression of miR-122 in the serum of patients with PCOS with impaired glucose tolerance was significantly associated with those without impaired glucose tolerance, and it is closely related to the regulation of insulin signaling pathway (48). Udesen and colleagues found that miR-122 was significantly reduced in the serum of women with PCOS after metformin treatment, and this report also suggests that miR-122 regulation may be related to insulin sensitivity (49). These all suggest that the regulation of miR-122 is crucial for the diagnosis and treatment of PCOS with insulin resistance, but the specific mechanism remains confused.
In our research, we have found that miR-122-5p was involved in multiple pathways by GO analysis. The analysis of biological processes and molecular functions showed that miR-122-5p was mainly related to the negative regulation of GTPase activity, while the analysis of cellular components showed that miR-122-5p was related to chloride channel complex, ESCRT complex, and ESCRT 1 complex. The KEGG pathway analysis showed that the target genes of miR-122-5p were mainly involved in two pathways, namely the TCA cycle and the biosynthesis of mucin-type O-glycans. The protein interaction network database analysis found that the target genes G6PC3, ALDOA, and CLIC4 were related to each other. The expression of miRNA in the human body is affected by many factors, such as intergenerational inheritance (50) and environmental factors (51). Based on these results, we need to re-validate in ovarian tissue, further explore the effect of miR-122 on ovarian function in women with PCOS, and further explore the role of miR-122 in animals and cell models. Therefore, it is necessary to collect more clinical specimens of patients with PCOS to determine the potential mechanism of miR-122-5p in regulating the insulin resistance pathway and participating in the progression of PCOS.
Acknowledgments
Funding: None.
Footnote
Reporting Checklist: The authors have completed the MDAR reporting checklist. Available at https://atm.amegroups.com/article/view/10.21037/atm-22-2941/rc
Data Sharing Statement: Available at https://atm.amegroups.com/article/view/10.21037/atm-22-2941/dss
Conflicts of Interest: All authors have completed the ICMJE uniform disclosure form (available at https://atm.amegroups.com/article/view/10.21037/atm-22-2941/coif). The authors have no conflicts of interest to declare.
Ethical Statement: The authors are accountable for all aspects of the work in ensuring that questions related to the accuracy or integrity of any part of the work are appropriately investigated and resolved. The study was conducted in accordance with the Declaration of Helsinki (as revised in 2013). This study was approved by Ethics Committee of the Second Hospital of Tianjin Medical University (No. KY2017K002). The informed consent of the patients under 18 years old was obtained from their legal guardians. The other patients and their family members gave informed consent and signed an informed consent form.
Open Access Statement: This is an Open Access article distributed in accordance with the Creative Commons Attribution-NonCommercial-NoDerivs 4.0 International License (CC BY-NC-ND 4.0), which permits the non-commercial replication and distribution of the article with the strict proviso that no changes or edits are made and the original work is properly cited (including links to both the formal publication through the relevant DOI and the license). See: https://creativecommons.org/licenses/by-nc-nd/4.0/.
References
- Patel S. Polycystic ovary syndrome (PCOS), an inflammatory, systemic, lifestyle endocrinopathy. J Steroid Biochem Mol Biol 2018;182:27-36. [Crossref] [PubMed]
- Bozdag G, Mumusoglu S, Zengin D, et al. The prevalence and phenotypic features of polycystic ovary syndrome: a systematic review and meta-analysis. Hum Reprod 2016;31:2841-55. [Crossref] [PubMed]
- Teede HJ, Misso ML, Costello MF, et al. Recommendations from the international evidence-based guideline for the assessment and management of polycystic ovary syndrome. Clin Endocrinol (Oxf) 2018;89:251-68. [Crossref] [PubMed]
- Anagnostis P, Tarlatzis BC, Kauffman RP. Polycystic ovarian syndrome (PCOS): Long-term metabolic consequences. Metabolism 2018;86:33-43. [Crossref] [PubMed]
- Qu X, Donnelly R. Sex Hormone-Binding Globulin (SHBG) as an Early Biomarker and Therapeutic Target in Polycystic Ovary Syndrome. Int J Mol Sci 2020;21:8191. [Crossref] [PubMed]
- Moghetti P. Insulin Resistance and Polycystic Ovary Syndrome. Curr Pharm Des 2016;22:5526-34. [Crossref] [PubMed]
- Tosi F, Bonora E, Moghetti P. Insulin resistance in a large cohort of women with polycystic ovary syndrome: a comparison between euglycaemic-hyperinsulinaemic clamp and surrogate indexes. Hum Reprod 2017;32:2515-21. [Crossref] [PubMed]
- Chehin MB, Fraietta R, Lorenzon AR, et al. The insulin signaling pathway is dysregulated in cumulus cells from obese, infertile women with polycystic ovarian syndrome with an absence of clinical insulin resistance. Ther Adv Reprod Health 2020;14:2633494120906866. [Crossref] [PubMed]
- Diamanti-Kandarakis E, Dunaif A. Insulin resistance and the polycystic ovary syndrome revisited: an update on mechanisms and implications. Endocr Rev 2012;33:981-1030. [Crossref] [PubMed]
- Lebovitz HE. Insulin resistance: definition and consequences. Exp Clin Endocrinol Diabetes 2001;109:S135-48. [Crossref] [PubMed]
- Barber TM, McCarthy MI, Wass JA, et al. Obesity and polycystic ovary syndrome. Clin Endocrinol (Oxf) 2006;65:137-45. [Crossref] [PubMed]
- Cusi K, Maezono K, Osman A, et al. Insulin resistance differentially affects the PI 3-kinase- and MAP kinase-mediated signaling in human muscle. J Clin Invest 2000;105:311-20. [Crossref] [PubMed]
- Muntoni S, Muntoni S. Insulin resistance: pathophysiology and rationale for treatment. Ann Nutr Metab 2011;58:25-36. [Crossref] [PubMed]
- Pennings N, Jaber J, Ahiawodzi P. Ten-year weight gain is associated with elevated fasting insulin levels and precedes glucose elevation. Diabetes Metab Res Rev 2018;34:e2986. [Crossref] [PubMed]
- Bednarska S, Siejka A. The pathogenesis and treatment of polycystic ovary syndrome: What's new? Adv Clin Exp Med 2017;26:359-67. [Crossref] [PubMed]
- Di F, Liu J, Li S, et al. Activating transcriptional factor 4 correlated with obesity and insulin resistance in polycystic ovary syndrome. Gynecol Endocrinol 2019;35:351-5. [Crossref] [PubMed]
- Li W, Li D, Kuang H, et al. Berberine increases glucose uptake and intracellular ROS levels by promoting Sirtuin 3 ubiquitination. Biomed Pharmacother 2020;121:109563. [Crossref] [PubMed]
- Zhang Q, Ren J, Wang F, et al. Mitochondrial and glucose metabolic dysfunctions in granulosa cells induce impaired oocytes of polycystic ovary syndrome through Sirtuin 3. Free Radic Biol Med 2022;187:1-16. [Crossref] [PubMed]
- Ha M, Kim VN. Regulation of microRNA biogenesis. Nat Rev Mol Cell Biol 2014;15:509-24. [Crossref] [PubMed]
- Schmartz GP, Kern F, Fehlmann T, et al. Encyclopedia of tools for the analysis of miRNA isoforms. Brief Bioinform 2021;22:bbaa346. [Crossref] [PubMed]
- Xue Y, Lv J, Xu P, et al. Identification of microRNAs and genes associated with hyperandrogenism in the follicular fluid of women with polycystic ovary syndrome. J Cell Biochem 2018;119:3913-21. [Crossref] [PubMed]
- Rashad NM, El-Shal AS, Abomandour HG, et al. Intercellular adhesion molecule-1 expression and serum levels as markers of pre-clinical atherosclerosis in polycystic ovary syndrome. J Ovarian Res 2019;12:97. [Crossref] [PubMed]
- Nanda D, Chandrasekaran SP, Ramachandran V, et al. Evaluation of Serum miRNA-24, miRNA-29a and miRNA-502-3p Expression in PCOS Subjects: Correlation with Biochemical Parameters Related to PCOS and Insulin Resistance. Indian J Clin Biochem 2020;35:169-78. [Crossref] [PubMed]
- Macut D, Bjekić-Macut J, Rahelić D, et al. Insulin and the polycystic ovary syndrome. Diabetes Res Clin Pract 2017;130:163-70. [Crossref] [PubMed]
- Starling S. Key role for gut microbiota in PCOS development. Nat Rev Endocrinol 2021;17:515. [Crossref] [PubMed]
- da Costa CS, Oliveira TF, Freitas-Lima LC, et al. Subacute cadmium exposure disrupts the hypothalamic-pituitary-gonadal axis, leading to polycystic ovarian syndrome and premature ovarian failure features in female rats. Environ Pollut 2021;269:116154. [Crossref] [PubMed]
- Mancini A, Bruno C, Vergani E, et al. Oxidative Stress and Low-Grade Inflammation in Polycystic Ovary Syndrome: Controversies and New Insights. Int J Mol Sci 2021;22:1667. [Crossref] [PubMed]
- Barber TM, Kyrou I, Randeva HS, et al. Mechanisms of Insulin Resistance at the Crossroad of Obesity with Associated Metabolic Abnormalities and Cognitive Dysfunction. Int J Mol Sci 2021;22:546. [Crossref] [PubMed]
- Shah A, Dodson WC, Kris-Etherton PM, et al. Effects of Oral Contraception and Lifestyle Modification on Incretins and TGF-ß Superfamily Hormones in PCOS. J Clin Endocrinol Metab 2021;106:108-19. [Crossref] [PubMed]
- Muscogiuri G, Barrea L, Caprio M, et al. Nutritional guidelines for the management of insulin resistance. Crit Rev Food Sci Nutr 2021; Epub ahead of print. [Crossref] [PubMed]
- Cena H, Chiovato L, Nappi RE. Obesity, Polycystic Ovary Syndrome, and Infertility: A New Avenue for GLP-1 Receptor Agonists. J Clin Endocrinol Metab 2020;105:dgaa285. [Crossref] [PubMed]
- Aly RH, Ahmed AE, Hozayen WG, et al. Patterns of Toll-Like Receptor Expressions and Inflammatory Cytokine Levels and Their Implications in the Progress of Insulin Resistance and Diabetic Nephropathy in Type 2 Diabetic Patients. Front Physiol 2020;11:609223. [Crossref] [PubMed]
- Snider AP, Wood JR. Obesity induces ovarian inflammation and reduces oocyte quality. Reproduction 2019;158:R79-90. [Crossref] [PubMed]
- Rostamtabar M, Esmaeilzadeh S, Tourani M, et al. Pathophysiological roles of chronic low-grade inflammation mediators in polycystic ovary syndrome. J Cell Physiol 2021;236:824-38. [Crossref] [PubMed]
- Mondal K, Chakraborty P, Kabir SN. Hyperhomocysteinemia and hyperandrogenemia share PCSK9-LDLR pathway to disrupt lipid homeostasis in PCOS. Biochem Biophys Res Commun 2018;503:8-13. [Crossref] [PubMed]
- Zhang C, Yu C, Lin Z, et al. MiRNAs expression profiling of rat ovaries displaying PCOS with insulin resistance. Arch Gynecol Obstet 2020;302:1205-13. [Crossref] [PubMed]
- Cui C, Wang J, Han X, et al. Identification of small extracellular vesicle-linked miRNA specifically derived from intrafollicular cells in women with polycystic ovary syndrome. Reprod Biomed Online 2021;42:870-80. [Crossref] [PubMed]
- Liu G, Liu S, Xing G, et al. lncRNA PVT1/MicroRNA-17-5p/PTEN Axis Regulates Secretion of E2 and P4, Proliferation, and Apoptosis of Ovarian Granulosa Cells in PCOS. Mol Ther Nucleic Acids 2020;20:205-16. [Crossref] [PubMed]
- Cave MC, Pinkston CM, Rai SN, et al. Circulating MicroRNAs, Polychlorinated Biphenyls, and Environmental Liver Disease in the Anniston Community Health Survey. Environ Health Perspect 2022;130:17003. [Crossref] [PubMed]
- Geng Y, Sui C, Xun Y, et al. MiRNA-99a can regulate proliferation and apoptosis of human granulosa cells via targeting IGF-1R in polycystic ovary syndrome. J Assist Reprod Genet 2019;36:211-21. [Crossref] [PubMed]
- Laganà AS, Garzon S, Casarin J, et al. Inositol in Polycystic Ovary Syndrome: Restoring Fertility through a Pathophysiology-Based Approach. Trends Endocrinol Metab 2018;29:768-80. [Crossref] [PubMed]
- Badacz R, Kleczyński P, Legutko J, et al. Expression of miR-1-3p, miR-16-5p and miR-122-5p as Possible Risk Factors of Secondary Cardiovascular Events. Biomedicines 2021;9:1055. [Crossref] [PubMed]
- Butler AE, Ramachandran V, Hayat S, et al. Expression of microRNA in follicular fluid in women with and without PCOS. Sci Rep 2019;9:16306. [Crossref] [PubMed]
- Kałużna M, Czlapka-Matyasik M, Bykowska-Derda A, et al. Indirect Predictors of Visceral Adipose Tissue in Women with Polycystic Ovary Syndrome: A Comparison of Methods. Nutrients 2021;13:2494. [Crossref] [PubMed]
- Mu J, Li Q. Anomalous expression of miR-103 in polycystic ovary syndrome influenced by hormonal, and metabolic variables. Exp Mol Pathol 2020;116:104482. [Crossref] [PubMed]
- Glueck CJ, Goldenberg N. Characteristics of obesity in polycystic ovary syndrome: Etiology, treatment, and genetics. Metabolism 2019;92:108-20. [Crossref] [PubMed]
- de Zegher F, López-Bermejo A, Ibáñez L. Central Obesity, Faster Maturation, and 'PCOS' in Girls. Trends Endocrinol Metab 2018;29:815-8. [Crossref] [PubMed]
- Jiang L, Huang J, Chen Y, et al. Identification of several circulating microRNAs from a genome-wide circulating microRNA expression profile as potential biomarkers for impaired glucose metabolism in polycystic ovarian syndrome. Endocrine 2016;53:280-90. [Crossref] [PubMed]
- Udesen PB, Glintborg D, Sørensen AE, et al. Metformin decreases miR-122, miR-223 and miR-29a in women with polycystic ovary syndrome. Endocr Connect 2020;9:1075-84. [Crossref] [PubMed]
- Mimouni NEH, Paiva I, Barbotin AL, et al. Polycystic ovary syndrome is transmitted via a transgenerational epigenetic process. Cell Metab 2021;33:513-30.e8. [Crossref] [PubMed]
- Makrinou E, Drong AW, Christopoulos G, et al. Genome-wide methylation profiling in granulosa lutein cells of women with polycystic ovary syndrome (PCOS). Mol Cell Endocrinol 2020;500:110611. [Crossref] [PubMed]
(English Language Editor: C. Gourlay)