Identification of key genes related to immune cells in patients with gram-negative sepsis based on weighted gene co-expression network analysis
Introduction
Sepsis is a potentially life-threatening condition caused by an excessive immune response against an invading pathogen (1). It occurs when the host immune system overreacts to infections and causes damage to the host tissues and organs. In 2017, there were an estimated 47–49 million cases of sepsis globally, with 11 million sepsis-related deaths, accounting for almost 1 in 5 total deaths worldwide (2). Thus, sepsis has become a significant global public health concern.
Sepsis resulting from gram-negative bacterial infection is characterized by severe inflammatory response, multi-organ dysfunction, and a high mortality rate (~60%) (3). Many studies have confirmed that lipopolysaccharide (LPS), a major microbial mediator of sepsis resulting from gram-negative bacterial infection, can elicit a strong immune response (4-6). However, clinical signs and symptoms of early sepsis are often non-specific and can easily be missed. Despite extensive research and continuous development of immunological events, the mortality associated with gram-negative sepsis remains high (2,7,8). Knowledge of early molecular and biological changes in sepsis may help practitioners assess the condition and initiate timely treatment (9). Therefore, an in-depth understanding of the pathogenesis of gram-negative sepsis is warranted.
It is now clear that the intricate disruption of the host response is a major feature of sepsis, involving both sustained and profound inflammation and immune suppression, and an inability to re-establish homeostasis (1). Studies on the genomic response to sepsis have provided valuable insights into this imbalance. Evidence suggests that host genetics can influence sepsis outcomes (10). Patients with sepsis show significantly different blood leukocyte transcriptomes, with more than 70% of the measured RNA transcripts in these cells involved in inflammatory, translation initiation, adaptive immunity, or mitochondrial dysfunction pathways (11-17).
Sepsis directly or indirectly affects the function of almost all types of immune cells. Among the gram-negative bacteremia species that have been interrogated using genome-wide approaches to fitness factor identification in the mammalian bloodstream, there has been a predominance of mechanisms that support evasion of immune defenses and promote metabolic diversity (18). The formation of inflammasome, polarization and apoptosis of immune cells, cytokine explosion and apoptosis, and even exosomes play orchestrated roles in microenvironment of sepsis. Genetic changes in peripheral blood mononuclear cells (PBMCs) have been found to be associated with severity, immunosuppression, and mortality in patients (19). PBMCs play a well-established role in monitoring infection because they can encounter the source of infection and interact with infected cells through secreted signaling molecules.
This current study used bioinformatics to identify the immune cells that are closely related to gram-negative sepsis and to analyze the potential molecular mechanisms of immune cell-related gene regulation, with the aim of screening sepsis-specific biomarkers for early diagnosis and treatment. Our study provides novel insights into the molecular mechanisms of immune cells in the pathogenesis of gram-negative sepsis. We present the following article in accordance with the STREGA reporting checklist (available at https://atm.amegroups.com/article/view/10.21037/atm-22-3307/rc).
Methods
Data source and the identification of differentially expressed genes
The gene expression profiles of 18 gram-negative sepsis samples and 16 normal samples were downloaded from the GSE9960 datasets (A total of 70 critically ill patients were enrolled in a single-centre observational study. Gene-expression profiling was performed using Affymetrix microarray with 54,675 transcripts) (19) and the differentially expressed genes were screened using the limma package (20) with P value <0.05 and |log2 (fold change)| >1 as the selection criteria.
Distribution of immune cells
The gene expression data in the GSE9960 dataset were uploaded to the CIBERSORT software (21) and the proportions of 22 immune cells in each sample were predicted. Immune cells that showed significantly different distribution between gram-negative sepsis and normal samples were selected for subsequent analyses.
Weighted gene co-expression network analysis (WGCNA)
A sample cluster tree map was constructed to explore and exclude outliers. WGCNA was then implemented on the gene expression data and information of the selected immune cells. For the purpose of selecting an optimal soft threshold, we used the pick soft threshold function of WGCNA to figure β from 1 to 30. According to the selected soft threshold, the adjacency matrix was transformed into a topological overlap matrix to establish the network. A gene dendrogram and module color were constructed according to the degree of dissimilarity. Furthermore, the initial module was divided by dynamic tree cutting and the similar ones were merged. The Pearson correlation coefficient between eigengenes of the module and the differential infiltration level of immune cells was calculated to determine the module most associated with the selected immune cells.
Selection of hub gene signatures by least absolute shrinkage and selection operator (LASSO) and support vector machine-recursive feature elimination (SVM-RFE)
The glmnet package (22) in R was used to apply the LASSO algorithm and explore gene signatures under the most desirable lambda with the smallest classification error. The e1071 package in R was used to implement SVM-RFE to determine the best variables (gene signatures) by deleting feature vectors generated by SVM in combination with 5-fold cross-validation. Finally, by overlapping gene signatures, hub gene signatures were identified from the LASSO and SVM-RFE algorithms.
Functional enrichment analysis
The Metascape online tool (https://metascape.org) was applied to analyze the biological functions of genes related to immune cells, which integrated the Kyoto Encyclopedia of Genes and Genomes (KEGG) and Reactome data resources. In addition, Ingenuity® Pathway Analysis (IPA) (23) was used to investigate disease and biological functions of hub gene signatures and to predict the interaction of hub gene signatures with other differentially expressed genes in gram-negative sepsis.
Validation of key differentially expressed genes by quantitative polymerase chain reaction (qRT-PCR)
A total of 32 participants, including 17 candidates who fulfilled the criteria for Sepsis 3.0 and 15 non-infectious disease volunteers, were recruited from the Emergency Medicine Clinical Research Center in Beijing Chaoyang Hospital, Capital Medical University. The study was conducted in accordance with the Declaration of Helsinki (as revised in 2013). The current study on human participants was examined and approved by the Research Ethics Committee of Beijing Chaoyang Hospital, Capital Medical University (No. 2021-ke-316). The candidates provided written informed consent to participate in this study. There was no significant difference in age (P=0.73) or sex (P=0.91) between the two groups. The PBMCs were purified via density-gradient separation. Blood samples were diluted (1:1) with phosphate-buffered saline, layered over the same volume of Ficoll-Paque (Solarbio, Code No. P9010, China), and centrifuged at 1,000 ×g for 30 minutes. The buffy coat (containing mononuclear cells) was aspirated and collected. The total RNA was extracted using RNAiso Plus (TaKaRa, Code No. 9109, Japan), chloroform, and isopropyl alcohol. The extracted RNA was reversed transcribed to cDNA and qRT-PCR was performed using One Step PrimeScriptTM RT-PCR Kit (TaKaRa, Code No. RR086A, Japan). Primer sequences (Sangon Biotech, Lot No. 2014250862, 2014250863, 2014423459, 2014423460, B661102, China) used in this study are shown in Table 1.
Table 1
Primer set | Real-time quantitative PCR primer |
---|---|
RGL4-F | ATGGATGCGGAGCTGTTCAAGAAG |
RGL4-R | AGCCTGTTGAAGTGTGCGATGG |
NLRC4-F | TGGCAAAGGCAAGTCCACTC |
NLRC4-R | CCTGCTGAGACGGAGGAAGA |
β-actin-F | CTACAATGAGCTGCGTGTGG |
β-actin-R | AAGGAAGGCTGGAAGAGTGC |
PCR, polymerase chain reaction; RGL4, ral guanine nucleotide dissociation stimulator like 4; NLRC4, NLR family CARD domain-containing 4.
Statistical analysis
All data were analyzed using R software (v.4.0.0, http://www.r-project.org/). The Wilcoxon test was applied to compare proportions of immune cells between gram-negative sepsis and normal samples, and a P value of <0.05 was considered statistically significant. The diagnostic value of the hub gene signatures was evaluated using the area under the receiver operating characteristic (ROC) curve. Unpaired t-test was performed for PCR results (2−ΔΔct) of clinical samples through GraphPad Prism version 8 (GraphPad software; https://www.graphpad.com/) and a P value <0.05 was considered statistically significant.
Results
Distribution of immune cells in gram-negative sepsis and normal samples
CIBERSORT was used to investigate the distribution of immune cells in gram-negative septic and normal samples. Follicular helper T cells, regulatory T cells, activated NK cells, M2 macrophages, resting dendritic cells, and activated mast cells were barely detected in either gram-negative sepsis samples or normal samples (Figure 1A). Interestingly, the proportions of plasma cells, M1 macrophages, and eosinophils were significantly higher in gram-negative sepsis samples compared to normal samples, while the proportion of resting CD4+ memory T cells was remarkably lower in gram-negative sepsis samples than in normal samples (Figure 1B). Therefore, plasma cells, M1 macrophages, eosinophils, and resting CD4+ memory T cells were selected for WGCNA.
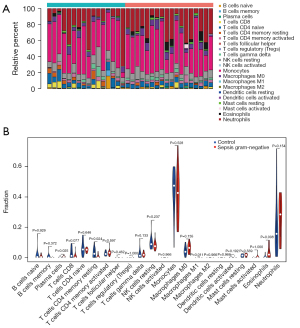
Identification of the key module associated with the selected immune cells
Outlier samples were not explored based on the clustering results. A dendrogram and trait heatmap were constructed, as shown in Figure 2A. Using the pick soft threshold function in WGCNA, the best soft threshold power was determined to be 30, in which R2 was approximately 0.9 (Figure 2B). A total of 8 modules were identified in the co-expression network after merging similar modules (Figure 2C). Correlations between module eigengenes and selected immune cells are displayed in Figure 2D. In the module-trait relationships shown in Figure 2D, the dark orange module (MEdarkorange) was most associated with the selected immune cells. The correlation coefficients between the dark orange module and plasma cells, M1 macrophages, eosinophils, and resting CD4+ memory T cells were 0.52, 0.48, 0.47, and −0.52, respectively. A total of 1,100 genes in the dark orange module were selected for further analysis.
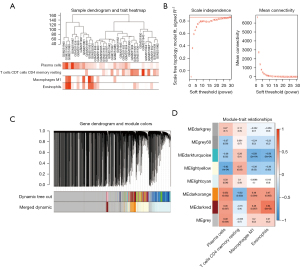
Identification of immune cell-related genes in gram-negative sepsis
A total of 87 differentially expressed genes were identified in gram-negative sepsis samples compared to normal samples, with 62 upregulated genes and 25 downregulated genes (Figure 3A), suggesting that they may be associated with the development of gram-negative sepsis. The expression of these 87 genes is shown in a heatmap in Figure 3B. Furthermore, to explore immune cell-related genes associated with the development of gram-negative sepsis, the 1,100 genes identified in the WGCNA analysis were overlapped with the 87 differentially expressed genes and a total of 46 immune cell-related genes in gram-negative sepsis were identified (Figure 3C). The potential biological functions of all 46 immune cell-related genes were investigated using Metascape. As shown in Figure 3D, these genes were primarily enriched in biological processes and pathways closely involved in immunity and inflammation, including neutrophil degranulation, leukocyte differentiation, regulation of phosphatidylinositol 3-kinase signaling, anti-inflammatory responses favoring Leishmania parasite infection, and regulation of leukocyte activation.
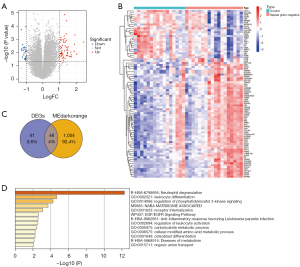
Identification of hub immune cell-related genes in gram-negative sepsis
To screen for hub immune cell-related genes in gram-negative sepsis, the LASSO algorithm was used to obtain 11 immune cell-related gene signatures, including NLR family CARD domain-containing 4 (NLRC4), platelet-derived growth factor C (PDGFC), NEDD4 E3 ubiquitin protein ligase (NEDD4), sulfotransferase family 1 B member 1 (SULT1B1), dachshund family transcription factor 1 (DACH1), CEA cell adhesion molecule 21 (CEACAM21), solute carrier family 26 member 8 (SLC26A8), cystatin F (CST7), ral guanine nucleotide dissociation stimulator like 4 (RGL4), G-quadruplex forming sequence containing lncRNA (ST3GAL4-AS1), and C-type lectin domain containing 5A (CLEC5A) (Figure 4A,4B). To obtain more robust gene signatures, the SVM-RFE algorithm was used and two immune cell-related gene signatures were detected at 5-fold cross-validation, namely, NLRC4 and RGL4 (Figure 4C,4D). After the overlapping immune cell-related gene signatures were algorithm-selected, NLRC4 and RGL4 were ascertained as hub immune cell-related genes in gram-negative sepsis (Figure 4E). Subsequently, ROC curve analysis was used to validate the diagnostic value of NLRC4 and RGL4. The area under the ROC curve for NLRC4 and RGL4 were 0.906 and 0.712, respectively (Figure 4F).
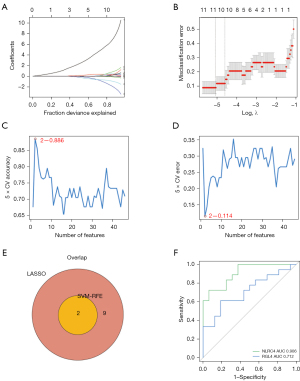
Gene interaction network of NLRC4 and RGL4 in gram-negative sepsis
IPA analysis was performed to investigate the interaction of NLRC4 and RGL4 with other differentially expressed genes in gram-negative sepsis and normal samples. Ten gene networks were identified, with scores ranging from 2 to 41. NLRC4 was in network 2 and was associated with developmental disorders, humoral immune responses, and protein synthesis (score =30). RGL4 was found in network 6 and was associated with hereditary disorders, organismal injury and abnormalities, and skeletal and muscular disorders (score =14). By connecting the two networks, NLRC4 and RGL4 were predicted to indirectly interact with other differentially expressed genes, such as NEDD4, CST7, and CLEC5A (Figure 5).
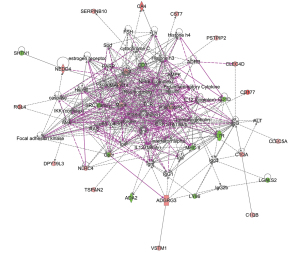
Verification of NLRC4 and RGL4 in patients with sepsis by qRT-PCR
The detection of NLRC4 and RGL4 in sepsis was performed for validation. The expression levels of NLRC4 and RGL4 in participants with sepsis were significantly higher than those in the non-infectious group (P=0.0021 and 0.0267, respectively; Figure 6A,6B).
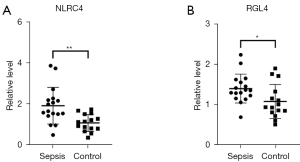
Discussion
Sepsis is characterized by the intricate interplay between varying cellular constituents of innate and adaptive immunity, in the context of tremendous pro-inflammatory and anti-inflammatory factors, in which a wide range of cytokines and chemokines are closely involved. Four types of immune cells with different distributions were found between gram-negative sepsis and normal samples, namely plasma cells, resting memory CD4 T cells, macrophages M1, and eosinophils. Indeed, sepsis has been shown to directly or indirectly affect almost all immune cell types, in number and function (24). The specific functions and mechanisms of these immune cells in sepsis have been studied extensively.
Plasma cells play a crucial role in the acute response to infections and act as antibody-secreting machines for long-term host protection by providing humoral immunity. Most pathogens transmit from cell to cell through extracellular fluids. The extracellular space is preserved by humoral immunity, being dependent on antibody-mediated responses (25). Antibodies produced by plasma cells damage extracellular microorganisms, thereby blocking the spread of infections (26). Although many pathogens can cause sepsis, plasma cell-mediated humoral immunity is important for sepsis response. Studies have shown that plasma cells generated from the lungs of mice or humans that have recovered from pneumococcal infections can protect against bacterial pneumonia (27). After challenge, plasma cells upregulate interleukin (IL)-10 expression via a Toll-like receptor-driven mechanism in a short time (28).
Resting memory CD4+ T cells are thought to be one of the HIV reservoirs, which represent a major barrier to HIV eradication (29). In addition, these cells are associated with allograft transplant rejection and tolerance, as well as immune infiltration in multiple cancers. While there is little in the literature regarding resting memory CD4+ T cells to sepsis or bacterial infections, there are some data regarding to cell heterogeneity. Heterogeneity refers to the diversity of the cell population, and not the conversion of a single differentiated cell into another subtype. Cell maintenance in a quiescent state is critical for self-renewal potential and differentiation plasticity. For cell survival and homeostatic proliferation, resting memory CD4+ T cells were dependent on signals with IL-7 and IL-15, but not major histocompatibility complex (MHC) class II (30). Using major lineage-defining phenotypic markers, resting memory CD4+ T cells have been shown to remain stable over time (31).
Macrophages are phagocytic cells that participate in steady-state tissue homeostasis via clearance of apoptotic cells and different types of growth factors (32). Macrophages are broadly divided into two categories: M1 and M2. The former recruit immune cells to the infection site, whereas M2 macrophages activate anti-inflammatory responses. The gene expression of M1 and M2 is modulated by various transcription factors that contribute to macrophage polarization (33). M1 macrophages express high levels of pro-inflammatory cytokines such as IL-1β, IL-6, TNF-α, and reactive nitrogen, which exert a central effect on host defense against infections. Recently, macrophages have been demonstrated to be the recipient cells for exogenous exosomes and to be the direct link with serum exosomes. However, the same origin exosomes in the early stage (excessive inflammatory response) and the late stage of sepsis (immunosuppression) may have opposite effects (34). Researchers have shown that exosomal miR-30d-5p in neutrophils induces M1 macrophage polarization and initiates macrophage pyroptosis in acute lung injury caused by sepsis (35). Thus, inhibition of M1-polarized macrophages may have a significant effect on sepsis treatment.
It is well-known that eosinophils are a crucial pathological component of bronchial asthma and play a significant role in allergic diseases, parasitic diseases, and infections. Eosinophils have been found to be tissue-damaging in acute lung injury (36). After being recruited to inflammatory tissues, the number and function of eosinophils may vary depending on the environment. A recent analysis of eosinophil transcriptome in mice demonstrated that the expression profile of eosinophils was similar to other bone marrow cells, such as macrophages and neutrophils (37). Eosinophils exhibit functional heterogeneity driven by their response to different inflammatory environments. Eosinophils can also downregulate the expression of pro-inflammatory genes (such as TNF-α and IL-6) and upregulate the expression of anti-inflammatory genes (such as IL-10). They also promote the transformation of macrophages from M1 to M2 type, suggesting that eosinophils play a role in inhibiting LPS-induced lung inflammation. Contrary to the results calculated by CIBERSORT in this study, eosinophils show a decrease in numbers during severe bacterial infections. Some researchers even consider the reduction in eosinophil numbers to be indicative of an infection (38-40). The secretion of adrenal corticosteroids may increase in severe bacterial infections and inhibit the release of eosinophils.
Using LASSO and SVM-RFE algorithms, 11 and 2 characteristic genes related to sepsis were identified, respectively. NLRC4 and RGL4 were obtained through intersections to retain more reliable core characteristic genes. Moreover, ROC curves revealed that the area under the curve of the two core genes were both greater than 0.7, indicating good diagnostic value and potential as candidate diagnostic markers for sepsis. This was consistent with the qRT-PCR results obtained from the clinical participants.
Actually, nearly 200 biomarkers have been found to identify early sepsis. However, sepsis is a highly heterogeneous syndrome that cannot be completely defined by one or more biomarkers. Biomarkers, we are familiar with, such as PCT, CRP, TNF α and IL-6 have no specificity in the early diagnosis of sepsis. More and more biomarkers related to genes have been discovered, which may provide new insights for the early identification and targeted therapy of sepsis.
Inflammasomes are key orchestrators of the innate immune response. The activation of neuronal apoptosis inhibitory protein (NAIP)/NLR family caspase and the recruitment of domain-containing protein 4 (NLRC4) inflammasome may be decisive elements for constraining gram-negative bacterial infections (41), especially those associated with Salmonella typhimurium. NLRC4 is a member of the NLR family that is associated with sensing cytoplasmic receptors in bacteria. NLRC4 is a dedicated flagellin sensor in eukaryotic cells and may be composed of a variety of immune complexes (42). In contrast to its protective role, a recent study showed that NLRC4 gene silencing alleviated inflammatory reactions and reduced inflammatory cell infiltration (43). Nlrc4−/− mice showed reduced inflammation and controlled bacterial load more effectively than wild-type infected mice during gram-positive pneumonia (44), providing novel ideas for the treatment of sepsis. To date, there have been no studies examining RGL4 expression during severe infection or sepsis. A study exploring the mechanism of RGL4 in lung adenocarcinoma (45) showed that RGL4 may be involved in immune infiltration, and its decreased expression is highly correlated with various types of tumor-infiltrating immune cells, especially memory B cells, CD8+T cells, macrophages, and neutrophils. RGL4 may have potential applications in causing or evading immunosuppression, and these warrant further investigation.
Finally, using IPA, we identified 10 gene networks involving NLRC4 and RGL4, indicating that there are many immune-related pathways involved in the classic pathway, such as primary immunodeficiency signaling, T cell receptor signaling, inducible co-stimulator ligand (iCOSL) signaling in T helper cells, and CTLA4 signaling in cytotoxic T lymphocytes. Functional disease pathways mainly involve immune inflammatory pathways, such as inflammatory responses, immunological diseases, and antigen presentation. We demonstrated that NLRC4 and RGL4 can interact with other differential genes, which also suggests that the two may regulate sepsis by affecting the expression of other differential genes in addition to their own roles. For instance, CST7, CLEC5a, and NEDD4 indirectly interact with NLRC4 and RGL4 in gene networks and are also involved in immune regulation and inflammatory responses. Transcriptomic profiling has verified that neutrophil-specific upregulation of cystatin F (encoded by CST7) is a sign of acute inflammation in humans (46). CST7 is significantly upregulated in patients with sepsis compared to their expression in healthy controls (46-48). Myeloid C-type lectin 5A (CLEC5A), namely myeloid DAP12-associated lectin-1 (49), plays an important role in immune defense, resulting from innate immunity. Studies have shown that CLEC5A is a critical pattern recognition receptor in Listeria infection (a gram-positive, intracellular bacterium) (50). Activation of CLEC5A/TLR2 heterocomplexes boosts formation of inflammasomes (NALP3, NLRC4, AIM-2) and causes NET (neutrophil extracellular trap) constitution and pro-inflammatory cytokine release (50-52), and thus, may have great potential in the treatment of sepsis. Researchers have shown that the E3 ubiquitin ligase Nedd4 is a considerable negative regulator of the non-canonical inflammasome pathway. Nedd4 deficiency promotes death from sepsis and pyroptosis in mice, as a result of non-canonical inflammasome activation (53). In fact, by comparison with the normal group, the differential genes were abnormally expressed in patients with gram-negative sepsis. Target binding miRNA and lncRNA were predicted around differential genes to build ceRNA (competing endogenous RNAs). Thus, lncRNA-miRNA-mRNA interaction was obtained.
Interestingly, Akkaya et al. (54) demonstrated a novel underlying effect of NLRC4 in mediating a variety of eosinophilic functions. NLRC4−/− mice displayed a decreased number of eosinophils and impaired T helper 2 (Th2) cell responses (such as decreased secretion of IL-5 and IL-13) in a model of allergic airway disease induction. They also found that Th2 responses weakened after the transfection of EoL-1 cells with short interfering RNAs targeting human NLRC4. This suggested that high levels of eosinophils and NLRC4 are not only involved in allergic responses, but also in acute inflammation.
Sepsis begins with a burst of inflammatory cytokines and then reaches an immunosuppression stage with cytokine depletion, eventually leading to host death. There is no accurate standard for the specific course and stage of sepsis. Therefore, we can only start to evaluate a patient’s stage after the onset of the disease upon admission to hospital, which imposes limitations for this study. This approach might be reconsidered because early warning signs of sepsis are usually nonspecific, and alerting systems may show false-positive signals in multiple instances. The functional validation of these potential biomarkers for sepsis requires further experimentation. In addition, the patient cases that satisfied the Sepsis 3.0 diagnostic criteria were not strictly associated with sepsis caused by gram-negative bacterial infection (no bacterial culture results have been confirmed). Nonetheless, our verification results demonstrated a difference in key genes, which indicated that the two immune cell-related genes may have diagnostic value.
In conclusion, this study examined the infiltration of immune cells by CIBERSORT in gram-negative sepsis and provided novel insights into the molecular mechanisms of immune cells associated with the pathogenesis of gram-negative sepsis. Moreover, this current report identified NLRC4 and RGL4 as potential diagnostic biomarkers for gram-negative sepsis.
Acknowledgments
The authors wish to thank all participants for their willingness to participate in the study and their cooperation which ensured smooth conduct of the research.
Funding: This work was supported by the Capital Health Development Scientific Research Project (No. 2021-1G-2031) and the Nature Science Foundation of Beijing (No. M21023).
Footnote
Reporting Checklist: The authors have completed the STREGA reporting checklist. Available at https://atm.amegroups.com/article/view/10.21037/atm-22-3307/rc
Data Sharing Statement: Available at https://atm.amegroups.com/article/view/10.21037/atm-22-3307/dss
Conflicts of Interest: All authors have completed the ICMJE uniform disclosure form (available at https://atm.amegroups.com/article/view/10.21037/atm-22-3307/coif). The authors have no conflicts of interest to declare.
Ethical Statement: The authors are accountable for all aspects of the work in ensuring that questions related to the accuracy or integrity of any part of the work are appropriately investigated and resolved. The study was conducted in accordance with the Declaration of Helsinki (as revised in 2013). The current study on human participants was examined and approved by the Research Ethics Committee of Beijing Chaoyang Hospital, Capital Medical University (No. 2021-ke-316). The candidates provided written informed consent to participate in this study.
Open Access Statement: This is an Open Access article distributed in accordance with the Creative Commons Attribution-NonCommercial-NoDerivs 4.0 International License (CC BY-NC-ND 4.0), which permits the non-commercial replication and distribution of the article with the strict proviso that no changes or edits are made and the original work is properly cited (including links to both the formal publication through the relevant DOI and the license). See: https://creativecommons.org/licenses/by-nc-nd/4.0/.
References
- van der Poll T, van de Veerdonk FL, Scicluna BP, et al. The immunopathology of sepsis and potential therapeutic targets. Nat Rev Immunol 2017;17:407-20. [Crossref] [PubMed]
- Rudd KE, Johnson SC, Agesa KM, et al. Global, regional, and national sepsis incidence and mortality, 1990-2017: analysis for the Global Burden of Disease Study. Lancet 2020;395:200-11. [Crossref] [PubMed]
- Martin GS, Mannino DM, Eaton S, et al. The epidemiology of sepsis in the United States from 1979 through 2000. N Engl J Med 2003;348:1546-54. [Crossref] [PubMed]
- Chu LH, Indramohan M, Ratsimandresy RA, et al. The oxidized phospholipid oxPAPC protects from septic shock by targeting the non-canonical inflammasome in macrophages. Nat Commun 2018;9:996. [Crossref] [PubMed]
- Cheng N, Liang Y, Du X, et al. Serum amyloid A promotes LPS clearance and suppresses LPS-induced inflammation and tissue injury. EMBO Rep 2018;19:e45517. [Crossref] [PubMed]
- Vanaja SK, Russo AJ, Behl B, et al. Bacterial Outer Membrane Vesicles Mediate Cytosolic Localization of LPS and Caspase-11 Activation. Cell 2016;165:1106-19. [Crossref] [PubMed]
- Landesberg G, Gilon D, Meroz Y, et al. Diastolic dysfunction and mortality in severe sepsis and septic shock. Eur Heart J 2012;33:895-903. [Crossref] [PubMed]
- Baghdadi JD, Brook RH, Uslan DZ, et al. Association of a Care Bundle for Early Sepsis Management With Mortality Among Patients With Hospital-Onset or Community-Onset Sepsis. JAMA Intern Med 2020;180:707-16. [Crossref] [PubMed]
- Eitze S, Fleischmann-Struzek C, Betsch C, et al. Determinants of sepsis knowledge: a representative survey of the elderly population in Germany. Crit Care 2018;22:273. [Crossref] [PubMed]
- Li Z, Huang B, Yi W, et al. Identification of Potential Early Diagnostic Biomarkers of Sepsis. J Inflamm Res 2021;14:621-31. [Crossref] [PubMed]
- Sweeney TE, Wong HR. Risk Stratification and Prognosis in Sepsis: What Have We Learned from Microarrays? Clin Chest Med 2016;37:209-18. [Crossref] [PubMed]
- Tsalik EL, Langley RJ, Dinwiddie DL, et al. An integrated transcriptome and expressed variant analysis of sepsis survival and death. Genome Med 2014;6:111. [Crossref] [PubMed]
- Scicluna BP, Klein Klouwenberg PM, van Vught LA, et al. A molecular biomarker to diagnose community-acquired pneumonia on intensive care unit admission. Am J Respir Crit Care Med 2015;192:826-35. [Crossref] [PubMed]
- van Vught LA, Klein Klouwenberg PM, Spitoni C, et al. Incidence, Risk Factors, and Attributable Mortality of Secondary Infections in the Intensive Care Unit After Admission for Sepsis. JAMA 2016;315:1469-79. [Crossref] [PubMed]
- Davenport EE, Burnham KL, Radhakrishnan J, et al. Genomic landscape of the individual host response and outcomes in sepsis: a prospective cohort study. Lancet Respir Med 2016;4:259-71. [Crossref] [PubMed]
- Rautanen A, Mills TC, Gordon AC, et al. Genome-wide association study of survival from sepsis due to pneumonia: an observational cohort study. Lancet Respir Med 2015;3:53-60. [Crossref] [PubMed]
- Cheng SC, Scicluna BP, Arts RJ, et al. Broad defects in the energy metabolism of leukocytes underlie immunoparalysis in sepsis. Nat Immunol 2016;17:406-13. [Crossref] [PubMed]
- Holmes CL, Anderson MT, Mobley HLT, et al. Pathogenesis of Gram-Negative Bacteremia. Clin Microbiol Rev 2021;34:e00234-20. [Crossref] [PubMed]
- Tang BM, McLean AS, Dawes IW, et al. Gene-expression profiling of peripheral blood mononuclear cells in sepsis. Crit Care Med 2009;37:882-8. [Crossref] [PubMed]
- Ritchie ME, Phipson B, Wu D, et al. limma powers differential expression analyses for RNA-sequencing and microarray studies. Nucleic Acids Res 2015;43:e47. [Crossref] [PubMed]
- Newman AM, Liu CL, Green MR, et al. Robust enumeration of cell subsets from tissue expression profiles. Nat Methods 2015;12:453-7. [Crossref] [PubMed]
- Friedman J, Hastie T, Tibshirani R. Regularization Paths for Generalized Linear Models via Coordinate Descent. J Stat Softw 2010;33:1-22. [Crossref] [PubMed]
- Krämer A, Green J, Pollard J Jr, et al. Causal analysis approaches in Ingenuity Pathway Analysis. Bioinformatics 2014;30:523-30. [Crossref] [PubMed]
- Hotchkiss RS, Monneret G, Payen D. Sepsis-induced immunosuppression: from cellular dysfunctions to immunotherapy. Nat Rev Immunol 2013;13:862-74. [Crossref] [PubMed]
- Ramos-Sevillano E, Ercoli G, Brown JS. Mechanisms of Naturally Acquired Immunity to Streptococcus pneumoniae. Front Immunol 2019;10:358. [Crossref] [PubMed]
- Takaya A, Yamamoto T, Tokoyoda K. Humoral Immunity vs. Salmonella. Front Immunol 2019;10:3155. [Crossref] [PubMed]
- Barker KA, Etesami NS, Shenoy AT, et al. Lung-resident memory B cells protect against bacterial pneumonia. J Clin Invest 2021;131:141810. [Crossref] [PubMed]
- Nutt SL, Hodgkin PD, Tarlinton DM, et al. The generation of antibody-secreting plasma cells. Nat Rev Immunol 2015;15:160-71. [Crossref] [PubMed]
- Bosque A, Planelles V. Induction of HIV-1 latency and reactivation in primary memory CD4+ T cells. Blood 2009;113:58-65. [Crossref] [PubMed]
- van Leeuwen EM, Sprent J, Surh CD. Generation and maintenance of memory CD4(+) T Cells. Curr Opin Immunol 2009;21:167-72. [Crossref] [PubMed]
- Crotty S. Do Memory CD4 T Cells Keep Their Cell-Type Programming: Plasticity versus Fate Commitment? Complexities of Interpretation due to the Heterogeneity of Memory CD4 T Cells, Including T Follicular Helper Cells. Cold Spring Harb Perspect Biol 2018;10:a032102. [Crossref] [PubMed]
- Geissmann F, Manz MG, Jung S, et al. Development of monocytes, macrophages, and dendritic cells. Science 2010;327:656-61. [Crossref] [PubMed]
- Murray PJ, Allen JE, Biswas SK, et al. Macrophage activation and polarization: nomenclature and experimental guidelines. Immunity 2014;41:14-20. [Crossref] [PubMed]
- Qiu P, Zhou J, Zhang J, et al. Exosome: The Regulator of the Immune System in Sepsis. Front Pharmacol 2021;12:671164. [Crossref] [PubMed]
- Jiao Y, Zhang T, Zhang C, et al. Exosomal miR-30d-5p of neutrophils induces M1 macrophage polarization and primes macrophage pyroptosis in sepsis-related acute lung injury. Crit Care 2021;25:356. [Crossref] [PubMed]
- Willetts L, Parker K, Wesselius LJ, et al. Immunodetection of occult eosinophils in lung tissue biopsies may help predict survival in acute lung injury. Respir Res 2011;12:116. [Crossref] [PubMed]
- Dolitzky A, Shapira G, Grisaru-Tal S, et al. Transcriptional Profiling of Mouse Eosinophils Identifies Distinct Gene Signatures Following Cellular Activation. Front Immunol 2021;12:802839. [Crossref] [PubMed]
- Wibrow BA, Ho KM, Flexman JP, et al. Eosinopenia as a diagnostic marker of bloodstream infection in hospitalised paediatric and adult patients: a case-control study. Anaesth Intensive Care 2011;39:224-30. [Crossref] [PubMed]
- Abidi K, Khoudri I, Belayachi J, et al. Eosinopenia is a reliable marker of sepsis on admission to medical intensive care units. Crit Care 2008;12:R59. [Crossref] [PubMed]
- Al Duhailib Z, Farooqi M, Piticaru J, et al. The role of eosinophils in sepsis and acute respiratory distress syndrome: a scoping review. Can J Anaesth 2021;68:715-26. [Crossref] [PubMed]
- Sundaram B, Kanneganti TD. Advances in Understanding Activation and Function of the NLRC4 Inflammasome. Int J Mol Sci 2021;22:1048. [Crossref] [PubMed]
- Abdelaziz DH, Amr K, Amer AO. Nlrc4/Ipaf/CLAN/CARD12: more than a flagellin sensor. Int J Biochem Cell Biol 2010;42:789-91. [Crossref] [PubMed]
- Wang SS, Yan CS, Luo JM. NLRC4 gene silencing-dependent blockade of NOD-like receptor pathway inhibits inflammation, reduces proliferation and increases apoptosis of dendritic cells in mice with septic shock. Aging (Albany NY) 2021;13:1440-57. [Crossref] [PubMed]
- Paudel S, Ghimire L, Jin L, et al. NLRC4 suppresses IL-17A-mediated neutrophil-dependent host defense through upregulation of IL-18 and induction of necroptosis during Gram-positive pneumonia. Mucosal Immunol 2019;12:247-57. [Crossref] [PubMed]
- Sun Y, Zhang Y, Ren S, et al. Low expression of RGL4 is associated with a poor prognosis and immune infiltration in lung adenocarcinoma patients. Int Immunopharmacol 2020;83:106454. [Crossref] [PubMed]
- Sawyer AJ, Garand M, Chaussabel D, et al. Transcriptomic Profiling Identifies Neutrophil-Specific Upregulation of Cystatin F as a Marker of Acute Inflammation in Humans. Front Immunol 2021;12:634119. [Crossref] [PubMed]
- Chen H, Li Y, Li T, et al. Identification of Potential Transcriptional Biomarkers Differently Expressed in Both S. aureus- and E. coli-Induced Sepsis via Integrated Analysis. Biomed Res Int 2019;2019:2487921. [Crossref] [PubMed]
- Ahmad S, Singh P, Sharma A, et al. Transcriptome Meta-Analysis Deciphers a Dysregulation in Immune Response-Associated Gene Signatures during Sepsis. Genes (Basel) 2019;10:1005. [Crossref] [PubMed]
- Bakker AB, Baker E, Sutherland GR, et al. Myeloid DAP12-associating lectin (MDL)-1 is a cell surface receptor involved in the activation of myeloid cells. Proc Natl Acad Sci U S A 1999;96:9792-6. [Crossref] [PubMed]
- Chen ST, Li FJ, Hsu TY, et al. CLEC5A is a critical receptor in innate immunity against Listeria infection. Nat Commun 2017;8:299. [Crossref] [PubMed]
- Sung PS, Huang TF, Hsieh SL. Extracellular vesicles from CLEC2-activated platelets enhance dengue virus-induced lethality via CLEC5A/TLR2. Nat Commun 2019;10:2402. [Crossref] [PubMed]
- Aoki N, Kimura Y, Kimura S, et al. Expression and functional role of MDL-1 (CLEC5A) in mouse myeloid lineage cells. J Leukoc Biol 2009;85:508-17. [Crossref] [PubMed]
- Liu Q, Zhang S, Sun Z, et al. E3 ubiquitin ligase Nedd4 is a key negative regulator for non-canonical inflammasome activation. Cell Death Differ 2019;26:2386-99. [Crossref] [PubMed]
- Akkaya I, Oylumlu E, Ozel I, et al. NLRC4 Inflammasome-Mediated Regulation of Eosinophilic Functions. Immune Netw 2021;21:e42. [Crossref] [PubMed]
(English Language Editor: J. Teoh)