Transcriptome analysis of well-differentiated laryngeal squamous cell carcinoma cells in below-background environment
Introduction
Deep underground exploitation has now reached a depth of over 5,000 m (1), where background radiation is shielded from cosmic rays and neutrons (2). Ambient radiation is considered to impose biological changes on living organisms (3), but how living creatures respond to below-background radiation (BBR) has not yet been comprehensively evaluated.
The known ‘Linear No-Threshold’ (LNT) model, which assumes that there is a linear increase in deleterious effects with no safe radiation dose level. However, increasing evidence suggests the risks of low doses of radiation might not strictly conform to the LNT model. Thus far, many studies have observed the behavior of life in deep underground laboratories with a dramatically reduced level of environmental radiation. As described by Planel et al. (4), the inhibitory effects on paramecia were revealed in caves 200 m underground, while the growth rate was restored after irradiation by a 60Co source at a dose rate close to natural radiation. Similar evidence suggested reduced growth of Deinococcus radiodurans and Shewanella oneidensis at 650 m underneath the Waste Isolation Pilot Plant (WIPP) (5,6). Further investigation showed altered activity of antioxidant enzymes and increased spontaneous mutation frequency of Chinese hamster V79 cells (V79) within the Gran Sasso National Laboratory (LNGS) in Italy (7). This preliminary evidence suggests that decreased ionizing radiation can contribute to cellular changes in living cultures.
In 2018, we set up a deep-underground cell culture laboratory (DUGL) at the Jiapigou Minerals Limited Corporation of China National Gold Group Corporation (CJEM), reaching a depth of 1470m rocky cover, as well as an above ground laboratory (AGL) at the CJEM external site as a control (1). Initial findings indicated a slower proliferation rate of V79 cells under BBR conditions, in accordance with Satta et al.’s study (8). Another tumor cell line of well-differentiated laryngeal squamous cell carcinoma cells, namely FD-LSC-1, was also reported to have a growth deceleration. As only few studies have extended experiments in BBR, the underlying mechanisms of the growth inhibition phenomenon on mammalian cells remain to be clarified, and thus understand the impact of low dose radiation on cell behaviors.
Hence, we cultured FD-LSC-1 cells, which are moderately sensitive to radiation, in both the DUGL and AGL simultaneously. Whole-transcriptome analysis of FD-LSC-1 cells was performed to obtain regulation pathways and factor genes concerning the mechanisms of cellular responses to low radiation levels.
The findings reported here provide new insights into the adaptations of short-term cell growth in BBR and further integrated differential expressed genes of FD-LSC-1 cells that may facilitate delayed tumor cell proliferation. Our data also give direction to deep underground space development and advancing this field of research. Raw sequence data has been submitted in the Sequence Read Archive at NCBI under accession code PRJNA799455. We present the following article in accordance with the MDAR reporting checklist (available at https://atm.amegroups.com/article/view/10.21037/atm-22-2997/rc).
Methods
Cell culture
The human cancer cell line FD-LSC-1 was obtained from the Chinese Academy of Science (Shanghai, China). The cells were maintained in Dulbecco’s modified Eagle’s medium (DMEM, Gibco, USA) with 10% fetal bovine serum (Gemini, USA) and 1% penicillin and streptomycin (Gibco, USA) solution. Cells at 80% confluency in 75 cm2 bottom flasks were assigned randomly into the DUGL or AGL for further incubation at 37 ℃ and 5% CO2. The DUGL provided a low radiation environment whilst the AGL served as the control. Both cell groups were cultured simultaneously for 4 days, after which they were harvested.
RNA preparation
Total RNA was extracted from cultured samples using Trizol reagent (Invitrogen, NY, USA). The RNA quantity was verified spectrophotometrically with a NanoDrop-2000 spectrometer (NanoDrop Technologies, DE, USA) according to the manufacturer’s instructions. The integrity of RNA was confirmed with 1% agarose gel and electrophoretically with a 2100 Bioanalyzer (Agilent Technologies, CA, USA). Briefly, ribosomal RNA (rRNA) was removed using Ribo-Zero™ GoldKits (Epicentre, WI, USA). Only samples with an RNA integrity number (RIN) more than 7.0 were acceptable for RNA library preparation.
Whole-transcriptome sequencing (RNA-Seq)
RNA fragmentation was performed followed by conversion of the rRNA-depleted RNA into single-stranded complementary DNA (cDNA). Subsequently, 3 µg of total RNA from each sample was used to construct the cDNA library (NEB Next Ultra Directional RNA LibraryPrep Kit for Illumina, Ispawich, USA). First-strand cDNA was synthesized using random hexamer primers while second-strand cDNA was generated using DNA Polymerase I and RNase H. After quality control, adapter ligation, and polymerase chain reaction (PCR) procedures, samples of total RNA from FD-LSC-1 cells were sequenced on NovaSeq 6000 Illumina equipment (Illumina, San Diego, CA, USA) in paired-end mode.
RNA-Seq data processing
The sequencing data was analyzed through CASAVA software for base calling and raw data were transferred into FASTQ stored files. Reads with low quality data, N ratio more than 5%, adapter sequences, and rRNA were filtered out from all count data. As for microRNAs (miRNAs), reads without 3’linker sequence or reads with ployA/T were excluded as well.
Clean reads of messenger RNA (mRNA) and long non-coding RNA (lncRNA) were mapped to a reference genome (GRCh38 (GCF_000001405.26), RefSeq assembly accession) (9) by HiSAT2 software. We subsequently used Coding-Non-Coding Index (CNCI), Coding Potential Calculator 2 (CPC2), and Coding Potential Assessment Tool (CPAT) to identify the potential coding ability of genes. The assembled transcripts without coding potential were the candidate set of lncRNAs.
The trimmed reads of miRNAs were aligned to the reference genome using Bowtie (10). Mapped reads to mature miRNAs in miRBase (release 21) were used to identify known miRNAs (11). The tool used for the identification and prediction of miRNAs was miRDeep2 software (12). Transcript per million (TPM) was implemented to determine the expression levels of miRNAs.
Sequence reads of circular RNAs (circRNAs) were mapped against the reference genome using BWA-MEM (13), and circRNA Identifier (CIRI) (14) was applied for efficient recognition. In addition, the expression levels of circRNAs were normalized via spliced reads per billion mapping (SRPBM) according to back-splicing reads.
Differential expression analysis
The DESeq2 package for R software (v3.5.1, 2018) was used to perform differential expression analysis of 2 conditions (DUGL and AGL) based on the count data from HTSeq (15). Fragments per kilobase million (FPKM) mapped reads were used to estimate gene expression levels using StringTie (16). The statistical significance criteria of |log2(fold-change)| ≥1.0 and q value (adjusted P value) of less than 0.05 for gene expression levels determined differentially expressed (DE) factors, which was used for bioinformatics analyses. The false discovery rate (FDR) was controlled by the Benjamini-Hochberg algorithm (17) to adjusted P values. Hierarchical clustering analysis for the expression profiles was performed using the “pheatmap” package from R software. The distributions of DE RNAs were visualized by a volcano plot with the “ggplot2” package. Functional enrichment based on Gene Ontology (GO, http://www.geneontology.org/) was performed with GeneCodis3, where the gene sets were separated in accordance with the GO terms for biological processes (BPs), cellular components (CCs), and molecular functions (MFs). Pathway analysis was performed using the Kyoto Encyclopedia of Genes and Genomes (KEGG) pathway database to detect potential targets of DE genes between the DUGL and AUL groups. Both GO terms and KEGG pathways with corrected P values less than 0.05 were considered to be significantly enriched.
Construction of co-expression and Bayesian network analysis
Co-expression levels of significantly regulated mRNAs were computed using Stringdb of R software (18), and gene-pairs were selected by setting combined score >0.9 for the causality network construction. We further implemented the bnlearn algorithm with Cytoscape (3.7.2) (19) to visualize the Bayesian network (200 iterations) (20) with mRNAs in selected nodes screened by degree >2 and weight >0.2.
Real-time quantitative polymerase chain reaction (qRT-PCR)
To validate the gene expression patterns detected by RNA-Seq analysis, differentially expressed genes (DEGs) from transcriptome sequencing were randomly selected for assessment through qRT-PCR including mRNAs, lncRNAs, circRNAs, and miRNAs. The PrimeScript RT Reagent Kit with gDNA Eraser (RR047A, Takara, Japan) was used to synthesize cDNA from extracted total RNA. Reactions were performed on a 7500 real-time system (Applied Biosystems, USA). Relative quantification was normalized against the housekeeping gene GAPDH for mRNAs, and β-actin for the others, by using the 2-ΔΔCT method (21). The forward primer sequence of GAPDH was 5'-GATCTGGCACCACACCTTCT-3' and the reverse primer sequence was 5'-GGGGTGTTGAAGGTCTCAAA-3'. The forward primer sequence of β-actin: 5'-ATAGCACAGCCTGGATAGCAACGTAC-3' and the reverse primer sequence was: 5'-CACCTTCTACAATGAGCTGCGTGTG-3'. More than 3 respective sets of experiments were performed.
Statistical analysis
For the sequencing data, the statistical significance criteria of |log2(fold-change)| ≥1.0 and q value (adjusted P value) of less than 0.05 for gene expression levels determined differentially expressed (DE) factors. The FDR was controlled by the Benjamini-Hochberg algorithm (17) to adjusted P values. For the RT-PCR, the data are expressed as the mean ± standard error of the mean (SEM). The statistical analysis was performed using GraphPad Prism version 9.0 (GraphPad Software Inc., San Diego, CA, USA).
Results
Overview of genome-wide analyses
Following sequencing on an Illumina MiSeq, total raw reads were obtained per sample after removal of adaptors, ambiguous reads, and low quality reads. The number of reads for mRNAs, lncRNAs, and circRNAs was 90 million reads on average, with over 90% mapping to the genome, while reads of miRNAs were much lower, with 8–11 million reads and 65% mapping on average. A summary of the sequencing results, mapping quality, and mapping rate are outlined in Table S1.
In all, we identified 1,458 DE RNA transcripts (q value <0.05 and |log2FC| ≥1.0) in FD-LSC-1 cell samples grown in the DUGL and AGL for 4 days (Figure 1A-1C). Among them, there were 671 mRNAs, 286 lncRNAs, 489 circRNAs, and 6 miRNAs present in the transcriptome database (GENCODE, Ensembl, and NCBI). Of these variably expressed coding genes, 465 genes were up-regulated, and 206 genes were down-regulated between the 2 cell groups. Specifically, 200 up-regulated and 86 down-regulated lncRNAs were detected, while the numbers of corresponding significantly regulated circRNAs were 285 and 204, respectively. Only 4 up-regulated and 2 down-regulated miRNAs were identified, but none were novel (Table 1).
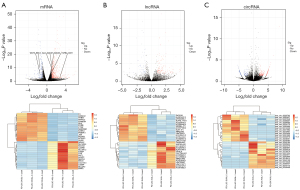
Table 1
RNAs | Up-regulated | Down-regulated | Total |
---|---|---|---|
mRNAs | 465 | 206 | 671 |
lncRNAs | 200 | 86 | 286 |
circRNAs | 285 | 204 | 489 |
miRNAs | 10 | 2 | 12 |
Total | 960 | 498 | 1,458 |
DUGL, deep underground laboratory; AGL, above ground laboratory.
Functional enrichment analysis of DEGs
The most abundant GO terms for BP were cell surface receptor signaling pathway (GO:0007166), tissue development (GO:0009888), and positive regulation of developmental process (GO:0051094) (Figure 2). Up-regulated genes were mainly involved in biological regulation (GO:0065007), response to stimulus (GO:0050896), regulation of biological process (GO:0050789), and regulation of cellular process (GO:0050794). In the CCs category, DEGs were enriched in 38 terms, and the top 3 were plasma membrane (GO:0005886), cell periphery (GO:0071944), and extracellular region (GO:0005576) (Figure 2B). For MFs, up-regulated genes were prominently enriched in terms of receptor binding (GO:0005102), transcription regulatory region DNA binding (GO:0044212), and regulatory region nucleic acid binding (GO:0001067), similar to the down-regulated genes (Figure 2C). These MF terms are general and did not suggest that the effects of BBR trigger a wide range of cellular responses. In the KEGG pathway enrichment analysis, up-regulated genes were significantly enriched in the Hippo signaling pathway (map04390) and pathways in cancer (map05200), indicative of cell proliferation or apoptosis in response to radiation reduction (Figure 2D).
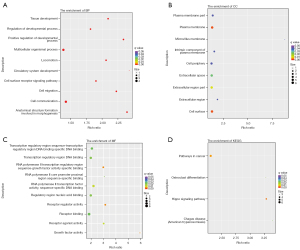
Functional enrichment analysis of DE lncRNAs
Metabolic process (GO:0008152) was one of the most representative subcategory GO terms under the BP category, which involved regulation of cellular metabolic process (GO:0031323), primary metabolic process (GO:0080090), nitrogen compound metabolic process (GO:0051171), nucleobase-containing compound metabolic process (GO:0034654), and RNA metabolic process (GO:0051252). Other important BP terms were also linked to the biosynthetic process of heterocycle (GO:0018130), nucleobase-containing compound (GO:0034654), aromatic compound (GO:0019438), and organic cyclic compound (GO:1901362) (Figure 3A). Accordingly, the results of genes enriched in the CC category were intracellular membrane-bounded organelle (GO:0043231), nucleoplasm (GO:0005654), intracellular part (GO:0044424), and intracellular (GO:0005622) (Figure 3B). Within the MF category, regulated genes were prominently enriched in the terms of transferase activity (GO:0016740), catalytic activity (GO:0003824), protein binding (GO:0005515), and DNA binding (GO:0003677) (Figure 3C). Then, we determined the 17 KEGG pathways that were significantly enriched in response to different radiation backgrounds. Metabolic and proliferative pathways (e.g., pathways in cancer, lysosome, amino sugar and nucleotide sugar metabolism, viral carcinogenesis, basal cell carcinoma, focal adhesion, Wnt signaling pathway, Hippo signaling pathway) were partially overlapped with the KEGG analysis outcomes of DEGs (Figure 3D).
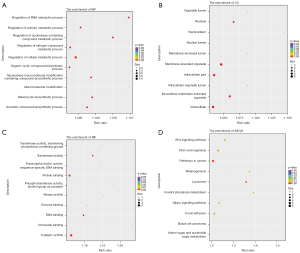
In the comparison of the overall GO enrichment, the host genes of circRNAs were mostly enriched in macromolecule modification (GO:0043412), protein modification process (GO:0036211), cellular protein modification process (GO:0006464), cell cycle (GO:0007049), and cellular response to stress (GO:0033554) (Figure 4). The cell cycle item needed further exploration, since this process was closely related to proliferation and apoptosis. A total of 50 terms in the CC category were significantly enriched, including intracellular (GO:0005622), intracellular part (GO:0044424), nuclear part (GO:0044428), intracellular organelle (GO:0043229), and intracellular membrane-bounded organelle (GO:0043231) (Figure 4B). The highest enrichment of the MF category was associated with catalytic activity (GO:0003824), while others were protein binding (GO:0005515) and transferase activity (GO:0016740), among others (Figure 4C). KEGG analysis referred to the lysine degradation (hsa00310) pathway which was significantly enriched, including 5 up-regulated and 2 down-regulated circRNAs (Figure 4D).
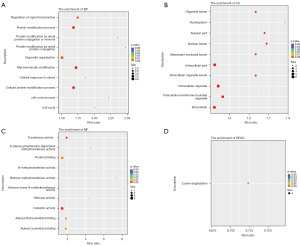
Co-expression and Bayesian causal network analysis
We performed co-expression network analysis based on the sequencing results to detect mRNA modules, of which 133 of 671 filtered target genes were significantly correlated and 192 interaction pairs were recognized by combined score >0.9 (Figure 5A). Among them, most connections with other members of the module were SMAD family member 3 (SMAD3), SMAD family member 7 (SMAD7), bone morphogenetic protein 2 (BMP2), Jun proto-oncogene (JUN), cadherin 1 (CDH1), early growth response 1 (EGR1), and fibroblast growth factor 2 (FGF2). According to screening based on degree >2 and weight >0.2, 49 candidate genes were further applied for Bayesian causal network construction, which depicted that the most significantly correlated hub genes were mitogen-activated protein kinase 13 (MAPK13), ETS1, SRY-box transcription factor 9 (SOX9), neurotensin receptor 1 (NTSR1), and SMAD7 (Figure 5B).
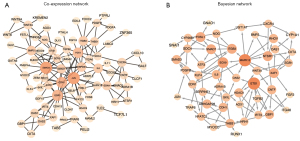
Verification of DEGs by qRT-PCR
To validate the gene expression profiles obtained by RNA-Seq analysis, we randomly assayed genes from each mRNA, lncRNA, miRNA and circRNA list for qRT-PCR verification. Consequently, these selected genes demonstrated concordant expression patterns between the RNA-Seq and qRT-PCR results (Figure 6A-6D). The primers are listed in Tables 2-5.
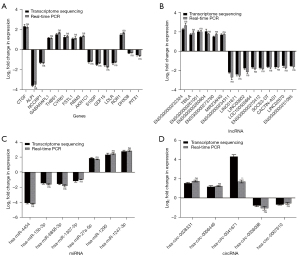
Table 2
Gene name | F-primer | R-primer | RNA-Seq | qPCR |
---|---|---|---|---|
CTGF | TTACCAATGACAACGCCTCC | GATGCACTTTTTGCCCTTCTTA | 2.310 | 2.35362 |
ALPI | CCATCTTCGGGTTGGCCCC | CTCTCATTCACGTCTGGTCGC | −3.713 | −3.716051 |
NCCRP1 | CATGGACTGGTTCGAGGACAG | TAATGGCGGAATACGTGGGA | −1.199 | −1.231767 |
GABARAPL1 | CCTTACTGTTGGCCAGTTCTA | CTCATCACTGTAGGCCACATA | 1.107 | 1.0973112 |
THBS1 | AAGACGCCTGCCCCATCAAT | GTTGTTGCAGAGACGACTACG | 1.164 | 1.6176506 |
CYR61 | GGGTCTGTGACGAGGATAGT | ATTCCAAAAACAGGGAGCCG | 1.213 | 1.2392157 |
FSTL1 | CCAGACCACGATGTGGAAACG | GCCTCTTGTGAGGTTTGCAT | 1.018 | 1.0304913 |
RBM3 | GGGCTCAACTTTAACACCGA | CATCCAGAGACTCTCCGTTC | 1.280 | 1.2968883 |
AKR1C1 | CTTGATATTTTTGCTGGCCCC | GCTGAAATCACCAAGCAGGA | −1.14 | −1.118154 |
S100P | AGGTGGGTCTGAATCTAGCA | GTCTTTTCCACTCTGCAGGAA | −1.396 | −1.391631 |
GDF15 | AGTTGCACTCCGAAGACTCC | AGCCGCACTTCTGGCGT | −1.646 | −1.668346 |
LDLR | TGGGGGTCTTCCTTCTATGG | CCATCTGTCTCGAGGGGTAG | −1.213 | −1.198412 |
ROR1 | TCTCAAGTGAACTCAACAAAGATTC | GAGGTGGATTCCCAGAGACT | 1.575 | 1.7494217 |
DHX38 | CTCTATGGTAGCTTTGGGCG | GGGATTTCTCTCACCAAGCG | −0.337 | −0.306457 |
PITX1 | CCAGCCAAGAAGAAGAAGCA | TTCTTGAACCAGACCCGCA | −0.642 | −0.603244 |
FC, fold change; PCR, polymerase chain reaction; DUGL, deep underground laboratory; AGL, above ground laboratory; qPCR, quantitative Real-time PCR.
Table 3
Gene name | F-primer | R-primer | RNA-Seq | qPCR |
---|---|---|---|---|
ENSG00000232324 | GGAATAACACACCCTCCCTC | AGAGGAAGGTAGAGCCTGTG | 2.3981793 | 2.4598464 |
TBILA | GTTGTTTCCAGTTTGGTCACT | GCAGTCCTGTATCTGCTTTTC | 1.8199685 | 1.9473007 |
ENSG00000261051 | CACGTCCTAGTGGTTTAGAGG | CTTCCCGAGGTCACACAAAG | 2.0321083 | 1.8752409 |
ENSG00000260604 | AGCTGTTTCCAAAGACACCC | CAGTGAGAGATTCACAGCCC | 2.0695075 | 1.8526164 |
ENSG00000273760 | CACGCCACTGCCTTCTC | CTGTGTGCTTGGAAGAGTGT | 1.5833876 | 1.7194941 |
MIR23AHG | GTAACTGGCTGCTAGGAAGG | CCAGCATAGATAGGTGGGTG | 1.7100933 | 1.6874927 |
ENSG00000234311 | GGACACGGACCTAGACACT | CTGACCTGCAAGACCGTAG | −2.2794272 | −2.8774890 |
LINC01671 | AGCCTTGGCAAACTCCAAGA | GACATCTGAACCCAATTCAGGA | −2.4803996 | −2.3549789 |
LINC00602 | TTGTGCTCTCAGGAACGACT | GTTCTGGCAACGAGGCTAC | −1.8677201 | −2.0195099 |
LOC100505664 | TCTGCTAGGACTTCTGCCAT | CTGGAAACTGCTGAGCCAT | −1.7630108 | −1.9146524 |
ENSG00000270412 | GTGCTTTCTTGCGGGTCAG | GCTGCCTTATGTAACCTGCGA | −1.8504048 | −1.7674258 |
SOCS3-DT | TTGAGGGCTCAGGAGCTATAC | CTCTGGAGCGTACCCTGT | −1.7010041 | −1.7056152 |
CACTIN-AS1 | CACGGGGAGGAAACTGAGG | GGCACAGTAAAGGGGCTTC | −1.6376511 | −1.5169541 |
LINC02870 | TGTGAACAGGAAGCTGAGAAC | GAGGCTTCTCTGGGTATAAAGC | −1.3689746 | −1.5157920 |
ENSG00000251095 | AGGTAGTCGTACAGTGTCCA | GTCTTTGGTGAGTCTCTCGGA | −1.5540582 | −1.5641373 |
FC, fold change; PCR, polymerase chain reaction; DUGL, deep underground laboratory; AGL, above ground laboratory; qPCR, quantitative real-time PCR.
Table 4
Gene name | Primer | RNA-Seq | qPCR |
---|---|---|---|
hsa-miR-4454 | GTACACTTAGGCCGGATCCGA | −4.191426 | −4.1926645 |
hsa-miR-15b-3p | GGCGAATCATTATTTGCTGCTCTA | −1.5221586 | −1.5908128 |
hsa-miR-6858-3p | CCAGCCCCTGCTCACCCCT | −1.9512010 | −1.4481064 |
hsa-miR-1307-5p | TCGACCGGACCTCGACCG | −0.9050458 | −1.1267616 |
hsa-miR-27a-5p | AGGGCTTAGCTGCTTGTGAGC | 1.8772767 | 1.8814850 |
hsa-miR-1290 | GCGCCGTGGATTTTTGGAT | 2.5306787 | 2.5546392 |
hsa-miR-1247-3p | CGGGAACGTCGAGACTGGAGC | 2.9733626 | 2.9044312 |
FC, fold change; PCR, polymerase chain reaction; DUGL, deep underground laboratory; AGL, above ground laboratory; qPCR, quantitative real-time PCR.
Table 5
Gene name | F-primer | R-primer | RNA-Seq | qPCR |
---|---|---|---|---|
hsa_circ_0028331 | ACGACAAACGCTCGGGCTTC | GCGGACCTTGTTGCTCTTGA | 1.54772896 | 1.8758754 |
hsa_circ_0006446 | TCTACCTCACGATGCTCCTCT | GCAGCCAAAACTCGGGACT | 1.18693227 | 1.1162001 |
hsa_circ_0041671 | GGTTCCAACAGTCATGCCAAA | GTGCCCAAAGTGGGTTATATGG | 4.54034592 | 1.9645740 |
hsa_circ_0036098 | CTGTGTGAAGGCCCAGACATT | GCCCATTTTCCAGACGAACAG | −0.73249871 | −0.932299 |
hsa_circ_0007610 | CAGTCATGGCCAAGGTTAACAA | TGTCTTCATCTGAATCATCTTCCC | −0.63495933 | −0.5582185 |
FC, fold change; PCR, polymerase chain reaction; DUGL, deep underground laboratory; AGL, above ground laboratory; qPCR, quantitative real-time PCR.
Discussion
Exploring life underground was once unimaginable, however, a series of evolution experiments and cultures of model organisms have become achievable at hundreds or even thousands of meters underground. Repeated observations regarding the delayed growth rate of prokaryotes, eukaryotes, and Drosophila from different studies indicate that living systems somehow adapt to the low radiation environment by cell population changes (22).
In our previous work, the growth reduction of V79 and FD-LSC-1 cells was observed compared to cultures grown at a surface radiation level (23). As levels of relative humidity, temperature, and carbon dioxide (CO2) concentration remained the same at both the DUGL and AGL incubators, reduced cell growth can be traced to radiation exposure, given that the total gamma (γ) radiation dose rate was estimated to be extremely low (0.035–0.045 µSv/h) in the DUGL (23). The stimulatory effect and potential mechanisms of cellular alterations in response to low radiation stress are still not well known; therefore, our study expected to unveil the underlying mechanisms contributing to the impeded growth rate of FD-LSC-1 cells at the gene regulation level.
Our findings identified 671 coding genes and 762 non-coding RNAs as significantly expressed among samples under different environmental stress. The results of the transcriptomic analyses displayed distinct genetic profiles when compared to cells grown in the AGL, with a majority of transcripts up-regulated. Particularly, a few genes, being proven to negatively regulate proliferation, were identified as up-regulated, such as testin (TES) (24) and headcase protein homolog (HECA) (25). Other genes that have central roles in proliferation and the cell cycle were also found to be differentially expressed (i.e., fibroblast growth factor 18, FGF18; ski-like protein, SKIL) (26,27). While some results might remain controversial, the changes in gene profiles predicted a trend that BBR could contribute to delayed growth. Also, we consistently observed DEGs associated with the flux of numerous amino acids (28), such as metabolism of tyrosine (tyrosine-protein kinase ABL2), histidine (phosphoglycerate mutase 1, PGAM1), and catabolism of arginine (protein-arginine deiminase type-1, PADI1), suggesting amino acid modulation in response to the stress of reduced environmental radiation. As noted, we identified 6 DE miRNAs, but none were novel or involved in functional enrichment.
GO analyses were subsequently implemented for the whole transcriptome. In the BP category, up-regulation of coding genes were mainly enriched in biological regulation and response to stimulus, indicating that these genes may be potentially targeted for stress responses and cell growth. Similar to our previous study, significant enrichment in metabolic process categories was detected among identified DE lncRNAs (29). Consistent with the above, modification process, cellular response to stress, and the cell cycle were representative items of the host genes of circRNAs, which implied interactions with mRNAs and lncRNAs under an ionizing radiation-deprived environment.
The KEGG analysis indicated significant enrichment of DEGs within the Hippo signaling pathway and pathways in cancer, which might play a prominent role in the suppression of tumor cells underground in a short duration. Some evidence suggests that the genes enriched in both pathways, such as SMAD3, CDH1, TGFβ2 (transforming growth factor beta 2), Wnt family member 6 (Wnt6), and GLI family zinc finger 2 (GLI2), have functions in proliferation and cell growth. CDH1, known as a tumor suppressor, is implicated in maintaining genomic stability and restraining cancer progression, which was highly expressed in FD-LSC-1 cells underground (30). Notably, unlike our preliminary observation of V79 cells (29), most of the enriched candidates were up-regulated, whereas only the expression level of BCL2 binding component 3 (BBC3), Wnt6, and BMP2 were decreased in the Hippo signaling pathway. Exposure to ionizing radiation can induce transcription of BBC3, causing cell apoptosis in response to DNA-damaging stimuli (31). Based on our results, BBC3 expression was decreased in tumor cells, which might be attributed to the reduced γ-radiation environment. It is also reported that Wnt6 and BMP2 are overexpressed in several malignancies (32,33). Conversely, their expression was decreased in FD-LSC-1 cells cultured in the DUGL, which suggested that Wnt6 and BMP2 might be involved in the growth repression caused by a low radiation environment.
Additionally, lncRNAs have evidently shown the ability to modulate proliferation, the cell cycle, and other physiological activities of cells (34). KEGG analysis uncovered target mRNAs of lncRNAs that were enriched in several pathways linked to proliferation and metabolism, but this was unsurprising since many were cancer-related pathways as well. Apart from the pathways in cancer and Hippo signaling, as both were consistent with the aforementioned outcomes of DEGs, we also focused on pathways of lysosomes, focal adhesion, as well as amino sugar and nucleotide sugar metabolism that were well represented in identified lncRNAs. Lysosomes are crucial organelles that function as metabolic signaling hubs and have been reported to integrate different environmental signals to regulate metabolic pathways (35). We speculated that adaptive responses may rely on the focal adhesion (FA) pathway due to environmental stress, as assembly of these structures are cell mechanosensitive (36).
Of the dysregulated circRNAs in our study, KEGG mapping revealed lysine degradation was the only significant pathway. There seems to be no direct evidence to explain the cellular changes within the BBR environment, but activation of lysine metabolism, especially degradation of lysine, is correlated with cell proliferation impairment of tumors (37). Considering the limited data in this study, further investigation should be undertaken to confirm the functional role of lysine metabolism in the DUGL.
In further network analysis, we observed co-expressed gene modules concerning a variety of signaling pathways that are likely to be responsible for the regulation of cell proliferation and the cell cycle. Of the hub genes identified, SMAD3 was markedly up-regulated in DUGL cultures, functioning as a tumor suppressor and as a primary mediator in TGF-β signaling (38). Importantly, our findings indicated that SMAD3, along with other regulated genes such as SMAD7, BMP2, and yes-associated protein (YAP), was closely related to either the canonical TGF-β or Hippo signaling, whereby shielded radiation might potentially lead to the outcome of FD-LSC-1 cell growth reduction in the DUGL. Overexpression of SMAD7, as shown in our study, was found to be a potent inhibitory regulator in TGF-β-induced proliferation (39). The antagonistic effect is triggered by inhibiting SMAD2 and SMAD3 (SMAD2/3) phosphorylation, and thus the SMAD2/3 protein fails to form a complex with SMAD4 that translocates into the nucleus to control gene transcription of proliferation and other cellular processes (40,41). Previous research has confirmed that the activation of SMAD2/3 also participated in crosstalk with Hippo signaling when SMAD2/3 bound to the pathway transducer YAP, leading to cell growth regulation (42). Likewise, stimulating BMP2 can directly interact with the SMAD complex and subsequently promotes nuclear translocation to regulate target genes (33). The expression level of YAP trended upwards in contrast to cultures grown in parallel, although failed to reach statistical difference. Further research on the interaction between TGF-β and the Hippo pathway is necessary to explore how they modulate cell populations in a radiation-deprived background.
Admittedly, our study has some limitations. One concern about the findings was the short-term incubation time (4 days) of cell samples, leading to limited long-term information of mechanisms under low-dose radiation. A lack of metabolomics and phosphoproteomics data limited our ability to conduct ‘omics integrative analyses which can provide greater insight and better characterization of biological effects in the DUGL. Notwithstanding these limitations, our study does suggest potential mechanisms of the environment-specific adaptations of FD-LSC-1 cells. Further study should not be confined to cell cultures, and in-depth experiments and animal models are necessary in the long term to answer whether low doses are beneficial for cancer patients.
Conclusions
Overall, the present study describes gene profiling of FD-LSC-1 cells cultured in different background radiation conditions and interprets the potential mechanisms of their environmental stress responses. The identified RNAs were involved in several proliferative and metabolic pathways that may induce proliferation differences of tumor cells caused by the physical environment. These findings contribute to understanding the implication of reduced radiation on living organisms and challenge the conventional perception that radiation only has detrimental effects. To further substantiate the suppressive growth effects, long-term observations underground will be conducted, which may help us contend with cancer progression as well.
Acknowledgments
We appreciate Chairman Zhiliang Wu and his colleagues for providing us with the experimental site at Erdaogou mine from Jiapigou Minerals Limited Corporation of China National Gold Group Corporation. We also thank Jisi Chen from Jiguang Gene Company for collaboration in transcriptional sequencing and bioinformatics analysis of all samples.
Funding: This work was supported by Chengdu Science & Technology grants (No. 2021-YF05-01024-SN), fund of Sichuan Provincial Science & Technology Department (No. 2021YJ0231), “1·3·5” Project (Nos. ZYJC18016 and ZYJC21048) provided by West China Hospital, Fundamental Research Funds for the Central Universities (No. 2022SCU12059), and the research fund of Health Commission of Sichuan Province (No. 20PJ029).
Footnote
Reporting Checklist: The authors have completed the MDAR reporting checklist. Available at https://atm.amegroups.com/article/view/10.21037/atm-22-2997/rc
Data Sharing Statement: Available at https://atm.amegroups.com/article/view/10.21037/atm-22-2997/dss
Conflicts of Interest: All authors have completed the ICMJE uniform disclosure form (available at https://atm.amegroups.com/article/view/10.21037/atm-22-2997/coif). The authors have no conflicts of interest to declare.
Ethical Statement: The authors are accountable for all aspects of the work in ensuring that questions related to the accuracy or integrity of any part of the work are appropriately investigated and resolved.
Open Access Statement: This is an Open Access article distributed in accordance with the Creative Commons Attribution-NonCommercial-NoDerivs 4.0 International License (CC BY-NC-ND 4.0), which permits the non-commercial replication and distribution of the article with the strict proviso that no changes or edits are made and the original work is properly cited (including links to both the formal publication through the relevant DOI and the license). See: https://creativecommons.org/licenses/by-nc-nd/4.0/.
References
- Liu J, Ma T, Liu Y, et al. History, advancements, and perspective of biological research in deep-underground laboratories: A brief review. Environ Int 2018;120:207-14. [Crossref] [PubMed]
- Wadsworth J, Cockell CS, Murphy AS, et al. There's plenty of room at the bottom: low radiation as a biological extreme. Front Astron Space Sci 2020;7:50. [Crossref]
- Pirkkanen J, Zarnke AM, Laframboise T, et al. A Research Environment 2 km Deep-Underground Impacts Embryonic Development in Lake Whitefish (Coregonus clupeaformis). Front Earth Sci 2020;8:327. [Crossref]
- Planel H, Soleilhavoup JP, Tixador R, et al. Influence on cell proliferation of background radiation or exposure to very low, chronic gamma radiation. Health Phys 1987;52:571-8. [Crossref] [PubMed]
- Smith GB, Grof Y, Navarrette A, et al. Exploring biological effects of low level radiation from the other side of background. Health Phys 2011;100:263-5. [Crossref] [PubMed]
- Castillo H, Schoderbek D, Dulal S, et al. Stress induction in the bacteria Shewanella oneidensis and Deinococcus radiodurans in response to below-background ionizing radiation. Int J Radiat Biol 2015;91:749-56. [Crossref] [PubMed]
- Fratini E, Carbone C, Capece D, et al. Low-radiation environment affects the development of protection mechanisms in V79 cells. Radiat Environ Biophys 2015;54:183-94. [Crossref] [PubMed]
- Satta L, Antonelli F, Belli M, et al. Influence of a low background radiation environment on biochemical and biological responses in V79 cells. Radiat Environ Biophys 2002;41:217-24. [Crossref] [PubMed]
- Phan L, Hsu J, Tri LQ, et al. dbVar structural variant cluster set for data analysis and variant comparison. F1000Res 2016;5:673. [Crossref] [PubMed]
- Langmead B. Aligning short sequencing reads with Bowtie. Curr Protoc Bioinformatics 2010;Chapter 11:Unit 11.7.
- Griffiths-Jones S, Saini HK, van Dongen S, et al. miRBase: tools for microRNA genomics. Nucleic Acids Res 2008;36:D154-8. [Crossref] [PubMed]
- Friedländer MR, Mackowiak SD, Li N, et al. miRDeep2 accurately identifies known and hundreds of novel microRNA genes in seven animal clades. Nucleic Acids Res 2012;40:37-52. [Crossref] [PubMed]
- Li H. Aligning sequence reads, clone sequences and assembly contigs with BWA-MEM. Cambridge: Broad Institute of Harvard and MIT. 2013.
- Gao Y, Wang J, Zhao F. CIRI: an efficient and unbiased algorithm for de novo circular RNA identification. Genome Biol 2015;16:4. [Crossref] [PubMed]
- Anders S, Huber W. Differential expression analysis for sequence count data. Genome Biol 2010;11:R106. [Crossref] [PubMed]
- Pertea M, Pertea GM, Antonescu CM, et al. StringTie enables improved reconstruction of a transcriptome from RNA-seq reads. Nat Biotechnol 2015;33:290-5. [Crossref] [PubMed]
- Benjamini Y, Hochberg Y. Controlling the false discovery rate: A practical and powerful approach to multiple testing. Journal of the Royal Statistical Society. Series B (Methodological) 1995;57:289-300. [Crossref]
- Szklarczyk D, Franceschini A, Wyder S, et al. STRING v10: protein-protein interaction networks, integrated over the tree of life. Nucleic Acids Res 2015;43:D447-52. [Crossref] [PubMed]
- Morris JH, Kuchinsky A, Ferrin TE, et al. enhancedGraphics: a Cytoscape app for enhanced node graphics. F1000Res 2014;3:147. [Crossref] [PubMed]
- Puga JL, Krzywinski M, Altman N. Points of Significance. Bayesian networks. Nat Methods 2015;12:799-800. [Crossref] [PubMed]
- Livak KJ, Schmittgen TD. Analysis of relative gene expression data using real-time quantitative PCR and the 2(-Delta Delta C(T)) Method. Methods 2001;25:402-8. [Crossref] [PubMed]
- Esposito G, Anello P, Ampollini M, et al. Underground Radiobiology: A Perspective at Gran Sasso National Laboratory. Front Public Health 2020;8:611146. [Crossref] [PubMed]
- Liu J, Ma T, Gao M, et al. Proteomic Characterization of Proliferation Inhibition of Well-Differentiated Laryngeal Squamous Cell Carcinoma Cells Under Below-Background Radiation in a Deep Underground Environment. Front Public Health 2020;8:584964. [Crossref] [PubMed]
- Popiel A, Kobierzycki C, Dzięgiel P. The Role of Testin in Human Cancers. Pathol Oncol Res 2019;25:1279-84. [Crossref] [PubMed]
- Son HJ, Choi EJ, Yoo NJ, et al. Mutational and expressional alterations of a candidate tumor suppressor HECA gene in gastric and colorectal cancers. Pathol Res Pract 2020;216:152896. [Crossref] [PubMed]
- Farooq M, Khan AW, Kim MS, et al. The Role of Fibroblast Growth Factor (FGF) Signaling in Tissue Repair and Regeneration. Cells 2021;10:3242. [Crossref] [PubMed]
- Yang C, Zhang Z, Ye F, et al. FGF18 Inhibits Clear Cell Renal Cell Carcinoma Proliferation and Invasion via Regulating Epithelial-Mesenchymal Transition. Front Oncol 2020;10:1685. [Crossref] [PubMed]
- Castillo H, Li X, Schilkey F, et al. Transcriptome analysis reveals a stress response of Shewanella oneidensis deprived of background levels of ionizing radiation. PLoS One 2018;13:e0196472. [Crossref] [PubMed]
- Duan L, Jiang H, Liu J, et al. Whole Transcriptome Analysis Revealed a Stress Response to Deep Underground Environment Conditions in Chinese Hamster V79 Lung Fibroblast Cells. Front Genet 2021;12:698046. [Crossref] [PubMed]
- Han T, Jiang S, Zheng H, et al. Interplay between c-Src and the APC/C co-activator Cdh1 regulates mammary tumorigenesis. Nat Commun 2019;10:3716. [Crossref] [PubMed]
- Wang J, Thomas HR, Li Z, et al. Puma, noxa, p53, and p63 differentially mediate stress pathway induced apoptosis. Cell Death Dis 2021;12:659. [Crossref] [PubMed]
- Gonçalves CS, Vieira de Castro J, Pojo M, et al. WNT6 is a novel oncogenic prognostic biomarker in human glioblastoma. Theranostics 2018;8:4805-23. [Crossref] [PubMed]
- Manzari-Tavakoli A, Babajani A, Farjoo MH, et al. The Cross-Talks Among Bone Morphogenetic Protein (BMP) Signaling and Other Prominent Pathways Involved in Neural Differentiation. Front Mol Neurosci 2022;15:827275. [Crossref] [PubMed]
- Chen L, Zhang YH, Lu G, et al. Analysis of cancer-related lncRNAs using gene ontology and KEGG pathways. Artif Intell Med 2017;76:27-36. [Crossref] [PubMed]
- Lamming DW, Bar-Peled L. Lysosome: The metabolic signaling hub. Traffic 2019;20:27-38. [Crossref] [PubMed]
- Legerstee K, Houtsmuller AB. A Layered View on Focal Adhesions. Biology (Basel) 2021;10:1189. [Crossref] [PubMed]
- Dai Z, Yang S, Xu L, et al. Identification of Cancer-associated metabolic vulnerabilities by modeling multi-objective optimality in metabolism. Cell Commun Signal 2019;17:124. [Crossref] [PubMed]
- Fleming NI, Jorissen RN, Mouradov D, et al. SMAD2, SMAD3 and SMAD4 mutations in colorectal cancer. Cancer Res 2013;73:725-35. [Crossref] [PubMed]
- Yang Y, Ye WL, Zhang RN, et al. The Role of TGF-β Signaling Pathways in Cancer and Its Potential as a Therapeutic Target. Evid Based Complement Alternat Med 2021;2021:6675208. [Crossref] [PubMed]
- Baba AB, Rah B, Bhat GR, et al. Transforming Growth Factor-Beta (TGF-β) Signaling in Cancer-A Betrayal Within. Front Pharmacol 2022;13:791272. [Crossref] [PubMed]
- Huynh LK, Hipolito CJ, Ten Dijke P. A Perspective on the Development of TGF-β Inhibitors for Cancer Treatment. Biomolecules 2019;9:743. [Crossref] [PubMed]
- Mullen AC. Hippo tips the TGF-β scale in favor of pluripotency. Cell Stem Cell 2014;14:6-8. [Crossref] [PubMed]
(English Language Editor: C. Betlazar-Maseh)