Optimal validation of accuracy in antibody assays and reasonable definition of antibody positive/negative subgroups in neuroimmune diseases: a narrative review
Introduction
Advances in diagnostic techniques have made it easier to diagnose neuroimmune diseases. Autoantibodies are markers of autoimmune diseases and are of great significance in diagnosis. Advances in antibody detection have led to the discovery of more and more new antibodies, and a variety of disease-related or pathogenic antibodies have been found within the scope of the same clinical disease entity. Research of immunological mechanisms further promotes the understanding of the relationship between autoantibodies and disease phenotypes, so that antibodies are not only used for diagnosis, but also as markers for assessing disease severity and treatment efficacy and as variables to predict the prognosis. In some diseases, subgroup classification based on the combination of clinical and serological features have been proposed (1).
The following questions arise: (I) relevant autoantibodies cannot be detected in all patients of a specific autoimmune disease diagnosed based on typical neurological involvement and disease course. How to do when relevant antibodies are not detected? On the contrary, autoantibodies may be detected in some clinically atypical patients. Could the detected antibodies facilitate the diagnosis? (II) Multiple antibodies related to a disease can be detected in the same patient. Which one is the culprit antibody determining the severity and course of the disease? Can it be used for monitoring treatment efficacy? (III) Multiple antibodies related to different autoimmune diseases of the nervous system may be detected in the same patient, but the clinical phenotype only corresponds to one of the diseases. What is the culprit antibody for the present phenotype? What is the significance of detected antibodies in the diagnosis? Can the detected antibodies predict the subsequent disease course? Before answering these questions, we should know the sensitivity and specificity of the antibody assay used. Dynamic observation of changes in antibody levels helps to confirm the role of antibodies, but only when the specificity of antibodies is ensured can they be reliably analyzed. At the same time, the sensitivity should not be sacrificed while improving the specificity of an assay. In fact, specificity and sensitivity of an assay is not contradictory with the improvement of the testing technical system (2,3). In order to improve accuracy of assays in antibody detection, systematic validation studies are needed.
It has been found that within the same disease entity, subgroups can be identified based on distinct antibodies, such as aquaporin-4 (AQP4) antibodies or myelin oligodendrocyte glycoprotein (MOG) antibodies in neuromyelitis optica spectrum disease (NMOSD) (4), and acetylcholine receptor (AChR) antibodies or muscle-specific kinase (MuSK) antibodies in myasthenia gravis (MG) (1), raising the question whether the disease entity can be further subdivided. It is rare for these distinct antibodies to coexist in the same subgroup. Although subgroups classified by antibodies may have different manifestations, it is impossible to distinguish them by clinical manifestations alone (5,6). There are also cases of “overlapping” phenotypes between different subgroups of the disease, such as in Guillain-Barre syndrome (GBS) patients, where relevant antibodies coexist in overlap between the Miller Fisher phenotype and the quadriplegic phenotype (7,8). The next question is what is the significance of an antibody-associated subgroup. The antibody positive subgroup is relevant to phenotype. Unknown antibodies may be present in the “antibody negative” subgroup. The antibody negative subgroup provides an opportunity for discovery of new antibodies. In the meantime, it is necessary to know how to define the antibody positive and antibody negative subgroups.
This narrative review aims to discuss the validation studies of diagnostic accuracy in antibody assays and reasonable definitions of antibody positive/negative subgroups in neuroimmune diseases. I present the following article in accordance with the Narrative Review reporting checklist (available at https://atm.amegroups.com/article/view/10.21037/atm-21-2384/rc).
Methods
The literature discussing validation studies of diagnostic accuracy in antibody assays and reasonable definition of antibody positive/negative subgroups in autoimmune diseases was broadly searched and discussed (Table 1).
Table 1
Items | Specification |
---|---|
Date of search | Dec 31, 2021 |
Databases and other sources searched | PubMed |
Search terms used | (Neuroimmune disease or myasthenia or Guillain-Barre syndrome or neuromyelitis optica or MOG antibody associated disease or autoimmune encephalitis or paraneoplastic syndrome) and antibody and (sensitivity or specificity or accuracy or subgroup or positive) |
Timeframe | 1980–2021 |
Inclusion criteria | Research article, review and editorial, English |
Selection process | HFL conducted the selection according to the principles of testing assays, interested diseases and study design |
Discussion
Validation of diagnostic accuracy in antibody assays
Research phases in diagnostic accuracy studies
There are three phases for the studies of diagnostic accuracy (9). The exploratory phase is to determine initial impression on diagnostic capacity of a new test. Retrospective studies of 10–50 patient with typical cases vs. healthy controls is used, and crude estimates of receiver operating characteristic (ROC) curve area, true positive rate (TPR) and false positive rate (FPR) should be reported. The challenge phase is to examine the accuracy by applying the test to subgroups of patients which constitute a spectrum of the target disease, and to patients with similar clinical features that mimic the target disease. Retrospective studies of 50–100 patients of disease spectrum vs. controls with mimicking diseases are used. ROC curve area with adjustment for covariates, clinically relevant FPR and false negative rate (FNR) should be reported. The clinical phase is to prospectively measure the accuracy in a representative sample of patients from a well-defined clinical population. Prospective study of at least 100 representative cases from target population are used. Measures of diagnostic accuracy are reported dependent on clinical application. Internal validity should be examined in phase I and II studies, and external validity should be examined in phase III studies. Repeated phase III studies at a different institution is important in determination of external validity (9).
Measures for diagnostic efficacy of an assay
Six levels were proposed for diagnostic efficacy of a test (10). Level 1 is technical efficacy, which is determined by optimal laboratory techniques, and is a prerequisite for subsequent efficacy levels. Level 2 is diagnostic accuracy efficacy, including intrinsic accuracy of diagnosis and predictive values for positive and negative results in patient populations. Other levels are diagnostic thinking efficacy, therapeutic efficacy, outcome efficacy and societal efficacy. In this article, we discuss the diagnostic accuracy efficacy, which is the basis for all the other higher levels and is closely related to technical efficacy.
There are mainly three measures for intrinsic diagnostic accuracy, including sensitivity, specificity and ROC curve. Sensitivity and specificity are basic measures. They are not affected by the prevalence of disease. The sensitivity and specificity estimated from a study sample are applicable to other populations with different prevalence. The ROC curve is a plot of sensitivity vs. its FPR (which is 1 − specificity), and hence overcomes the limitations of a single sensitivity and specificity pair when comparing various tests. Sensitivity and FPR are easily read from the plot. Hence, the ROC curve provides a direct visual comparison of two or more tests. All possible decision cutoffs are included in the ROC curve. The optimal cutoff is determined based on the balancing between the benefit for patients with specific diseases (e.g., early detection may promote effective treatment) vs. the harm to healthy individuals or patients with mimic diseases. False positive results might lead to unnecessary and even potentially harmful treatment.
The choice of measure for accuracy is dependent on the study phase, the objective of the study, and the particular clinical application. In phase I studies, the ROC curve is often used to judge whether the test can distinguish patients with and without the disease. In phase II studies, the ROC curve is also used to discriminate the patients within disease spectrum and controls with mimicking diseases of similar pathologic, clinical, co-morbid conditions. In phase III studies, the ROC curve should not be used as the primary measure because it is too general. The measure of accuracy should depend on the clinical application and should have a clinically useful interpretation. For example, for a screening test, the sensitivity is most important, but positives then need to be confirmed by assays with higher specificity. However, for the diagnosis of neuroimmune diseases, specificity is preferred when the disease can be diagnosed with other clinical and radiological evidences (9).
Covariates may influence the test results while not altering the ROC curve. This is important in phase II and III studies. Matching does not eliminate this confounding. Covariate-specific ROC curves should be used to describe the accuracy for each level of the covariate. An important covariate is severity of disease. Test accuracy is often higher for more severe disease and lower for milder disease. If the sample size is small or a single summary curve is desirable to compare against a competitor diagnostic test, a covariate-adjusted curve can be used (9).
Principles in defining the disease spectrum and biases in validation studies
Validation studies begin with identification of the sample population, including the patients and the reference (control group). Although not affected by the prevalence of the disease, sensitivity, specificity and the ROC curve are affected by the spectrum of disease, as well as by patient characteristics. This may lead to bias in the validation of tests. Bias may also arise from the agreed gold standard for diagnosing a disease, the involvement of tests in diagnostic procedures, potential influence on subsequent work-up needed to establish the disease diagnosis, blinding of the gold standard or tests, and the potential influence of disease progression and treatment. Biases should be checked in each research phase. Especially in phase III studies, it is important to recruit the patient population beyond a single institution or single geographic location.
Phase I studies often retrospectively recruit patients from test records and disease-specific registries. With all patients being typical and all controls being healthy, estimates of accuracy are higher than they would be for the target patient population as a whole. Phase II studies usually retrospectively recruit more challenging cases within the disease spectrum. Patients with heterogeneity in pathological manifestations (extent, location, tissue/cell types), clinical manifestations (chronicity, severity, age, gender) and co-morbidity should be included. Patients with mimicking disease may also be included as controls. Matching is commonly used in retrospective studies to minimize differences in patients with and without the conditions.
Phase III studies consecutively recruit suspected patients to measure the accuracy of a test and the difference in accuracy of two or more tests. The sample of patients must closely represent the target population. Bias occurs when an important patient subgroup is missing, or when the diagnostic test under evaluation is incorporated or influences the subsequent clinical work-up for the final diagnosis of patients. To avoid such bias, standard administration of the test for all study patients, blinding the person performing the test, and collecting information in a standardized fashion are needed.
It is important to establish operational standards for diagnostic validity (gold standard). If imperfect criteria are used as a gold standard, the estimates of test accuracy will be affected. There are several approaches to minimize this bias: to define the disease in terms of clinical phenotype and to use an expert review panel to arrive at consensus diagnosis for difficult cases. It is important to establish the diagnosis completely independent of the diagnostic test under study. A disease can progress or regress during the delay between specimen collection and final diagnosis. This bias can be avoided by standardized collection of the clinical data at the time of specimen collection.
Interpretation of the results
Antibody testing may be expressed as quantitative and qualitative results dependent on the testing principles. For quantitative assays such as enzyme-linked immunosorbent assay (ELISA), radioimmunoassay (RIA) and flow cytometry (FCM), a cutoff is optimally set as the reference value. For qualitative assays such as blotting and indirect immunofluorescence (IIF) assays, the staining patterns should be standardized. Training of readers is important, and using non-study samples will allow them to become familiar with the study forms and the setting for the interpretations. Positive, negative and equivocal samples should be included.
The tests must be interpreted without knowledge of the final diagnosis. Test review bias may arise when the reader is not blinded to diagnosis. Inversely, diagnostic review bias occurs when the gold standard procedure is performed and interpreted with knowledge of the test results. This may overestimate the test accuracy. The reader should perform the gold standard procedure after the diagnostic test is interpreted, or the gold standard procedure should be performed by different researchers in the study group.
Specific considerations for validation studies in neuroimmune diseases
Inclusion criteria
The “gold standard” of any disease is relative. The process of understanding a disease always stems from the “prototype” disease formed by a combination of several essential clinical features, and then expands to the “atypical” cases that comprise of an optimal combination of clinical features and various diagnostic markers. Each disease has its distinctive spatial, temporal, imaging, biologic, or pathologic features. The spectrum of neuroimmune disease is expanding with the introduction of new autoantibodies. Hence, the clinical phenotype and its relationship with antibodies should be re-examined.
GBS is one of the earliest recognized and best studied neuroimmune diseases (7). The expansion of its clinical phenotype started from the collection of “variants” with different spatial involvement. After the discovery of the anti-GM1 antibody (11), systematic screening in GBS patients revealed a variety of anti-ganglioside antibodies, some of which were corresponding to a specific clinically spatial involvement with myelin/axon damage. Finally, a comprehensive scenario of clinical involvement, pathology, electrophysiology and antibody was formed in GBS. The clinically spatial involvement with axonal/myelin damage were taken as the key thread of GBS subgroup classification, and the existence of overlap types was also noted (7). MG and NMOSD have also been known as neuroimmune diseases for a long time. Detailed understanding of their heterogeneity started from the discovery of MuSK antibodies (12) and the AQP4 antibodies (13) respectively. With systematic screening for antibodies, association with clinical manifestations beyond the “prototype” phenotype were found. A correlation between antibody binding epitopes in nervous system and clinically spatial involvement were established. Lesion location and morphology in NMOSD patients depend on the binding sites of AQP4 antibodies (14,15). The recognition of autoimmune encephalitis was based on a similar strategy. Systematic screening of antibodies in patients with clinically suspected autoimmune diseases makes it possible to detect new antibodies. Whether the newly discovered antibodies have corresponding and specific phenotypes with uniquely spatial, temporal, imaging, biologic, or pathologic features will determine the significance of the antibodies. Based on the combination of clinical and serological characteristics, multiple new “core phenotypes” related to various antibodies have been formed within a disease entity. Although phase I studies often recruit patients with typical prototype phenotypes, patients with new sub-phenotypes should be included in phase II and III studies.
The core phenotype based on pure clinical features is relatively simple. The cases diagnosed based on antibody detection and confirmed by clinical follow-up enrich the phenotypes and change the umbrella of the disease entity. For example, NMOSD, MOG antibody related encephalomyelitis and glial fibrillary acidic protein (GFAF) antibody related astrocytopathy were previously classified as idiopathic demyelinating diseases. With the development of antibody detection and the fine characterization of detailed imaging features, different typical core phenotypes have been formed (16,17). However, it is difficult to distinguish the core phenotypes of disease subgroups simply from clinical features. For example, bulbar involvement is typical but not unique for MG patients with MuSK antibodies. Bulbar involvement is also commonly seen in MG patients with thymoma. On the other hand, there are also MuSK antibody positive MG patients who only show extraocular muscle involvement (6). At present, establishment of distinct core sub-phenotypes is helpful to describe typical manifestations of several subgroups within the spectrum of a disease. They may even herald an independent disease entity in the future. The diagnostic accuracy of a test for a specific “disease” will change when the disease classification changes.
Overlapping manifestations are typical in several autoimmune diseases of the nervous system. In GBS, GM1 antibodies and GQ1b antibodies coexist, and accordingly, quadriplegia, extraocular palsy and ataxia coexist clinically (8). In patients with autoimmune encephalitis after infection, multiple clinical syndromes and antibody overlaps are also present in the early course of disease (18). Phenotypic overlap and antibody overlap are common phenomena in neuroimmune diseases after infection. In patients with paraneoplastic syndromes, syndromes may expand due to the spreading of pathogenic epitopes with tumor progression, leading to the overlap of multiple syndromes (19,20). Therefore, it is difficult to measure the diagnostic accuracy for para-infectious and anti-neoplastic autoimmune syndromes. However, non-infectious and non-neoplastic autoimmune responses are usually limited to a few specific epitopes, which determine the core phenotype and the corresponding culprit antibodies (21).
The diagnostic criteria for neuroimmune diseases should fully consider the various clinical sub-phenotypes of the disease, as well as reflect the pathogenic mechanism and pathophysiological mechanism. Taking MG diagnostic criteria as an example, it has been described for many years as: muscle fatigue and fluctuating symptoms, positive fatigue test, positive cholinesterase inhibitor test, detection of auto-antibodies and typical electrophysiology. Specific requirements regarding above items have not been clarified. The diagnostic criteria in the Japanese MG diagnosis and treatment guideline (Appendix 1) (22) reflects this requirement: column A lists the typical symptoms of affected muscle groups in MG, and clarifies fatigue and fluctuation of symptoms as the basis for MG diagnosis. The pathogenic antibodies are listed in column B. Clinical and laboratory evidence reflecting the pathophysiological mechanisms of MG are listed in column C. The diagnosis is based on the clinical involvement, especially the muscle weakness pattern and the characteristic fatigability and daily fluctuation. Due to the high specificity of pathogenic antibodies, MG can be diagnosed with typical clinical manifestations and one antibody (diagnostic definition 1). The diagnostic specificity of clinical and electrophysiological tests is far below that of the pathogenic antibodies. In patients with a short disease course, a diagnosis of MG without detectable antibodies can be difficult. With disease progression, the involved muscle groups and positive evidence from column C often increase, and MG can be easily diagnosed. Therefore, diagnostic accuracy tends to be more reliable, in the cases with a longer follow-up. This is especially for patients diagnosed without information of antibodies.
It is necessary to understand the historical evolution of diagnostic criteria, and to avoid simply taking a diagnosis as configuration for inclusion in a validation study. In one study (23), MG was diagnosed by clinical and electromyographic evidence. In patients with normal neurophysiology and lacking detectable autoantibodies, MG was diagnosed based on the presence of fatigable weakness and a positive response to treatment with cholinesterase inhibitors or immunosuppression by experienced MG specialists. In another study (2), MG was diagnosed by clinical features provided by MG specialists and supportive features of evidence including >10% decrement on repetitive nerve stimulation and/or neuromuscular jitter on single-fiber EMG, treatment response to cholinesterase inhibitors, and treatment response to immunotherapy. In our study (24), MG was diagnosed based on typical clinical symptoms of fluctuating muscle weakness which improved after rest and fatigue or weakness of muscles examined by physical examination, plus at least one of the following conditions: unequivocally positive response to anticholinesterase drug treatment; reproducible decrement response of >10% on repetitive nerve stimulation. In one study of seronegative MG (25), MG was diagnosed by a consultant neurologist based on compatible clinical features together with 1 or more of the following criteria: electrophysiological findings compatible with a postsynaptic neuromuscular junction disorder, and a response to cholinesterase inhibitors.
In an age when antibody testing is widely used in clinical practice, it is difficult to diagnose a neuroimmune disease without considering antibody results detected by commercial kits. This means that the disease population of today is different from the population before antibodies were examined. More patients diagnosed based on antibodies may have atypical clinical manifestations. Patients diagnosed by clinical data alone were typical cases. The inclusion criteria used in the validation studies should include both types of patients.
Definition for control group
The selection of the control group plays a decisive role in determining the reference value of quantitative detection and the negative standard for qualitative detection. This is crucial for test specificity in the validation population. Control groups that should be considered in antibody validation studies for neuroimmune diseases include: (I) other neuroimmune diseases, (II) other autoimmune diseases, (III) other neurological diseases, (IV) non-autoimmune diseases, and (V) healthy controls. The inclusion of control groups should be based on the purpose of the study. There has been expert consensus on the selection of a control group for specific neuroimmune disease (26).
Neuroimmune diseases usually occur without any obvious infectious or neoplastic autoimmune responses. Although there is epitope spreading, the spreading is usually limited to the adjacent regions of the major immunogenicity region (MIR) in the pathogenic antigen molecules, and the antibodies are mostly from limited B cell clones. Some immune-mediated neurological diseases are associated with infections and tumors. Post-infectious ADEM, paraneoplastic syndromes, and neurological involvements associated with thymoma belongs to this condition. In these cases, polyclonal proliferation caused by nonspecific immune responses to infection (18,27), poly-antigenic epitope spreading and associated antibodies caused by tumor immunity (28), and the elimination of forbidden clones caused by thymus tolerance abnormalities (29,30) are important mechanisms. Therefore, these patients are less suited as controls in antibody validation studies of neuroimmune disease. Patients with classic neuroimmune diseases (such as multiple sclerosis, NMOSD, GBS, chronic inflammatory demyelinating polyneuropathy, MG, and myositis) other than the studied disease should be preferred. Definite autoimmune diseases not affecting the neuromuscular system can also be used as controls, in order to eliminate background immune-reactive responses. Patients who have poly-autoimmune syndromes need to be examined for thymoma (30,31), and should not be used as controls. The longer the disease duration, the more reliable as a control with a prototype phenotype.
Autoimmune diseases can also exist in a presumed health population, such as blood donors. Patients with non-immunological neurological diseases and other diseases may also be selected as controls. Elderly patients with cerebrovascular disease with a non-immunological origin and atherosclerosis could be safely selected for controls, while young patients with cerebrovascular disease should be screened for early atherosclerosis caused by autoimmune factors (32). Patients with rapid-progressive dementia need to have autoimmune encephalitis ruled out before being included as controls. Typical Alzheimer disease (AD) patients with a long course of disease can be used as the control group, although it has also been reported that anti-ganglioside antibodies can be detected in some AD patients (33).
Dynamic changes of antibody composition and sampling time
Although binding antibodies usually can be observed before the onset of the disease, there are also cases where binding antibodies cannot be detected within a short time after the clinical onset (34). This may be related to the types of antibodies. For example, commercial kits of AChR antibodies detect binding antibodies, but blocking antibodies can also cause symptoms. Blocking antibodies usually occur in association with AChR-binding antibodies and have a higher prevalence in generalized MG (35). They might appear earlier than binding antibodies and rapidly affect the function of the neuromuscular junction, thus leading to symptoms when binding antibodies are not detectable. However, most patients produced a very stable pattern of AChR antibody specificity over years despite changes in antibody concentrations and clinical status (36). Hence, the binding antibodies tend to be present in the long term once the disease has established.
It is particularly important to note that ganglioside antibodies, MOG antibodies and NMDAR antibodies detected shortly after the onset of infection-associated diseases such as GBS (37), ADEM (38,39) and autoimmune encephalitis after herpes simplex virus infection (18) may disappear during improvement of diseases. Among antibodies detected after infection, persistence of a single pathogenic antibody predicted the transformation into chronic neuroimmune disease (18,39). Persistence of pathogenic antibodies suggests the long-term memory against limited pathogenic epitopes on nervous system instead of short-term response of polyclonal activation after infectious insults.
Relationship between duration and severity of disease and antibody status
Most antibodies in MG and autoimmune encephalitis act on synaptic ion channels, causing rapid dysfunction that lead to symptoms. Timely treatment can reduce antibody levels (35,40), alleviate symptoms, and decrease the risk of permanent injury of target tissues. Antibody levels correlate with severity in some of these diseases (35). However, in patients with long disease duration without proper treatment, the correlation between antibody levels and severity decreases due to potential destruction of target tissue. The severity of symptoms in NMOSD is determined by the magnitude and location of the injury. Severe permanent injury may be caused by the first clinical attack. The symptoms determined by location within central nervous system are reflected differently in the quantitative assessments of severity. Therefore, the antibody level does not parallel the severity even in the early stage of NMOSD. Although inclusion of subgroups of different severity should be secured in phase II and III studies, the complex relationship between the measures of severity and antibody levels needs consideration. Moreover, although the levels of pathogenic antibodies usually change during the course of the disease, their actual levels continue to be higher than the test cutoffs (unpublished data, Figure 1). Duration of disease has therefore little effect on the positive rate of antibodies. Our study found no difference in the positive rates of AChR, MuSK, titin and RyR antibodies in patients with different duration of MG (41).
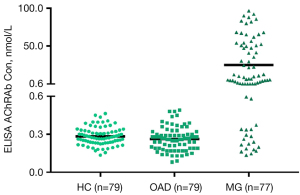
The influence of pre-test factors
Pre-test factors included freeze-thaw and storage temperatures which are related to sample quality, as well as the effects of concurrent infection and treatment. Antibodies are relatively stable and not affected by a limited number of freezing-thawing procedures (42,43). The level of specific antibodies is not affected by the incidental short-term infections. Whether the disease is active or stable needs to be recorded, and can be determined by dynamic assessment of the symptoms and signs, with the use of severity scales. The levels of pathogenic antibodies may rise before the disease relapse, while the levels of concomitant antibodies (such as antibodies in paraneoplastic syndromes) rarely change with the disease status. Once appearing, pathogenic autoantibodies tends to persist long term even if their levels drop after treatment in the majority of patients. Their actual levels in chronic neuroimmune disease are much higher than the detecting cutoffs. Our study found no difference in the positive rates of AChR, MuSK, titin and RyR antibodies in MG patients with and without immunotherapy (41).
From accuracy validation studies to improvement of antibody assays
When the sensitivity and specificity of a new antibody assay in phase II and III studies are found to be inferior compared with previous assays, the proportion of included patients with core sub-phenotypes and clinical subgroups should be considered. Furthermore, the possible confounding cases in the control group, any influence of disease course and severity, and relevant non-disease pre-test factors should be analyzed. If still not satisfactory, the testing techniques should be reassessed and improved. The processing of raw quantitative data should also be considered.
Improving the testing techniques is exemplified by measuring MuSK antibodies using the cell based assay (CBA) method (2). Initially, the secondary antibody used was a fluorescence-labeled anti-human IgG. Although this secondary antibody was raised against purified IgG, it is not IgG specific. It reacts with both the heavy and light chains of the IgG molecules and can, therefore, also detect binding of IgM antibodies that share the light chains with IgG. To overcome this shortcoming, a specific secondary antibody that binds only to the IgG-specific heavy chain Fc region was introduced, and the MuSK CBA provided an IgG-specific test. This innovation also increased the sensitivity. The same approach is now used for other CBAs, particularly for those in which a high antigen density (e.g., MOG) can non-specifically bind IgM (3).
It is important to compare the dispersion of data in the raw test value and the transformed value with the standard curve in quantitative assays. Take the competitive inhibition ELISA AChR antibody kit as an example. We found that transformation of raw values enlarged the coefficient of variation, and may changes the test specificity and sensitivity (unpublished data, Figure 2).
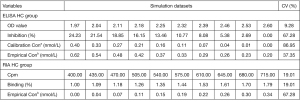
Determination of antibody positive and negative subgroups
AChR antibodies and MuSK antibodies define subgroups in MG, and the AQP4 antibodies and MOG antibodies define subgroups in NMOSD. There is little antibody overlap between subgroups. However, the clinical manifestations of antibody positive and negative subgroups tends to be similar within a disease entity. New antibodies might help to identify new subgroups. The key question is always whether a new antibody is related with clinical phenotypes, and is helpful for clinical decisions. Patients without any detected antibodies after an extensive detection of known antibodies designated as “antibody negative” cases, represent a good opportunity to explore new pathogenic antibodies.
Antibody status is usually not affected by the disease duration, severity, and previous treatment, so the accuracy of the antibody positive status is mainly determined by the testing techniques and the positive cutoffs, as discussed in the previous section. Commercial kits are usually optimized with both techniques and cutoffs, and have good diagnostic performance. However, sometimes in “antibody-negative” patients, antibody positive patients could still be found with new modified testing techniques which are more sensitive (24).
The definition of antibody positive/negative subgroups ideally requires using at least two assays of different detection principles, preferably one qualitative (including immunofluorescence-based CBA or blotting-based assays with purified antigen) and one quantitative (RIA or ELISA) method. CBA maintains the dimensional structures of the antigens and can detect antibodies that are not detected by quantitative method using purified antigen. Test results of two assays can be inconsistent because of the different dimensional structures of the antigen used. High quantitative levels and strong/moderate characteristic CBA staining prove the antibody positive status, while quantitative results below or around the cutoffs with a negative CBA illustrate antibody negative status. For borderline results, repeated tests are required. Consistent antibody status by two assays together with clinical symptoms and signs, is more reliable for defining the antibody positive/negative subgroup.
There are two procedures for defining antibody positive or negative, parallel testing and sequential testing. The parallel testing has two alternative modes of evaluations: (I) the OR rule (“believe the positive”), in which the conclusion is positive if either test A or B is positive. Both must be negative for the conclusion to be negative. (II) The AND rule (“believe the negative”), in which the conclusion is positive only if both test A and B are positive. The sequential testing also has two alternatives: (I) the OR rule (“believe the positive”), if the first test is positive, then the conclusion is positive. If negative, perform the second test. If the second test is positive, the conclusion is positive. If not, the conclusion is negative. (II) The AND rule (“believe the negative”), if the first test is positive, apply the second test. If the second test is also positive, the conclusion is positive; otherwise it is negative.
In defining positive or negative antibody subgroups, the rules should be applied with consideration on the accuracy of the assays and the purpose for the classification. In daily clinical practice, since patients with typical clinical and radiological phenotypes of target diseases may be diagnosed clinically, applying the OR rule may facilitate to initiation of specific treatment. For the research purpose, the AND rule is more preferred to define accurate positive subgroup for phenotype comparison, and the OR rule is more preferred to define a rigorous negative subgroup for exploration of new antibodies. If only one assay is available, a sequential testing can be ordered after a short interval with the same assay in another laboratory. However, it is less rigorous in defining subgroups than with assays with assays of different detection principles.
Summary
In conclusion, the successful implementation of validation studies of autoantibody assays in neuroimmune diseases depends on reasonable design, phenotypic profiles of included patients and potential inclusion bias, test result interpretation and the influence from inclusion criteria, control group selection, patient characteristics on specimen sampling and pre-test factors. Reasonable definitions on antibody positive/negative groups may be different in clinical practice and researches, and rigorous definitions are conducive to constellation of disease phenotype and the exploration of new antibodies within a disease entity.
Acknowledgments
Funding: This work was supported by the National Natural Science Foundation of China (Nos. 81771362 and 82171397 to HFL).
Footnote
Provenance and Peer Review: This article was commissioned by the editorial office, Annals of Translational Medicine for the series “Laboratory Investigations in Neuroimmunological Diseases and Their Clinical Significance”. The article has undergone external peer review.
Reporting Checklist: The author has completed the Narrative Review reporting checklist. Available at https://atm.amegroups.com/article/view/10.21037/atm-21-2384/rc
Conflicts of Interest: The author has completed the ICMJE uniform disclosure form (available at https://atm.amegroups.com/article/view/10.21037/atm-21-2384/coif). The series “Laboratory Investigations in Neuroimmunological Diseases and Their Clinical Significance” was commissioned by the editorial office without any funding or sponsorship. HFL served as the unpaid Guest Editor of the series. HFL reports that this work was supported by the National Natural Science Foundation of China (Nos. 81771362 and 82171397 to HFL). The author has no other conflicts of interest to declare.
Ethical Statement: The author is accountable for all aspects of the work in ensuring that questions related to the accuracy or integrity of any part of the work are appropriately investigated and resolved.
Open Access Statement: This is an Open Access article distributed in accordance with the Creative Commons Attribution-NonCommercial-NoDerivs 4.0 International License (CC BY-NC-ND 4.0), which permits the non-commercial replication and distribution of the article with the strict proviso that no changes or edits are made and the original work is properly cited (including links to both the formal publication through the relevant DOI and the license). See: https://creativecommons.org/licenses/by-nc-nd/4.0/.
References
- Gilhus NE, Verschuuren JJ. Myasthenia gravis: subgroup classification and therapeutic strategies. Lancet Neurol 2015;14:1023-36. [Crossref] [PubMed]
- Huda S, Waters P, Woodhall M, et al. IgG-specific cell-based assay detects potentially pathogenic MuSK-Abs in seronegative MG. Neurol Neuroimmunol Neuroinflamm 2017;4:e357. [Crossref] [PubMed]
- Waters P, Woodhall M, O’Connor KC, et al. MOG cell-based assay detects non-MS patients with inflammatory neurologic disease. Neurol Neuroimmunol Neuroinflamm 2015;2:e89. [Crossref] [PubMed]
- Sepúlveda M, Armangué T, Sola-Valls N, et al. Neuromyelitis optica spectrum disorders: Comparison according to the phenotype and serostatus. Neurol Neuroimmunol Neuroinflamm 2016;3:e225. [Crossref] [PubMed]
- Ricken G, Schwaiger C, De Simoni D, et al. Detection Methods for Autoantibodies in Suspected Autoimmune Encephalitis. Front Neurol 2018;9:841. [Crossref] [PubMed]
- Morren J, Li Y. Myasthenia gravis with muscle-specific tyrosine kinase antibodies: A narrative review. Muscle Nerve 2018;58:344-58. [Crossref] [PubMed]
- Wakerley BR, Uncini A, Yuki N, et al. Guillain-Barré and Miller Fisher syndromes—new diagnostic classification. Nat Rev Neurol 2014;10:537-44. [Crossref] [PubMed]
- Willison HJ, Jacobs BC, van Doorn PA. Guillain-Barré syndrome. Lancet 2016;388:717-27. [Crossref] [PubMed]
- Zhou XH, Obuchowski NA, McClish DK. Design of Diagnostic Accuracy Studies. In: Statistical Methods in Diagnostic Medicine. 2nd edition. Hoboken, NJ, USA: John Wiley & Sons, Inc., 2011:57-102.
- Fryback DG, Thornbury JR. The efficacy of diagnostic imaging. Med Decis Making 1991;11:88-94. [Crossref] [PubMed]
- Ilyas AA, Willison HJ, Quarles RH, et al. Serum antibodies to gangliosides in Guillain-Barré syndrome. Ann Neurol 1988;23:440-7. [Crossref] [PubMed]
- Hoch W, McConville J, Helms S, et al. Auto-antibodies to the receptor tyrosine kinase MuSK in patients with myasthenia gravis without acetylcholine receptor antibodies. Nat Med 2001;7:365-8. [Crossref] [PubMed]
- Lennon VA, Wingerchuk DM, Kryzer TJ, et al. A serum autoantibody marker of neuromyelitis optica: distinction from multiple sclerosis. Lancet 2004;364:2106-12. [Crossref] [PubMed]
- Pittock SJ, Weinshenker BG, Lucchinetti CF, et al. Neuromyelitis optica brain lesions localized at sites of high aquaporin 4 expression. Arch Neurol 2006;63:964-8. [Crossref] [PubMed]
- Roemer SF, Parisi JE, Lennon VA, et al. Pattern-specific loss of aquaporin-4 immunoreactivity distinguishes neuromyelitis optica from multiple sclerosis. Brain 2007;130:1194-205. [Crossref] [PubMed]
- Reindl M, Waters P. Myelin oligodendrocyte glycoprotein antibodies in neurological disease. Nat Rev Neurol 2019;15:89-102. [Crossref] [PubMed]
- Fang B, McKeon A, Hinson SR, et al. Autoimmune Glial Fibrillary Acidic Protein Astrocytopathy: A Novel Meningoencephalomyelitis. JAMA Neurol 2016;73:1297-307. [Crossref] [PubMed]
- Armangue T, Spatola M, Vlagea A, et al. Frequency, symptoms, risk factors, and outcomes of autoimmune encephalitis after herpes simplex encephalitis: a prospective observational study and retrospective analysis. Lancet Neurol 2018;17:760-72. [Crossref] [PubMed]
- Wei YC, Huang CC, Liu CH, et al. Peripheral neuropathy in limbic encephalitis with anti-glutamate receptor antibodies: Case report and systematic literature review. Brain Behav 2017;7:e00779. [Crossref] [PubMed]
- Kamiya-Matsuoka C, Blas-Boria D, Williams MD, et al. N-type calcium channel antibody-mediated paraneoplastic limbic encephalitis: a diagnostic challenge. J Neurol Sci 2014;338:188-90. [Crossref] [PubMed]
- Prüss H, Finke C, Höltje M, et al. N-methyl-D-aspartate receptor antibodies in herpes simplex encephalitis. Ann Neurol 2012;72:902-11. [Crossref] [PubMed]
- Murai H. Japanese clinical guidelines for myasthenia gravis: Putting into practice. Clin Exp Neuroimmunol 2015;6:21-31. [Crossref]
- Rodríguez Cruz PM, Al-Hajjar M, Huda S, et al. Clinical Features and Diagnostic Usefulness of Antibodies to Clustered Acetylcholine Receptors in the Diagnosis of Seronegative Myasthenia Gravis. JAMA Neurol 2015;72:642-9. [Crossref] [PubMed]
- Hong Y, Zisimopoulou P, Trakas N, et al. Multiple antibody detection in ‘seronegative’ myasthenia gravis patients. Eur J Neurol 2017;24:844-50. [Crossref] [PubMed]
- Cortés-Vicente E, Gallardo E, Martínez MÁ, et al. Clinical Characteristics of Patients With Double-Seronegative Myasthenia Gravis and Antibodies to Cortactin. JAMA Neurol 2016;73:1099-104. [Crossref] [PubMed]
- Teunissen C, Menge T, Altintas A, et al. Consensus definitions and application guidelines for control groups in cerebrospinal fluid biomarker studies in multiple sclerosis. Mult Scler 2013;19:1802-9. [Crossref] [PubMed]
- Cats EA, van der Pol WL, Tio-Gillen AP, et al. Clonality of anti-GM1 IgM antibodies in multifocal motor neuropathy and the Guillain-Barré syndrome. J Neurol Neurosurg Psychiatry 2015;86:502-4. [Crossref] [PubMed]
- Maverakis E, Goodarzi H, Wehrli LN, et al. The etiology of paraneoplastic autoimmunity. Clin Rev Allergy Immunol 2012;42:135-44. [Crossref] [PubMed]
- Alberti P, Handel AE. The contribution of thymic tolerance to central nervous system autoimmunity. Semin Immunopathol 2021;43:135-57. [Crossref] [PubMed]
- Holbro A, Jauch A, Lardinois D, et al. High prevalence of infections and autoimmunity in patients with thymoma. Hum Immunol 2012;73:287-90. [Crossref] [PubMed]
- Evoli A, Caliandro P, Iorio R, et al. Poly-autoimmunity in patients with myasthenia gravis: A single-center experience. Autoimmunity 2015;48:412-7. [Crossref] [PubMed]
- Haładyj E, Paradowska-Gorycka A, Felis-Giemza A, et al. Immunity and early atherosclerosis in the course of systemic lupus erythematosus, mixed connective tissue disease and antiphospholipid syndrome. Reumatologia 2016;54:187-95. [Crossref] [PubMed]
- Hatzifilippou E, Koutsouraki E, Costa VG, et al. Antibodies against gangliosides in patients with dementia. Am J Alzheimers Dis Other Demen 2014;29:660-6. [Crossref] [PubMed]
- Vincent A, Newsom-Davis J. Acetylcholine receptor antibody as a diagnostic test for myasthenia gravis: results in 153 validated cases and 2967 diagnostic assays. J Neurol Neurosurg Psychiatry 1985;48:1246-52. [Crossref] [PubMed]
- Meriggioli MN, Sanders DB. Muscle autoantibodies in myasthenia gravis: beyond diagnosis? Expert Rev Clin Immunol 2012;8:427-38. [Crossref] [PubMed]
- Tzartos SJ, Seybold ME, Lindstrom JM. Specificities of antibodies to acetylcholine receptors in sera from myasthenia gravis patients measured by monoclonal antibodies. Proc Natl Acad Sci U S A 1982;79:188-92. [Crossref] [PubMed]
- Odaka M, Koga M, Yuki N, et al. Longitudinal changes of anti-ganglioside antibodies before and after Guillain-Barré syndrome onset subsequent to Campylobacter jejuni enteritis. J Neurol Sci 2003;210:99-103. [Crossref] [PubMed]
- Pröbstel AK, Dornmair K, Bittner R, et al. Antibodies to MOG are transient in childhood acute disseminated encephalomyelitis. Neurology 2011;77:580-8. [Crossref] [PubMed]
- López-Chiriboga AS, Majed M, Fryer J, et al. Association of MOG-IgG Serostatus With Relapse After Acute Disseminated Encephalomyelitis and Proposed Diagnostic Criteria for MOG-IgG-Associated Disorders. JAMA Neurol 2018;75:1355-63. [Crossref] [PubMed]
- Dalmau J, Gleichman AJ, Hughes EG, et al. Anti-NMDA-receptor encephalitis: case series and analysis of the effects of antibodies. Lancet Neurol 2008;7:1091-8. [Crossref] [PubMed]
- Hong Y, Li HF, Skeie GO, et al. Autoantibody profile and clinical characteristics in a cohort of Chinese adult myasthenia gravis patients. J Neuroimmunol 2016;298:51-7. [Crossref] [PubMed]
- Jarius S, Wildemann B. Effect of storage conditions and freeze/thaw cycles on aquaporin-4 antibody (NMO-IgG) serum levels. Clin Chem Lab Med 2011;49:2121-2. [Crossref] [PubMed]
- Hong Y, Hao HJ, Xie YC, et al. Effect of storage conditions and freeze/thaw cycles on serum and plasma levels of anti-acetylcholine receptor (AchR) antibody. Clin Chem Lab Med 2014;52:e103-5. [Crossref] [PubMed]