Application of metal artifact reduction software in gemstone spectral computed tomography for patients after total knee arthroplasty
Introduction
Total knee arthroplasty (TKA) is the surgical replacement of a damaged knee joint with artificial joints, partly or in entirety. TKA was first introduced in the 1970s and has become the most effective treatment for end-stage knee osteoarthritis (1-3). Multi-slice spiral computed tomography (MSCT) can provide high-resolution and clear contrast tomography images and can obtain intuitive three-dimensional (3D) volume rendering (VR) images using post-processing software. It plays an important role in the preoperative evaluation of patients undergoing TKA, the evaluation of surgical and curative effects, and the diagnosis of postoperative infection (4-7). Computed tomography (CT) scanning of postoperative patients is an important method for postoperative evaluation and the diagnosis of complications, but high-density metal prostheses and bone cement will lead to different degrees of stellate and radial metal artifacts in CT images, affecting image quality and diagnostic accuracy. With the rapid development of MSCT, many techniques for reducing metal artifacts have emerged. For example, dual-energy CT (DECT) or gemstone energy spectrum CT scanning is used to obtain single-energy CT images, as well as metal artifact reduction software (MARs) and other post-processing technologies or algorithms (8-10). Gemstone spectral imaging (GSI) is a new technique used to reduce metal artifacts. Different from the polychromatic images obtained by traditional CT scanning, the virtual monochromatic spectral (VMS) images obtained by GSI scanning can overcome the influence of the hardening effect and significantly reduce beam hardening artifacts (11). MARs is a standalone method based on CT number threshold segmentation and reconstruction to reduce metal artifacts, and can correct low signals caused by photon starvation (12-14). Therefore, GSI-MARs technology may have the potential to significantly reduce metal artifacts.
To date, there have been some studies on MARs technology for artifact reduction in metal implants such as hip prostheses and pedicle screws, but only a few studies have examined the use of MARs in TKA (13,15,16). The purpose of this study was to explore the feasibility and effectiveness of the MARs reconstruction algorithm in reducing metal artifacts of knee prostheses and to explore the optimal monochromatic level of the VMS images for artifact reduction to provide high-quality images and reliable diagnosis for patients after TKA. We present the following article in accordance with the MDAR reporting checklist (available at https://atm.amegroups.com/article/view/10.21037/atm-22-3286/rc).
Methods
Study objects
We retrospectively analyzed the GSI CT scan results of 31 (14 men, 17 women; age range, 49–83 years; mean age, 64.4±11.3 years) patients who underwent TKA at West China Hospital between December 2019 and December 2020 and compared the VMS images with and without the MARs algorithm at different keV levels. The time from surgery to CT examination ranged from 3 to 6 months. All TKA patients enrolled in this study received knee prostheses made of Co-Cr-Mo alloys, and patients undergoing bilateral surgery were excluded. This retrospective study was conducted in accordance with the ethical guidelines of the Declaration of Helsinki (as revised in 2013) and was approved by the Ethics Committee of West China Hospital (No. 2019-742). Written informed consent was obtained from all patients or their next of kin before CT examination.
Image acquisition and reconstruction
All patients were examined with a 256-slice CT scanner (Revolution CT, GE Healthcare, Milwaukee, WI, USA). The CT acquisition parameters were adjusted for the GSI-MARs protocol: 315 mA in the GSI manual mode, fast kV switching between 80 and 140 kVp, detector coverage =40 mm, thickness =0.625 mm, pitch =0.984:1, and rotation time =0.8 s.
VMS images with and without MARs were reconstructed at different keV levels (80–140 keV, 20 keV intervals), with 0.625-mm section thickness, standard reconstruction type, and 50% ASiR-V (adaptive statistical iterative reconstruction VEO). The raw images were sent to an AW4.2 Workstation for post-processing analysis using the software GSI Viewer. Axial, coronal, sagittal, and 3D images of the knee joint were obtained using multiplanar reconstruction and VR.
Subjective image analysis
For our subjective evaluation, all images of these 31 patients were anonymized, randomized, and presented to 2 radiologists with 5 and 11 years of experience, respectively. All images, including axial, coronal, sagittal, and 3D VR images, were comprehensively scored using a 4-point scale to evaluate the influence of metal artifacts: 4, no metal artifact affecting the diagnosis; 3, mild metal artifacts but enough to distinguish the boundary and shape of surrounding structures, do not interfere with diagnostic decisions; 2, moderate metal artifacts covering the boundary and shape of surrounding structures, but the boundary or structure of the metal prosthesis is visually measurable, barely meeting the diagnostic requirements; and 1, severe metal artifacts that obscure the boundary and shape of the surrounding structure, and deter accurate diagnosis (17). Before the subjective evaluation, the 2 observers were trained with 5 unrelated cases (not included in this study) to ensure that the evaluation criteria were as consistent as possible.
Objective image analysis
Axial images of the knee prosthesis with fixed nails were selected for analysis. Three regions of interest (ROIs) were placed in anterior, posterior, and lateral muscle tissue near the knee prosthesis, and 1 background ROI was placed in subcutaneous fat on the femoral side far away from the knee prosthesis. The ROI size and position were made as consistent as possible in different groups of images, and the ROI size was approximately 70 mm2 (Figure 1). The CT numbers and standard deviation (SD) values of the CT numbers were measured for all ROIs. The artifact indices (AIs) of the 3 target ROIs were calculated using SD measurements as follows: AI = SQRT (SDlocation2 − SDfat2), where SDlocation is the SD value of the ROIs in the 3 target locations in the homogenous muscle adjacent to the knee prosthesis, SDfat is the SD value of subcutaneous fat, and SQRT stands for the square root operation (13). A smaller AI value suggests a lesser influence of metal artifacts. Simultaneously, ImageJ software was used to analyze the selected level of each set of images, and the percentage of pixels with CT number above 500 Hounsfield units (HU) (percentage500HU, which is the number of pixels with CT number above 500 HU divided by the total number of pixels of knee joint tissue) was calculated (Figure 2). All measurements were performed thrice, and the average of each parameter was calculated to reduce any variation.
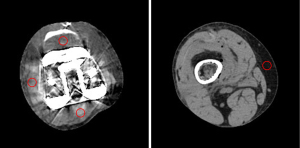
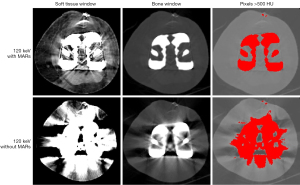
In addition, the structural similarity index measure (SSIM) values of every selected axial image were calculated by using the pytorch code (https://github.com/Po-Hsun-Su/pytorch-ssim) in reference to 140 keV images with MARs. SSIM is a quantitative value used to measure the similarity between two given images, indicating how much structural information has changed in the image based on the reference image (18,19). We took the image of 140 keV with MARs as the reference (SSIM =1) and calculated SSIM values of the images under the other seven conditions. The higher SSIM value (closer to 1), the more similarity there is to the reference image, indicating a low amount of metal artifacts. Conversely, the lower SSIM value (closer to 0) indicated a lower similarity to the 140 keV with MARs reference image and severe metal artifacts.
The calculation process of SSIM is relatively complex, which is briefly described as below (17,18). In the case of an N × N image, assuming that the original image is represented by x = {xi|i = 1, 2, …, N × N}. The distorted image is represented by y = {yi|i = 1, 2, …, N× N}, then the SSIM could be expressed by multiplying luminance, contrast, and structure. The luminance (l), contrast (c), and structural (s) are defined by the following equations.
where µx and µy represent the means of the original and coded images (respectively), σx and σy represent the SDs of each of the signals (respectively), and σxy is the covariance of the two images. Then SSIM index is calculated based on luminance, contrast, and structure values as follows:
Usually, SSIM is expressed simply by setting α = 1, β = 1, γ = 1 and C3 = C2/2, which appears as:
The arithmetic average of each local score is calculated to obtain the final score of the entire image, known as the mean SSIM (MSSIM) (17,18).
Statistical analysis
Continuous variables that conformed to a normal distribution are expressed as mean ± SD. The subjective scores of the 2 observers were evaluated using the Kappa consistency test; if the agreement was good (kappa value <0.40 indicates poor agreement, 0.40–0.74 is moderate, and ≥0.75 is good agreement), the scores of the 2 observers were combined for analysis. The Wilcoxon signed rank sum test was performed to compare the subjective scores of images with and without MARs in different keV groups, while the AI values, percentages500HU, and SSIM values were compared using a paired t-test. One-way analysis of variance (ANOVA) was used to compare the AI values and percentages500HU of different keVs in the groups with and without MARs, and the Student-Newman-Keuls method was used for pairwise comparisons. The level of statistical significance was set at P<0.05. The statistical analyses were performed using SPSS 19.0 software (SPSS® Inc., Chicago, IL, USA).
Results
Subjective analysis
The kappa value between the 2 observers for image quality scores was 0.78 (P<0.001), indicating good consistency. The subjective scores of the 2 observers and the total scores are shown in Table 1. The Wilcoxon signed rank sum test showed that the scores of groups with MARs were higher than those of groups without MARs under different keV conditions (P<0.001). In the VMS images without MARs, metal artifacts were shown as radiating hypo- and hyperdense lines or flakes centered around the knee prosthesis. Acicular hyperdense artifacts were distributed all around and flake low-density artifacts were distributed in the left and right lateral areas. In contrast, VMS images with MARs showed a significant reduction in artifacts in the area around the prosthesis, with the boundary between the prosthesis and bone interface clearly visible (Figures 3,4).
Table 1
Energy value | With MARs | Without MARs | P |
---|---|---|---|
Observer 1 | |||
80 keV | 3.65±0.55 | 1.32±0.48 | <0.001 |
100 keV | 3.77±0.43 | 1.94±0.57 | <0.001 |
120 keV | 3.94±0.25 | 2.48±0.68 | <0.001 |
140 keV | 3.97±0.18 | 2.68±0.54 | <0.001 |
Observer 2 | |||
80 keV | 3.61±0.50 | 1.39±0.49 | <0.001 |
100 keV | 3.81±0.40 | 1.87±0.50 | <0.001 |
120 keV | 3.90±0.32 | 2.29±0.64 | <0.001 |
140 keV | 3.97±0.18 | 2.61±0.56 | <0.001 |
Total | |||
80 keV | 3.63±0.52 | 1.35±0.48 | <0.001 |
100 keV | 3.79±0.41 | 1.90±0.53 | <0.001 |
120 keV | 3.92±0.27 | 2.39±0.66 | <0.001 |
140 keV | 3.97±0.18 | 2.65±0.55 | <0.001 |
MARs, metal artifact reduction software; SD, standard deviation.
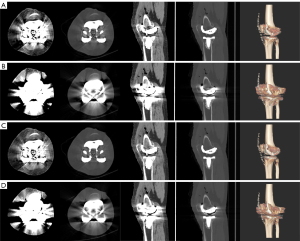
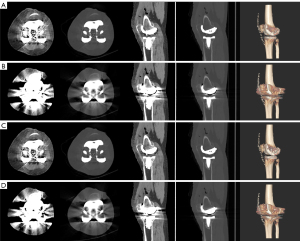
Objective analysis
The AI values of the 3 target ROIs in different keV VMS images are shown in Table 2, the percentages of pixels with CT numbers above 500 HU are shown in Table 3, and the SSIM values of images in each group are shown in Table 4. The results of the paired t-test showed that AI values of the group with MARs were lower than those of the group without MARs at different keV levels, the percentages500HU were also lower, while SSIM values were higher (all P values <0.001), as shown in Tables 2-4 and Figures 5-7.
Table 2
Energy value | With MARs | Without MARs | P |
---|---|---|---|
Anterior | |||
80 keV | 39.45±15.52 | 249.51±74.51 | <0.001 |
100 keV | 24.34±10.59 | 144.84±27.98 | <0.001 |
120 keV | 16.38±6.99 | 117.10±30.90 | <0.001 |
140 keV | 15.87±6.97 | 96.57±26.03 | <0.001 |
Lateral | |||
80 keV | 74.38±31.03 | 297.39±86.37 | <0.001 |
100 keV | 44.50±19.23 | 171.79±49.57 | <0.001 |
120 keV | 32.16±13.78 | 136.87±42.94 | <0.001 |
140 keV | 27.68±11.67 | 113.21±42.11 | <0.001 |
Posterior | |||
80 keV | 54.08±15.33 | 211.80±55.64 | <0.001 |
100 keV | 21.55±5.88 | 168.69±49.30 | <0.001 |
120 keV | 14.60±4.03 | 140.29±41.32 | <0.001 |
140 keV | 11.13±4.60 | 105.33±36.85 | <0.001 |
AI, artifact index; MARs, metal artifact reduction software; ROIs, regions of interest; SD, standard deviation; VMS, virtual monochromatic spectral.
Table 3
Energy value | Percentage500HU | P | |
---|---|---|---|
With MARs | Without MARs | ||
80 keV | 19.52±1.97 | 45.08±3.69 | <0.001 |
100 keV | 16.62±1.62 | 36.70±2.99 | <0.001 |
120 keV | 16.28±1.67 | 31.70±3.35 | <0.001 |
140 keV | 16.14±1.63 | 28.34±2.83 | <0.001 |
CT, computed tomography; HU, Hounsfield units; MARs, metal artifact reduction software; SD, standard deviation.
Table 4
Energy value | SSIM | P | |
---|---|---|---|
With MARs | Without MARs | ||
80 keV | 0.818±0.023 | 0.606±0.016 | <0.001 |
100 keV | 0.913±0.016 | 0.624±0.012 | <0.001 |
120 keV | 0.978±0.010 | 0.626±0.011 | <0.001 |
140 keV | 1.000 | 0.628±0.011 | <0.001 |
SSIM, structural similarity index measure; MARs, metal artifact reduction software; SD, standard deviation.
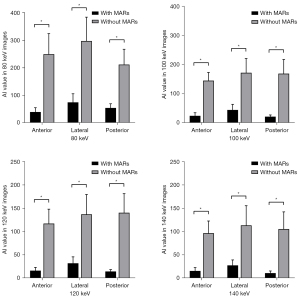
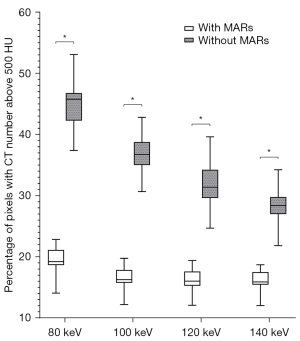
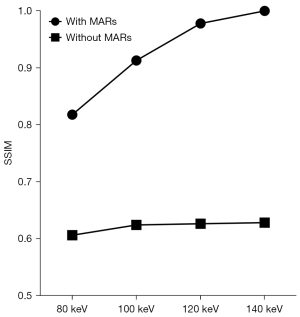
The results of the one-way ANOVA showed that there were significant differences between AI values at different keV levels (all P values <0.05). In the MARs and non-MARs groups, AI values decreased with an increase in keV in the range of 80–120 keV. The AI value of the 140 keV image was not significantly different from that of the 120 keV image (except for the posterior ROI), as shown in Figure 8. This suggests that after the energy value is increased to 120 keV, increasing keV may have little significance for reducing artifacts and improving image quality.
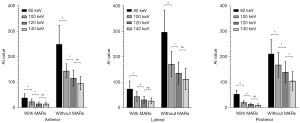
Percentages500HU of the without MARs group gradually decreased with the increase of keV in the range of 80–140 keV. The MARs group percentages500HU did not decrease after 100 keV, and there was no statistically significant difference between the values of the 100, 120, and 140 keV groups (as shown in Figure 9). This indicates that in the VMS images with MARs post-processing technology, a good metal artifact removal effect can be achieved when the energy value is above 100 keV, which can reflect the real shape and boundary of the metal prosthesis.
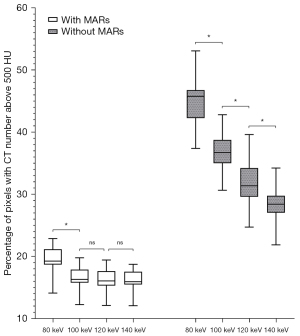
Discussion
Total knee replacement or TKA can relieve knee pain and improve knee function in patients with end-stage knee osteoarthritis and is the most effective method for the treatment of end-stage knee osteoarthritis (20). Similar to other orthopedic operations, TKA can cause various postoperative complications, such as aseptic loosening, polyethylene wear, periprosthetic infection, osteolysis, instability, and dislocation (17,21). MSCT can obtain thin-slice images of the knee joint, which can provide an important reference for preoperative surgical planning, positioning, and postoperative evaluation of patients undergoing total knee replacement combined with image post-processing software and new techniques such as 3D printing (6,22). However, because of the high density of metal prostheses, metal artifacts can appear on CT images, which affect the observation of the surrounding tissues of the prostheses, thus limiting the application of CT scanning in postoperative TKA patient evaluation. The contributors to metal artifacts are beam hardening, scattering, noise, photon starvation, and edge effects (23,24). VMS CT images can be computed using dual-energy scanning to reduce beam-hardening artifacts. The MARs algorithm mainly suppresses metal artifacts caused by photon starvation, and its basic concept is to detect the projection data corrupted by the metal hardware and replace it with the estimated and corrected values (25). MARs can effectively suppress clinically common metal artifacts and other beam-hardening artifacts, particularly for major joint replacements (12,13,26). Although some existing studies have confirmed that VMS images combined with MARs are effective in reducing metal artifacts, many of them lack discussion on the optimal display keV, and there are still few studies on the application of TKA in patients.
Yue et al. studied the CT image quality of patients after unilateral hip replacement using DECT monochromatic spectral imaging combined with MARs. They combined subjective scores with objective evaluation indicators including AI values and found that the VMS images at 120 and 140 keV could significantly reduce metal artifacts, and the observation quality of the prosthesis and surrounding tissues was the best (13). Kim et al. studied the effect of GSI-VMS images combined with the MARs algorithm on reducing metal artifacts in CT images of patients after total knee replacement. They compared images at 4 energy levels of 70, 95, 115, and 140 keV and found that 140 keV was the best observation level (17). Zeng et al. explored the display effect of virtual monoenergetic imaging using a noiseoptimized algorithm for pedicle screws under different keVs, and the results proved that the VMS image of 130 keV had the best display effect for the metal-bone interface and surrounding soft tissue, while for the spinal canal 120 keV had the best display effect (27). Zeng et al. also summarized and discussed previous studies, suggesting that different keV levels should be recommended depending on the scanning region and the metal prosthesis.
In our study, both subjective and objective evaluations confirmed that the image quality of the MARs group was significantly better than that of the non-MARs group. In the subjective evaluation, the 2 observers had a relatively consistent understanding of the image quality, and the consistency of the subjective scores was good. Compared with conventional VMS images, metal artifacts in the VMS images with MARs are effectively reduced, the prosthesis and bone structures are clearer, and the boundary between the prosthesis and surrounding soft tissues is clearly visible. The MARs algorithm provides a more accurate image for imaging diagnosis, which is consistent with the conclusions of previous research (13,26).
In the objective evaluation, we referred to previous studies and used the 3 parameters of AI value, percentage500HU, and SSIM value to evaluate the impact of metal artifacts and image quality (13,28). Among them, the AI value reflects the average degree of influence of metal artifacts on the surrounding tissues in the ROI, including white high-density artifacts and black low-density artifacts, while the percentage500HU reflects the proportion of pixels, including the prosthesis itself and high-density artifacts, and mainly evaluates the influence of acicular high-density artifacts. And SSIM value reflects the similarity between each group of images and reference images through the three dimensions of luminance, contrast, and structure, so as to evaluate the effect of metal artifact reduction comprehensively. According to the results of our study, both in the MARs group and without MARs group, the AI values of images in the high keV groups were lower than those in the low keV groups in the range of 80–120 keV. The AI value did not decrease significantly after the energy value exceeded 120 keV, and increasing keV had little significance in reducing the impact of metal artifacts. In addition, after the MARs algorithm was used, the percentage500HU did not obviously decrease when the keV rose above 100 keV, indicating that there are fewer high-density artifacts in images with energy value above 100 keV, which is sufficient to reflect the real shape and contour boundary of the prosthesis. However, in the without MARs group, the percentage500HU still changed significantly even when the energy value increased from 120 to 140 keV, and the high-density artifacts were still serious. According to the changing trend of SSIM value, we also found that in MARs group, when the energy level reached 120 keV, SSIM value was already close to 1, indicating that the 120 keV image was very similar to 140 keV image, and had good metal artifact reduction. In fact, higher keV levels are not always better, and VMS images of DECT will suppress metal artifacts and improve the clarity of metal prostheses and surrounding tissues with an increase in keV, but also lose some contrast and detail between soft tissues (29).
In summary, when using VMS images with MARs to evaluate patients after TKA, we recommend setting different keV levels for various diagnostic purposes. If soft tissue around the prosthesis is mainly observed, such as infection and edema in the soft tissue, to ensure the contrast of soft tissue as far as possible under the premise of a good metal artifact removal effect, 100–120 keV energy value is recommended. If the prosthesis is the primary focus, such as assessing instability or dislocation after surgery, 140 keV is recommended to ensure the best metal artifact removal. Furthermore, the material composition and size of the prosthesis may also affect the effect GSI-MARs algorithm. For example, larger metal prosthesis may have more severe artifacts, and different alloy materials may have different densities, thus affecting the image quality. In order to control variables, we only included patients who received knee prostheses made of Co-Cr-Mo alloys in this study, and patients undergoing bilateral surgery were excluded. The effect of different material and size of prosthesis on image quality remains to be further studied.
Our study has some limitations. Due to various factors, the sample size included in this study was relatively small, the conclusion needs to be further confirmed by multi-center and large-sample clinical trials. And there is no comparative study on metal artifact removal algorithms of different CT equipment vendors. Whether the MARs algorithm has advantages in TKA patients compared to other technologies needs to be confirmed by further research.
Acknowledgments
Funding: This study was supported by “Sichuan Provincial Department of Science and Technology, Regional Innovation Cooperation project” (program No. 22QYCX0106, to Jinge Zhang).
Footnote
Reporting Checklist: The authors have completed the MDAR reporting checklist. Available at https://atm.amegroups.com/article/view/10.21037/atm-22-3286/rc
Data Sharing Statement: Available at https://atm.amegroups.com/article/view/10.21037/atm-22-3286/dss
Conflicts of Interest: All authors have completed the ICMJE uniform disclosure form (available at https://atm.amegroups.com/article/view/10.21037/atm-22-3286/coif). The authors have no conflicts of interest to declare.
Ethical Statement: The authors are accountable for all aspects of the work in ensuring that questions related to the accuracy or integrity of any part of the work are appropriately investigated and resolved. This retrospective study was conducted in accordance with the ethical guidelines of the Declaration of Helsinki (as revised in 2013) and was approved by the Ethics Committee of West China Hospital (No. 2019-742). Written informed consent was obtained from all patients or their next of kin before CT examination.
Open Access Statement: This is an Open Access article distributed in accordance with the Creative Commons Attribution-NonCommercial-NoDerivs 4.0 International License (CC BY-NC-ND 4.0), which permits the non-commercial replication and distribution of the article with the strict proviso that no changes or edits are made and the original work is properly cited (including links to both the formal publication through the relevant DOI and the license). See: https://creativecommons.org/licenses/by-nc-nd/4.0/.
References
- Ryan J, Mora JP, Scuderi GR, et al. Total Knee Arthroplasty Design and Kinematics: Past, Present, and Future. J Long Term Eff Med Implants 2021;31:1-14. [Crossref] [PubMed]
- Meftah M, White PB, Ranawat AS, et al. Long-term results of total knee arthroplasty in young and active patients with posterior stabilized design. Knee 2016;23:318-21. [Crossref] [PubMed]
- Ranawat CS, Insall J, Shine J. Duo-condylar knee arthroplasty: hospital for special surgery design. Clin Orthop Relat Res 1976;76-82. [Crossref] [PubMed]
- Sodhi N, Jacofsky DJ, Chee A, et al. Benefits of CT Scanning for the Management of Knee Arthritis and Arthroplasty. J Knee Surg 2021;34:1296-303. [Crossref] [PubMed]
- Steinert AF, Holzapfel BM, Sefrin L, et al. Total knee arthroplasty. Patient-specific instruments and implants. Der Orthopade 2016;45:331-40. [Crossref] [PubMed]
- Vaishya R, Vijay V, Vaish A, et al. Computed tomography based 3D printed patient specific blocks for total knee replacement. J Clin Orthop Trauma 2018;9:254-9. [Crossref] [PubMed]
- Pietrzak JRT, Rowan FE, Kayani B, et al. Preoperative CT-Based Three-Dimensional Templating in Robot-Assisted Total Knee Arthroplasty More Accurately Predicts Implant Sizes than Two-Dimensional Templating. J Knee Surg 2019;32:642-8. [Crossref] [PubMed]
- Puvanasunthararajah S, Fontanarosa D, Wille ML, et al. The application of metal artifact reduction methods on computed tomography scans for radiotherapy applications: A literature review. J Appl Clin Med Phys 2021;22:198-223. [Crossref] [PubMed]
- Pan YN, Chen G, Li AJ, et al. Reduction of Metallic Artifacts of the Post-treatment Intracranial Aneurysms: Effects of Single Energy Metal Artifact Reduction Algorithm. Clin Neuroradiol 2019;29:277-84. [Crossref] [PubMed]
- Fang J, Zhang D, Wilcox C, et al. Metal implants on CT: comparison of iterative reconstruction algorithms for reduction of metal artifacts with single energy and spectral CT scanning in a phantom model. Abdom Radiol (NY) 2017;42:742-8. [Crossref] [PubMed]
- Andersson KM, Nowik P, Persliden J, et al. Metal artefact reduction in CT imaging of hip prostheses—an evaluation of commercial techniques provided by four vendors. Br J Radiol 2015;88:20140473. [Crossref] [PubMed]
- Pessis E, Campagna R, Sverzut JM, et al. Virtual monochromatic spectral imaging with fast kilovoltage switching: reduction of metal artifacts at CT. Radiographics 2013;33:573-83. [Crossref] [PubMed]
- Yue D, Fan Rong C, Ning C, et al. Reduction of metal artifacts from unilateral hip arthroplasty on dual-energy CT with metal artifact reduction software. Acta Radiol 2018;59:853-60. [Crossref] [PubMed]
- Han SC, Chung YE, Lee YH, et al. Metal artifact reduction software used with abdominopelvic dual-energy CT of patients with metal hip prostheses: assessment of image quality and clinical feasibility. AJR Am J Roentgenol 2014;203:788-95. [Crossref] [PubMed]
- Liu Q, Wang Y, Qi H, et al. Exploring the best monochromatic energy level in dual energy spectral imaging for coronary stents after percutaneous coronary intervention. Sci Rep 2021;11:17576. [Crossref] [PubMed]
- Wang Y, Qian B, Li B, et al. Metal artifacts reduction using monochromatic images from spectral CT: evaluation of pedicle screws in patients with scoliosis. Eur J Radiol 2013;82:e360-6. [Crossref] [PubMed]
- Kim J, Park C, Jeong HS, et al. The Optimal Combination of Monochromatic and Metal Artifact Reconstruction Dual-energy CT to Evaluate Total Knee Replacement Arthroplasty. Eur J Radiol 2020;132:109254. [Crossref] [PubMed]
- Wang Z, Bovik AC, Sheikh HR, et al. Image quality assessment: from error visibility to structural similarity. IEEE Trans Image Process 2004;13:600-12. [Crossref] [PubMed]
- Renieblas GP, Nogués AT, González AM, et al. Structural similarity index family for image quality assessment in radiological images. J Med Imaging (Bellingham) 2017;4:035501. [Crossref] [PubMed]
- Canovas F, Dagneaux L. Quality of life after total knee arthroplasty. Orthop Traumatol Surg Res 2018;104:S41-6. [Crossref] [PubMed]
- Mulcahy H, Chew FS. Current concepts in knee replacement: complications. AJR Am J Roentgenol 2014;202:W76-86. [Crossref] [PubMed]
- Scott AM. Total Knee Replacement and Imaging. Radiol Technol 2015;87:65-86. [PubMed]
- Boas FE, Fleischmann D. CT artifacts: causes and reduction techniques. Imaging Med 2012;4:229-40. [Crossref]
- Wellenberg RHH, Hakvoort ET, Slump CH, et al. Metal artifact reduction techniques in musculoskeletal CT-imaging. Eur J Radiol 2018;107:60-9. [Crossref] [PubMed]
- Katsura M, Sato J, Akahane M, et al. Current and Novel Techniques for Metal Artifact Reduction at CT: Practical Guide for Radiologists. Radiographics 2018;38:450-61. [Crossref] [PubMed]
- Lee YH, Park KK, Song HT, et al. Metal artefact reduction in gemstone spectral imaging dual-energy CT with and without metal artefact reduction software. Eur Radiol 2012;22:1331-40. [Crossref] [PubMed]
- Zeng Y, Deng K, Yang H, et al. Noise-optimised virtual monoenergetic imaging of dual-energy CT: effect on metal artefact reduction in patients with lumbar internal fixation. Eur Spine J 2019;28:1783-92. [Crossref] [PubMed]
- Bolstad K, Flatabø S, Aadnevik D, et al. Metal artifact reduction in CT, a phantom study: subjective and objective evaluation of four commercial metal artifact reduction algorithms when used on three different orthopedic metal implants. Acta Radiol 2018;59:1110-8. [Crossref] [PubMed]
- van Ommen F, Kauw F, Bennink E, et al. Image Quality of Virtual Monochromatic Reconstructions of Noncontrast CT on a Dual-Source CT Scanner in Adult Patients. Acad Radiol 2021;28:e323-30. [Crossref] [PubMed]
(English Language Editor: C. Betlazar-Maseh)