Non-linear relationship between baseline mean arterial pressure and 30-day mortality in patients with sepsis: a retrospective cohort study based on the MIMIC-III database
Introduction
With the increase in global life expectancy, the aging of the population is becoming more common. At present, the rate of bacterial resistance is alarming and the research and development of new antibiotics are progressing slowly (1,2). There has been vigorous development of surgical techniques in recent years, with thoracotomy, complicated abdominal tumor surgery, and large organ transplantation being widely carried out in China. The incidence of sepsis is increasing every year (3,4), affecting millions of patients worldwide, with a mortality rate of 15–30%. Sepsis and septic shock have long been major medical and health problems (5). Hemodynamic management of patients with sepsis has always been an important means of treatment (6,7), and the establishment of initial mean arterial pressure (MAP) is key (8). MAP that is too low may result in hypoperfusion of important organs in sepsis patients, while excessively high MAP cannot avoid re-injury caused by large doses of vasoactive drugs. Thus, titrating a suitable MAP has been challenging. A recent multicenter randomized controlled trial (RCT) study (9) found that there was no significant difference in the mortality rate of patients with vasodilatory shock using application-permissive hypotension (MAP: 60–65 mmHg) compared with the usual care group (41.0% vs. 43.8%). Another multicenter RCT (10) also found no difference in the 28-day mortality between the high and low MAP groups. The 2021 edition of the International Guidelines for the Management of Sepsis and Septic Shock (11) set an initial MAP target of 65 mmHg in patients with sepsis rather than a higher MAP target. However, in all of the above studies, the actual clinical blood pressure in the low MAP group was significantly higher than the target blood pressure preset in the study, and the fact that the actual blood pressure could not meet the preset blood pressure level inevitably affected the accuracy of the conclusions to a certain extent. Vincent et al.’s study (12) has confirmed that MAP does not follow a linear relationship with ICU mortality, but the study did not further explore MAP’s inflection point for the lowest 30-day mortality. Herein, a retrospective study method was adopted to collect and organize the established data in the Medical Information Mart for Intensive Care III (MIMIC-III) large-scale public clinical database during the daily diagnosis and treatment process, with the aim of restoring the true MAP of patients to the greatest extent possible. At the same time, this study will further confirm the non-linear relationship between MAP and 30-day case fatality, and determine the low inflection point. We present the following article in accordance with the STROBE reporting checklist (available at https://atm.amegroups.com/article/view/10.21037/atm-22-3457/rc).
Methods
Source
This study was based on data from the MIMIC-III database, which includes 38,597 different adult patients admitted to Beth Israel Deaconess Medical in Boston, Massachusetts, from 2001 to 2012. Important clinical information such as the times of admission and death, vital signs of each period during hospitalization, and kind of clinical intervention were recorded in detail (13). The data included in the MIMIC-III dataset can be used free of charge after passing the official assessment (14) and has been widely used by researchers around the world to forecast model development, epidemiological investigations, and other studies. All authors undertook a series of courses offered by the National Institutes of Health, passed the examination, and obtained the authorization to use relevant information from the MIMIC-III database (certification number: 36221369). Our study was performed in accordance with the reports of studies conducted using the observation routine collected health data (RECORD) (15). The study was conducted in accordance with the Declaration of Helsinki (as revised in 2013).
Study population screening
The following inclusion criteria were applied: data included in the MIMIC-III database from June 2001 to October 2012 met the diagnostic criteria for sepsis according to the third international consensus definition of sepsis and septic shock (Sepsis-3.0), i.e., suspected infection + all patients with a Sequential Organ Failure Assessment (SOFA) score ≥2 (from first day in ICU) (16). The exclusion criteria were as follows: (I) patients who were not admitted to the intensive care unit (ICU) for the first time; (II) patients younger than 18 years old; (III) patients with no recorded MAP on the first day of ICU admission; and (IV) patients with an inaccurately recorded time of death.
Study variables and data
Baseline variables in the MIMIC-III database were extracted using a structured query language (SQL) for relational databases, including patient demographics (gender, age, weight), ICU admission category, critical illness score, vital signs, pooled disease, life support measures, and laboratory test results (from the first day of admission). The ICU admission categories included medical ICU, surgery/trauma surgery ICU, and cardiology/cardiac surgery ICU. The critical illness scores included the SOFA, Simplified Acute Physiology Score II (SAPS II), and Oxford Acute Severity of Illness (OASIS) scores. Vital signs included the heart rate, MAP, respiratory rate, body temperature, and oxygen saturation. Comorbidities included congestive heart failure, arrhythmia, hypertension, stroke, chronic obstructive pulmonary disease, diabetes, chronic renal failure, liver disease, malignancy, and coagulation disorders. Life support treatments included mechanical ventilation, renal replacement therapy, vasoactive drugs, and the use of vasopressors (within 24 hours of admission). Laboratory tests included white blood cell (WBC) count, hemoglobin, platelet count, cell volume, international prothrombin time, intrinsic clotting time, intrinsic clotting time, blood urea nitrogen, creatinine, serum potassium, serum sodium bicarbonate, potential of hydrogen (PH), oxygen partial pressure, carbon dioxide partial pressure, lactic acid, and anion gap. The end point was whether death occurred within 30 days. The research and design process is shown in Figure 1.
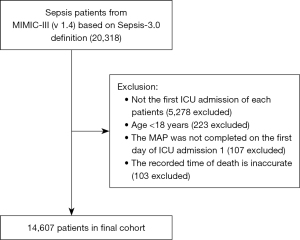
Statistical analysis
Baseline measurement data that conformed to a normal distribution were expressed as the mean ± standard deviation (), while those that did not conform to a normal distribution were expressed as the median (interquartile range) [M (IQR)]. Counting data are expressed as frequency and percentage (%). For baseline characteristic analysis, one-way ANOVA was used for statistical differences in continuous variables among the five MAP groups, and the Chi-square test was used for categorical variables. Hazard ratios (HRs) and 95% confidence intervals (CIs) for the different MAP levels and the final 30-day mortality were calculated using Cox multivariate analysis. To control confounding factors, we input the covariates into the Cox regression model one by one in the basic model or successively eliminated the covariates in the complete model, and compared the regression coefficients. The covariates were included as conditions in the Cox regression for adjustment. In this study, the Cox model was adjusted for age, sex, heart rate, SOFA score, SAPS II score, OASIS score, heart failure, hypertension, vasoactive drug use, hemoglobin concentration, blood urea nitrogen, sodium ion, bicarbonate, pH, and lactate level. A linear regression equation was used to test for trends, and the median of each group of MAPs was entered into the model as a continuous variable.
A generalized additive model was used to assess the non-linear relationship between MAP and 30-day mortality in patients with sepsis. Based on the smoothed curve, we further developed a two-segment linear regression model to determine the threshold effect and adjusted for potential confounders. The inflection point MAP was determined recursively, including selecting the inflection point along a predetermined interval that yields the maximum likelihood of the model. Based on the R language, the log-likelihood ratio test was used to compare the two-segment linear regression model and the one-segment linear model (17). Survival curves were drawn using the Kaplan-Meier method and log-rank analysis. Subgroup analyses were performed using a hierarchical Cox regression model to determine whether patients had heart failure, hypertension, vasoactive drugs, invasive mechanical ventilation, or renal replacement therapy. Interactions between subgroups were examined using a variance ratio test. To avoid the bias caused by missing data, five sets of complete data were obtained by multiple imputations according to the Monte Carlo method, and the data was analyzed after multiple imputations.
Statistical analyses were performed using R Statistical Software (https://www.r-project.org, The R Foundation) and Free Statistics analysis platform. Two-sided values of P<0.05 were considered statistically significant.
Results
Study population and baseline characteristics
After reviewing the data of 20,318 sepsis patients in the MIMIC-III database, a total of 14,607 patients were included in this retrospective parallel study according to the various exclusion criteria. The patient selection flowchart is shown in Figure 1. In this study, the MAP of the overall population was equally divided into five groups: Q1 was defined as MAP ≤67.4 mmHg, Q2 was defined as 67.4–72.5 mmHg, Q3 was defined as 72.5–77.6 mmHg, Q4 was defined as 77.6–84.6 mmHg, and Q5 was defined as MAP ≥84.6 mmHg. The patients’ demographic characteristics (gender, age, body weight), type of ICU admission, critical illness score, vital signs, co-morbidities, life support measures, and laboratory test results are displayed in a centralized manner according to the total sample and the characteristics of each group (Table 1). The critical illness scores between the groups are shown in Figure S1.
Table 1
Variables | All participants | Mean arterial pressure quintiles (mmHg) | P value | ||||
---|---|---|---|---|---|---|---|
Q1 (≤67.4) | Q2 (67.4–72.5) | Q3 (72.5–77.6) | Q4 (77.6–84.6) | Q5 (≥84.6) | |||
Participants | 14,607 | 2,922 | 2,921 | 2,920 | 2,921 | 2,923 | |
Age | 67.2±16.3 | 72.1±14.8 | 68.6±15.8 | 67.1±16.0 | 65.8±16.4 | 62.5±17.2 | <0.001 |
Sex, n (%) | <0.001 | ||||||
Female | 6,844 (46.9) | 1,561 (53.4) | 1,374 (47.0) | 1,304 (44.7) | 1,299 (44.5) | 1,306 (44.7) | |
Male | 7,763 (53.1) | 1,361 (46.6) | 1,547 (53.0) | 1,616 (55.3) | 1,622 (55.5) | 1,617 (55.3) | |
Weight, kg | 80.7±25.2 | 78.0±25.3 | 80.0±23.7 | 81.9±25.6 | 82.3±25.5 | 81.4±25.6 | <0.001 |
30-day mortality | 3,071 (21.0) | 968 (33.1) | 628 (21.5) | 536 (18.4) | 469 (16.1) | 470 (16.1) | <0.001 |
Service unit, n (%) | <0.001 | ||||||
MICU | 7,383 (50.5) | 1,740 (59.5) | 1,501 (51.4) | 1,379 (47.2) | 1,331 (45.6) | 1,432 (49.0) | |
SICU/TSICU | 3,725 (25.5) | 499 (17.1) | 603 (20.6) | 708 (24.2) | 885 (30.3) | 1,030 (35.2) | |
CCU/CSRU | 3,499 (24.0) | 683 (23.4) | 817 (28.0) | 833 (28.5) | 705 (24.1) | 461 (15.8) | |
Severity of illness | |||||||
SOFA | 5.0 (3.0, 7.0) | 6.0 (4.0, 8.0) | 5.0 (3.0, 8.0) | 5.0 (3.0, 7.0) | 4.0 (3.0, 7.0) | 4.0 (3.0, 6.0) | <0.001 |
SAPS II | 41.1±14.3 | 46.6±14.8 | 43.0±14.3 | 41.0±13.5 | 38.9±13.5 | 35.8±12.7 | <0.001 |
OASIS | 34.7±8.7 | 35.9±8.9 | 35.5±8.8 | 34.9±8.5 | 33.9±8.7 | 33.1±8.3 | <0.001 |
Vital signs | |||||||
Heart rate (bpm) | 88.1±16.5 | 85.3±16.5 | 87.6±16.5 | 88.6±16.4 | 88.7±16.3 | 90.3±16.5 | <0.001 |
MAP (mmHg) | 76.3±10.9 | 62.8±4.0 | 70.0±1.5 | 75.0±1.5 | 80.8±2.0 | 92.8±7.6 | <0.001 |
Respiratory rate (bpm) | 19.9±4.4 | 20.1±4.4 | 19.9±4.3 | 20.0±4.5 | 19.6±4.3 | 19.7±4.5 | <0.001 |
Temperature (°C) | 36.9±0.7 | 36.7±0.7 | 36.9±0.7 | 36.9±0.7 | 36.9±0.7 | 36.9±0.7 | <0.001 |
SpO2 | 96.9±2.8 | 96.5±3.6 | 96.9±2.9 | 97.0±2.8 | 97.1±2.5 | 97.2±1.9 | <0.001 |
ELF, n (%) | |||||||
RRT use (first 24 h) | 764 (5.2) | 228 (7.8) | 143 (4.9) | 128 (4.4) | 113 (3.9) | 152 (5.2) | <0.001 |
MV use (first 24 h) | 7,173 (49.1) | 1,079 (36.9) | 1,498 (51.3) | 1,636 (56.0) | 1,537 (52.6) | 1,423 (48.7) | <0.001 |
Vasopressor use (first 24 h) | 2,484 (17.1) | 544 (18.7) | 508 (17.5) | 481 (16.5) | 452 (15.5) | 499 (17.2) | 0.023 |
Comorbidities, n (%) | |||||||
CHF | 3,497 (23.9) | 874 (29.9) | 722 (24.7) | 680 (23.3) | 607 (20.8) | 614 (21.0) | <0.001 |
Cardiac arrhythmias | 3,621 (24.8) | 842 (28.8) | 758 (26.0) | 699 (23.9) | 685 (23.5) | 637 (21.8) | <0.001 |
Hypertension | 2,127 (14.6) | 547 (18.7) | 435 (14.9) | 379 (13.0) | 379 (13.0) | 387 (13.2) | <0.001 |
Stroke | 545 (3.7) | 80 (2.7) | 102 (3.5) | 109 (3.7) | 114 (3.9) | 140 (4.8) | 0.001 |
COPD | 3,135 (21.5) | 663 (22.7) | 654 (22.4) | 635 (21.7) | 629 (21.5) | 554 (19.0) | 0.004 |
Diabetes mellitus | 4,165 (28.5) | 928 (31.8) | 860 (29.4) | 807 (27.6) | 838 (28.7) | 732 (25.0) | <0.001 |
Renal failure | 2,600 (17.8) | 696 (23.8) | 549 (18.8) | 455 (15.6) | 450 (15.4) | 450 (15.4) | <0.001 |
Liver disease | 1,233 (8.4) | 312 (10.7) | 235 (8.0) | 216 (7.4) | 223 (7.6) | 247 (8.5) | <0.001 |
Malignancy | 1,569 (10.7) | 389 (13.3) | 347 (11.9) | 293 (10.0) | 284 (9.7) | 256 (8.8) | <0.001 |
Coagulopathy | 2,599 (17.8) | 640 (21.9) | 544 (18.6) | 521 (17.8) | 437 (15.0) | 457 (15.6) | <0.001 |
Laboratory tests | |||||||
WBC (×109/L) | 13.1 (9.3, 18.3) |
13.3 (9.2, 19.3) |
13.6 (9.2, 19.2) |
13.3 (9.6, 18.6) |
12.9 (9.4, 17.7) |
12.5 (9.1, 17.3) |
<0.001 |
Hemoglobin (g/dL) | 9.9±2.0 | 9.4±1.8 | 9.6±1.9 | 9.7±2.0 | 10.1±2.1 | 10.5±2.2 | <0.001 |
Platelet (×109/L) | 184.0 (120.0, 259.0) |
179.0 (114.0, 257.2) |
180.0 (116.0, 256.8) |
181.0 (121.0, 254.0) |
186.0 (124.0, 261.0) |
191.0 (125.0, 263.0) |
0.002 |
Hematocrit (%) | 29.2±6.1 | 28.2±5.4 | 28.5±5.7 | 28.9±5.9 | 29.8±6.2 | 30.9±6.5 | <0.001 |
Inr | 1.4 (1.2, 1.8) |
1.5 (1.2, 2.1) |
1.4 (1.2, 1.8) |
1.4 (1.2, 1.8) |
1.3 (1.2, 1.7) |
1.3 (1.1, 1.6) |
<0.001 |
PT (seconds) | 15.0 (13.5, 18.0) |
15.9 (14.0, 19.9) |
15.3 (13.8, 18.5) |
15.0 (13.5, 17.7) |
14.7 (13.3, 17.1) |
14.3 (13.2, 16.7) |
<0.001 |
APTT (seconds) | 33.9 (28.2, 47.1) |
36.8 (29.9, 53.3) |
35.5 (29.3, 48.0) |
34.4 (28.5, 48.0) |
32.7 (27.6, 44.5) |
31.0 (26.8, 39.7) |
<0.001 |
Bun (mg/dL) | 26.0 (17.0, 43.0) |
34.0 (21.0, 56.0) |
28.0 (18.0, 46.0) |
25.0 (17.0, 40.0) |
23.0 (16.0, 37.0) |
22.0 (15.0, 37.0) |
<0.001 |
Creatinine (mg/dL) | 1.2 (0.9, 2.0) |
1.6 (1.0, 2.6) |
1.3 (0.9, 2.1) |
1.2 (0.8, 1.8) |
1.2 (0.8, 1.7) |
1.1 (0.8, 1.8) |
<0.001 |
Potassium (mmol/L) | 3.7 (3.4, 4.1) |
3.8 (3.4, 4.3) |
3.7 (3.4, 4.1) |
3.7 (3.4, 4.1) |
3.7 (3.4, 4.1) |
3.7 (3.3, 4.1) |
<0.001 |
Sodium (mmol/L) | 136.3±5.7 | 135.8±6.0 | 136.0±5.5 | 136.3±5.8 | 136.5±5.2 | 137.1±5.7 | <0.001 |
Bicarbonate (mmol/L) | 21.8±5.4 | 20.9±5.6 | 21.5±5.4 | 21.9±5.3 | 22.1±5.3 | 22.6±5.2 | <0.001 |
PH | 7.4±0.1 | 7.3±0.1 | 7.4±0.1 | 7.4±0.1 | 7.4±0.1 | 7.4±0.1 | <0.001 |
PO2 (mmHg) | 120.0 (78.0, 223.0) |
105.0 (71.0, 195.2) |
118.0 (77.0, 231.0) |
124.0 (79.0, 244.0) |
130.0 (82.0, 233.0) |
123.0 (83.0, 209.0) |
<0.001 |
PCO2 (mmHg) | 42.4±12.8 | 42.3±13.3 | 42.6±12.9 | 42.8±12.9 | 42.3±12.1 | 41.9±12.8 | 0.175 |
Lac (mmol/L) | 2.3 (1.5, 3.8) | 2.3 (1.6, 3.8) | 2.3 (1.5, 3.8) | 2.4 (1.5, 3.9) | 2.3 (1.5, 4.0) | 2.1 (1.4, 3.5) | <0.001 |
Anion gap (mmol/L) | 16.0 (13.0, 19.0) |
16.0 (14.0, 20.0) |
16.0 (13.0, 18.0) |
16.0 (13.0, 19.0) |
16.0 (13.0, 18.0) |
16.0 (14.0, 19.0) |
<0.001 |
Variables are presented as mean ± SD, median (IQR) or N (%). MICU, medical intensive care unit; SICU, surgical intensive care unit; TSICU, trauma surgical intensive care unit; CCU, coronary care unit; CSRU, cardiac surgery unit; SOFA, Sequential Organ Failure Assessment; SAPS II, Simplified Acute Physiology Score II; OASIS, Oxford Acute Severity of Illness Score; MAP, mean arterial pressure; ELF, extracorporeal life support; RRT, renal replacement therapy; MV, mechanical ventilation; CHF, congestive heart failure; COPD, chronic obstructive pulmonary disease; WBC, white blood cell; INR, international normalized ratio; PT, prothrombin time; APTT, activated partial thromboplastin time; Bun, blood urea nitrogen; PH, potential of hydrogen; PO2, partial pressure of oxygen; PCO2, partial pressure of carbon dioxide; Lac, lactic acid.
Main results
Of the 14,607 patients, a total of 3,071 patients had died by day 30, resulting in an overall case fatality rate (CFR) of 21.0%. The Kaplan-Meier curve showed that the Q1 (≤67.4 mmHg) group had the highest mortality rate, followed by the Q2 (67.4–72.5 mmHg) group, Q3 (72.5–77.6 mmHg), and Q4 (77.6–84.6 mmHg) groups. The Q5 (≥84.6 mmHg) group had the lowest fatality rate (P<0.0001 by log-rank test, see Figure 2). A total of 3,777 patients died at day 60, with an overall case fatality rate of 25.9%. However, the trend of Kaplan-Meier curve at 60 days was almost the same as that at 30 days in the five groups (Figure S2). In the adjust III COX regression model without adjustment for multiple imputations, the absence of each covariate before multiple imputations (Table S1) increased the MAP by 1 mmHg and decreased the 30-day mortality by 2% (HR: 0.98, 95% CI: 0.97–0.99, P=0.001, Table S2). By fitting a multivariate Cox regression model to a smooth curve, we found that the relationship between MAP and 30-day mortality was non-linear (Figure 3). The relationship between MAP and 30-day CFR was fitted by piecewise multivariate Cox regression with two different slopes, and a two-piece model was applied to fit the relationship between MAP and 30-day CFR separately.
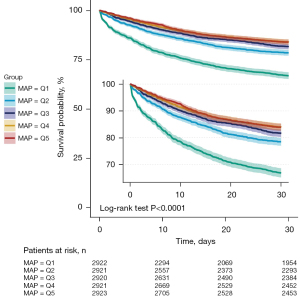
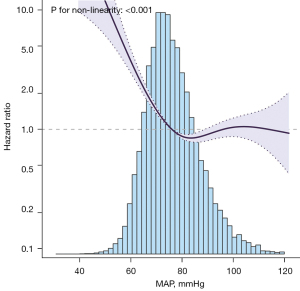
To determine whether there is a dose-response between MAP and 30-day CFR, we used a smooth curve function to adjust for potential confounders and found that the lowest inflection point for 30-day CFR was approximately 68.6 mmHg. For every 10 mmHg change in MAP, the effect size was to the left of the inflection point (HR: 0.479, 95% CI: 0.403–0.569, P<0.001). To the right of the inflection point, the effect size was (HR: 0.996, 95% CI: 0.931–1.065, P<0.9018) (Table 3).
Table 3
Outcome | HR | 95% CI | P value |
---|---|---|---|
<68.6 mmHg | 0.479 | (0.403, 0.569) | <0.001 |
≥68.6 mmHg | 0.996 | (0.931, 1.065) | 0.9018 |
Likelihood ratio test | – | – | <0.001 |
Adjusted for age and gender, heart rate, SOFA, SAPS II, OASIS, congestive heart failure, hypertension, vasopressor use, hemoglobin, Bun, sodium, bicarbonate, PH, lac. MAP, mean arterial pressure; HR, hazard ratio; CI, confidence interval.
Sensitivity analysis
Using the Q1 group as a reference, we found that the HRs of the Q3 and Q4 groups were at lower levels in the unadjusted, adjusted 1, adjusted 2, and adjusted 3 models after multiple imputations. In the adjusted 3 model, we can see that a 1 mmHg increase in MAP was associated with a 2% decrease in the 30-day mortality (HR: 0.98, 95% CI: 0.97–0.99, P<0.001, see Table 2). Taking the Q1 group as a reference, we found that Q2 (HR: 0.64, 95% CI: 0.58–0.71, P<0.001), Q3 (HR: 0.57, 95% CI: 0.52–0.64, P<0.001), Q4 (HR: 0.57, 95% CI: 0.51–0.63, P<0.001), and Q5 (HR: 0.65, 95% CI: 0.58–0.73, P<0.001). For further sensitivity analysis, we used MAP as a categorical variable (quintile) and found a P value <0.001 in the trend test (Table 2).
Table 2
Exposure | Non-adjusted | Adjust I | Adjust II | Adjust III | |||||||
---|---|---|---|---|---|---|---|---|---|---|---|
HR (95% CI) | P value | HR (95% CI) | P value | HR (95% CI) | P value | HR (95% CI) | P value | ||||
MAP mmHg | 0.97 (0.97–0.98) | <0.001 | 0.97 (0.96–0.98) | <0.001 | 0.98(0.97–0.99) | <0.001 | 0.98(0.97–0.99) | <0.001 | |||
MAP quintiles | |||||||||||
Q1 (≤67.4) | Reference | Reference | Reference | Reference | |||||||
Q2 (67.4–72.5) | 0.59 (0.53–0.65) | <0.001 | 0.62 (0.56–0.69) | <0.001 | 0.63 (0.57–0.7) | <0.001 | 0.64 (0.58–0.71) | <0.001 | |||
Q3 (72.5–77.6) | 0.49 (0.44–0.55) | <0.001 | 0.53 (0.48–0.59) | <0.001 | 0.58 (0.52–0.64) | <0.001 | 0.57 (0.52–0.64) | <0.001 | |||
Q4 (77.6–84.6) | 0.42 (0.38–0.47) | <0.001 | 0.47 (0.42–0.52) | <0.001 | 0.56 (0.5–0.63) | <0.001 | 0.57 (0.51–0.63) | <0.001 | |||
Q5 (≥84.6) | 0.42 (0.38–0.47) | <0.001 | 0.49 (0.44–0.55) | <0.001 | 0.68 (0.61–0.77) | <0.001 | 0.65 (0.58–0.73) | <0.001 | |||
P for trend | <0.001 | <0.001 | <0.001 | <0.001 |
Non-adjusted: no covariates were adjusted; the adjust I model adjusted for age and gender; the adjust II adjusted for adjust I + heart rate, SOFA, SAPS II, OASIS; the Adjust III model adjusted for adjust II + congestive heart failure, hypertension, vasopressor use, hemoglobin, bun, sodium, bicarbonate, PH, lac. MAP, mean arterial pressure; SOFA, Sequential Organ Failure Assessment; SAPS II, Simplified Acute Physiology Score II; OASIS, Oxford Acute Severity of Illness Score.
Heart failure, hypertension, and the use of vasoactive drugs are known confounding factors for 30-day mortality in patients with sepsis. Invasive mechanical ventilation and renal replacement therapy are commonly used life support methods. To observe the MAP levels in different subgroups and determine whether the relationship with sepsis patients with 30-day mortality is stable, we conducted stratified and interaction analyses (see Table 4). The data showed that there was an interaction between the use of vasoactive drugs and whether renal replacement therapy was used in the relationship between MAP and 30-day mortality in patients with sepsis [renal replacement therapy (RRT) use (P for interaction =0.038), vasoactive drugs (P for interaction =0.013)]. Therefore, these associations are worthy of further investigation.
Table 4
Confounding factor category | Mean arterial pressure quintiles (mmHg) | P for trend | P for interaction | ||||
---|---|---|---|---|---|---|---|
Q1 (≤67.4) | Q2 (67.4–72.5) | Q3 (72.5–77.6) | Q4 (77.6–84.6) | Q5 (≥84.6) | |||
CHF | 0.265 | ||||||
0 | 1 (Ref) | 0.64 (0.55–0.74) | 0.54 (0.46–0.63) | 0.53 (0.45–0.63) | 0.58 (0.49–0.69) | <0.001 | |
1 | 1 (Ref) | 0.62 (0.5–0.77) | 0.52 (0.41–0.66) | 0.58 (0.45–0.75) | 0.65 (0.49–0.86) | <0.001 | |
Hypertension | 0.234 | ||||||
0 | 1 (Ref) | 0.66 (0.58–0.75) | 0.55 (0.47–0.63) | 0.57 (0.49–0.66) | 0.63 (0.54–0.74) | <0.001 | |
1 | 1 (Ref) | 0.51 (0.38–0.7) | 0.44 (0.31–0.62) | 0.44 (0.31–0.62) | 0.46 (0.31–0.69) | <0.001 | |
Vasopressor use | 0.013 | ||||||
0 | 1 (Ref) | 0.69 (0.6–0.8) | 0.57 (0.49–0.67) | 0.61 (0.52–0.72) | 0.71 (0.6–0.84) | <0.001 | |
1 | 1 (Ref) | 0.5 (0.4–0.64) | 0.45 (0.35–0.58) | 0.41 (0.31–0.54) | 0.42 (0.31–0.56) | <0.001 | |
MV use | 0.718 | ||||||
0 | 1 (Ref) | 0.63 (0.5–0.78) | 0.67 (0.53–0.86) | 0.64 (0.49–0.82) | 0.64 (0.49–0.84) | <0.001 | |
1 | 1 (Ref) | 0.65 (0.56–0.75) | 0.51 (0.44–0.6) | 0.55 (0.46–0.64) | 0.62 (0.51–0.74) | <0.001 | |
RRT use | 0.038 | ||||||
0 | 1 (Ref) | 0.65 (0.57–0.74) | 0.53 (0.46–0.61) | 0.56 (0.48–0.64) | 0.64 (0.55–0.74) | <0.001 | |
1 | 1 (Ref) | 0.47 (0.3–0.74) | 0.56 (0.36–0.87) | 0.42 (0.25–0.7) | 0.33 (0.18–0.59) | <0.001 |
Adjusted for age and gender, heart rate, SOFA, SAPS II, OASIS, CHF, hypertension, Vasopressor use, hemoglobin, Bun, sodium, bicarbonate, PH, lac. MAP, mean arterial pressure; MIMIC-III, Medical Information Mart for Intensive Care-III; CHF, congestive heart failure; MV, mechanical ventilation; RRT, renal replacement therapy.
In each subgroup analysis, we found that the relationship between MAP level and 30-day mortality in sepsis patients remained stable. Taking the Q1 group as the reference, the HRs of Q2, Q3, Q4, and Q5 groups were all lower than those of the Q1 group. The P-value for trend was less than 0.001. We also found that in the subgroup that underwent renal replacement therapy, the lowest effect size occurred in the Q5 group (HR: 0.33, 95% CI: 0.18–0.59) versus the Q1 group (HR: 1), and the remaining subgroups were lower; the HRs were mainly concentrated in the Q3 and Q4 groups, and the specific effect sizes and CIs are centrally displayed in the table.
Discussion
This retrospective cohort study based on the MIMIC-III dataset had three main findings. Firstly, we found that the 30-day mortality rate of sepsis patients in the Q1 group with the lowest MAP on the first day was significantly higher than that of other groups. Secondly, after multiple imputations and adjustment for potential confounding factors, we found that the MAP of sepsis patients on the first day in the Q2 (67.4–72.5 mmHg), Q3 (72.5–77.6 mmHg), and Q4 (77.6–84.6 mmHg) groups was relatively low risk, among which the Q3 and Q4 groups typically had the lowest risk. Finally, we found that the MAP of sepsis patients on the first day was not as high as possible, and there is a non-linear relationship between the 30-day mortality rate and the first day MAP in these patients. The exclusion of grouping effects was associated with the lowest risk of 30-day mortality when the MAP on the first day as about 68.6 mmHg; when the MAP was less than 68.6 mmHg, the risk of 30-day mortality in sepsis patients decreased with the increasing MAP. When MAP >68.6 mmHg, there was no statistical difference in the 30-day mortality risk of patients with sepsis with the increase of MAP.
Numerous large studies (18-20) have been conducted over the past decade comparing the relationship between different pressure indicators and the prognosis of patients with sepsis, such as central venous pressure, pulmonary capillary wedge pressure, and MAP. Since MAP is not only a determinant of mean circulatory filling pressure but also a major driver of venous return and cardiac output, research into the relationship between MAP and the prognosis of patients with sepsis has been favored by scholars (21,22). At the same time, the mean arterial pressure is also the focus of successive international guidelines for the management of sepsis and septic shock (11,23-27). A retrospective study based on MIMIC data (12), which included 5,347 patients with distributive shock, found that the mortality rates of ICU patients with MAPs of <80, <75, <65, <60, and <55 mmHg for a duration of less than 2 hours were 20.2%, 18.7%, 22.1%, 26.1%, and 31.0%, respectively. This is also consistent with our results that higher is not better for MAP. In our study, we determined that there is a non-linear relationship between the 30-day mortality and MAP in sepsis patients, which corresponds to the lowest mortality rate after adjusting for potential confounding factors. The MAP corresponding to the lowest 30-day case fatality rate may be around 68.6mmHg. At the same time, our study refined the analysis of MAP levels and 30-day mortality in sepsis patients across different subgroups, further determining the stability of this non-linear relationship.
Some previous studies have reported findings that are not completely consistent with our results. In one multicenter, randomized clinical trial conducted in 65 ICUs in the United Kingdom (9), a total of 2,598 patients aged ≥65 years with vasodilatory hypotension were randomly assigned into a permissive hypotension group (60–65 mmHg) and a usual care group. After obtaining extended retrospective consent, data from 1,221 permissive hypotension and 1,242 usual care group patients were included in the primary outcome analysis. At 90 days, there were 500 (41.0%) deaths in the permissive hypotension group versus 544 (43.8%) deaths in the usual care group (P=0.15). In another open-label multicenter RCT involving 11 ICUs in Canada and the United States (10), a total of 120 patients with vasodilatory shock were included and randomized (1:1) into high (75–80 mmHg) and low (60–65 mmHg) MAP groups. The clinical data of 60 patients in the low MAP group and 58 patients in the high MAP group were finally included in the primary outcome analysis (as two patients withdrew their informed consent). The proportion of patients who died or had persistent organ dysfunction within 28 days did not differ between the groups (44% in the low MAP group vs. 46% in the high MAP group, P=0.21). From the aforementioned study of 65 centers in the United Kingdom (9), we can see that the actual average clinical MAP of the permissive hypotension group reached 66.7mmHg, which is higher than the upper limit of the preset MAP. Also, from the abovementioned study of 11 ICUs in Canada and the United States (10), and the actual MAP was significantly higher than the preset upper limit. Secondly, compared with these two studies, our study further subdivided the MAP levels, and this finer grouping allowed us to observe subtle differences between the various groups. These two situations may be the reason why the results of our study are not exactly the same as those of the above two studies. Using the continuously-adjusted Cox regression model combined with the Kaplan-Meier curve, it is not difficult to see that the Q2, Q3, and Q4 groups exhibited lower HRs and better clinical prognoses. Despite the retrospective nature of our study, the MAP values were all generated before the study, and the groups was divided according to the established facts, which resolves the problem of the actual blood pressure not being completely controlled at the preset target level in the prospective study.
Although the vital organs of the body have a certain self-regulation ability (28,29), which allows important organs such as the heart, brain, kidney, and other important organs to maintain perfusion through their own regulation when the blood pressure decreases, this ability is lost when the blood pressure is lower than a certain level. Moreover, if the blood pressure continues to fail to recover, severe organ failure and death are inevitable, and higher blood pressures also present the risk of re-injury caused by hyperperfusion. Sepsis patients are prone to shock, but not all patients with sepsis require the use vasoactive drugs. Some patients can avoid or reduce the use of vasoactive drugs after timely anti-infective treatment and fluid expansion. The current guidelines only propose an initial MAP target for sepsis patients who require vasoactive drugs, but there is no recommendation regarding the MAP level to be maintained in sepsis patients as a whole. This study is mainly aimed at the overall population of sepsis without initial target MAP orientation. In this study, we refined MAP into groups, and the relationship between different MAP levels and 30-day mortality was continuously and dynamically observed. From this study, it is clear that patients in the Q1 group with lower MAPs had a higher mortality rate. After adjusting for potential confounding factors, we found that when the MAP was less than 68.6 mmHg, the 30-day mortality rate decreased with the increase of MAP. However, when the MAP was greater than 68.6 mmHg, changes in the 30-day mortality with the increase of MAP were not statistically significant. This may also be the reason why the MAPs of the experimental and control groups in many two-arm studies were located to the right of the inflection point or were close to each other and were not statistically different.
The present study was based on the large MIMIC public database with a large sample size, which avoids the influence of individual differences in income or extreme values on the results of small sample studies (30). Secondly, the data obtained from the MIMIC database were all unvarnished data generated from the daily diagnosis and treatment process. Also, the specific values were all collected before the study and divided according to the established facts, which ideally resolves the dilemma caused by the fact that the actual MAP of patients in the RCTs (9-10) were generally higher than the initially set MAP level of their study (31).
However, this study also had some limitations that should be noted. Firstly, this was a retrospective cohort study, and unlike RCTs, did not involve strict inclusion and exclusion criteria, fewer comorbidities, and a high degree of uniformity in disease severity. Patients with sepsis in the MIMIC database have a variety of comorbidities, and the continuous income regardless of the severity of the disease was closer to the actual clinical conditions of patients, with strong universality and good extrapolation (32). Secondly, there were some missing data in this study, which makes it impossible to include all samples, and some covariates were also missing. However, we used multiple imputation methods to minimize the bias caused by the missing data. Thirdly, although the sample size of this study was relatively large, it was still a single-center study and lacks patients from different countries and races to offset the differences between regions and races. Finally, the blood pressure values obtained from the MIMIC database were from both invasive and non-invasive measurements, and may have been collected using equipment from different manufacturers, so there was no way to strictly standardize the blood pressure measurements. However, each medical center regularly calibrates its instruments to prevent errors in clinical work. Furthermore, the key to Beth Israel Deaconess Medical Center’s world-renowned practices is their strict maintenance and control of clinical details.
In the future, we will further explore the relationship between various stress indicators and the prognosis of patients with sepsis, and compare the relationship between permissible low MAP and high MAP based on MAP application propensity-score matching. We will also develop big data to a greater extent, expand multi-center clinical open databases such as the Emergency Intensive Care Unit (EICU), and further explore the relationship between MAP and sepsis patient mortality. Prospective RCTs are also needed to verify our results.
Conclusions
Taken together, our study demonstrated a non-linear relationship between MAP and patient 30-day mortality in patients with sepsis, with lower MAP values being significantly associated with higher 30-day mortality rates. The optimal MAP level associated with the lowest risk of 30-day mortality was approximately 68.6 mmHg.
Acknowledgments
We would like to thank Prof. Lv Jun from the Clinical Research Department of Jinan University and Prof. Yang Qilin from the Second Affiliated Hospital of Guangzhou Medical University for their early guidance in big data extraction, Prof. Liu Jie from the Department of Vascular Surgery of Beijing 301 Hospital for the research design, statistics from Union Hospital of Fujian Medical University Suggestions, and guidance of Professor Li Haibo from the Teaching and Research Office in statistical analysis.
Funding: The study was supported by the Clinical Medicine Foundation of Guangdong Province (No. 20212T14).
Footnote
Reporting Checklist: The authors have completed the STROBE reporting checklist. Available at https://atm.amegroups.com/article/view/10.21037/atm-22-3457/rc
Conflicts of Interest: All authors have completed the ICMJE uniform disclosure form (available at https://atm.amegroups.com/article/view/10.21037/atm-22-3457/coif). The authors have no conflicts of interest to declare.
Ethical Statement: The authors are accountable for all aspects of the work in ensuring that questions related to the accuracy or integrity of any part of the work are appropriately investigated and resolved. The study was conducted in accordance with the Declaration of Helsinki (as revised in 2013).
Open Access Statement: This is an Open Access article distributed in accordance with the Creative Commons Attribution-NonCommercial-NoDerivs 4.0 International License (CC BY-NC-ND 4.0), which permits the non-commercial replication and distribution of the article with the strict proviso that no changes or edits are made and the original work is properly cited (including links to both the formal publication through the relevant DOI and the license). See: https://creativecommons.org/licenses/by-nc-nd/4.0/.
References
- Morrison L, Zembower TR. Antimicrobial Resistance. Gastrointest Endosc Clin N Am 2020;30:619-35. [Crossref] [PubMed]
- McEwen SA, Collignon PJ. Antimicrobial Resistance: a One Health Perspective. Microbiol Spectr 2018; [Crossref] [PubMed]
- Angus DC, Linde-Zwirble WT, Lidicker J, et al. Epidemiology of severe sepsis in the United States: analysis of incidence, outcome, and associated costs of care. Crit Care Med 2001;29:1303-10. [Crossref] [PubMed]
- Gohil SK, Cao C, Phelan M, et al. Impact of Policies on the Rise in Sepsis Incidence, 2000-2010. Clin Infect Dis 2016;62:695-703. [Crossref] [PubMed]
- Cecconi M, Evans L, Levy M, et al. Sepsis and septic shock. Lancet 2018;392:75-87. [Crossref] [PubMed]
- Lipcsey M, Castegren M, Bellomo R. Hemodynamic management of septic shock. Minerva Anestesiol 2015;81:1262-72. [PubMed]
- García-de-Acilu M, Mesquida J, Gruartmoner G, et al. Hemodynamic support in septic shock. Curr Opin Anaesthesiol 2021;34:99-106. [Crossref] [PubMed]
- Leone M, Asfar P, Radermacher P, et al. Optimizing mean arterial pressure in septic shock: a critical reappraisal of the literature. Crit Care 2015;19:101. [Crossref] [PubMed]
- Lamontagne F, Richards-Belle A, Thomas K, et al. Effect of Reduced Exposure to Vasopressors on 90-Day Mortality in Older Critically Ill Patients With Vasodilatory Hypotension: A Randomized Clinical Trial. JAMA 2020;323:938-49. [Crossref] [PubMed]
- Lamontagne F, Meade MO, Hébert PC, et al. Higher versus lower blood pressure targets for vasopressor therapy in shock: a multicentre pilot randomized controlled trial. Intensive Care Med 2016;42:542-50. [Crossref] [PubMed]
- Evans L, Rhodes A, Alhazzani W, et al. Surviving sepsis campaign: international guidelines for management of sepsis and septic shock 2021. Intensive Care Med 2021;47:1181-247. [Crossref] [PubMed]
- Vincent JL, Nielsen ND, Shapiro NI, et al. Mean arterial pressure and mortality in patients with distributive shock: a retrospective analysis of the MIMIC-III database. Ann Intensive Care 2018;8:107. [Crossref] [PubMed]
- Johnson AE, Pollard TJ, Shen L, et al. MIMIC-III, a freely accessible critical care database. Sci Data 2016;3:160035. [Crossref] [PubMed]
- Johnson AE, Stone DJ, Celi LA, et al. The MIMIC Code Repository: enabling reproducibility in critical care research. J Am Med Inform Assoc 2018;25:32-9. [Crossref] [PubMed]
- Langan SM, Schmidt SA, Wing K, et al. The reporting of studies conducted using observational routinely collected health data statement for pharmacoepidemiology (RECORD-PE). BMJ 2018;363:k3532. [Crossref] [PubMed]
- Singer M, Deutschman CS, Seymour CW, et al. The Third International Consensus Definitions for Sepsis and Septic Shock (Sepsis-3). JAMA 2016;315:801-10. [Crossref] [PubMed]
- Muggeo V. Segmented: an R package to fit regression models with broken-line relationships. R News 2008;8:20-5.
- Jones AE, Shapiro NI, Trzeciak S, et al. Lactate clearance vs central venous oxygen saturation as goals of early sepsis therapy: a randomized clinical trial. JAMA 2010;303:739-46. [Crossref] [PubMed]
- Houwink AP, Rijkenberg S, Bosman RJ, et al. The association between lactate, mean arterial pressure, central venous oxygen saturation and peripheral temperature and mortality in severe sepsis: a retrospective cohort analysis. Crit Care 2016;20:56. [Crossref] [PubMed]
- Santos TM, Franci D, Gontijo-Coutinho CM, et al. Inflammatory lung edema correlates with echocardiographic estimation of capillary wedge pressure in newly diagnosed septic patients. J Crit Care 2018;44:392-7. [Crossref] [PubMed]
- Wolff CB, Collier DJ, Shah M, et al. A Discussion on the Regulation of Blood Flow and Pressure. Adv Exp Med Biol 2016;876:129-35. [Crossref] [PubMed]
- Magder S. Volume and its relationship to cardiac output and venous return. Crit Care 2016;20:271. [Crossref] [PubMed]
- Dellinger RP, Carlet JM, Masur H, et al. Surviving Sepsis Campaign guidelines for management of severe sepsis and septic shock. Crit Care Med 2004;32:858-73. [Crossref] [PubMed]
- Dellinger RP, Levy MM, Carlet JM, et al. Surviving Sepsis Campaign: international guidelines for management of severe sepsis and septic shock: 2008. Crit Care Med 2008;36:296-327. [Crossref] [PubMed]
- Dellinger RP, Levy MM, Rhodes A, et al. Surviving sepsis campaign: international guidelines for management of severe sepsis and septic shock: 2012. Crit Care Med 2013;41:580-637. [Crossref] [PubMed]
- Rhodes A, Evans LE, Alhazzani W, et al. Surviving Sepsis Campaign: International Guidelines for Management of Sepsis and Septic Shock: 2016. Crit Care Med 2017;45:486-552. [Crossref] [PubMed]
- Levy MM, Evans LE, Rhodes A. The Surviving Sepsis Campaign Bundle: 2018 update. Intensive Care Med 2018;44:925-8. [Crossref] [PubMed]
- Meng L, Wang Y, Zhang L, et al. Heterogeneity and Variability in Pressure Autoregulation of Organ Blood Flow: Lessons Learned Over 100+ Years. Crit Care Med 2019;47:436-48. [Crossref] [PubMed]
- Meng L. Heterogeneous impact of hypotension on organ perfusion and outcomes: a narrative review. Br J Anaesth 2021;127:845-61. [Crossref] [PubMed]
- Schneeweiss S. Learning from big health care data. N Engl J Med 2014;370:2161-3. [Crossref] [PubMed]
- Sanchez-Pinto LN, Luo Y, Churpek MM. Big Data and Data Science in Critical Care. Chest 2018;154:1239-48. [Crossref] [PubMed]
- Li X, Zheng R, Zhang T, et al. Association between blood urea nitrogen and 30-day mortality in patients with sepsis: a retrospective analysis. Ann Palliat Med 2021;10:11653-63. [Crossref] [PubMed]
(English Language Editor: A. Kassem)