Single-cell immune ecosystem and metabolism reprogramming imprinted by psoriasis niche
Introduction
Psoriasis is one of the most prevalent immune-related skin diseases (1). Inside the psoriasis microenvironment, dendritic cells (DCs) can produce certain interleukins such as interleukin (IL)-23 and IL-12 to recruit T cell subtypes, which in turn release interferon γ (IFN-γ) or tumor necrosis factor (TNF) and generate the inflammation (2). Recent high-throughput single-cell RNA-sequencing (scRNA-seq) data showed that T helper 17 (Th17) cells displayed distinct transcriptome profiles in the psoriasis niche (3). Further computational evidence showed that the immune microenvironment of psoriasis was strongly associated with the skin phenotypes and clinical outcomes (4). All those results highlight the fundamental role of the immune microenvironment in controlling the fate of psoriasis initiation and progression.
The abnormal metabolic activity of immune cells inside the tissue microenvironment was strongly linked with disease progression. For example, we recently developed the scMetabolism pipeline and showed that macrophage subsets are metabolically remodeled during cancer metastasis (5). Inside the tumor, regulatory T (Treg) cells can flexibly reprogram their metabolic profile and help cancer cell survive with the aid of lactic acid and lipid signaling (6,7). As for autoimmune diseases, Th17 cells switch between glycolysis and beta oxidation (8). Interestingly, clinical evidence showed that psoriasis patients showed a higher incidence of altered metabolic status (9,10). Those data allowed us to hypothesize that inflammatory skin disease may also show altered immunometabolism imprinted by the tissue microenvironment.
Here, by utilizing the state-of-the-art scRNA-seq data, we systematically compared the immune microenvironment difference between psoriasis and normal skin tissues. We observed a broad spectrum of immune cells with notably altered pathway activity especially oxidative phosphorylation. In particular, glycolysis and fatty acid metabolism were significantly enhanced in exhausted CD8 T cells. Our work uncovered the immunometabolism landscape of psoriasis microenvironment and indicated the potential treatments for targeting this disease. We present the following article in accordance with the MDAR reporting checklist (available at https://atm.amegroups.com/article/view/10.21037/atm-22-1810/rc).
Methods
Data source and data availability
The scRNA-seq data (barcodes, features, and matrix of gene expression) was downloaded from Gene Expression Omnibus (ID: GSE151177; GSE41664. https://www.ncbi.nlm.nih.gov/geo/query/acc.cgi?acc=GSE151177; https://www.ncbi.nlm.nih.gov/geo/query/acc.cgi?acc= GSE41664). All data and code are available by request.
scRNA-seq quality control, processing, and visualization
Seurat (V4.0) (11) was used to create the gene expression matrix object (min.cells =3, min.features =200). Then, data normalization, variable feature identification, data scaling, and principal component analysis (PCA) were performed. Harmony (12) was further used to integrate all cells based on the sample ID. Next, dimensional reduction and clustering analysis were performed on all cells (reduction = “harmony”, dims =1:20) and only the CD45+ cell clusters were kept. The “dittoSeq” package was used to visualize the cell type proportion across conditions (13).
Cell type annotation
The main cell types of CD45+ cells were annotated by using the marker of CD3D, LYZ, KLRF1, and LAMP3 for the identification of T cells, myeloid cells, natural killer (NK) cells, and DCs, respectively as we and others described before (3,5). SingleR was also utilized to confirm the main cell type identification (14). The CD8 T cell subsets and CD4 T cell subsets were further annotated. In detail, 6 distinct clusters were observed for CD4 T cells. The top markers are CCL5, CCD7, FOXP3, LTB, SELL, and KLRB1. We hence define them into CCL5+ CD4 T cells, CCR7+GPR183+ CD4 T cells, FOXP3+ Treg cells, LTB+ CD4 T cells, naive CD4 T cells, and Th17 cells. As for CD8 T cells, we observed 5 distinct clusters for CD8 T cells. The top markers are CTLA4, GZMB, GZMK, SLC4A10, and SELL. They were hence defined into CTLA4+ CD8 T cells, GZMB+ CD8 T cells, GZMK+ CD8 T cells, MAIT cells, and naive CD8 T cells.
Cell trajectory inference
Monocle2 was utilized to infer the trajectory of T cells between conditions. In detail, only GZMB+ CD8 T cells, CTLA4+ CD8 T cells, GZMK+ CD8 T cells, naive CD8 T cells were kept to infer the trajectory. The top 500 variable genes were reserved to perform downstream analysis. The reduceDimension function was used to construct the trajectory (max_components =2, auto_param_selection = T, method = “ICA”).
Single-cell metabolism quantification
The “scMetabolism” package was used to compute the single cell metabolic activity of T cells. In detail, the method was set as “VISION” and Kyoto Encyclopedia of Genes and Genomes (KEGG) metabolic gene sets were used for analysis (5). The function DotPlot.metabolism was used for visualization.
Pathway enrichment analysis
The “clusterprofiler” package was used to performed the gene enrichment analysis (15). The KEGG and hallmark gene sets were set as input (16).
Statistical analysis
Wilcox test and spearman correlation test was used.
Ethical statement
This paper is a data analysis paper based on open access data, hence the ethics approval is not required. The study was conducted in accordance with the Declaration of Helsinki (as revised in 2013).
Results
Charting the immune profile of psoriasis at the single cell level
To understand the immune cell landscape at the single-cell level, we retrieved the scRNA-seq data of 10x Genomics Chromium Single Cell platform as is described before (3) (see Methods). We split out the immune cells [defined as CD45+ cells as we described before (5)] and integrated all cells by utilizing harmony (Figure 1A) (12). On average, an average of 1,777 genes could be detected in each cell and a total of 7,944 cells were included for analysis. To annotate the immune cell types, we combined the SingleR (14) and manual annotation based on markers (Figure 1B) as we described before (5). In general, we observed the rich enrichment of diverse immune cell types (Figure 1C) such as myeloid cells (n=2,033), T cells (n=4,646; CD8 T cells, n=1,607; CD4 T cells, n=3,039), NK cells (n=565), and DC cells (n=700), which is consistent with the original annotation (3). We next compared the cellular proportion of main immune cell types between normal and psoriasis groups. Interestingly, the T cells showed higher infiltration level inside the psoriasis (59.7% vs. 38.7%, Figure 1D). This result indicated the immune microenvironment of psoriasis is potentially distinct from the normal skin. We hence performed the unsupervised clustering of the pseudo-bulk transcriptional profiles of immune cells in each sample (Figure 1E,1F). As expected, control samples were clustered into single cluster while most psoriasis samples were classified into other clusters. Further PCA of pseudo-bulk CD45+ cells also indicate the specific transcriptional profile of psoriasis immune microenvironment. We further utilized the too-many-cell algorithm and observed the unique clustering result of psoriasis compared with normal controls (Figure 1G). In summary, all those data indicated that the immune profile of psoriasis niche is largely different from normal controls.
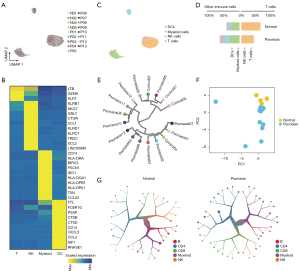
Heterogeneous T cell subtypes and states inside the psoriasis microenvironment
Due to the higher proportion of T cells inside the psoriasis, we next split the T cells out and annotate their subset cell types manually. We performed the unsupervised clustering analysis of CD4/CD8 T cells, computed their highly expressed genes, and annotate them according to their markers (Figure 2A,2B). As for the CD4 T cells, we observed a high fraction of CCL5+ CD4 T cells (n=1,213). The immunosuppressive cells, FOXP3+ Treg cells, also harbored rich infiltration (Figure 2C,2D). As for the CD8 T cells, we also observed the high diversity of different cell subsets such as naive CD8 T cells, GZMB+ CD8 T cells, GZMK+ CD8 T cells, and CTLA4+ CD8 T cells (Figure 2E,2F). Those results led us to compare the T cell composition difference between normal and psoriasis samples (Figure 2G). As a result, the psoriasis skin exhibited higher infiltration level of CCR7+GPR183+ CD4 T cells and GZMK+ CD8 T cells. The immunosuppressive cells such as Treg cells and CTLA4+ CD8 T cells also showed higher infiltration in psoriasis skin samples. All those results highlighted the heterogeneity of immune microenvironment of psoriasis and implied the potential key role of T cells especially the suppressive T cells in psoriasis development.
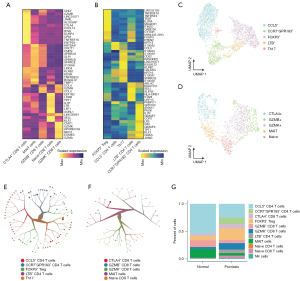
Suppressive T cells undergo extensive reprogramming imprinted by psoriasis
To understand how the suppressive T cells underwent reprogramming during psoriasis, we first compared the cellular proportion of immunosuppressive cells between normal and psoriasis samples. Strikingly, we observed the significant upregulated infiltration level of FOXP3+ Treg cells and CTLA4+ CD8 cells inside the psoriasis tissues (Figure 3A), which is partly in agreement with previous reports (17). To validate the immunosuppressive cell enrichment, we examined the gene signature in another cohort. In consistency with the scRNA-seq data, we observed that exhausted T cell signature is significantly higher in lesioned region and is decreased after the etanercept treatment (Figure S1). Such observation further explained the immune microenvironment dynamics along with psoriasis initiation and progression. Given the high proportion of those immunosuppressive cells, we next investigated the phenotypic difference of T cells between normal and psoriasis samples. We performed the phenotypic scoring of naiveness and cytotoxicity of all T cells and observed the significantly enhanced T cell phenotyping in psoriasis (Figure 3B). To fully capture the unbiased molecular difference between normal and psoriasis samples, we performed the differential expression analysis of all CD8 T cells between normal and psoriasis samples and computed the pathway enrichment analysis. Interestingly, CD8 T cells infiltrated in psoriasis skins were enriched with higher activity of oxidative phosphorylation but low activity of TNF-α (Figure 3C). These data indicated the potential role of metabolic regulation in psoriasis immune microenvironment. Next, we used the “monocle” package to trace the developmental trajectory of CD8 T cells. The results showed that naïve CD8 T cells developed into two branches including cytotoxic CD8 T cells (i.e., GZMB+ CD8 T cells) and exhausted CD8 T cells (i.e., CTLA4+ CD8 T cells), indicating the potential differentiation lineage of CD8 T cells imprinted by the psoriasis microenvironment (Figure 3D). In summary, these results highlighted suppressive T cells potentially undergo extensive phenotypic and state reprogramming imprinted by psoriasis.
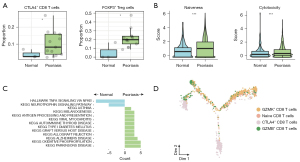
Rewired intercellular network reprogramming driven by psoriasis
Given the altered immune cell states and phenotypes inside the psoriasis niche, we next investigated whether such reprogramming can drive the altered cell-cell crosstalk. We first computed the incoming and outgoing signaling intensity of all immune cells. As a result, cytotoxic T cells such as GZMK+ CD8 T cells and GZMB+ CD8 T cells showed the top incoming ligand-receptor incoming intensity (Figure 4A). On the contrary, memory CD4 T cells showed the highest outgoing. Of note, the suppressive immune cells including CTLA4+ CD8 T cells and Treg cells showed intermediate incoming and outgoing signaling intensity. To understand the detailed signaling pathways, we computed all 16 ligand-receptor pathways and ranked them according to their overall intensity (Figure 4B,4C). As a result, MIF, GALECTIN, CCL, and CXCL showed the top activation intensity. CCL is potentially conserved across all outgoing signaling patterns, while CXCL is specifically active in certain cell types such as CTLA4+ exhausted CD8 T cells. These data led us to hypothesize CXCL and CCL pathways may play fundamental roles in psoriasis microenvironment formation. We hence performed the CCL/CXCL signaling pathway network analysis between all immune cells. Interestingly, CTLA4+ CD8 T cells can serve as the sender of CXCL pathway and interact with GZMB+ CD8 T cells (Figure 4D,4E). Among all ligand-receptor pairs, CXCL13-CXCR3 showed the highest interaction score and is associated with the wide crosstalk between exhausted T cells and cytotoxic T cells (Figure 4F). Collectively, all these data highlighted the rewired cellular crosstalk network linked with psoriasis and indicated the central role of exhausted T cells in driving intercellular crosstalk.
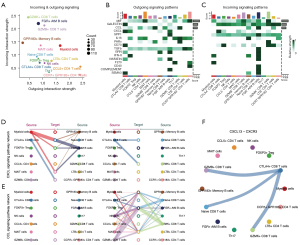
Hot immunometabolism of T cells inside the psoriasis niche
To explore the metabolic state switching between psoriasis and normal skins, we utilized the “scMetabolism” package that we developed before (5) to quantify the metabolic activity of single T cells. We first filtered the metabolic pathways according to their variability (top 50% variable pathways) and compared their activity between different immune cell subsets. As a result, we observed the diverse metabolic profile across different T cell subsets (Figure 5A). For example, we observed the high glycolysis/gluconeogenesis activity of LTB+ CD4 T cells, FOXP3+ Treg, and Th17 cells inside the psoriasis, while such glycolytic activity is lower in normal skin enriched cells such as GZMB+ CD8 T cells. Pyruvate metabolism, the final product of glycolysis, also exhibited similar metabolic profile spanning all T cells. We next explored the overall metabolic activity of all T cells and ranked their scores. Interestingly, the psoriasis-infiltrated T cells showed overall higher metabolic activity while the normal skin enriched T cells are metabolically cold. In detail, the immunosuppressive T cells inside the psoriasis such as FOXP3+ Treg cells and CTLA4+ CD8 T cells showed the higher rankings among all T cells (Figure 5B), indicating that psoriasis microenvironment may imprint the unique metabolic profile of infiltrated T cells. In summary, the immunometabolism of T cells inside the psoriasis niche is generally fueled up and the immunosuppressive cells showed higher metabolic activity, raising up the possibility of targeting such unique cell types to fight against psoriasis.
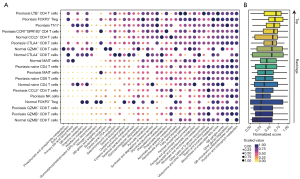
Discussion
In this work, we utilized the single-cell transcriptomics data and observed the inflamed immune microenvironment of psoriasis. In particular, psoriasis tissues exhibited higher suppressive T cell subsets such as exhausted T cells and Treg cells. Those cells were featured with high metabolic activity and broad spectrum of metabolic enzyme activation. Our results highlighted the unique niche formed by such disease and raised the possibility of targeting the specific immunometabolism to fight against psoriasis.
The immune microenvironment of psoriasis is unique compared to the normal skins. For example, a retrospective study composed of 1,145 skin samples showed that psoriatic microenvironment can be clustered into two distinct immunophenotypes (4). Such immunophenotype can effectively predict the drug responsive patterns and tightly associate with clinical outcomes. Our data showed that immunosuppressive cells are significantly enriched in psoriatic microenvironment. Beyond those findings, we also observed that CCR7+GPR183+ CD4 cells or GZMK+ CD8 cells were enriched in psoriatic microenvironment while GZMB+ CD8 cells were decreased, although these alterations did not reach the statistical significance. Such divergence of immunosuppressive cells (CTLA4+ CD8 T cells) and cytotoxic cells (GZMB+ CD8 T cells) also explained the suppressive microenvironment switching during psoriasis progression.
Immune metabolism was recently reported to be necessary to drive specific immune lineage differentiation and support immune protection or pathogenic responses (18). For example, we and others recently showed that unique immune subsets such as MRC1+CCL18+ macrophages (5) and FOXP3+ Treg cells (6) can undergo metabolic remodelling during cancer progression or metastasis. Those evidence drove us to hypothesize that the unique ecosystem of psoriasis may also imprint certain immune subset states and enforces their functional specialization. Our results showed that immunosuppressive cells such as Treg cells and exhausted T cells exhibited higher metabolic activity (i.e., glycolysis). These data indicated that further experimentally inhibiting glycolytic metabolism of exhausted immune cells may balance the disrupted ecosystem of skin.
Our study did have some limitations. In this study, almost all results are descriptive rather than casual. Further experimental validation is required to prove that such state shift of T cells is the causal reason underlying psoriasis or the result of psoriasis progression.
In summary, our work provided detailed evidence regarding the unique immune ecosystem of psoriasis and revealed how psoriasis microenvironment contribute to inframammary skin disease. These data not only helped us understand how immune metabolism is regulated under the condition of psoriasis, but also opened new opportunities for targeting metabolism in treating such skin diseases.
Acknowledgments
We thank Alice Yang from UCLA for the language editing.
Funding: None.
Footnote
Reporting Checklist: The authors have completed the MDAR reporting checklist. Available at https://atm.amegroups.com/article/view/10.21037/atm-22-1810/rc
Conflicts of Interest: All authors have completed the ICMJE uniform disclosure form (available at https://atm.amegroups.com/article/view/10.21037/atm-22-1810/coif). The authors have no conflicts of interest to declare.
Ethical Statement: The authors are accountable for all aspects of the work in ensuring that questions related to the accuracy or integrity of any part of the work are appropriately investigated and resolved. This paper is a data analysis paper based on open access data, hence the ethics approval is not required. The study was conducted in accordance with the Declaration of Helsinki (as revised in 2013).
Open Access Statement: This is an Open Access article distributed in accordance with the Creative Commons Attribution-NonCommercial-NoDerivs 4.0 International License (CC BY-NC-ND 4.0), which permits the non-commercial replication and distribution of the article with the strict proviso that no changes or edits are made and the original work is properly cited (including links to both the formal publication through the relevant DOI and the license). See: https://creativecommons.org/licenses/by-nc-nd/4.0/.
References
- Langley RG, Krueger GG, Griffiths CE. Psoriasis: epidemiology, clinical features, and quality of life. Ann Rheum Dis 2005;64:ii18-23; discussion ii24-5. [Crossref] [PubMed]
- Lowes MA, Suárez-Fariñas M, Krueger JG. Immunology of psoriasis. Annu Rev Immunol 2014;32:227-55. [Crossref] [PubMed]
- Kim J, Lee J, Kim HJ, et al. Single-cell transcriptomics applied to emigrating cells from psoriasis elucidate pathogenic versus regulatory immune cell subsets. J Allergy Clin Immunol 2021;148:1281-92. [Crossref] [PubMed]
- Wang G, Miao Y, Kim N, et al. Association of the Psoriatic Microenvironment With Treatment Response. JAMA Dermatol 2020;156:1057-65. [Crossref] [PubMed]
- Wu Y, Yang S, Ma J, et al. Spatiotemporal Immune Landscape of Colorectal Cancer Liver Metastasis at Single-Cell Level. Cancer Discov 2022;12:134-53. [Crossref] [PubMed]
- Lim SA, Wei J, Nguyen TM, et al. Lipid signalling enforces functional specialization of Treg cells in tumours. Nature 2021;591:306-11. [Crossref] [PubMed]
- Watson MJ, Vignali PDA, Mullett SJ, et al. Metabolic support of tumour-infiltrating regulatory T cells by lactic acid. Nature 2021;591:645-51. [Crossref] [PubMed]
- Wagner A, Wang C, Fessler J, et al. Metabolic modeling of single Th17 cells reveals regulators of autoimmunity. Cell 2021;184:4168-4185.e21. [Crossref] [PubMed]
- Gelfand JM, Yeung H. Metabolic syndrome in patients with psoriatic disease. J Rheumatol Suppl 2012;89:24-8. [Crossref] [PubMed]
- Kim ES, Han K, Kim MK, et al. Impact of metabolic status on the incidence of psoriasis: a Korean nationwide cohort study. Sci Rep 2017;7:1989. [Crossref] [PubMed]
- Hao Y, Hao S, Andersen-Nissen E, et al. Integrated analysis of multimodal single-cell data. Cell 2021;184:3573-3587.e29. [Crossref] [PubMed]
- Korsunsky I, Millard N, Fan J, et al. Fast, sensitive and accurate integration of single-cell data with Harmony. Nat Methods 2019;16:1289-96. [Crossref] [PubMed]
- Bunis DG, Andrews J, Fragiadakis GK, et al. dittoSeq: Universal User-Friendly Single-Cell and Bulk RNA Sequencing Visualization Toolkit. Bioinformatics 2020;btaa1011. Epub ahead of print. [Crossref] [PubMed]
- Aran D, Looney AP, Liu L, et al. Reference-based analysis of lung single-cell sequencing reveals a transitional profibrotic macrophage. Nat Immunol 2019;20:163-72. [Crossref] [PubMed]
- Yu G, Wang LG, Han Y, et al. clusterProfiler: an R package for comparing biological themes among gene clusters. OMICS 2012;16:284-7. [Crossref] [PubMed]
- Liberzon A, Birger C, Thorvaldsdóttir H, et al. The Molecular Signatures Database (MSigDB) hallmark gene set collection. Cell Syst 2015;1:417-25. [Crossref] [PubMed]
- Nussbaum L, Chen YL, Ogg GS. Role of regulatory T cells in psoriasis pathogenesis and treatment. Br J Dermatol 2021;184:14-24. [Crossref] [PubMed]
- Ganeshan K, Chawla A. Metabolic regulation of immune responses. Annu Rev Immunol 2014;32:609-34. [Crossref] [PubMed]