Exploration of hub genes, lipid metabolism, and the immune microenvironment in stomach carcinoma and cholangiocarcinoma
Introduction
Gastric cancer (GC) is the 5th most common cancer and the 3rd leading cause of death in the world (1). The incidence rate is the highest in Asia and lowest in Africa (1). Cholangiocarcinoma (CCA) is rare, but it may be found after an initial diagnosis as GC. In 1.1–4.7% of GC cases, at least 1 other primary tumor can be found (1). The most common tumors associated with GC are colon cancer, lung cancer, and hepatocellular carcinoma. CCA is relatively rare, and is found synchronously in 1.6% of all GCs (2). However, the incidence rate of CCA is rising (2).
The risk of GC and CCA is associated with deoxyribonucleic acid (DNA) replication defects, DNA methylation, and chromosomal and microsatellite instability. Surgery alone is the standard of care for early-stage GC, while surgery and chemotherapy with or without radiation therapy is used for advanced-staged GC and CCA (2,3). However, even after aggressive therapy, recurrence rates are high (4). Additionally, the mortality rate is high because most patients are diagnosed relatively late (4). Thus, it is necessary to understand the molecular mechanism involved in the carcinogenesis to determine prognostic biomarkers and potential treatment targets.
High-throughput sequencing technology provides a new perspective on the genomic, transcriptome, and epigenome characteristics of cancer. Systems biology can effectively analyze complex human diseases, especially in large-scale cancer data sets (5). The weighted-gene co-expression network analysis (WGCNA) is an accurate and efficient multigene analysis method, and it is possible to construct a scale-free network to investigate the correlation between different genomes and genes. WGCNAs have been used to identify the clinical modules and hub genes of cancer (6).
Lipid metabolism reprogramming has always been regarded as a new method for identifying malignant tumors, and there is increasing evidence from clinical and experimental studies that lipid metabolism disorder plays a key role in tumorigenesis (7,8). The pathogenesis of gastric cancer is complex and diverse, in which lipid metabolism plays a crucial role. Increasing the synthesis of new lipids or the uptake of exogenous lipids can promote the rapid growth of cancer cells and the formation of tumors. Lipids are not only the structural basis of biofilm, but also signal molecules and energy. It is worth noting that lipid metabolism can induce drug resistance of gastric cancer cells by reshaping the tumor microenvironment. Highly proliferative human CCA cells rely on lipid and lipoprotein uptake to promote fatty acid catabolism, suggesting that inhibiting fatty acid oxidation and/or lipid uptake may be the treatment strategy of this subclass of CCA (2,3). Some studies have shown that good control of blood lipid levels can improve the tumor immune microenvironment (7,8). The tumor immune microenvironment (TIME) reflects the immune landscape of the tumor microenvironment, which is very important in the occurrence and development of tumors (6). Immune cells play a key role in cell reprogramming. Immune cells can modify the microenvironment of tumor cells by secreting various biological factors, impacting tumorigenesis (8,9). Thus, studying the immune microenvironment of lipid metabolism-related genes (LMRGs) is of great significance in tumor risk assessment and biomarker screening.
As such, we examined hub genes and role of lipid metabolism in the development and diagnosis of GC and CCA. This study used WGCNA to analyze the pathogenesis of GC and CCA. The ribonucleic acid (RNA) sequencing data of the GC and CCA samples were downloaded from TCGA, and the differentially expressed genes (DEGs) between GC, CCA, and normal tissues were analyzed to determine their expression and function levels. A Gene Ontology (GO) analysis was conducted to examine the functional enrichment of the DEGs. A WGCNA of the DEG matrix identified the modules related to the clinical characteristics of patients with GC and CCA. The hub genes identified by the bioinformatics analysis were verified by a survival analysis. Finally, we analyzed the immune microenvironment of GC and CCA as well as how genes related to lipid metabolism impact tumorigenesis. It is different from previous reports that our research explored the common hub genes of gastric cancer and CCA. We present the following article in accordance with the STREGA reporting checklist (available at https://atm.amegroups.com/article/view/10.21037/atm-22-3530/rc).
Methods
Data collection and pre-processing
The RNA-sequencing data and clinical information of patients were obtained from the “Genomic Data Commons (GDC) TCGA Stomach Cancer (STAD)” and “GDC TCGA Bile Duct Cancer (CHOL)” data sets, hosted by the Xena website of the University of California at Santa Cruz (http://xena.ucsc.edu/). The RNA-sequencing data included 415 GC samples and 35 normal tissue samples, and 36 CHOL samples and 9 normal tissue samples. The workflow of the study is shown in Figure 1. The study was conducted in accordance with the Declaration of Helsinki (as revised in 2013).
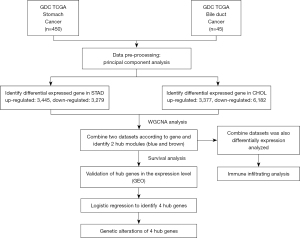
Identification of DEGs and statistical analysis
The “limma” package in R language (version 4.1.2) was used to identify the DEGs between STAD, CHOL, and normal tissues. A DEG was defined as a DEG with a log2(fold change) value >1 and a P value <0.01. The volcano map of the DEGs was drawn using the “ggplot2” software package.
Functional enrichment of DEGs
After using “org.Hs.eg.db” package (version: 3.10) to convert the DEG identifiers, “clusterprofiler” software package (version: 3.14.3) was used to perform the function enrichment analysis of the DEG based on GO dataset. The GO terms with a P value <0.05 were considered statistically significant.
WGCNA
WGCNA package (version: 1.70-3) was used to construct the DEG networks. First, ineligible genes and samples were removed using the “good samples genes” function to filter the expression matrix in the WGCNA. Second, the samples were clustered using the “flash cluster” tool in R. Third, the Pearson correlation coefficient matrix was calculated for the paired gene comparison. Fourth, using the “picks of threshold” function, the appropriate soft threshold power (β) was selected to ensure that the network was scale-free. Finally, the “power” function was used to construct the adjacency matrix.
Identification of clinically significant modules and hub genes
First, we described the characteristics of each gene expression module. Second, we calculated the correlation between the characteristic genes and clinical characteristics to determine which modules had clinical significance. The linear relationship between gene expression and clinical features had significance (GS). If the GS was closely related to the module members (MMs), it was defined as the correlation between the module feature genes and single gene expression profiles, and the hub genes of the module were related to CHOL’s STAD. These hub genes were candidate hub genes. Since there were >20 related hub genes, we used a logistic regression to further screen the most important hub genes.
Validation of hub genes
The Gene Expression Profiling Interactive Analysis (GEPIA) website was used to study the expression levels of hub genes in the CHOL STAD sample (http://gepia.cancer-pku.cn/). The ability of the hub genes to predict survival was evaluated by a Kaplan-Meier analysis using the “survival” software package (version: 3.2-7). First, we obtained the DEG expression profiles and the prognostic data of the tumor samples of STAD and CHOL from TCGA. Second, we determined the median expression value of each gene. The samples were assigned to the “high expression” or “low expression” group according to whether the expression level of a specific gene was above or below the median. A log-rank test was used to evaluate the significance of the survival difference between the high expression group and low expression group. If the test was associated with a P value <0.05, the gene was considered an effective hub gene.
Next, we screened the differences in hub gene expression between the normal and STAD and CHOL tissues according to the data of colon adenocarcinoma and rectal adenocarcinoma in TCGA and the Genotype Tissue Expression Project (GTEX) on the GEPIA website. The expression level was standardized by its mean value, and a difference with a P value <0.05 was considered statistically significant. By using the “ggpubr” software package (version: 0.4.0) and the GSE66229 data set (STAD) and GSE26566 data set (CHOL) in Gene Expression Omnibus (GEO) database, the expression differences between STAD with CHOL and normal tissues were analyzed to further verify the hub genes (https://www.ncbi.nlm.nih.gov/geo/). The independent sample test was adopted as the standard.
We mapped the genome of the hub genes, including the mutation, copy number variation, and messenger RNA expression Z-score (rnaseqv2 rsem), using the data of the STAD and CHOL samples in the data set in the TCGA pan cancer map. We also used the mutation mapping tool to describe the mutation of each hub gene. We used the cBioPortal to access and analyze the data (http://www.cbioportal.org/).
Selection of the subgroups and the TIME evaluation
First, the univariate Cox regression analysis showed that 11 genes were associated with the prognosis of CHOL complicated with STAD. According to the expression matrix of the 11 genes, the R software package “consensusclusterplus” (version: 1.58.0) was used. For consistency clustering, the expression data (estimation) algorithm was used to estimate the stromal cells and immune cells in the malignant tumor tissues, and the stromal score, immune score, and tumor purity were calculated.
Immune analyses
A TIMER immune-infiltration analysis was conducted to evaluate the abundance of 6 immune infiltrating cells [i.e., B cells, dendritic cells, macrophages, neutrophils, cluster of differentiation (CD)4 T cells, and CD8 T cells]. Data, including data for 28 immune infiltrating cells and related genes, were obtained from the molecular feature database, and the concentration of 28 immuno-invasive cells in the tumor samples was evaluated by a single sample gene set enrichment analysis (SSGSEA).
Results
Pre-processing of data
Our findings were obtained from 415 GC samples, 35 normal tissue samples, 36 CCA samples, and 9 normal tissue samples. It is common practice to distinguish between the two main components of tumor samples and normal samples (8). After a principal component analysis, we found that both CHOL and STAD had obvious clusters (see Figure 2A-2C).
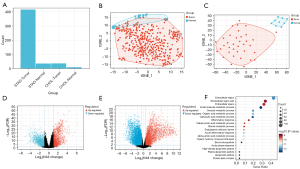
Identification of DEGs in the STAD with CHOL samples and the GO enrichment analysis
In 44 normal samples, 36 CHOL, and 415 STAD samples, a total of 908 DEGs were identified, including 450 upregulated genes and 458 downregulated genes. STAD volcanic plot is 2D and CHOL volcanic plot is 2E (see Figure 2D,2E). To explore the potential biological function of the DEGs in the STAD, we conducted an enrichment analysis (see Figure 2F). The upregulated DEGs were mainly involved in extracellular regions and small molecule metabolism. In contrast to oxyacetic acid metabolism and organic acid metabolism (see Figure 2F), the downregulated DEGs were mainly involved in the development of the nervous system, cell projection, and neurogenesis. These results are consistent with the known STAD dysfunction in STAD and CHOL patients; thus, our results appear to be reliable.
WGCNA and identification of critical modules
A WGCNA was used to build a network based on the DEG expression matrix and clinical data from the STAD and CHOL samples. We performed a cluster analysis and checked the quality of the STAD and CHOL samples. All the samples were in the cluster and within the threshold range (height <200). Thus, there were no outliers that needed to be removed. The WGCNA applied the following 6 clinical variables (see Figure 3A): disease status (normal or tumor), gender (female or male), pathological stage (I–IV), sample type (primary tumor or normal solid tissue), age, weight, and survival time. The STAD sample containing CHOL was divided into the tumor sample and the normal sample (10).
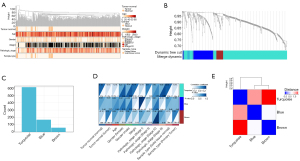
To build a scalable network, we used a soft threshold power β set to 7. The independence level was set to 0.9, and the average connectivity was close to 0. We clustered DEGs for same DEGs of grouping with the same expression module and merged the module with a <0.25 height difference (11). This process resulted in the following 3 co-expression modules: turquoise, blue, and brown (see Figure 3B,3C).
The characteristic gene of the blue module [correlation (COR) =0.31, P=4.8×10−5] and turquoise module (COR =0.19, P=3.7×10−6) was significantly positively correlated with STAD and CHOL (see Figure 3D). These correlations were confirmed by hierarchical clustering, heat map, and adjacency analyses (see Figure 3E). The results suggested that the cyan and blue modules may contribute to the tumorigenesis of CHOL and STAD. Thus, the turquoise and blue modules were analyzed based on hub genes (12).
Identification of candidate hub genes from the turquoise and blue modules
In the turquoise and blue modules, the MMs and GS scores were strongly positively correlated. The selection criteria for the hub genes were relatively lower than the standard cut-off threshold (MM >0.8). In the turquoise module, the genes identified reached the thresholds of a “cor.gene” module membership >0.75 and a “cor.genetraitsignicac” >0.6. In the blue module, the genes met the thresholds of a “cor.gene module membership” >0.75 and a “cor.gene traitsential” >0.7.
Hub gene expression and correlation with survival
Based on the expression data and clinical information of the STAD containing CHOL tumor samples in TCGA, we studied the potential association between the expression of 37 genes identified in the turquoise module and 9 genes identified in the blue module and patient survival. The details of TCGA participants are shown in Tables 1,2. To further analyze the relationship between the genes in the module and survival status, a forest plot was generated (see Figure 4A). There were over 20 candidate hub genes, and we conducted a logistic regression to identify 4 important hub genes. The turquoise module genes APBB1, ARMCX1, and MSRB3 were associated with prognosis, as was the blue module gene DZIP1. The increased expression of these genes was associated with inferior overall survival (OS), the OS details of four genes was as follow: low level APBB1 had hazard ratio (HR) 1.46 (95% CI: 1.08, 1.97) with P value 0.01 compared with high level, low level ARMCX1 had HR 1.48 (95% CI: 1.10, 2.00) with P value 0.01, low level DZIP1 had HR 1.62 (95% CI: 1.20, 2.19) with P value <0.001 and low level MSRB3 had HR 1.54 (95% CI: 1.14, 2.08) with P value <0.001 (see Figure 4B). Thus, we defined these genes as the “final” hub genes.
Table 1
Stomach cancer | Dead (N=280) | Alive (N=170) | Total (N=450) | P value |
---|---|---|---|---|
Tumor-normal, n (%) | 0.32 | |||
Normal | 25 (5.6) | 10 (2.2) | 35 (7.8) | |
Tumor | 255 (56.7) | 160 (35.6) | 415 (92.2) | |
Age (years), mean ± SD | 65.18±11.06 | 66.68±9.78 | 65.75±10.60 | 0.13 |
Gender, n (%) | 0.18 | |||
Female | 106 (23.6) | 53 (11.8) | 159 (35.3) | |
Male | 174 (38.7) | 117 (26.0) | 291 (64.7) | |
Weight (kg), mean ± SD | 67.55±22.59 | 65.21±18.72 | 66.63±24.59 | 0.24 |
Pathologic stage, n (%) | <0.001 | |||
Stage I | 47 (11.1) | 15 (3.5) | 62 (14.6) | |
Stage II | 101 (23.7) | 40 (9.4) | 141 (33.1) | |
Stage III | 96 (22.5) | 82 (19.3) | 178 (41.8) | |
Stage IV | 20 (4.7) | 25 (5.9) | 45 (10.6) | |
Sample type, n (%) | 0.32 | |||
Primary tumor | 255 (56.7) | 160 (35.6) | 415 (92.2) | |
Solid tissue normal | 25 (5.6) | 10 (2.2) | 35 (7.8) |
GDC, Genomic Data Commons; TCGA, The Cancer Genome Atlas; SD, standard deviation.
Table 2
Cholangiocarcinoma | Dead (N=23) | Alive (N=22) | Total (N=45) | P value |
---|---|---|---|---|
Tumor-normal, n (%) | 1 | |||
Normal | 5 (11.1) | 4 (8.9) | 9 (20.0) | |
Tumor | 18 (40.0) | 18 (40.0) | 36 (80.0) | |
Age (years), mean ± SD | 63.87±12.13 | 65.27±13.04 | 64.56±12.46 | 0.71 |
Gender, n (%) | 1 | |||
Female | 12 (26.7) | 11 (24.4) | 23 (51.1) | |
Male | 11 (24.4) | 11 (24.4) | 22 (48.9) | |
Weight (kg), mean ± SD | 81.43±23.42 | 81.91±19.57 | 81.67±21.38 | 0.94 |
Pathologic stage, n (%) | 0.37 | |||
Stage I | 12 (26.7) | 14 (31.1) | 26 (57.8) | |
Stage II | 5 (11.1) | 5 (11.1) | 10 (22.2) | |
Stage III | 0 (0.0) | 1 (2.2) | 1 (2.2) | |
Stage IV | 6 (13.3) | 2 (4.4) | 8 (17.8) | |
Sample type, n (%) | 1 | |||
Primary tumor | 18 (40.0) | 18 (40.0) | 36 (80.0) | |
Solid tissue normal | 5 (11.1) | 4 (8.9) | 9 (20.0) |
GDC, Genomic Data Commons; TCGA, The Cancer Genome Atlas; SD, standard deviation.
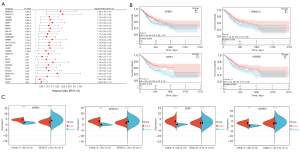
Using the GEPIA website, we confirmed that there were significant differences in the expression of all these hub genes in the normal and CHOL bearing STAD tissues (see Figure 4C). To further validate the genes related to survival, we used the GEO data sets GSE66229 and GSE26566 to identify the significant survival-related genes for STAD and CHOL, respectively (see Figure 5A,5B). The results of TCGA analysis were consistent with those of the GEO analysis.
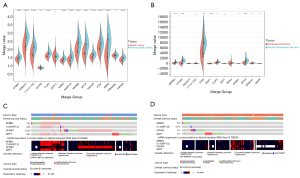
Mutation landscape of hub genes
According to the data of the STAD patients with CHOL in TCGA, the OncoPrint view of the hub genes in the cBioPortal database was used to visualize the mutation of the 4 hub genes. In some STAD patients, there were 4 hub gene mutations (29%). In a small number of CHOL patients (11%), there were 4 hub gene mutations. DZIP1 had the highest STAD mutation rate (8%), and the most missense mutations, and mutations leading to a higher expression of messenger RNA (see Figure 5C), while DZIP1 (4%) had the highest cholesterol mutation rate (see Figure 5D).
Clustering of 2 molecular subtypes based on LMRGs
According to the 11 prognostic genes generated by the univariate Cox analysis, the patients with STAD and CHOL in the training cohort were divided into subgroups using the consistent clustering method (see Figure 6A-6C). When k=2, the optimal clustering stability was reached. In total, 332 patients were clustered in group 1 and 163 patients were clustered in group 2. The heat maps showed the expression levels of the LMRGs in the two subtypes (see Figure 6D), and a significant difference in expression between cluster 1 and cluster 2 was found. These results suggest that the LMRGs divide the STAD with CHOL patients into two molecular subtypes.
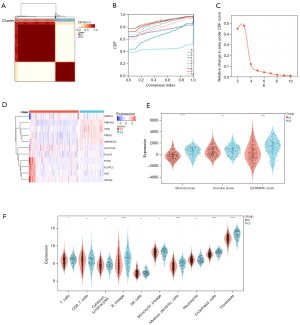
Two molecular subtypes exhibited different TIME and immune status
Additionally, we also performed immunoassays to explore the immune differences between the two molecular subtypes. The estimation algorithm showed that the immune score (P<0.05) and estimated score (P<0.05) of the patients with CHOL in group 2 were significantly lower than those of patients in group 1. Cluster 1 had a lower stromal score than cluster 2 (P<0.05). Additionally, the TIMER algorithm showed that cluster 1 had a greater number of B cells (P<0.05), macrophages (P<0.05), NK cells (P<0.05), CD4 T cells (P<0.05), CD8 T cells (P<0.05), endothelial cells (P<0.05), and natural killer cells (P<0.05) (Figure 6E,6F). These results showed that there were significant differences in the TIME and immune status between the two molecular subtypes.
Discussion
In this study, the WGCNA method was used to analyze the pathogenesis of GC and CCA. The RNA-sequencing data of the GC and CCA samples were downloaded from TCGA, and the DEGs between the GC, CCA, and normal tissues were analyzed in relation to their expression and function levels. The GO database was used to analyze the functional enrichment of DEGs. The WGCNA of the DEG matrix identified modules related to the clinical characteristics of patients with GC and CCA. The hub genes found by the bioinformatics analysis were verified by survival rates, and an immunohistochemical GC and CCA analysis. Additionally, an in-depth analysis of the lipid metabolism genes was conducted to explore the effect of lipid metabolism on the pathogenesis of GC and CCA patients, and to analyze the immune microenvironments of GC and CCA patients.
This study provides a relatively novel idea for combination of four cancer hub genes. We performed a bioinformatics analysis on an independent group of patients to identify biomarkers. Our results suggest that the poor prognosis of GC and CCA is related to the overexpression of APBB1, ARMCX1, DZIP1, and MSRB3. Our results of the functional enrichment analysis of the GC and CCA genes are consistent with previous reports (13,14). The metabolism of cancer cells plays an important role in the occurrence and development of tumors.
Through the WGCNA, we found that central modules and hub genes play an important role in cancer development. We identified two key modules (i.e., the turquoise and blue modules), whose genes were associated with GC and CCA. Complex gene networks regulate the occurrence and development of GC and CCA. We found that 4 hub genes (i.e., APBB1, ARMCX1, DZIP1 and MSRB3) were closely related to the OS rate of GC patients. ARMCX1 has been reported to have potential prognostic value for GC and may have clinical application value (13). However, further basic research needs to be conducted to clarify the specific mechanism of ARMCX1 in GC. Both ARMCX1 and ARMCX2 are involved in the biological pathways and processes related to the progression and treatment of GC. Additionally, DZIP1 is an independent prognostic factor of GC and may be involved in the progression of GC (14). DZIP1 is involved in the activation of the emergency phenotype and is related to the massive infiltration of a variety of immune cells. Additionally, the DZIP1 mutation may be related to a good prognosis in GC patients. Finally, the methylation of DZIP1, a DNA promoter, can be used as a target for future GC therapy. Additionally, patients with GC have increased expression of MSRB3 which is associated with poor prognosis (15). Research on APBB1 in GC is limited, but it may be a potential biomarker of GC. Since the completion of this study on CCA biomarkers, we have not found any other relevant studies on these 4 CCA hub genes.
Consensus clustering is a reliable method for dividing samples into different subgroups based on gene expression matrices. According to the LMRG expression matrix of the GC and CCA patients, we first identified 2 molecular subgroups by consensus clustering. Next, an immune function analysis was carried out to examine the role of LMRGs in GC and CCA (16,17). Since the progress of a tumor is related to changes in the surrounding matrix, and the immune cell is the important component of the tumor matrix, the TIME plays the important role in the prognosis of the patient (18). Additionally, the abnormal metabolism of cancer cells will lead to a change in the metabolism of the TIME (19). TIME was different between the two subgroups that cluster 1 was more immunogenic. Cluster 1 may benefit more because of greater abundance of CD8 T cells. In fact, recent trials (TOPAZ-1 and KEYNOTE-811) have shown that immunotherapy can improve clinical outcomes in patients with GC and CCA (20,21).
The estimation algorithm is an innovative method for calculating tumor purity and the ratio of immune cells and stromal cells in tumors according to gene expression values (22). The immune score produced by the estimation algorithm quantitatively shows the immune component in the tumor sample and reflects the TIME (23). The purity of a tumor is defined as the proportion of the malignant cells in the tumor tissue, and it is significantly correlated with the prognosis of the tumor (24). Consistent with previous findings, we found that patients with a better prognosis had a higher immune score. The cluster 1 immune score was higher than that of the cluster 2. Additionally, we also used TIMER to evaluate the immune status of these 2 molecular subsets, which was helpful in quantifying the 6 tumor infiltrating immune subsets. The TIMER analysis showed that the abundance of all the immune cells in Cluster 1 increased significantly, which indicated that the immune cells in Cluster 1 were upregulated. In addition, four hug genes APBB1, ARMCX1, DZIP1, and MSRB3 were related with TIME of GC or CCA.
In conclusion, this study conducted a WGCNA and found 4 hub genes implicated in GC and CCA: APBB1, ARMCX1, DZIP1, and MSRB3. These findings are helpful in fully understanding the gene network of GC and CCA (25). Through consensus clustering, 2 molecular subtypes were identified in GC and CCA based on the LMRGs. The immunoassays showed that abnormal lipid metabolism hinders the immune system. Further studies are warranted to determine how to best use this molecular information to improve clinical outcomes in GC and CCA.
Acknowledgments
The authors appreciate the academic support from the AME Gastrointestinal Cancer Collaborative Group.
Funding: None.
Footnote
Reporting Checklist: The authors have completed the STREGA reporting checklist. Available at https://atm.amegroups.com/article/view/10.21037/atm-22-3530/rc
Conflicts of Interest: All authors have completed the ICMJE uniform disclosure form (available at https://atm.amegroups.com/article/view/10.21037/atm-22-3530/coif). The authors have no conflicts of interest to declare.
Ethical Statement: The authors are accountable for all aspects of the work in ensuring that questions related to the accuracy or integrity of any part of the work are appropriately investigated and resolved. The study was conducted in accordance with the Declaration of Helsinki (as revised in 2013).
Open Access Statement: This is an Open Access article distributed in accordance with the Creative Commons Attribution-NonCommercial-NoDerivs 4.0 International License (CC BY-NC-ND 4.0), which permits the non-commercial replication and distribution of the article with the strict proviso that no changes or edits are made and the original work is properly cited (including links to both the formal publication through the relevant DOI and the license). See: https://creativecommons.org/licenses/by-nc-nd/4.0/.
References
- Rawla P, Barsouk A. Epidemiology of gastric cancer: global trends, risk factors and prevention. Prz Gastroenterol 2019;14:26-38. [Crossref] [PubMed]
-
. Available online: https://www.nccn.org/professionals/physician_gls/pdf/hepatobiliary.pdfNCCN Clinical Practice Guidelines in Hepatobiliary Cancers -
. Available online: https://www.nccn.org/professionals/physician_gls/pdf/gastric.pdfNCCN Clinical Practice Guidelines in Gastric Cancers - Koushyar S, Powell AG, Vincan E, et al. Targeting Wnt Signaling for the Treatment of Gastric Cancer. Int J Mol Sci 2020;21:3927. [Crossref] [PubMed]
- Feng X, Liu S, Cheng H, et al. Weighted Gene Co-Expression Network Analysis Reveals Hub Genes Contributing to Fuzz Development in Gossypium arboreum. Genes (Basel) 2021;12:753. [Crossref] [PubMed]
- Zuo Z, Shen JX, Pan Y, et al. Weighted Gene Correlation Network Analysis (WGCNA) Detected Loss of MAGI2 Promotes Chronic Kidney Disease (CKD) by Podocyte Damage. Cell Physiol Biochem 2018;51:244-61. [Crossref] [PubMed]
- Ma K, Zhang L. Overview: Lipid Metabolism in the Tumor Microenvironment. Adv Exp Med Biol 2021;1316:41-7. [Crossref] [PubMed]
- Fukunaga-Kalabis M, Santiago-Walker A, Herlyn M. Matricellular proteins produced by melanocytes and melanomas: in search for functions. Cancer Microenviron 2008;1:93-102. [Crossref] [PubMed]
- Yu W, Lei Q, Yang L, et al. Contradictory roles of lipid metabolism in immune response within the tumor microenvironment. J Hematol Oncol 2021;14:187. [Crossref] [PubMed]
- Ye X, Liang T, Deng C, et al. MSRB3 promotes the progression of clear cell renal cell carcinoma via regulating endoplasmic reticulum stress. Pathol Res Pract 2020;216:152780. [Crossref] [PubMed]
- Zhang B, Zhou BH, Xiao M, et al. KDM5C Represses FASN-Mediated Lipid Metabolism to Exert Tumor Suppressor Activity in Intrahepatic Cholangiocarcinoma. Front Oncol 2020;10:1025. [Crossref] [PubMed]
- Mannucci A, Zuppardo RA, Crippa S, et al. MSH6 gene pathogenic variant identified in familial pancreatic cancer in the absence of colon cancer. Eur J Gastroenterol Hepatol 2020;32:345-9. [Crossref] [PubMed]
- Xie A, Wang P, Chen D, et al. Aberrant ARMCX1 Expression Is an Independent Predictor of Poor Prognosis in Gastric Cancer. J Oncol 2022;2022:9348917. [Crossref] [PubMed]
- Liu YJ, Li JP, Zeng SH, et al. DZIP1 Expression as a Prognostic Marker in Gastric Cancer: A Bioinformatics-Based Analysis. Pharmgenomics Pers Med 2021;14:1151-68. [Crossref] [PubMed]
- Ma X, Wang J, Zhao M, et al. Increased expression of methionine sulfoxide reductases B3 is associated with poor prognosis in gastric cancer. Oncol Lett 2019;18:465-71. [Crossref] [PubMed]
- Minchenko OH, Tsuchihara K, Minchenko DO, et al. Mechanisms of regulation of PFKFB expression in pancreatic and gastric cancer cells. World J Gastroenterol 2014;20:13705-17. [Crossref] [PubMed]
- Park TJ, Han SU, Cho YK, et al. Methylation of O(6)-methylguanine-DNA methyltransferase gene is associated significantly with K-ras mutation, lymph node invasion, tumor staging, and disease free survival in patients with gastric carcinoma. Cancer 2001;92:2760-8. [Crossref] [PubMed]
- Ge Q, Li G, Chen J, et al. Immunological Role and Prognostic Value of APBB1IP in Pan-Cancer Analysis. J Cancer 2021;12:595-610. [Crossref] [PubMed]
- Tan R, Zhang G, Liu R, et al. Identification of Early Diagnostic and Prognostic Biomarkers via WGCNA in Stomach Adenocarcinoma. Front Oncol 2021;11:636461. [Crossref] [PubMed]
- Takei S, Kawazoe A, Shitara K. The New Era of Immunotherapy in Gastric Cancer. Cancers (Basel) 2022;14:1054. [Crossref] [PubMed]
- A phase 3 randomized, double-blind, placebo-controlled study of durvalumab in combination with gemcitabine plus cisplatin (GemCis) in patients (pts) with advanced biliary tract cancer (BTC): TOPAZ-1. Available online: https://meetings.asco.org/abstracts-presentations/204876
- Katoh Y, Katoh M. Hedgehog signaling pathway and gastrointestinal stem cell signaling network Int J Mol Med 2006;18:1019-23. (review). [Crossref] [PubMed]
- Zheng X, Wang X, Zheng L, et al. Construction and Analysis of the Tumor-Specific mRNA-miRNA-lncRNA Network in Gastric Cancer. Front Pharmacol 2020;11:1112. [Crossref] [PubMed]
- Nakazawa MA, Tamada Y, Tanaka Y, et al. Novel cancer subtyping method based on patient-specific gene regulatory network. Sci Rep 2021;11:23653. [Crossref] [PubMed]
- Pfrepper C, Knödler M, Schorling RM, et al. Predictors for thromboembolism in patients with cholangiocarcinoma. J Cancer Res Clin Oncol 2021; Epub ahead of print. [Crossref] [PubMed]
(English Language Editor: L. Huleatt)