Identification of molecular subtypes in lung adenocarcinoma based on DNA methylation and gene expression profiling—a bioinformatic analysis
Introduction
Lung cancer is the leading cause of death and morbidity globally, and accounts for 18.4% of cancer-related deaths and 11.6% of total cancer cases (1). Non-small cell lung carcinomas (NSCLCs) and small-cell carcinomas represent 85% of lung cancers. Lung adenocarcinoma (LUAD) represents up to 40% of NSCLCs (2) and is highly invasive and metastatic, with a dismal 5-year survival rate of 19.5% (3). Despite substantial improvements in computed-tomography imaging, bronchoscopy, sputum cytology, and therapy (4), patient survival rates remain unsatisfactory. Thus, it is necessary to explore the key factors and molecular mechanisms associated with lung cancer to refine current diagnostic and therapeutic strategies.
In cancer, deviant epigenetic regulation includes miRNA gene silencing, DNA methylation, mRNA and non-coding RNA methylation, histone methylation, and histone acetylation (5). Deoxyribonucleic acid (DNA) methylation is an important mechanism of gene epigenetics and is involved in regulating gene expression and cell differentiation. DNA methylation regulates gene expression by recruiting proteins involved in gene repression or by inhibiting the binding of transcription factor(s) to DNA (6). Methylation at the 5th carbon atom of cytosine residues is the most widely studied epigenetic modification in plants and mammals. In mammals, DNA methylation mainly occurs in cytosine-phosphoric acid-guanine (CpG) islands (i.e., 300–3,000 bp DNA fragments rich in CpG dinucleotide), and 40% of the gene promoter regions contain CpG islands (7). Studies have shown that many tumors, including LUAD, undergo methylation at an early stage (8,9), and the progression of tumors is positively correlated with the accumulation of aberrant DNA methylation (10). DNA methylation is a genetic modification that does not change the DNA sequence and is associated with the subtypes and prognosis of LUAD. Although cumulating evidences have demonstrated the abnormal DNA methylations level in LUAD, the comprehensive regulatory network and pathways analyses of DNA methylation levels and miRNA epigenetic alterations have not yet been conducted (11).
Previous studies have reported different LUAD subtypes based on methylation or gene expression (12,13). Moreover, methylation and gene expression have been combined to identify the pathologic subtypes of NSCLC, including small cell lung cancer and LUAD, instead of molecular typing (14). However, it has been established that methylation and gene expression are intrinsically linked, and most importantly, methylation can affect gene expression. Thus, this study sought to classify LUAD samples by integrating methylation and transcriptome data to find a novel typing approach and identify novel genes that affect prognosis and diagnosis to provide a theoretical basis for individualized treatment. We present the following article in accordance with the STREGA reporting checklist (available at https://atm.amegroups.com/article/view/10.21037/atm-22-3340/rc).
Methods
Study design
This is a bioinformatics analysis study and the molecular subtypes of LUAD were identified based on DNA methylation and gene expression profiling. The study was conducted in accordance with the Declaration of Helsinki (as revised in 2013).
Data download and preliminary process
Gene expression and DNA methylation data sets of LUAD (comprising 59 normal and 526 tumor samples) were obtained from The Cancer Genome Atlas (TCGA) (https://www.cancer.gov/about-nci/organization/ccg/research/structural-genomics/tcga). The differential methylation sites were identified using the ChAMP package based on paired tumor and non-tumor samples (n=24). The differentially expressed genes (DEGs) were screened using the R package limma [the screening criteria were a |log fold change (FC)| value >0.5 and a P value <0.05 for the gene expression and methylation sites].
Identification of molecular subtypes related to prognosis
In total, 454 LUAD samples with prognostic information, gene expression, and methylation data from TCGA data sets were used for the molecular typing. Methylation sites with missing values were removed from the data set before analysis. First, feature selection was applied to select the most variable genes based on their expression, and a Cox regression analysis was conducted to predict the methylation sites. The LUAD samples were also classified into different subtypes using the non-negative matrix factorization (NMF) method using the R package CancerSubtypes. NMF (15) is currently recognized as one of the most effective clustering methods based on omics features. The effectiveness of the clustering was measured by the silhouette width, which ranges from –1 to 1. The larger the silhouette width, the better the degree of separation. The default number of runs was set to 30. The DEGs between the molecular subtypes were screened using the following criteria: a |logFC| value >0.8 and a false discovery rate (FDR) <0.05 in the limma package, and an FDR <0.01 for the methylation sites in the ChAMP package.
Analysis of differences among different subtypes and validation
The clinical characteristics and prognostic data between the clusters were compared using the t-test, chi-square test, or rank-sum test as appropriate in the R package tableone. A P value <0.05 was considered statistically significant. We downloaded the GSE32863 data set (tumor samples, n=58), which included gene expression, methylation sites, and clinical data, such as recurrence and tumor stage data, from the Gene Expression Omnibus (GEO) database as a validation set. The GSE32863 data set was classified according to the expression of the DEGs and methylation sites, and the clinical data among the different subtypes were compared (see Table 1). The “Complex Heatmap” package was then used to draw heatmaps.
Table 1
Characteristics | Subtype 1 (n=37) | Subtype 2 (n=21) | P |
---|---|---|---|
Age (years), mean (SD) | 69.41 (9.05) | 66.29 (9.06) | 0.213 |
Gender (male), n (%) | 8 (21.6) | 5 (23.8) | 1.000 |
Smoking status (never), n (%) | 22 (59.5) | 7 (33.3) | 0.101 |
Stage, n (%) | 0.003 | ||
Stage I | 28 (75.7) | 6 (28.6) | |
Stage II | 3 (8.1) | 8 (38.1) | |
Stage III | 6 (16.2) | 6 (28.6) | |
Stage IV | 0 (0.0) | 1 (4.8) | |
Recurrence (yes), n (%) | 10 (27.0) | 9 (42.9) | 0.345 |
KRAS mutation type, n (%) | 0.757 | ||
G12A | 1 (2.9) | 1 (4.8) | |
G12C | 5 (14.3) | 2 (9.5) | |
G12D | 4 (11.4) | 1 (4.8) | |
G12E | 0 (0.0) | 1 (4.8) | |
G12V | 3 (8.6) | 2 (9.5) | |
G13V | 1 (2.9) | 0 (0.0) | |
WT | 21 (60.0) | 14 (66.7) |
SD, standard deviation; WT, wild type.
Gene Ontology (GO) term enrichment and Kyoto Encyclopedia of Genes and Genomes (KEGG) pathway analyses
GO and KEGG pathway enrichment analyses were performed to identify the key genes and pathways involved in LUAD. The GO functional annotation was composed of cellular components (CCs), molecular functions (MFs), and biological processes (BPs). KEGG is a public database used to analyze gene-related pathways to explore biological systems (16). The “clusterProfiler” and “ggplot2” R packages were used to visualize the enrichment results.
Construction of protein-protein interaction (PPI) network and analysis of representative genes
The PPI network of the representative genes and methylation sites was generated using Search Tool for the Retrieval of Interacting Genes/Proteins (STRING; http://string-db.org) (17). To obtain the best results, the minimum required interaction score was set to 0.4. The PPI network was visualized with Cytoscape 3.9.0 (18) and the MCODE plug-in was used to build subnetworks using the following parameters: a MCODE score >5, degree cutoff =2, node score cutoff =0.2, node density cutoff =0.1, k-score =2, and maximum depth =100 (19).
Statistical analysis
The distribution of the differentially expressed genes was shown by heatmap and volcano map. The differences of gene expression between the two groups were compared by t-test and expressed by boxplot. All the statistical analyses were performed by using R software (Version 4.1.1). A P value <0.05 was considered as statistical significance.
Results
Data download and preliminary process
The data sets of LUAD expression and methylation obtained from TCGA included 59 normal and 526 tumor samples. The workflow of the data analysis conducted in this study is shown in Figure 1. First, we analyzed the difference in gene expression using the R package limma. The number of dysregulated genes was 2,874. The ChAMP package was used to identify the differential methylation sites based on paired tumor and non-tumor samples (n=24). The number of dysregulated methylation sites was 453 (for further details see Figure 2A,2B). After feature selection by Cox regression, 1,622 genes and 83 methylation sites were selected for molecular typing.
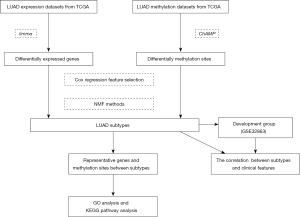
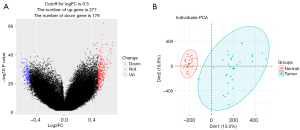
Identification of 2 subtypes by an unsupervised hierarchical cluster analysis
The average silhouette showed that the optimal number of cluster K was 2 (see Figure 3A,3B). We successfully divided LUAD into 2 subtypes using the NMF method, and the specific results are shown in Figure 3. The average silhouette width of the 2 subtypes was 0.98 (see Figure 4A). The survival rate differed significantly between the 2 groups (see Figure 4B). The heatmap of the sample similarity matrix is shown in Figure 4C. The relationships between stage, methylation site, and gene expression are shown in Figure 5. We next identified 379 DEGs genes (see Figure S1A) and 67 methylation sites (see Figure S1B) between the subtypes. The DEGs between the subtypes were screened using the limma package (criteria: a |logFC| value >0.8 and an FDR <0.05), and the methylation sites were screened using the ChAMP package (criteria: an adjusted P value <0.01). The corresponding genes of the methylation sites are shown in Table S1, included BCAT1, CDC42, DLX5, HOXA5, and OTX1, and were closely associated with the development of LUAD.
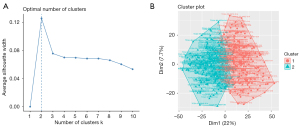
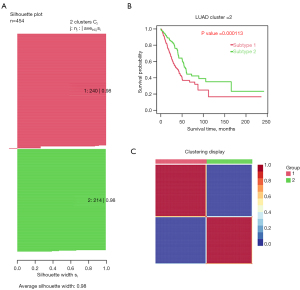
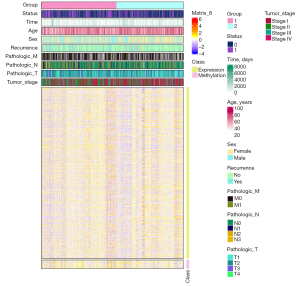
Analysis of differences among different subtypes
We also explored the differences in age, survival status, gender, recurrence, and tumor, node, metastasis (TMN) stage between the molecular subtypes. The survival rates were 0.18% and 0.25% for subtypes 1 and 2, respectively, with a mean survival time of 27.0 and 33.3 months, respectively (P=0.02) (see Table 2). With the exception of recurrence status, there were significant differences in the survival status, age, gender, pathologic M stage, pathologic N stage, pathologic T stage, and clinical stage between the 2 subtypes (see Table 2). Significant differences were found in the proportions of patients between subtype 1 (of whom 46.7%, 29.2%, 18.8%, and 5.0% had tumor stage I, II, III, and IV, respectively) and subtype 2 (of whom 64.5%, 18.2%, 11.7%, and 3.7% had tumor stage I, II, III, and IV, respectively) (P=0.001).
Table 2
Characteristics | Group 1 (n=240) | Group 2 (n=214) | P |
---|---|---|---|
Patient status (death), n (%) | 102 (42.5) | 61 (28.5) | 0.003 |
Survival time (months), mean (SD) | 27.0 (27.9) | 33.3 (32.4) | 0.02 |
Age (years), mean (SD) | 63.40 (10.57) | 66.63 (9.62) | 0.001 |
Sex (male), n (%) | 129 (53.8) | 82 (38.3) | 0.001 |
Recurrence | 0.22 | ||
Missing | 39 (16.3) | 33 (15.4) | |
No | 129 (53.8) | 131 (61.2) | |
Yes | 72 (30.0) | 50 (23.4) | |
Pathologic_M | 0.38 | ||
Missing | 72 (30.0) | 75 (35.0) | |
M0 | 156 (65.0) | 132 (61.7) | |
M1 | 12 (5.0) | 7 (3.3) | |
Pathologic_N | 0.001 | ||
Missing | 2 (0.8) | 9 (4.2) | |
N0 | 143 (59.6) | 157 (73.4) | |
N1 | 52 (21.7) | 28 (13.1) | |
N2 | 42 (17.5) | 20 (9.3) | |
N3 | 1 (0.4) | 0 (0.0) | |
Pathologic_T | 0.007 | ||
Missing | 1 (0.4) | 2 (0.9) | |
T1 | 65 (27.1) | 91 (42.5) | |
T2 | 145 (60.4) | 94 (43.9) | |
T3 | 21 (8.8) | 19 (8.9) | |
T4 | 8 (3.3) | 8 (3.7) | |
Tumor_stage | 0.001 | ||
Missing | 1 (0.4) | 4 (1.9) | |
Stage I | 112 (46.7) | 138 (64.5) | |
Stage II | 70 (29.2) | 39 (18.2) | |
Stage III | 45 (18.8) | 25 (11.7) | |
Stage IV | 12 (5.0) | 8 (3.7) |
SD, standard deviation; Pathologic_M, pathological metastasis; Pathologic_T, primary pathological tumor; Pathologic_N, pathological lymph node status.
GO and KEGG enrichment analysis and PPI network construction of hub genes
The DEGs and corresponding genes of the methylation sites were used to perform an enrichment analysis and construct a PPI network. The GO annotation showed the MFs included tubulin-binding, microtubule-binding, and DNA replication origin binding (see Figure S2A). The CCs included chromosomal region, spindle, and kinetochore (see Figure S2B). The BPs included organelle fission mitotic nuclear division, and sister chromatid segregation (see Figure S2C). The KEGG signaling pathways related to the hub genes mainly included the cell cycle, human T-cell leukemia virus (type 1) infection, inflammatory bowel disease, and the intestinal immune network for immunoglobulin A (IgA) production (see Figure S2D).
A PPI network was constructed using STRING to identify the hub genes (see Figure S3). The PPI network was imported into Cytoscape, and 3 subnetworks were generated using the MCODE plug-in. Hub genes in each subnetwork were defined by the degree of neighborhood connectivity. The hub genes of cluster 1, which included NCAPG, CCNB1, and DLGAP5, were correlated with cell cycle regulation. The hub genes of cluster 2, which included HLA-DQA1, HLA-DPA1, and HLA-DPB1, were correlated with T cell recruitment and anti-programmed death-1 (PD-1) therapy. Finally, the hub genes of cluster 3, which consisted of SPTPA, SFTPB and SFTPC, were correlated with anti-inflammatory processes (see Figure S4).
Validation of subtypes and the correlation between subtypes and clinical data in LUAD
Among the DEGs and corresponding genes of the methylation sites, the validation group (i.e., the GSE32863 data set) only comprised 298 genes and 2 methylation sites. Next, we classified the 58 samples into 2 subtypes using the NMF method (see Figure S5). Consistent with subtype 2 from the training data set, subtype 1 in the validation data set similarly exhibited high expressions of E2FB, CENPU, NDC80, TYMS, SC14L6, PLA2G1B, LHFPL3-AS1, C8orf34-AS1, and DMBT1. The gene corresponding to methylation site cg0544326 was downregulated in subtype 2 in the training data set and subtype 1 in the validation data set. Additionally, the hub genes of NCAPG, CCNB1, and DLGAP5 were overexpressed in subtype1 in the training data set (see Figure 5) and subtype 2 in the validation data set (see Figure S5). Consistent with the literature, we found that DLGAP5 overexpression in tumor patients was associated with a poor prognosis (20,21). The clinical data of the LUAD patients were also analyzed. In the validation group, the proportions of patients with stage I, II, III, IV were 28.6%, 38.1%, 28.6% and 4.8% in subtype 2, respectively, and 75.7%, 8.1%, 16.2% and 0.0% in subtype 1, respectively. Group 2 in the validation data set and subtype 1 in TCGA data set shared a similar expression pattern and advanced clinical stage.
Discussion
We successfully stratified LUAD into 2 clinically relevant subtypes with a high silhouette width (0.98) using unsupervised multi-omics integration methods. Subtype 1 in TCGA data set had a worse prognosis and higher tumor stage than subtype 2 (P<0.05). We also identified the representative genes and genes corresponding to the methylation sites for the 2 molecular types and validated our molecular typing results using an external validation data set. Group 2 in the validation data set exhibited a similar expression pattern to subtype 1 in TCGA data set, consisting of samples associated with more advanced tumor staging. Interestingly, the functional enrichment analysis showed that the representative genes and the corresponding genes of the methylation sites were enriched in GO terms, including “cell cycle” and “immune”, which are significantly correlated with the development of LUAD. The PPI network analysis generated 3 subnetworks. The identified hub genes in these subnetworks, including NCAPG, CCNB1, DLGAP5, HLA-DQA1, HLA-DPA1, HLA-DPB1, SPTPA, SFTPB and SFTPC, were correlated with cell cycle regulation, T cell recruitment, anti-PD-1 therapy, and anti-inflammatory processes. This study identified some candidate molecules and pathways associated with the prognosis of LUAD, providing potential therapeutic targets for the comprehensive treatment of this subtype of lung cancer.
The high expression of the DEGs of E2FB, CENPU, NDC80, and TYMS in subtype 1 was related to higher mortality and more advanced tumor stage, consistent with the literature (22,23). For example, CENPU downregulation significantly inhibited lung adenocarcinoma cell (LAC) proliferation, migration, and invasion, mediated by phosphatidylinositide 3-kinases (PI3K)/protein kinase B pathway inactivation (23). Further, TYMS downregulation has been found to be associated with sensitivity to pemetrexed in lung cancer cell lines (24). Thus, genes can also be used to predict responses to therapeutic drugs.
The tumor microenvironment plays an important role in supporting tumor growth. A number of studies have investigated the effect of DNA methylation on the behavior of tumor-associated stromal cells. DNA methylation is involved in the activation of stromal cells, and the methylation of certain genes contributes to the precancerous activity of stromal cells (25). The genes corresponding to the methylation sites included BCAT1, CDC42, DLX5, HOXA5, and OTX1. The alteration of methylation levels is reportedly involved in the development and progression of lung cancer (26,27). BCAT1 has been shown to induce proliferation and invasion in lung cancer (28), while CDC42 has been shown to be involved in cell transformation, proliferation, survival, invasion, and metastasis in multiple cancer types (29). Further, it has been shown that DLX5 promotes cell proliferation by upregulating MYC (30) and upregulated OTX1 can enhance the proliferation, invasion, and migration of lung cancer cells by activating the JAK/STAT signaling pathway (31).
In the present study, HLA-DQA1, HLA-DPA1, and HLA-DPB1 were the hub genes in subnetwork 2. As previously reported, the DR and DQ isotypes of the human leukocyte antigen (HLA) class II heterodimeric molecules (32) are strongly associated with autoimmune diseases. Further, the overall survival time of patients with LUAD has been shown to be affected by HLA-DR (33).
The upregulated genes of SPTPB and SFTPD in subnetwork 3 have been reported to be immune- and inflammation-related genes (34,35). Notably, the signaling pathways related to the hub genes mainly include human T-cell leukemia virus (type 1) infection, the intestinal immune network for IgA production, and inflammatory bowel disease, which are also related to the immune system. Consistent with previous studies, our findings suggest that the prognosis of LUAD is closely related to immunity, which is the basis of immunotherapy (22,36). For example, the mechanism of the immunosuppressive effect of anti-PD-1 involves the inhibition of cell signal transduction and activity by mediated PD-1 in immune cells. This revolutionary breakthrough has played an important part in improving LUAD treatment efficacy (22,36).
A major limitation of this study is the lack of prognostic data in the validation group, which could not be solved, as the data was downloaded from a public database. Subtype 1 in the training data set had a relatively worse prognosis as compared with subtype 2. No prognostic information was available; however, it can be concluded that a poor prognosis could be associated with subtype 2 in the validation data set, as both subtypes exhibited similar expression patterns and were associated with a more advanced tumor stage. Further, other critical parameters such as non-coding ribonucleic acid (RNA) data and clinical characteristics were not considered when the molecular typing was conducted in our study, such as micro-RNA.
Conclusions
In summary, using the NMF method and the multi-omics information of gene expression and DNA methylation sites, we successfully divided LUAD into 2 clinically relevant subtypes. The representative genes and the corresponding genes of the methylation sites were identified. The functional analysis showed significant enrichment in GO terms “cell cycle” and “immune”, which were significantly correlated with the development of LUAD. Our research highlights the prognostic role of DNA methylation sites and extends understandings of the mechanisms underlying LUAD tumorigenesis.
Acknowledgments
We would like to thank the National Natural Science Foundation of China for supporting this study.
Funding: This work was supported by the National Natural Science Foundation of China (Nos. 81970167 and 81800108).
Footnote
Reporting Checklist: The authors have completed the STREGA reporting checklist. Available at https://atm.amegroups.com/article/view/10.21037/atm-22-3340/rc
Conflicts of Interest: All authors have completed the ICMJE uniform disclosure form (available at https://atm.amegroups.com/article/view/10.21037/atm-22-3340/coif). The authors have no conflicts of interest to declare.
Ethical Statement: The authors are accountable for all aspects of the work in ensuring that questions related to the accuracy or integrity of any part of the work are appropriately investigated and resolved. The study was conducted in accordance with the Declaration of Helsinki (as revised in 2013).
Open Access Statement: This is an Open Access article distributed in accordance with the Creative Commons Attribution-NonCommercial-NoDerivs 4.0 International License (CC BY-NC-ND 4.0), which permits the non-commercial replication and distribution of the article with the strict proviso that no changes or edits are made and the original work is properly cited (including links to both the formal publication through the relevant DOI and the license). See: https://creativecommons.org/licenses/by-nc-nd/4.0/.
References
- Inamura K. Lung Cancer: Understanding Its Molecular Pathology and the 2015 WHO Classification. Front Oncol 2017;7:193. [Crossref] [PubMed]
- Coffer PJ, Burgering BM. Forkhead-box transcription factors and their role in the immune system. Nat Rev Immunol 2004;4:889-99. [Crossref] [PubMed]
- Siegel RL, Miller KD, Jemal A. Cancer statistics, 2020. CA Cancer J Clin 2020;70:7-30. [Crossref] [PubMed]
- Doll KM, Rademaker A, Sosa JA. Practical Guide to Surgical Data Sets: Surveillance, Epidemiology, and End Results (SEER) Database. JAMA Surg 2018;153:588-9. [Crossref] [PubMed]
- Maruyama R, Choudhury S, Kowalczyk A, et al. Epigenetic regulation of cell type-specific expression patterns in the human mammary epithelium. PLoS Genet 2011;7:e1001369. [Crossref] [PubMed]
- Moore LD, Le T, Fan G. DNA methylation and its basic function. Neuropsychopharmacology 2013;38:23-38. [Crossref] [PubMed]
- Li XS, Nie KC, Zheng ZH, et al. Molecular subtypes based on DNA methylation predict prognosis in lung squamous cell carcinoma. BMC Cancer 2021;21:96. [Crossref] [PubMed]
- Shen N, Du J, Zhou H, et al. A Diagnostic Panel of DNA Methylation Biomarkers for Lung Adenocarcinoma. Front Oncol 2019;9:1281. [Crossref] [PubMed]
- Li C, Long Q, Zhang D, et al. Identification of a four-gene panel predicting overall survival for lung adenocarcinoma. BMC Cancer 2020;20:1198. [Crossref] [PubMed]
- Rhee YY, Lee TH, Song YS, et al. Prognostic significance of promoter CpG island hypermethylation and repetitive DNA hypomethylation in stage I lung adenocarcinoma. Virchows Arch 2015;466:675-83. [Crossref] [PubMed]
- Cai W, Jing M, Wen J, et al. Epigenetic Alterations of DNA Methylation and miRNA Contribution to Lung Adenocarcinoma. Front Genet 2022;13:817552. [Crossref] [PubMed]
- Selamat SA, Chung BS, Girard L, et al. Genome-scale analysis of DNA methylation in lung adenocarcinoma and integration with mRNA expression. Genome Res 2012;22:1197-211. [Crossref] [PubMed]
- Yang K, Wu Y. A prognosis-related molecular subtype for early-stage non-small lung cell carcinoma by multi-omics integration analysis. BMC Cancer 2021;21:128. [Crossref] [PubMed]
- Zhang H, Jin Z, Cheng L, et al. Integrative Analysis of Methylation and Gene Expression in Lung Adenocarcinoma and Squamous Cell Lung Carcinoma. Front Bioeng Biotechnol 2020;8:3. [Crossref] [PubMed]
- Xu T, Le TD, Liu L, et al. CancerSubtypes: an R/Bioconductor package for molecular cancer subtype identification, validation and visualization. Bioinformatics 2017;33:3131-3. [Crossref] [PubMed]
- Tanabe M, Kanehisa M. Using the KEGG database resource. Curr Protoc Bioinformatics 2012;Chapter 1:Unit1.12.
- Szklarczyk D, Morris JH, Cook H, et al. The STRING database in 2017: quality-controlled protein-protein association networks, made broadly accessible. Nucleic Acids Res 2017;45:D362-8. [Crossref] [PubMed]
- Shannon P, Markiel A, Ozier O, et al. Cytoscape: a software environment for integrated models of biomolecular interaction networks. Genome Res 2003;13:2498-504. [Crossref] [PubMed]
- Kwon S, Kim H, Kim HS. Identification of Pharmacologically Tractable Protein Complexes in Cancer Using the R-Based Network Clustering and Visualization Program MCODER. Biomed Res Int 2017;2017:1016305. [Crossref] [PubMed]
- Shi YX, Yin JY, Shen Y, et al. Genome-scale analysis identifies NEK2, DLGAP5 and ECT2 as promising diagnostic and prognostic biomarkers in human lung cancer. Sci Rep 2017;7:8072. [Crossref] [PubMed]
- Zhang H, Liu Y, Tang S, et al. Knockdown of DLGAP5 suppresses cell proliferation, induces G2/M phase arrest and apoptosis in ovarian cancer. Exp Ther Med 2021;22:1245. [Crossref] [PubMed]
- Li JX, Huang JM, Jiang ZB, et al. Current Clinical Progress of PD-1/PD-L1 Immunotherapy and Potential Combination Treatment in Non-Small Cell Lung Cancer. Integr Cancer Ther 2019;18:1534735419890020. [Crossref] [PubMed]
- Li J, Wang ZG, Pang LB, et al. Reduced CENPU expression inhibits lung adenocarcinoma cell proliferation and migration through PI3K/AKT signaling. Biosci Biotechnol Biochem 2019;83:1077-84. [Crossref] [PubMed]
- Wu MF, Hsiao YM, Huang CF, et al. Genetic determinants of pemetrexed responsiveness and nonresponsiveness in non-small cell lung cancer cells. J Thorac Oncol 2010;5:1143-51. [Crossref] [PubMed]
- Zhang MW, Fujiwara K, Che X, et al. DNA methylation in the tumor microenvironment. J Zhejiang Univ Sci B 2017;18:365-72. [Crossref] [PubMed]
- Diaz-Lagares A, Mendez-Gonzalez J, Hervas D, et al. A Novel Epigenetic Signature for Early Diagnosis in Lung Cancer. Clin Cancer Res 2016;22:3361-71. [Crossref] [PubMed]
- Zhu X, Li Y, Shen H, et al. miR-137 inhibits the proliferation of lung cancer cells by targeting Cdc42 and Cdk6. FEBS Lett 2013;587:73-81. [Crossref] [PubMed]
- Lin X, Tan S, Fu L, et al. BCAT1 Overexpression Promotes Proliferation, Invasion, and Wnt Signaling in Non-Small Cell Lung Cancers. Onco Targets Ther 2020;13:3583-94. [Crossref] [PubMed]
- Chen QY, Jiao DM, Yao QH, et al. Expression analysis of Cdc42 in lung cancer and modulation of its expression by curcumin in lung cancer cell lines. Int J Oncol 2012;40:1561-8. [Crossref] [PubMed]
- Xu J, Testa JR. DLX5 (distal-less homeobox 5) promotes tumor cell proliferation by transcriptionally regulating MYC. J Biol Chem 2009;284:20593-601. [Crossref] [PubMed]
- Lu Y. miR-223-5p Suppresses OTX1 to Mediate Malignant Progression of Lung Squamous Cell Carcinoma Cells. Comput Math Methods Med 2021;2021:6248793. [Crossref] [PubMed]
- Farina F, Picascia S, Pisapia L, et al. HLA-DQA1 and HLA-DQB1 Alleles, Conferring Susceptibility to Celiac Disease and Type 1 Diabetes, are More Expressed Than Non-Predisposing Alleles and are Coordinately Regulated. Cells 2019;8:751. [Crossref] [PubMed]
- Riemann D, Cwikowski M, Turzer S, et al. Blood immune cell biomarkers in lung cancer. Clin Exp Immunol 2019;195:179-89. [Crossref] [PubMed]
- Wei X, Wang C, Feng H, et al. Effects of ALOX5, IL6R and SFTPD gene polymorphisms on the risk of lung cancer: A case-control study in China. Int Immunopharmacol 2020;79:106155. [Crossref] [PubMed]
- Lin SL, Le TX, Cowen DS. SptP, a Salmonella typhimurium type III-secreted protein, inhibits the mitogen-activated protein kinase pathway by inhibiting Raf activation. Cell Microbiol 2003;5:267-75. [Crossref] [PubMed]
- Abu Hejleh T, Furqan M, Ballas Z, et al. The clinical significance of soluble PD-1 and PD-L1 in lung cancer. Crit Rev Oncol Hematol 2019;143:148-52. [Crossref] [PubMed]