Using search trends before and after the COVID-19 outbreak in China to analyze digestive symptoms: medical informatics study of the Baidu Index data
Introduction
From the beginning of 2020, the world was plunged into a global pandemic due to the novel coronavirus disease-19 (COVID-19). By August 2021, the cumulative number of confirmed cases in the world exceeded 200 million, resulting in 4.3 million deaths (1). China, the world’s most populous country, had 121,845 confirmed cases and 5,662 deaths (2). When the fear of the disease gripped the world, human activities were limited and living habits were forced to change. People tended to stay at home to avoid the risk of catching the virus (3-6). In such a situation, people were more inclined to search for information related to COVID-19 on the internet, including the latest news about COVID-19, how the outbreak is progressing, and medical information including likely symptoms.
Based on the analysis of the confirmed cases, fever, cough, fatigue, and dyspnea were identified as predominant symptoms of COVID-19 (7,8). Thus, these also became important keywords when searching for information related to COVID-19. Early studies reported that gastrointestinal symptoms, such as nausea, vomit, abdominal pain, and diarrhea, were also a primary manifestation in 3–37% of patients, and indeed, these symptoms may even precede clinical diagnosis (7,9,10). During the COVID-19 epidemic, clinicians mainly use traditional methods to determine the prevalence of a particular symptom from confirmed cases (11). However, due to the limitations of disease-related testing and the high incidence of subclinical and minimally symptomatic diseases, the effectiveness of traditional methods of investigation is often less than ideal. Internet-based methods with wide coverage and large audiences can improve the efficiency of a symptom survey in the general population.
Information epidemiology is an innovative discipline. By analyzing information from the Internet, we can gain insights into changes in population health that could ultimately inform public health and policy, especially during disease outbreaks and epidemics. This research method has been widely used in previous medical ethics research. Matsuyama et al. reviewed global cases of Middle East respiratory syndrome (MERS) up to July 2015, by measuring the following three indicators: Google Trends, ProMED-mail, and Disease Outbreak News (12). Farhadloo et al. examined topics from a nationally representative survey of American adults by analyzing the content of online Twitter exchanges (13). van Lent et al. also used the same method to analyze the epidemiological data and media data of the 2014 Ebola outbreak (14). Other studies have reportedly helped to predict symptom-based flu transmission and onset patterns by analyzing Internet search queries that reflect user activity in seeking health information (15-17). These previous studies have shown that analyzing variation in Internet searches for a disease keyword can help further research.
Baidu is the most used search engine in China (18), and the Baidu Search Index is calculated to help medical informatics research across China. This current informational epidemiological study investigated the China-wide trends in Baidu search queries for gastrointestinal symptoms during COVID-19.
Methods
Data sources
The Baidu Index is a publicly available data sharing platform based on the behavioral data of the vast number of netizens on Baidu. It provides information related to the search volume of a particular keyword and its fluctuations caused by news and public opinion over a period of time (19). The main data provided is the search index (SI), which represents the interest given to certain keywords by website users. The SI takes keyword as the statistical object and is based on the search volume of netizens in Baidu. It is obtained by scientific analysis and calculation of the weighted search frequency of each keyword in the Baidu web search. According to the data source, the SI is divided into personal computer (PC) search index and mobile search index (20).
In Chinese, several words can express the same meaning. We set up 7 topics, each topic contains multiple search keywords, covering both academic and colloquial expressions to maximize the integrity of the search (Table 1). These topics include 2 recognized respiratory symptoms caused by COVID-19 infection, namely, “fever” and “cough” (21,22), and 5 gastrointestinal symptoms, namely, “nausea”, “vomit”, “abdominal pain”, “diarrhea”, and “constipation” (23-25). The SI variation of the 7 topics was analyzed over a 6-month period before and after the outbreak of COVID-19 in China to reflect the variation of people’s search volume (available online: https://cdn.amegroups.cn/static/public/atm-22-3465-1.docx). The greater the volume of searches, the more likely it is to be a gastrointestinal symptom of COVID-19 infection (26,27).
Table 1
Topics in English | Keywords in Chinese |
---|---|
Nausea | E xin |
Vomit | Ou tu, tu |
Abdominal pain | Fu tong; du zi teng; du zi tong |
Diarrhea | Fu xie; la du zi; la xi |
Fever | Fa re; fa shao |
Cough | Ke sou; gan ke |
Constipation | Bian mi |
Real-time data on COVID-19 infections and the cumulative number of confirmed cases were obtained through Chinese government-certified news websites (28) (available online: https://cdn.amegroups.cn/static/public/atm-22-3465-2.docx). A total of 10 provinces in China were selected for separate analyses, including Beijing, Gansu, Guangdong, Guangxi, Heilongjiang, Hubei, Sichuan, Shanghai, Xinjiang, and Tibet. These regions represent different geographical locations, economic strength, and different populations. The economic data and demographic data of each province are available from China’s official statistics website (29).
Data analysis
“COVID-19” was used as a search keyword in China on January 9, 2020 (marked as date a) for the first time (30). Using this date as a partition, the period from June 1, 2019 to June 1, 2020 was divided into two parts. The SI of the first half (June 1, 2019 to January 8, 2020) and the SI of the second half (January 9, 2020 to June 1, 2020) were calculated as pre-SI and post-SI, respectively. The latter half of the period was further divided into three sections by two dates (January 25, 2020 and April 8, 2020 marked as date b and c, respectively), which were defined as periods I, II, and III, successively. The average daily increase (ADI) during period I and the average daily decrease (ADD) during period II were calculated using the following formulas:
Comparisons and correlation analyses were performed to determine if the SI of gastrointestinal symptoms differed among provinces. The SI growth rate of each province was calculated using the following formula:
Statistical analysis
The SPSS software version 26.0 (SPSS Company, Chicago, IL, USA) was used for all statistical analyses. A general linear model was constructed to compare the ADI and ADD of the SI for different symptoms. The independent sample t test was used to compare the SI over different time periods, and the Welch correction was used when the variance was uneven. Correlation tests were used to identify indicators related to SI in each province. For all statistical analyses, a P value less than 0.05 was considered statistically significant. The GraphPad Prism version 9 software (GraphPad Prism Software Inc.) was used for figure production and rendering.
Results
The variation trend of search index across China
As of June 1, 2020, China had a total of 84,597 confirmed cases of COVID-19 (2). “COVID-19” was first used as a search keyword in China on January 9, 2020. Prior to that, the SI of all the symptoms included remained stable. After January 9, 2020, the SI of the 2 major respiratory symptoms of COVID-19, fever and cough, increased rapidly and peaked at about 2 weeks later (around January 25, 2020). The peak SI of “cough” was higher than that of “fever”. Over time, the SI of both symptoms returned to their pre-outbreak plateau, at around April 8, 2020. The SI of the gastrointestinal symptom “diarrhea” showed a similar variation during the period. Among the other gastrointestinal symptoms included, there was a brief increase in the SI of “vomit” around February 10, 2020. The SI of the other three symptoms, “nausea”, “abdominal pain”, and “constipation” did not change significantly during the outbreak (Figure 1).
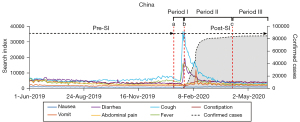
During period I (from January 9 to January 25, 2020), “fever”, “cough”, and “diarrhea” showed a noteworthy increased trend. A general linear model was constructed to compare the increased rate of SI for “diarrhea” with that of the other two respiratory symptoms. The ADI of the SI for “diarrhea” was lower than that for “cough”, with statistical significance (889.47 vs. 1799.12, F=11.43, P=0.002), while no statistical difference was found between the ADI of the SI for “diarrhea” and “fever” (889.47 vs. 719.18, F=0.85, P=0.37). In period II (from January 25 to April 8, 2020), “fever”, “cough”, and “diarrhea” showed a noteworthy downward trend. The ADD of the SI for “diarrhea” was lower than that for “cough”, with statistical significance, but higher than that for “fever”, with no statistical significance (cough, 191.40 vs. 441.44, F=68.66, P<0.001; fever, 191.40 vs. 188.61, F=3.71, P=0.06). In period III, the mean post-SI was significantly lower than the mean pre-SI with statistical significance (fever, 2,616.41±116.92 vs. 3,724.51±867.81, P<0. 001; cough, 3,260.04±308.43 vs. 5,590.66±874.25, P<0.001; diarrhea, 4,128.80±200.82 vs. 4,423.55±1,058.01, P<0.001) (Table 2).
Table 2
Time points & time range | Projects | Diarrhea | Cough | Fever |
---|---|---|---|---|
a | SI | 3,399 | 6,313 | 4,647 |
b | SI | 18,520 | 36,898 | 16,873 |
c | SI | 4,165 | 3,790 | 2,727 |
Period I | Number of days | 17 | ||
ADI (%) | 889.47 | 1,799.12 | 719.18 | |
P valuea | – | 0.002 | 0.37 | |
Period II | Number of days | 75 | ||
ADD (%) | 191.40 | 441.44 | 188.61 | |
P valueb | – | <0.001 | 0.06 | |
Period III | Post-SI (mean ± SE) | 4,128.80±200.82 | 3,260.04±308.43 | 2,616.41±116.92 |
Pre-SI (mean ± SE) | 4,423.55±1,058.01 | 5,590.66±874.25 | 3,724.51±867.81 | |
P valuec | <0.001 | <0.001 | <0.001 |
a, January 9, 2020; b, January 25, 2020; c, April 8, 2020; period I, from January 9, 2020 to January 25, 2020; period II, from January 25, 2020 to April 8, 2020; period III, from April 8, 2020 to June 1, 2020; post-SI, the search index from January 9, 2020 to June 1, 2020; P valuea, P values obtained by comparing ADI of respiratory symptoms and “diarrhea”; P valueb, P values obtained by comparing ADD of respiratory symptoms and “diarrhea”; P valuec, P values obtained by comparing pre-SI and post-SI of different symptoms; SE, standard error; –, meaningless. ADD, average daily increase; ADI, average daily decrease.
The variation trend of the search index in different provinces
The SI trend of the 10 selected provinces is shown in Figure 2. The SI changes of “fever”, “cough”, and “diarrhea” were more prominent than that of the other symptoms in all provinces, with the exception of Tibet and Xinjiang. The SI of all terms increased rapidly after the keyword “COVID-19” appeared for the first time on January 9, 2020, and reached a peak at around January 25, 2020, and then declined to a stable level around April 8, 2020. This was consistent with the overall phenomenon of COVID-19 in China. In all provinces examined, the peak value of SI for “cough” was higher than those for “diarrhea” and “fever”, while the latter two were comparable. For Xinjiang and Tibet, the SI of all studied symptoms fluctuated greatly over time. The SI of “cough” and “fever”, recognized as major symptoms of COVID-19, showed an upward trend after 9 January 2020, while that of “diarrhea” and other digestive symptoms did not.
The correlation analysis of “diarrhea” search index variation in different provinces
The search term “diarrhea” was further analyzed. The pre-SI and post-SI of “diarrhea” was obtained for each province, the mean value (pre-SI mean) and the peak value (post-SI peak) were calculated, and the growth rate was obtained using the formula. The demographic and economic data, and the real-time outbreak data such as population, gross domestic product (GDP), per capita disposable income (PCDI), previously confirmed cases on the day of SI peak (PCC), were obtained from the official website of China for all provinces (29) (Table 3). The provinces were then ranked for each indicator and color-coded on the map. Rank 1 to 10 are indicated by color from dark to light (Figure 3). Correlation analysis was conducted between SI data (pre-SI mean, post-SI peak, and SI growth rate) and demographic, economic, and real-time outbreak data. The percentage of demographic, economic and real-time outbreak data in each province was calculated (Figure 4). The pre-SI mean was significantly correlated with population (P=0.004, R=0.813) and GDP (P<0.001, R=0.966), with statistical significance, but not with PCDI (P=0.12, R=0.519) nor PCC (P=0.53, R=0.229). The post-SI peak was significantly correlated with population (P=0.007, R=0.789), GDP (P=0.005, R=0.804), and PCC (P=0.03, R=0.670), with statistical significance, but not with PCDI (P=0.59, R=0.193). The growth rate of SI was correlated with post-SI peak (P=0.04, R=0.649) and PCC (P=0.003, R=0.835), but not with the pre-SI mean (P=0.79, R=0.099), population (P=0.44, R=0.277), GDP (P=0.72, R=0.129), nor PCDI (P=0.38, R=−0.311).
Table 3
Names of provinces | Pre-SI mean (%a) |
Post-SI peak (%a) |
SI growth rate (%) | Population (million) (%a) |
GDP (trillion yuan) (%a) |
PCDI (million yuan) (%a) |
PCC (%a) |
---|---|---|---|---|---|---|---|
Beijing | 662.93 (13.11) | 1,429 (11.59) | 115.56 | 21.89 (4.86) | 3.61 (10.68) | 694.34 (19.70) | 68 (4.58) |
Gansu | 256.05 (5.06) | 549 (4.45) | 114.41 | 25.02 (5.55) | 0.9 (2.66) | 203.35 (5.77) | 14 (0.94) |
Guangdong | 1,120.94 (22.16) | 2,365 (19.18) | 110.99 | 126.01 (27.96) | 11.07 (32.76) | 410.29 (11.64) | 146 (9.84) |
Guangxi | 420.09 (8.31) | 1,056 (8.56) | 151.37 | 50.13 (11.12) | 2.21 (6.54) | 245.62 (6.97) | 46 (3.10) |
Heilongjiang | 348.21 (6.88) | 722 (5.86) | 107.35 | 31.85 (7.07) | 1.36 (4.02) | 249.02 (7.06) | 21 (1.42) |
Hubei | 588.80 (11.64) | 2,597 (21.06) | 341.06 | 57.75 (12.81) | 4.34 (12.84) | 278.81 (7.91) | 1,052 (70.89) |
Shanghai | 642.43 (12.70) | 993 (8.05) | 54.57 | 24.87 (5.52) | 3.87 (11.45) | 722.32 (20.49) | 80 (5.39) |
Sichuan | 636.65 (12.59) | 1,925 (15.61) | 202.36 | 83.67 (18.56) | 4.86 (14.38) | 265.22 (7.52) | 44 (2.96) |
Tibet | 100.86 (1.99) | 244 (1.98) | 141.91 | 3.65 (0.81) | 0.19 (0.56) | 217.44 (6.17) | 0 (0.00) |
Xinjiang | 281.35 (5.56) | 450 (3.65) | 49.94 | 25.85 (5.74) | 1.38 (4.08) | 238.45 (6.76) | 13 (0.88) |
Total | 5,058.32 (100.00) | 12,330 (100.00) | – | 450.69 (100.00) | 33.79 (100.00) | 3,524.86 (100.00) | 1,484 (100.00) |
GDP, gross domestic product; pre-SI mean, the mean value of search index from June 1, 2019 to January 8, 2020; post-SI peak, the peak value of search index from January 9, 2020 to June 1, 2020; PCDI, per capita disposable income; PCC, previously confirmed cases on the day of SI peak; (%a), the percentage of each province’s value in the total of all included provinces; –, meaningless.
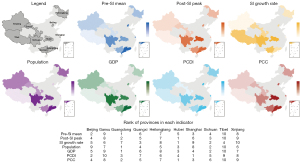
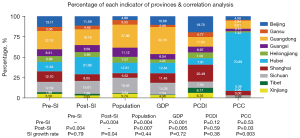
Discussion
In this modern era, people prefer using the Internet to obtain information because of its convenience. During the COVID-19 pandemic, the Internet played an even more important role in people’s access to information due to the limited scope of movement and activities. Baidu is the most widely used search engine in China, with 558 million monthly active users on its mobile app alone, as of August 2021 (18). The Baidu Index is a data analysis platform based on the behavioral data of Baidu’s massive netizens. It is one of the most important statistical analysis platforms in the era of the Internet and even the whole data era (19). The SI is the main data type provided by the Baidu Index website, which is obtained by scientific analysis and calculation of the weighted search frequency of each keyword in the Baidu web search. The SI reflects the search volume of a certain keyword in a certain period of time and is positively correlated with it (20). In this current study, the SI of the Baidu website was analyzed for keywords related to COVID-19 symptoms.
Chronologically, attention to symptoms of an epidemic begins before the news media officially reports on it (31). The first confirmed case is usually not the very first case. A doctor’s judgment of disease symptoms is mostly based on the collection of confirmed cases. A review of big data on the Internet can help to trace the earliest emergence of an epidemic. Looking back at variations in Internet search volume for a symptom which people were concerned about can help to study the earliest onset of the disease and its clinical manifestations (31-34).
During the COVID-19 pandemic, for many non-medical people, searching the Internet for information related to COVID-19 symptoms was a combination of interest and curiosity about the situation, as well as concern about whether they were infected if they presented with similar symptoms. People’s search volume of a certain symptom keyword directly reflects the population’s attention to this symptom. Therefore, according to the observation of the SI, the symptoms corresponding to keywords with obvious changes in search volume during the epidemic were most likely to be the main clinical manifestations of COVID-19. The increase the SI of these symptom-keywords went earlier with the very beginning of epidemic than the official media reports.
Cough and fever were recognized as the primary respiratory symptoms of COVID-19. Figure 1 shows that people’s attention to these two respiratory symptoms increased significantly. After the media first reported “COVID-19” on January 9, 2020, people searched for “cough” and “fever” on the Internet out of concern. They hoped to determine whether they were likely to be infected with COVID-19 through the information they obtained from the Internet when they experienced similar symptoms. This upward trend was only reflected in “diarrhea” among the 5 digestive symptoms we analyzed. We speculated that diarrhea was one of the main clinical manifestations of COVID-19 infection in the digestive system. Meanwhile, the upward trend of the SI for “cough”, “fever”, and “diarrhea” were already shown before the media reported “COVID-19”, suggesting that the epidemic of COVID-19 in China began earlier than January 9, 2020. As shown in Figure 1, the SI for “cough”, “fever”, and “diarrhea” all reached peak values around January 25, 2020, and returned to stable around April 8, 2020. These correspond to the time points of the Wuhan blockade and deblocking, respectively (35,36), which reflected the progress of the epidemic in China. People’s attention on Wuhan, the worst-hit city in China (29), may have impacted the search volume of symptoms.
During period I (from January 9, 2020 to January 25, 2020), the SI of “diarrhea” increased rapidly, and decreased slowly in period II (from January 25, 2020 to April 8, 2020), with rangeability significantly lower than that of the SI for “cough” (lower ADI and ADD). However, there was no significant difference between “diarrhea” and “fever”, indicating that people paid less attention to “diarrhea” than to “cough”. The results suggested that diarrhea may be the main symptom of COVID-19 in the digestive system, but its incidence is not as common as cough. This result is consistent with the view of Hajifathalian et al. who proposed that diarrhea may be the main gastrointestinal manifestation of COVID-19 in the United States, with a lower incidence compared to fever (37). This discrepancy may be due to the diverse expressions available for a keyword in Chinese, while English may only have a singular expression (Table 1). The SI of “cough”, “fever”, and “diarrhea” all leveled off after April 8, 2020, with lower values than those before the outbreak. The drop in attention to symptoms may be due to the presence of other COVID-19 news, such as “vaccines” and “international news” (38-40).
Ten representative provinces (Beijing, Gansu, Guangdong, Guangxi, Heilongjiang, Hubei, Sichuan, Shanghai, Xinjiang, and Tibet) in China were selected based on different geographical location, economic strength, and population. The curves of the SI variation were plotted for each province (Figure 2). Except for Tibet and Xinjiang, the SI absolute value of all the other eight provinces shown great differences, while the variation tendencies of the curves were consistent with that of the whole country. The SI of “cough”, “fever”, and “diarrhea” in Xinjiang peaked around January 25, 2020, but “fever” was the highest. The SI curves of all symptoms in Tibet fluctuated greatly during the study period. This may result from the poor internet usage in Xinjiang and Tibet. There are also language barriers and different local customs in Tibet, which may comprehensively affect the variation tendency of the SI.
As for “diarrhea” alone, we combined the economic indicators, population, and epidemic situation of the included provinces to draw Figure 3, ranking provinces from 1 to 10, and color-coded them from dark to light. In this way, the differences between all provinces are observed. The percentage of demographic, economic and real-time outbreak data in each province were calculated. The correlation between the indicators was analyzed (Figure 4). Before the outbreak, Guangdong, Beijing, and Shanghai had the highest SI for “diarrhea”, which was related to their large population base and high level of economic development. People there more frequently use the Internet and tend to be more skilled in using the Internet. Guangdong, Beijing, and Shanghai were still at the top of the SI after the outbreak, while Hubei jumped from fifth place to first place because its capital city Wuhan was the worst-hit city and people were far more concerned about COVID-19 than in other provinces. It is worth noting that in the calculation of the SI growth rate, Sichuan and Guangxi are in second and third place, respectively, while the more developed Beijing, Shanghai, and Guangdong ranked lower. Hubei undoubtedly was in the first place for SI growth rate. This likely reflected the far-reaching impact of the epidemic, which aroused widespread attention in China. Second, there may have been saturation in the growth of the SI. The SI growth rate in Beijing, Shanghai, and Guangdong were lower than that in other provinces, as people in these provinces already paid great attention to the epidemic. Xinjiang and Tibet were at the bottom of nearly all indicators, which may be closely related to language barriers and inconvenient transportation. Correlation analysis revealed that the variation of pre-SI was significantly associated with population and economic development, which was determined by the calculation principle of the SI (20). The more people searching, the more search volume will be generated, and the higher the SI will be.
There were some limitations to this study. First, the English expression of a symptom may correspond to multiple Chinese expressions, which may affect the results of the SI statistics. Second, Baidu is only widely used in China. More advanced search engines are needed to conduct a worldwide analysis. Third, the included provinces were representations of different geographical location, economic strength, and population. All provinces need to be included in future in-depth research. Fourth, diarrhea itself is common in daily life. The results of our study on diarrhea during the COVID-19 epidemic warrant further verification. In addition, the impact of seasonal changes on epidemic diseases should be considered in the study, which is included in this paper and needs to be improved in further studies.
As mentioned above, diarrhea was of widespread concern in all provinces before and after the COVID-19 outbreak and may be associated with novel coronavirus infection. The variation of people’s attention to diarrhea was associated with the real-time outbreak of the province. Changes in the SI when diarrhea is used as a search keyword can help doctors assess the outbreak, progress, and control of COVID-19, and provide guidance for predicting the trend of the epidemic. Internet big data can reflect the public's concern about diseases, which is of great significance for the study of the epidemiological characteristics of diseases.
Acknowledgments
Funding: None.
Footnote
Conflicts of Interest: All authors have completed the ICMJE uniform disclosure form (available at https://atm.amegroups.com/article/view/10.21037/atm-22-3465/coif). The authors have no conflicts of interest to declare.
Ethical Statement: The authors are accountable for all aspects of the work in ensuring that questions related to the accuracy or integrity of any part of the work are appropriately investigated and resolved.
Open Access Statement: This is an Open Access article distributed in accordance with the Creative Commons Attribution-NonCommercial-NoDerivs 4.0 International License (CC BY-NC-ND 4.0), which permits the non-commercial replication and distribution of the article with the strict proviso that no changes or edits are made and the original work is properly cited (including links to both the formal publication through the relevant DOI and the license). See: https://creativecommons.org/licenses/by-nc-nd/4.0/.
References
- World Health Organization. Coronavirus disease (COVID-19) Situation Report. Available online: https://www.who.int/publications/m/item/weekly-epidemiological-update-on-covid-19---3-august-2021 [accessed 2021-08-10].
- Sogou News. Live Coronavirus disease (COVID-19) Outbreak. Available online: https://sa.sogou.com/new-weball/page/sgs/epidemic?type_page=VR [accessed 2021-08-10].
- Cancello R, Soranna D, Zambra G, et al. Determinants of the Lifestyle Changes during COVID-19 Pandemic in the Residents of Northern Italy. Int J Environ Res Public Health 2020;17:6287. [Crossref] [PubMed]
- Chaturvedi K, Vishwakarma DK, Singh N. COVID-19 and its impact on education, social life and mental health of students: A survey. Child Youth Serv Rev 2021;121:105866. [Crossref] [PubMed]
- Elmaslar Özbaş E, Akın Ö, Güneysu S, et al. Changes occurring in consumption habits of people during COVID-19 pandemic and the water footprint. Environ Dev Sustain 2022;24:8504-20. [Crossref] [PubMed]
- Zhu Y, Wang Z, Maruyama H, et al. Effect of the COVID-19 lockdown period on the physical condition, living habits, and physical activity of citizens in Beijing, China. J Phys Ther Sci 2021;33:632-6. [Crossref] [PubMed]
- Huang C, Wang Y, Li X, et al. Clinical features of patients infected with 2019 novel coronavirus in Wuhan, China. Lancet 2020;395:497-506. Erratum in: Lancet 2020;395:496. [Crossref] [PubMed]
- Gu J, Han B, Wang J. COVID-19: Gastrointestinal Manifestations and Potential Fecal-Oral Transmission. Gastroenterology 2020;158:1518-9. [Crossref] [PubMed]
- Wang D, Hu B, Hu C, et al. Clinical Characteristics of 138 Hospitalized Patients With 2019 Novel Coronavirus-Infected Pneumonia in Wuhan, China. JAMA 2020;323:1061-9. [Crossref] [PubMed]
- Luo S, Zhang X, Xu H. Don't Overlook Digestive Symptoms in Patients With 2019 Novel Coronavirus Disease (COVID-19). Clin Gastroenterol Hepatol 2020;18:1636-7. [Crossref] [PubMed]
- Abat C, Chaudet H, Rolain JM, et al. Traditional and syndromic surveillance of infectious diseases and pathogens. Int J Infect Dis 2016;48:22-8. [Crossref] [PubMed]
- Matsuyama R, Nishiura H, Kutsuna S, et al. Clinical determinants of the severity of Middle East respiratory syndrome (MERS): a systematic review and meta-analysis. BMC Public Health 2016;16:1203. [Crossref] [PubMed]
- Farhadloo M, Winneg K, Chan MS, et al. Associations of Topics of Discussion on Twitter With Survey Measures of Attitudes, Knowledge, and Behaviors Related to Zika: Probabilistic Study in the United States. JMIR Public Health Surveill 2018;4:e16. [Crossref] [PubMed]
- van Lent LG, Sungur H, Kunneman FA, et al. Too Far to Care? Measuring Public Attention and Fear for Ebola Using Twitter. J Med Internet Res 2017;19:e193. [Crossref] [PubMed]
- Desai R, Hall AJ, Lopman BA, et al. Norovirus disease surveillance using Google Internet query share data. Clin Infect Dis 2012;55:e75-8. [Crossref] [PubMed]
- Ginsberg J, Mohebbi MH, Patel RS, et al. Detecting influenza epidemics using search engine query data. Nature 2009;457:1012-4. [Crossref] [PubMed]
- Eysenbach G. Infodemiology: tracking flu-related searches on the web for syndromic surveillance. AMIA Annu Symp Proc 2006;2006:244-8. [PubMed]
- Baidu. Baidu Search Engine. Available online: https://www.baidu.com/ [accessed 2021-08-15].
- Baidu Encyclopedia. Baidu Index. Available online: https://baike.baidu.com/item/%E7%99%BE%E5%BA%A6%E6%8C%87%E6%95%B0/106226 [accessed 2021-08-15].
- Baidu. Baidu Index. Available online: https://index.baidu.com/v2/index.html#/ [accessed 2021-08-15].
- Huang C, Huang L, Wang Y, et al. 6-month consequences of COVID-19 in patients discharged from hospital: a cohort study. Lancet 2021;397:220-32. [Crossref] [PubMed]
- Wiersinga WJ, Rhodes A, Cheng AC, et al. Pathophysiology, Transmission, Diagnosis, and Treatment of Coronavirus Disease 2019 (COVID-19): A Review. JAMA 2020;324:782-93. [Crossref] [PubMed]
- Galanopoulos M, Gkeros F, Doukatas A, et al. COVID-19 pandemic: Pathophysiology and manifestations from the gastrointestinal tract. World J Gastroenterol 2020;26:4579-88. [Crossref] [PubMed]
- Jin X, Lian JS, Hu JH, et al. Epidemiological, clinical and virological characteristics of 74 cases of coronavirus-infected disease 2019 (COVID-19) with gastrointestinal symptoms. Gut 2020;69:1002-9. [Crossref] [PubMed]
- Pascarella G, Strumia A, Piliego C, et al. COVID-19 diagnosis and management: a comprehensive review. J Intern Med 2020;288:192-206. [Crossref] [PubMed]
- Stricker BH. Epidemiology and 'big data'. Eur J Epidemiol 2017;32:535-6. [Crossref] [PubMed]
- Nakayama T. Evidence-based healthcare and health informatics: derivations and extension of epidemiology. J Epidemiol 2006;16:93-100. [Crossref] [PubMed]
- China Epidemic Prevention Network. Epidemic data. Available online: https://www.ncovchina.com/data.html [accessed 2021-08-10].
- National Bureau of Statistics. National Bureau of Statistics. Available online: http://www.stats.gov.cn/ [accessed 2021-08-24].
- Baidu Index. Search Index of COVID-19. Available online: https://index.baidu.com/v2/main/index.html#/trend/%E6%96%B0%E5%9E%8B%E5%86%A0%E7%8A%B6%E7%97%85%E6%AF%92?words=%E6%96%B0%E5%9E%8B%E5%86%A0%E7%8A%B6%E7%97%85%E6%AF%92 [accessed 2021-08-24].
- Sina Finance and Economics. What happened in the four weeks from "unknown pneumonia" to more than 2000 confirmed cases? Available online: https://baijiahao.baidu.com/s?id=1656790067341979140&wfr=spider&for=pc [accessed 2021-11-24].
- Wei S, Ma M, Wu C, et al. Using Search Trends to Analyze Web-Based Interest in Lower Urinary Tract Symptoms-Related Inquiries, Diagnoses, and Treatments in Mainland China: Infodemiology Study of Baidu Index Data. J Med Internet Res 2021;23:e27029. [Crossref] [PubMed]
- Menendez ME, Moverman MA, Moon AS, et al. State-level Google search volumes for neck and shoulder pain correlate with psychosocial and behavioral health indicators. J Natl Med Assoc 2021;113:522-7. [Crossref] [PubMed]
- Roger VL. Epidemiology of Heart Failure: A Contemporary Perspective. Circ Res 2021;128:1421-34. [Crossref] [PubMed]
- Hubei by the apparent. On January 23, traffic in Wuhan was suspended and the outbound passage was temporarily closed. Available online: https://baijiahao.baidu.com/s?id=1656463652711594195&wfr=spider&for=pc [accessed 2021-11-20].
- Hubei Provincial People's Government official website. Wuhan unsealed at midnight today. Available online: http://www.hubei.gov.cn/2019/tpyw/202004/t20200408_2207205.shtml [accessed 2021-12-11].
- Hajifathalian K, Krisko T, Mehta A, et al. Gastrointestinal and Hepatic Manifestations of 2019 Novel Coronavirus Disease in a Large Cohort of Infected Patients From New York: Clinical Implications. Gastroenterology 2020;159:1137-1140.e2. [Crossref] [PubMed]
- International news CCTV net. China continues to provide COVID-19 vaccine to many countries. Available online: http://news.cctv.com/2021/03/01/ARTIcfjz3boMa8iMNH9NXwgv210301.shtml [accessed 2021-12-11].
- The People's Daily. China has officially launched emergency use of COVID-19 vaccines. Available online: https://baijiahao.baidu.com/s?id=1675792577378666690&wfr=spider&for=pc [accessed 2021-12-21].
- Sajjadi NB, Nowlin W, Nowlin R, et al. United States internet searches for "infertility" following COVID-19 vaccine misinformation. J Osteopath Med 2021;121:583-7. [Crossref] [PubMed]
(English Language Editor: J. Teoh)