Exploration of new therapeutic targets for viral hepatic fibrosis, alcoholic hepatic fibrosis, and non-alcoholic hepatic fibrosis
Introduction
Hepatic fibrosis (HF) is a chronic liver disease and the main cause of hepatocellular carcinoma in the end stage. If the liver sustains continuous damage [e.g., from hepatitis B virus (HBV) or hepatitis C virus (HCV) infections, or chemical insults], the liver parenchymal cells could not be repaired to healthy cells. Moreover, hepatic stellate cells (HSCs) would be activated and transformed into a myofibroblast-like phenotype, leading to excessive extracellular matrix (ECM) deposition. Eventually, the normal structure of the liver is destroyed, resulting in hepatic fibrosis and liver dysfunction. Mild hepatic fibrosis is reversible, but in severe forms, it can progress to cirrhosis or even liver cancer (1,2).
At present, the main causes of hepatic fibrosis include viruses, alcohol, obesity, and cholestasis. Non-alcoholic liver disease is generally caused by obesity. When the fat accumulates around the liver tissue, it can trigger steatosis and cytotoxicity, resulting in hepatocyte response, HSCs transform into fibrocytes, and ECM proliferation causes non-alcoholic hepatic fibrosis (3). Virus-induced liver fibrosis is mainly caused by the HBV and HCV. HBV is transported into liver cells through receptors, and the nucleocapsid enveloping the virus deoxyribonucleic acid (DNA) enters the liver nucleus. The virus DNA is then released and integrates with the host DNA, and viral proteins are expressed after transcription, and the HBV virus infection is completed (4). The HCV attaches to liver cells and enters cells in a similar way to HBV, but it is ribonucleic acid (RNA) that carries the genetic information (5,6). Persistent viral infection leads to liver cell damage, causing liver fibrosis (7,8). In the long-term, heavy drinking can lead to the accumulation of acetaldehyde, which is a breakdown product of ethanol. Acetaldehyde can cause the metabolic disorder of liver cells, destroy liver cells, and cause liver inflammation (9). However, in the course of liver fibrosis caused by different factors, such as viral hepatitis, alcoholic hepatitis and non-alcoholic fatty hepatitis, whether the differentially expressed mRNAs and their functions are related, there are still no relevant studies.
In this study, transcriptome data were used to analyze the messenger RNA (mRNA) molecules commonly expressed in HBV, alcoholic and non-alcoholic liver fibrosis, which were expected to be common markers of hepatic fibrosis caused by different triggers and provide novel insights into the treatment of liver fibrosis.
The mRNA high-throughput sequencing data of this study were obtained from 3 microarray datasets (i.e., GSE171294, GSE142530, and GSE126848), which were downloaded from the Gene Expression Omnibus (GEO) database. Using these 3 datasets, the differentially expressed mRNAs in patients with HBV, alcoholic, and non-alcoholic hepatic fibrosis, and the differentially expressed mRNAs co-expressed in the 3 fibrotic diseases were identified. Afterwards, Gene Ontology (GO) and Kyoto Encyclopedia of Genes and Genomes (KEGG) pathway analyses were conducted to examine the enriched functions of the differential expressed mRNAs, and their biological functions were also explored. Finally, a protein-protein interaction (PPI) network analysis was performed to search for key protein interaction nodes. We present the following article in accordance with the STREGA reporting checklist (available at https://atm.amegroups.com/article/view/10.21037/atm-22-3593/rc).
Methods
Downloading the expression profile matrix
The GSE171294 dataset representing HBV liver fibrosis was obtained from the GPL24676 Illumina NovaSeq 6000 platform (Homo sapiens) and comprised 4 healthy samples and 4 liver fibrosis samples. The GSE142530 dataset representing alcoholic liver fibrosis was obtained from the GPL11154 Illumina HiSeq 2000 platform (Homo sapiens) and comprised 12 healthy samples and 6 hepatic fibrosis samples. Finally, the GSE126848 dataset for non-alcoholic hepatic fibrosis was obtained from the GPL18573 Illumina NextSeq 500 platform (Homo sapiens) and comprised 14 control samples and 16 hepatic fibrosis samples (see Table 1). The study was conducted in accordance with the Declaration of Helsinki (as revised in 2013).
Table 1
Data set | Type | Platforms | Normal | Hepatic fibrosis |
---|---|---|---|---|
GSE171294 | HBV hepatic fibrosis | GPL24676 Illumina NovaSeq 6000 (Homo sapiens) | 4 | 4 |
GSE142530 | Alcoholic hepatic fibrosis | GPL11154 Illumina HiSeq 2000 (Homo sapiens) | 12 | 6 |
GSE126848 | Non-alcoholic hepatic fibrosis | GPL18573 Illumina NextSeq 500 (Homo sapiens) | 14 | 16 |
HBV, hepatitis B virus.
Differential mRNA screening
Each dataset was analyzed separately, and the data were divided into “Control” and “Case” groups. The P value was calculated using the unpaired T test in the limma program package in R language, and the P value was corrected using the Benjamini & Hochberg (BH) method. For each significantly differentially expressed mRNA, a P value <0.05 and |log fold change| >0.5 were required. The differentially expressed mRNA heat map was generated by the R package pheatmap, and the volcano map was generated by the ggplot.
Pathway enrichment analysis
The GO and KEGG pathway functional enrichment analyses of the differentially expressed mRNAs were conducted using the “Database for Annotation, Visualization and Integrated Discovery (DAVID)” analysis tool. The biological processes (BPs), molecular functions (MFs), and cellular components (CCs) in which the differential mRNAs were involved were analyzed by a GO functional enrichment analysis. A Kyoto Encyclopedia of Genes and Genomes (KEGG) analysis was conducted to determine the signaling pathways in which the differentially expressed mRNAs might participate. A P value <0.05 indicated a significantly enriched result.
Construction of the PPI network
The Search Tool for the Retrieval of Interacting Genes (STRING) was used to predict whether there was any interaction between the proteins encoded by the differentially expressed mRNAs. The input gene datasets comprised the differentially expressed mRNAs and the species of “Homo sapiens” was selected. The parameter PPI score was set to 0.4, which required that the protein nodes in which the interactions occurred were the differentially expressed mRNAs. PPI networks of differentially expressed mRNAs were constructed using Cytoscape software. The scores of nodes in the network were analyzed by a measure of topological properties; that is, the degree centrality. The higher the score of a node, the more important it is in the network and the more likely it is to be a critical node.
Results
Results of the differential mRNA screening
Using the Ensembl official website (http://asia.ensembl.org/index.html), we converted the original data (Ensemble_ID) into the corresponding name (symbol), and the average values of multiple probes were taken as the expression values of the genes. The differential genes were analyzed and screened with R language. Specifically, 1,071, 2,752, and 9,721 were screened from the GSE171294, GSE142530, and GSE126848 datasets, respectively. Heat maps were drawn for the differential mRNAs of the control group and the case group in the 3 datasets (see Figure 1A-1C). After the Venn diagram was intersected, 54 differentially expressed mRNAs were identified in the 3 datasets, which accounted for 0.5% of the total differentially expressed mRNAs (see Figure 2).
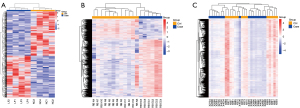
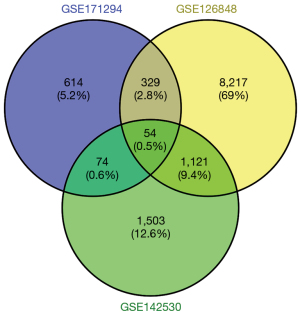
Pathway enrichment analysis
To further investigate the function and mechanism of the 54 differentially expressed mRNAs, GO and KEGG enrichment analyses were performed in DAVID. The results showed that the differential genes were enriched in 75 KEGG pathways (see Figure 3), 2,344 GO-BP pathways, 324 GO-CC pathways, and 346 GO-MF pathways (see Figures 4,5). Among them, 3 KEGG pathways (see Table 2), 654 GO-BPs, 126 GO-CCs, and 124 GO-MFs (Table 3) were significantly enriched.
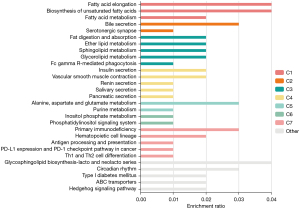
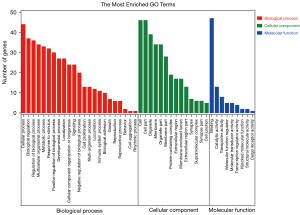
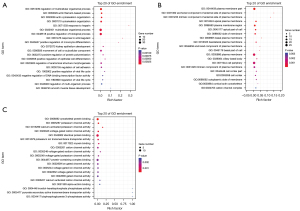
Table 2
Pathway | Pathway ID | P value | Gene |
---|---|---|---|
GnRH secretion | hsa04929 | <0.05 | CACNA1H, CAV3.2, KCNN2, KCA2.2 |
Bile secretion | hsa04976 | <0.05 | KCNN2, KCA2.2, OSTALPHA, OSTA, SLC51A1 |
Insulin secretion | hsa04911 | <0.05 | KCNMA1, KCA1.1, KCNN2, KCA2.2 |
KEGG, Kyoto Encyclopedia of Genes and Genomes; mRNAs, messenger ribonucleic acids.
Table 3
GO-annotations | Description | Count | P value |
---|---|---|---|
GO-BP | Regulation of multicellular organismal process | 22 | <0.05 |
Actin filament-based process | 10 | <0.05 | |
Actin cytoskeleton organization | 9 | <0.05 | |
Cytoskeleton organization | 13 | <0.05 | |
Response to heparin | 2 | <0.05 | |
GO-CC | Plasma membrane part | 21 | <0.05 |
Anchored component of external side of plasma membrane | 3 | <0.05 | |
Intrinsic component of external side of plasma membrane | 3 | <0.05 | |
Apical plasma membrane | 7 | <0.05 | |
Plasma membrane region | 12 | <0.05 | |
GO-MF | Cytoskeletal protein binding | 10 | <0.05 |
Potassium channel activity | 4 | <0.05 | |
Calcium-activated potassium channel activity | 2 | <0.05 | |
Voltage-gated cation channel activity | 4 | <0.05 | |
Identical protein binding | 14 | <0.05 |
GO, Gene Ontology; mRNAs, messenger ribonucleic acids; BP, biological process; CC, cellular component; MF, molecular function.
Results of the PPI network analysis
Venn diagrams were used to intersect the differential mRNAs in the 3 expression profiling datasets, which resulted in 54 differential mRNAs. The PPI network was constructed, and a total of 16 nodes and 12 interaction pairs were found (see Figure 6). The thicker the line between the nodes, the more important the node. The expression levels of the key genes associated with 12 interaction pairs from the 3 samples for the case and control groups are shown in Figure 7.
Discussion
Following the development of sequencing technology, an increasing number of hepatic fibrosis molecules with different etiologies have been able to be identified, and these molecules have become new therapeutic targets for hepatic fibrosis with different triggers. Little research has been conducted on whether these molecules or pathways regulate hepatic fibrosis of different etiologies. The chronic stimulation of the hepatitis virus, alcohol, lipids, and other stressors cause damage to the liver parenchymal cells. In response to liver injury, HSCs are activated and exhibit a myofibroblast-like phenotype, which contributes to the excessive synthesis and deposition of the ECM. The excessive deposition of the type I collagen-dominated ECM leads to liver fibrogenesis. These are the common pathological features of liver fibrosis induced by different factors. Thus, determining the molecules or pathways of liver fibrosis with different triggers could lead to the development of novel strategies for the treatment and intervention of hepatic fibrosis and its progression.
At present, transforming growth factor (TGF)-β, Wnt/β-catenin, PDGF and other signaling pathways have been widely studied in the molecular mechanism of liver fibrosis (10). After hepatocytes injury, the expression of TGF-β is up-regulated, which promotes the transformation of hepatic stellate cells into myofibroblasts (MFB) that secrete extracellular matrix (11). However, TGF-β has been found to inhibit inflammation while promoting fibrosis (12). In addition to TGF-β, it can also induce the upregulation of growth factors in MFB cells through Wnt signaling pathway, such as the upregulation of Wnt-5, which promotes the proliferation of MFB and the secretion of extracellular matrix (11). ROS is also closely related to the occurrence of liver fibrosis. When there is an imbalance between oxidation and anti-oxidation, the level of ROS increases, which affects the proliferation and apoptosis of hepatocytes. At the same time, TGF-β and ROS act as activators of each other’s signaling pathways, respectively, inducing damage and fibrosis (13). Stemming form the concern of clinicians about the common denominator of any liver disease characterized by chronic tissue damage, therapeutic strategies for hepatic fibrosis have primarily focused on (I) inducing specific elimination of profibrotic cells, or, reversing or senescence; (II) increasing degradation of ECM; (III) transplantation of bone marrow-derived cells (i.e., macrophages); (IV) targeting myofibroblasts (MFs) and/or the mechanisms and signaling pathways of their activating and profibrotic effects; (V) targeting Kupffer cells (KC, liver resident and self-sustaining macrophages) and monocyte-derived macrophages (MoMF) and/or the mechanisms and signaling pathways of their recruitment/activation; (VI) drugs that reduce liver parenchymal injury (14). Therefore, currently, the research on therapeutic targets for liver fibrosis mainly focuses on which stages of fibrosis evolution to maximize targeting and which are relatively realistic clinical endpoints (14).
Bioinformatic transcriptome analyses were performed on the HBV hepatic fibrosis microarray GSE171294 dataset, alcoholic hepatic fibrosis microarray GSE142530 dataset, and non-alcoholic hepatic fibrosis microarray GSE126848 dataset from the GEO public database, and 1,071, 2,752, and 9,721 differentially expressed mRNAs were identified for the GSE171294, GSE142530, and GSE126848 datasets, respectively. Among them, there were 54 common differentially expressed mRNAs. KGEE and GO enrichment analyses were then conducted for the 54 differentially expressed mRNAs. KEGG enrichment analysis showed that the differential gene expression was related to the Gonadotropin Releasing Hormone (GnRH) secretion, bile secretion, insulin secretion, vascular smooth muscle contraction, biosynthesis of unsaturated fatty acids, and other signaling pathways, especially the first three. The GO enrichment analysis showed that the differential mRNAs were mainly present in the cytoplasmic membrane region and exerted biological functions, such as activating channels and binding proteins by regulating cells, cytoskeleton, heparin and other BPs. The PPI network analysis revealed 16 critical nodes, which may serve as common therapeutic targets for the treatment of hepatic fibrosis induced by different causes.
GnRH is associated with cholestatic hepatic fibrosis. GnRH is secreted by the hypothalamus, and can bind to the GnRH receptors present in the human biliary tract (15,16). In a rat model of bile duct ligation, GnRH secretion was shown to be increased. Conversely, research has also shown that after treatment with melatonin, which is another hormone secreted by the hypothalamus, GnRH secretion is decreased, and liver biochemical indicators, liver tissue inflammation, and fibrosis are improved (17,18). In the 1990s, it was revealed that patients with alcoholic and non-alcoholic cirrhosis had significant differences in GnRH levels compared to healthy individuals (19). However, little mechanistic research or research on GnRH and hepatitis virus-induced hepatic fibrosis has been conducted. In addition, after stimulation with the air pollutant of perfluorohexanesulfonic acid, which relies on Na+ participation in bile acid enterohepatic circulation, and the elimination of toxic substances through biliary excretion, disrupts lipid metabolism in liver tissues causing non-alcoholic steatohepatitis (20,21). Intestinal microbes also have an effect on liver fibrogenesis; for example, individuals with alcoholic, non-alcoholic hepatic fibrosis, HBV- and HCV-induced hepatic fibrosis have increased bile acids compared to healthy, for the altered intestinal flora or over-expression of bile acid-related DNA in the flora (22-25).
In addition to ethanol stimulation, reduced bile acid excretion has also been found to be another cause of alcoholic hepatic fibrosis (26). Decreased or inhibited insulin secretion is the main cause of diabetes mellitus. Diabetic patients are also usually obese and have non-alcoholic hepatic fibrosis (27). Japanese scholars have found that fasting insulin secretion could decrease with the process of non-alcoholic hepatic fibrosis (28), which may be related to glucose metabolism disorder and lipid metabolism disorder caused by islet β cell dysfunction (29). Moreover, advanced HCV hepatic fibrosis is associated with insulin resistance (30).
According to a previous study (31), the ion channels are mainly related to HSCs, while the differentially expressed mRNAs in cells are mainly enriched in the plasma membrane and cell membrane. Thus, these differentially expressed molecules may mainly be present on the cell membrane of HSCs. HSCs can be stimulated to transform into fibroblasts. When the calcium-activated potassium channel (KCa) activity was inhibited, the HSC proliferation was decreased, TGF-β1 activation was inhibited and hepatic fibrosis was reversed (31,32). As the process of hepatic fibrosis worsens, KCa3.1 expression also increases in the liver, and anti-fibrotic effects can be achieved in non-alcoholic fatty liver disease by inhibiting KCa3.1 (33). In addition, other ion channels in fibroblasts, such as inwardly rectifying potassium channels, have been reported in fibrosis tissues except for hepatic fibrosis tissues (34). Actin, myosin, and the mechanism of hepatic fibrosis development has also attracted a great deal of attention. Actin and myosin are important components of the cytoskeleton, and are involved in a variety of biological cell functions, such as the proliferation and migration of cells. The knockdown of smooth muscle α-actin was shown to reduce the expression of hepatic fibrosis (35) and targeting myosin 1c (Myo1c) was shown to suppress hepatic fibrosis in mice (36). Inward rectifying potassium channels have been reported in other tissue fibrosis, but not in liver fibrosis.
Ca2+-activated K+ channel protein gene 2 (KCNN2) is expressed in liver tissue (37) and a small-conductance Ca2+-activated potassium channel (SK2) is encoded in biliary epithelial cells that affect bile secretion through ion channels (38). In addition, the level of intramyocardial fibrosis has also been found to decrease following the sacubitril/valsartan treatment of cardiac arrhythmias, due to the downregulation of KCNN2 (39). No research has shown that KCNN2 has a supporting effect on hepatic fibrosis; however, from the above, it can be speculated that KCNN2 encodes the biliary epithelial cell SK2, which could affect bile flow through SK2 and induce cholestasis, which in turn could lead to hepatic fibrosis.
CD4 has frequently been reported in viral hepatic fibrosis and non-alcoholic hepatic fibrosis (37,38). However, the effect of CD4 on liver fibrosis appears to be insignificant. For example, while the number of CD4+ T cells is reduced after antiviral therapy, HCV hepatic fibrosis is not alleviated (40). Moreover, there is no significant difference in CD4+ lymphocytes between patients with non-alcoholic hepatic fibrosis and controls (40,41). The analysis results of a study on CD4+ T lymphocytes and hepatic fibrosis did not comply with the expected assumptions, and no other relevant studies have been conducted; however, this issue is worthy of further in-depth study.
The expression of SOX9 in the liver, skin and other tissues is associated with fibrosis, and the liver fibrosis caused by biliary atresia is related to the abnormal expression of SOX9 and the proliferation of hepatic progenitor cells (42). PKHD1 or PKD2 mutations are the main cause of autosomal recessive polycystic kidney disease (ARPKD) (43). The organoid models of hepatic fibrosis are often designed as ARPKD, and studies have shown that TGF-β activation in the model is involved in fibrosis production (10,43,44). KCNMA1, the pore-forming α subunit of the large-conductance Ca2+-activated K+ channel (BK channels) is expressed in HSCs, and KCNMA1 over-expression leads to reduced collagen expression in HSCs, which inhibits hepatic fibrosis (45). In addition to the above-mentioned molecules, CD24 and B lymphocytoma 6 (BCL6), which are mainly involved in the immune response, as well as CACNA1H, which is a molecule related to calcium channels, have rarely been examined; however, we intend to examine these molecules in our future studies.
The use of large databases for bioinformatics analysis of clinical diseases has attracted more and more attention. Statistical methods are used to screen differentially expressed genes, to understand the functions of differentially expressed genes, to analyze the relationship between proteins encoded by differentially expressed molecules, and to verify target genes by in vitro and in vivo experiments. It is a good way to provide candidate targets for the prevention and treatment of liver fibrosis.
Diverse mechanisms underlie hepatic fibrosis in vivo. The results of the present study showed that liver fibrosis is mainly related to cellular ion channels and channel molecules. The mechanism of the same molecule differs in different inducing factors of liver fibrosis, and the mechanism of different molecules is similar in different inducing factors of liver fibrosis. Voltage-gated cation channels (i.e., KCNN2, CD4, CD24, BCL6, KCNMA1, and other molecules) could be used as novel research targets for hepatic fibrosis induced by different causes, which could provide novel ideas for the treatment of hepatic fibrosis.
Acknowledgments
Funding: The study was funded by Youth Science and Technology Research Fund of Shanxi Provincial Department of Science and Technology (No. 201901D211529); Youth Fund of Health Commission of Shanxi Province (No. 2021081); and Research Fund of Shanxi Provincial Administration of Traditional Chinese Medicine (No. 2020ZYYC025).
Footnote
Reporting Checklist: The authors have completed the STREGA reporting checklist. Available at https://atm.amegroups.com/article/view/10.21037/atm-22-3593/rc
Conflicts of Interest: All authors have completed the ICMJE uniform disclosure form (available at https://atm.amegroups.com/article/view/10.21037/atm-22-3593/coif). The authors have no conflicts of interest to declare.
Ethical Statement: The authors are accountable for all aspects of the work in ensuring that questions related to the accuracy or integrity of any part of the work are appropriately investigated and resolved. The study was conducted in accordance with the Declaration of Helsinki (as revised in 2013).
Open Access Statement: This is an Open Access article distributed in accordance with the Creative Commons Attribution-NonCommercial-NoDerivs 4.0 International License (CC BY-NC-ND 4.0), which permits the non-commercial replication and distribution of the article with the strict proviso that no changes or edits are made and the original work is properly cited (including links to both the formal publication through the relevant DOI and the license). See: https://creativecommons.org/licenses/by-nc-nd/4.0/.
References
- Marrone G, Shah VH, Gracia-Sancho J. Sinusoidal communication in liver fibrosis and regeneration. J Hepatol 2016;65:608-17. [Crossref] [PubMed]
- Friedman SL. Liver fibrosis -- from bench to bedside. J Hepatol 2003;38:S38-53. [Crossref] [PubMed]
- Kumar S, Duan Q, Wu R, et al. Pathophysiological communication between hepatocytes and non-parenchymal cells in liver injury from NAFLD to liver fibrosis. Adv Drug Deliv Rev 2021;176:113869. [Crossref] [PubMed]
- Tsai E. Review of Current and Potential Treatments for Chronic Hepatitis B Virus Infection. Gastroenterol Hepatol (N Y) 2021;17:367-76. [PubMed]
- Gerold G, Moeller R, Pietschmann T, Hepatitis C. Virus Entry: Protein Interactions and Fusion Determinants Governing Productive Hepatocyte Invasion. Cold Spring Harb Perspect Med 2020;10:a036830. [Crossref] [PubMed]
- Miao Z, Xie Z, Miao J, et al. Regulated Entry of Hepatitis C Virus into Hepatocytes. Viruses 2017;9:100. [Crossref] [PubMed]
- Zhao F, Xie X, Tan X, et al. The Functions of Hepatitis B Virus Encoding Proteins: Viral Persistence and Liver Pathogenesis. Front Immunol 2021;12:691766. [Crossref] [PubMed]
- Biliotti E, Giampaoli O, Sciubba F, et al. Urinary metabolomics of HCV patients with severe liver fibrosis before and during the sustained virologic response achieved by direct acting antiviral treatment. Biomed Pharmacother 2021;143:112217. [Crossref] [PubMed]
- Patel AM, Liu YS, Davies SP, et al. The Role of B Cells in Adult and Paediatric Liver Injury. Front Immunol 2021;12:729143. [Crossref] [PubMed]
- Dewidar B, Meyer C, Dooley S, et al. TGF-β in Hepatic Stellate Cell Activation and Liver Fibrogenesis-Updated 2019. Cells 2019;8:1419. [Crossref] [PubMed]
- Beljaars L, Daliri S, Dijkhuizen C, et al. WNT-5A regulates TGF-β-related activities in liver fibrosis. Am J Physiol Gastrointest Liver Physiol 2017;312:G219-G227. [Crossref] [PubMed]
- Qiao X, Rao P, Zhang Y, et al. Redirecting TGF-β Signaling through the β-Catenin/Foxo Complex Prevents Kidney Fibrosis. J Am Soc Nephrol 2018;29:557-70. [Crossref] [PubMed]
- Roehlen N, Crouchet E, Baumert TF. Liver Fibrosis: Mechanistic Concepts and Therapeutic Perspectives. Cells 2020;9:875. [Crossref] [PubMed]
- Parola M, Pinzani M. Liver fibrosis: Pathophysiology, pathogenetic targets and clinical issues. Mol Aspects Med 2019;65:37-55. [Crossref] [PubMed]
- Neill JD. GnRH and GnRH receptor genes in the human genome. Endocrinology 2002;143:737-43. [Crossref] [PubMed]
- Cheng CK, Leung PC. Molecular biology of gonadotropin-releasing hormone (GnRH)-I, GnRH-II, and their receptors in humans. Endocr Rev 2005;26:283-306. [Crossref] [PubMed]
- McMillin M, DeMorrow S, Glaser S, et al. Melatonin inhibits hypothalamic gonadotropin-releasing hormone release and reduces biliary hyperplasia and fibrosis in cholestatic rats. Am J Physiol Gastrointest Liver Physiol 2017;313:G410-8. [Crossref] [PubMed]
- Ray D, Han Y, Franchitto A, et al. Gonadotropin-releasing hormone stimulates biliary proliferation by paracrine/autocrine mechanisms. Am J Pathol 2015;185:1061-72. [Crossref] [PubMed]
- Bell H, Raknerud N, Falch JA, et al. Inappropriately low levels of gonadotrophins in amenorrhoeic women with alcoholic and non-alcoholic cirrhosis. Eur J Endocrinol 1995;132:444-9. [Crossref] [PubMed]
- Sen P, Qadri S, Luukkonen PK, et al. Exposure to environmental contaminants is associated with altered hepatic lipid metabolism in non-alcoholic fatty liver disease. J Hepatol 2022;76:283-93. [Crossref] [PubMed]
- Zhao W, Zitzow JD, Ehresman DJ, et al. Na+/Taurocholate Cotransporting Polypeptide and Apical Sodium-Dependent Bile Acid Transporter Are Involved in the Disposition of Perfluoroalkyl Sulfonates in Humans and Rats. Toxicol Sci 2015;146:363-73. [Crossref] [PubMed]
- Adams LA, Wang Z, Liddle C, et al. Bile acids associate with specific gut microbiota, low-level alcohol consumption and liver fibrosis in patients with non-alcoholic fatty liver disease. Liver Int 2020;40:1356-65. [Crossref] [PubMed]
- Inoue T, Funatsu Y, Ohnishi M, et al. Bile acid dysmetabolism in the gut-microbiota-liver axis under hepatitis C virus infection. Liver Int 2022;42:124-34. [Crossref] [PubMed]
- Hartmann P, Hochrath K, Horvath A, et al. Modulation of the intestinal bile acid/farnesoid X receptor/fibroblast growth factor 15 axis improves alcoholic liver disease in mice. Hepatology 2018;67:2150-66. [Crossref] [PubMed]
- Kassa Y, Million Y, Gedefie A, et al. Alteration of Gut Microbiota and Its Impact on Immune Response in Patients with Chronic HBV Infection: A Review. Infect Drug Resist 2021;14:2571-8. [Crossref] [PubMed]
- Kong B, Zhang M, Huang M, et al. FXR deficiency alters bile acid pool composition and exacerbates chronic alcohol induced liver injury. Dig Liver Dis 2019;51:570-6. [Crossref] [PubMed]
- Fernandes GW, Bocco BMLC. Hepatic Mediators of Lipid Metabolism and Ketogenesis: Focus on Fatty Liver and Diabetes. Curr Diabetes Rev 2021;17:e110320187539. [Crossref] [PubMed]
- Araki N, Takahashi H, Takamori A, et al. Decrease in fasting insulin secretory function correlates with significant liver fibrosis in Japanese non-alcoholic fatty liver disease patients. JGH Open 2020;4:929-36. [Crossref] [PubMed]
- Chen X, Xiao J, Pang J, et al. Pancreatic β-Cell Dysfunction Is Associated with Nonalcoholic Fatty Liver Disease. Nutrients 2021;13:3139. [Crossref] [PubMed]
- Gualerzi A, Bellan M, Smirne C, et al. Improvement of insulin sensitivity in diabetic and non diabetic patients with chronic hepatitis C treated with direct antiviral agents. PLoS One 2018;13:e0209216. [Crossref] [PubMed]
- Freise C, Heldwein S, Erben U, et al. K+-channel inhibition reduces portal perfusion pressure in fibrotic rats and fibrosis associated characteristics of hepatic stellate cells. Liver Int 2015;35:1244-52. [Crossref] [PubMed]
- Sevelsted Møller L, Fialla AD, Schierwagen R, et al. The calcium-activated potassium channel KCa3.1 is an important modulator of hepatic injury. Sci Rep 2016;6:28770. [Crossref] [PubMed]
- Paka L, Smith DE, Jung D, et al. Anti-steatotic and anti-fibrotic effects of the KCa3.1 channel inhibitor, Senicapoc, in non-alcoholic liver disease. World J Gastroenterol 2017;23:4181-90. [Crossref] [PubMed]
- Roach KM, Bradding P. Ca2+ signalling in fibroblasts and the therapeutic potential of KCa3.1 channel blockers in fibrotic diseases. Br J Pharmacol 2020;177:1003-24. [Crossref] [PubMed]
- Rockey DC, Du Q, Weymouth ND, et al. Smooth Muscle α-Actin Deficiency Leads to Decreased Liver Fibrosis via Impaired Cytoskeletal Signaling in Hepatic Stellate Cells. Am J Pathol 2019;189:2209-20. [Crossref] [PubMed]
- Arif E, Wang C, Swiderska-Syn MK, et al. Targeting myosin 1c inhibits murine hepatic fibrogenesis. Am J Physiol Gastrointest Liver Physiol 2021;320:G1044-53. [Crossref] [PubMed]
- Szatanik M, Vibert N, Vassias I, et al. Behavioral effects of a deletion in Kcnn2, the gene encoding the SK2 subunit of small-conductance Ca2+-activated K+ channels. Neurogenetics 2008;9:237-48. [Crossref] [PubMed]
- Feranchak AP, Doctor RB, Troetsch M, et al. Calcium-dependent regulation of secretion in biliary epithelial cells: the role of apamin-sensitive SK channels. Gastroenterology 2004;127:903-13. [Crossref] [PubMed]
- Sung YL, Lin TT, Syu JY, et al. Reverse electromechanical modelling of diastolic dysfunction in spontaneous hypertensive rat after sacubitril/valsartan therapy. ESC Heart Fail 2020;7:4040-50. [Crossref] [PubMed]
- Zientarska A, Kaczmarek M, Mozer-Lisewska I, et al. Treg cells in the course of chronic hepatitis C virus infection partially normalize in longitudinal observation after successful DAA treatment regardless of hepatic fibrosis stage. Clin Exp Hepatol 2021;7:196-204. [Crossref] [PubMed]
- Cairoli V, De Matteo E, Rios D, et al. Hepatic lymphocytes involved in the pathogenesis of pediatric and adult non-alcoholic fatty liver disease. Sci Rep 2021;11:5129. [Crossref] [PubMed]
- El-Araby HA, Saber MA, Radwan NM, et al. SOX9 in biliary atresia: New insight for fibrosis progression. Hepatobiliary Pancreat Dis Int 2021;20:154-62. [Crossref] [PubMed]
- Guan Y, Enejder A, Wang M, et al. A human multi-lineage hepatic organoid model for liver fibrosis. Nat Commun 2021;12:6138. [Crossref] [PubMed]
- Adhyatmika A, Putri KSS, Gore E, et al. Osteoprotegerin Expression in Liver is Induced by IL13 through TGFβ. Cell Physiol Biochem 2022;56:28-38. [Crossref] [PubMed]
- Yang L, Han B, Zhang M, et al. Activation of BK Channels Prevents Hepatic Stellate Cell Activation and Liver Fibrosis Through the Suppression of TGFβ1/SMAD3 and JAK/STAT3 Profibrotic Signaling Pathways. Front Pharmacol 2020;11:165. [Crossref] [PubMed]
(English Language Editor: L. Huleatt)