Identification of key genes and pathway related to chemoresistance of small cell lung cancer through an integrative bioinformatics analysis
Introduction
In the global cancer statistics for 2020, lung cancer ranked 2nd in terms of new cases and deaths (1). Thus, lung cancer represents a serious threat to human health and survival. Lung cancer is divided into 2 categories; that is, non-small cell lung cancer (NSCLC) and small cell lung cancer (SCLC) (2). SCLC accounts for only 15% of all lung cancers (2). Conventional SCLC treatments include chemotherapy, radiation therapy, and surgery, among which chemotherapy is the primary treatment approach (3). The traditional 1st-line chemotherapy regimen comprises etoposide (VP-16) and cisplatin (CDDP) (4). However, due to chemoresistance, SCLC is characterized by high recurrence and metastasis rates after 1st-line chemotherapy and low efficiency and short remission periods after 2nd-line chemotherapy; thus, the 5-year survival rate of SCLC patients is low, and their prognosis is poor (5). The identification of key genes in the pathogenesis of SCLC would provide an important theoretical basis for discovering possible diagnostic or prognostic biomarkers.
Protein-coding genes play an important role in the development of SCLC; however, the sequencing of the human genome has shown that <3% of genomes can encode proteins (6). Long non-coding RNA (lncRNA) is a non-coding RNA molecule >200 nucleotides in length (7). Its function has received increasing attention in cancer research, including in SCLC (8). An increasing number of reports suggest that lncRNAs are involved in the development and chemotherapy resistance of SCLC (9). For example, MCM3AP-AS1 could promote cell invasion and migration by sponging miR-148a (10). ZFPM2-AS1 could facilitate cell proliferation and invasion by sponging miR-3612 (11). However, the underlying mechanisms need to be explored further.
In recent years, gene microarray technology and bioinformatics analyses have been widely used in genomics research. However, the high aggressiveness and short survival of SCLC have resulted in limited relevant gene microarray data and limited drug resistance-related data sets. We previously performed microarray-based messenger RNA (mRNA) and lncRNA expression profiling using the SCLC sensitive cell line, H69, and the SCLC chemoresistant cell line, H69AR. Among the differentially expressed genes (DEGs), some have important significance in SCLC. For example, according to our previous studies, HOTTIP (12) and NCRNA00173 (13) appear to induce SCLC chemoresistance by upregulating BCL-2 and Etk expression, respectively. However, this microarray the microarray-based mRNA and lncRNA expression profiling of H69 and H69AR has not been comprehensively analyzed.
In this study, we conducted an integrative analysis of the lncRNA-mRNA expression profiles and functional networks involved in the chemoresistance of SCLC. By searching for the DEGs and differentially expressed lncRNAs (DELs) using bioinformatics techniques, performing a functional analysis, constructing protein-protein interaction (PPI) networks, conducting a co-expression analysis and a cis-regulation analysis, integrating the SCLC microarray data set GSE149507 from the Gene Expression Omnibus (GEO) database, and validating the gene expression in vitro, we sought to improve understandings of the molecular mechanisms of SCLC chemoresistance. We present the following article in accordance with the MDAR reporting checklist (available at https://atm.amegroups.com/article/view/10.21037/atm-22-3642/rc).
Methods
Cell culture
The human SCLC cell lines NCI-H69 and NCI-H446, and the chemoresistant cell line NCI-H69AR were purchased from the American Type Culture Collection (USA). The CDDP-resistant NCI-H446DDP cell line was constructed in our laboratory (14). These cell lines were all cultured in RPMI-1640 (Invitrogen) supplemented with 10% fetal bovine serum (HyClone) at 37 ℃ with 5% carbon dioxide.
CCK-8 assays
At a density of 5×103 cells per well, the H69, H69AR, H446, and H446DDP cells were inoculated in 96-well plates and treated for 24 h with the chemotherapy drugs of CDDP (Shandong, China), VP-16 (Jiangshu, China), or Adriamycin (ADM; Jiangshu, China). Next, the cells were incubated with 10 µL of Cell Counting Kit-8 (CCK-8; C0038, Beyotime, China) solution for 2 h, and measured for the absorbance at 450 nm, which were used. The value of absorbance at 450 nm were used to calculate the IC50 values of each chemotherapeutic drug after 3 independent experiments. The data described biological replicates.
LncRNA/mRNA expression profiling
The total RNA from each sample was extracted and quantified by the NanoDrop ND-1000. RNA integrity was assessed by standard denaturing agarose gel electrophoresis. For the microarray analysis, Human LncRNA Array v2.0 (8 x 60K, Arraystar, USA) was employed. The sample preparation and microarray hybridization were performed based on the manufacturer’s standard protocols with minor modifications. The arrays were scanned by the Agilent Scanner G2505B. Agilent Feature Extraction software (version 11.0.1.1) was used to analyze the acquired array images. Quantile normalization and subsequent data processing were performed using the GeneSpring GX v12.0 software package (Agilent Technologies, USA). After the quantile normalization of the raw data, lncRNAs and mRNAs for which at least 3 out of the 6 samples had flags in the Present or Marginal (“All Targets Value”) were chosen for further data analysis.
Identification of the DEGs and DELs
R package limma (version 3.40.6) (15) was used to identify the DEGs and DELs between the H69 group and H69AR group based on the following screening criteria: a |log fold change (FC)| ≥1 and an adjusted P value <0.05, as shown by volcano plot filtering. Hierarchical clustering was performed to show the distinguishable DEG and DEL expression patterns among the samples.
Gene Ontology (GO) and Kyoto Encyclopedia of Genes and Genomes (KEGG) enrichment analyses
ID Conversion was performed via org.Hs.eg.db package (3.10.0). GO and KEGG enrichment analyses were performed by the clusterProfiler package (3.14.3) (16) against the background of Homo sapiens. The top 10 enriched terms with the smallest adjusted P values were visualized.
PPI network construction
The DEGs were uploaded onto the Search Tool for the Retrieval of Interacting Genes/Proteins (STRING) database (11.5) for the PPI network construction with an interaction score of 0.7. The top 10 hub genes were analyzed in terms of betweenness, closeness, and degree. The overlapping genes were identified as hub genes.
The co-expressed DEGs of the top 10 upregulated DELs and the hub genes
The correlated genes of the top 10 DELs were screened by a Spearman analysis, with the threshold of a |c| ≥0.9 and a P value <0.05. A PPI network based on the correlated genes was constructed with an interaction score of 0.4, and the hub genes were screened out using the degree algorithm.
Cis-regulation prediction
The DEGs that were situated within 100 kbp range upstream and downstream of the selected lncRNAs and correlated with the selected lncRNAs (P<0.05) were predicted as the cis-regulated DEGs by the top 10 DELs.
Gene expression validation in GSE149507
The DEGs in the GSE149507 data set from the GEO database were analyzed using limma R package. The expression of each gene was merged based on the median expression value of their multiple probes, and then measured by both a Wilcoxon rank-sum test and paired t-test. The study was conducted in accordance with the Declaration of Helsinki (as revised in 2013).
qRT-PCR validation
The total RNA was extracted from the H69, H69AR, H446, and H446DDP cells by TRIzol (Invitrogen, Grand Island, NY, USA), reverse transcribed into complementary DNA according to the instructions of the AMV reverse transcription kit (Invitrogen, USA), and amplified according to the instructions of the SYBR green PCR Master kit (TaKaRa, Dalian Baosheng Bio, China) on a real-time polymerase chain reaction (PCR) instrument according to the manufacturer’s instructions. GAPDH served as the internal reference. The quantitative PCR (qPCR) primers used are listed in Table S1. The PCR amplification conditions were: 95 ℃ for 10 min, 40 cycles of 95 ℃ for 15 s, 60 ℃ for 30 s, and 72 ℃ for 30 s. The relative expression levels of the genes were quantified using the 2-ΔΔCt method after 3 independent experiments. The data described biological replicates.
Statistical analysis
The data analyses were performed using R language (3.6.3) and GraphPad Prism (9.0.0, GraphPad Software, Inc.). The IC50 values of the sensitive and chemoresistance cells were compared, and the DEGs in the 2 pairs of the sensitive and chemoresistance SCLC cell lines were tested by t-tests. The bioinformatics analysis visualization was achieved mainly through ggplot2 (3.6.3) package. A P value <0.05 indicated a statistically significant difference.
Results
The chemosensitivity and mRNA/lncRNA expression profiles of the sensitive and chemoresistant SCLC cells
The sensitive cell line H69 and chemoresistant cell line H69AR were incubated with chemotherapeutic drugs (i.e., ADM, CDDP, and VP-16), and their cell IC50 values were measured by CCK-8 assays. The results showed that the H69AR cells had higher IC50 values than the H69 cells (see Figure 1A).
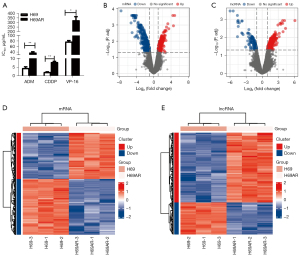
We collected the H69 and H69AR cells, extracted the total RNA, and conducted a microarray analysis. We screened out the DEGs and DELs using the following criteria: a |logFC| ≥1, and an adjusted P value <0.05. The volcanao maps of mRNA and lncRNAs showed that we identified 609 DEGs, of which 276 were upregulated and 333 were downregulated (see Figure 1B), and 394 DELs, of which 270 were upregulated and 124 were downregulated (see Figure 1C). The results above were also demonstrated by heat maps (Figure 1D,1E). The top 10 mRNAs were FAM167A, NM_014621, HOXB9, NEUROG2, KCNQ2, COL23A1, C2orf70, FAM38A, LNPEP, and C3orf70 (see Table 1). The top 10 lncRNAs were BC084573, AC009336.24, RP11-153F5.1, AK093987, lincRNA-EN2-1, RP11-513G11.1, RP11-520D19.2, BC047481, NCRNA00173, and CR936677 (see Table 2).
Table 1
Gene name | LogFC | Regulation |
---|---|---|
FAM167A | 4.8416287 | Up |
NM_014621 | 4.5614647 | Up |
HOXB9 | 4.4114865 | Up |
NEUROG2 | 3.4677203 | Up |
KCNQ2 | 3.253462333 | Up |
COL23A1 | 2.9453295 | Up |
C2orf70 | 2.868844433 | Up |
FAM38A | 2.827368867 | Up |
LNPEP | 2.746439533 | Up |
C3orf70 | 2.680554733 | Up |
LogFC, Log fold change.
Table 2
Gene name | LogFC | Regulation |
---|---|---|
BC084573 | 6.112119233 | Up |
AC009336.24 | 4.360588 | Up |
RP11-153F5.1 | 4.175123267 | Up |
AK093987 | 3.898287567 | Up |
lincRNA-EN2-1 | 3.829717533 | Up |
RP11-513G11.1 | 3.820596633 | Up |
RP11-520D19.2 | 3.5520305 | Up |
BC047481 | 3.4735432 | Up |
NCRNA00173 | 3.320569967 | Up |
CR936677 | 3.199325267 | Up |
LogFC, Log fold change.
Enrichment analysis and PPI network construction of the DEGs
The enrichment analysis of the DEGs showed that the DEGs were enriched in 363 kinds of biological processes (BPs), 21 kinds of cellular components (CCs), and 18 kinds of molecular functions (MFs) (see https://cdn.amegroups.cn/static/public/atm-22-3642-1.docx). The top 10 BP terms were urogenital system development, mesenchyme development, response to metal ion, mesenchymal cell differentiation, mesonephros development, kidney epithelium development, kidney development, semaphorin-plexin signaling pathway, ureteric bud development, and response to calcium ion (see Figure 2A). The top 10 CC terms were costamere, collagen-containing extracellular matrix, perikaryon, contractile fiber part, transcription factor complex, neuronal cell body, contractile fiber, axon part, distal axon, and Z disc (see Figure 2B). The top 10 MF terms were receptor ligand activity, chemorepellent activity, core promoter binding, core promoter sequence-specific DNA binding, semaphorin receptor binding, DNA-binding transcription activator activity, RNA polymerase II-specific, G protein-coupled receptor binding, cell adhesion molecule binding, collagen binding, and S100 protein binding (see Figure 2C).
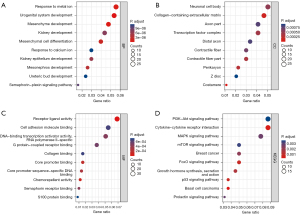
A total of 26 kinds of KEGG pathways were enriched (see Table S2), of which the most enriched were basal cell carcinoma, the cytokine-cytokine receptor interaction, the p53 signaling pathway, the FoxO signaling pathway, growth hormone synthesis, secretion and action, breast cancer, the mitogen-activated protein kinase (MAPK) signaling pathway, prolactin signaling pathway, mammalian target of rapamycin (mTOR) signaling pathway, and the phosphatidylinositol-3-kinase and protein kinase b (PI3K-Akt) signaling pathway (see Figure 2D).
In the PPI network of the DEGs (see Figure 3A), we screened out the top 10 hub genes based on the following measures of centrality: betweenness (see Figure 3B), closeness (see Figure 3C), and degree (see Figure 3D). By intersecting these hub genes, we identified 5 hub genes; that is, JUN, INS, STAT1, CHRM1, and WNT1 (see Figure 3E), whose expressions in our microarray profile are shown in Figure 3F.
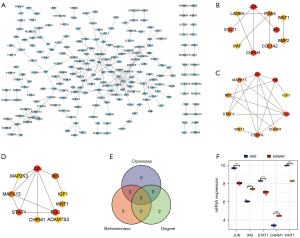
The roles of the top 10 DELs
To characterize the roles of the top 10 DELs (see Figure 4A), we screened out the DEGs co-expressed with the top 10 DELs and constructed a lncRNA-mRNA co-expression network (see Figure 4B). Next, the co-expressed DEGs were further used to construct a PPI (see Figure 5A), and the hub genes were screened out using the degree algorithm (see Figure 5B). We also performed an enrichment analysis of the co-expressed DEGs (see Figure S1). The results revealed that the mTOR signaling pathway was enriched. Given that NCRNA00173 is involved in the development of many tumors (17-21) and our previous study indicated that NCRNA00173 is also involved in the chemoresistance of SCLC (13), we specifically extracted the DEGs co-expressed with NCRNA00173 and found that they were also enriched in many cancer-related pathways, such as proteoglycans in cancer, the Wnt signaling pathway, the Hippo signaling pathway, and the mTOR signaling pathway (see Figure 5C,5D), which suggests that the mTOR signaling pathway might be a key pathway involved in SCLC development and chemoresistance. Additionally, we identified 4 pairs of differentially expressed lncRNA-mRNA neighbor relationships (see Table 3). AC009336.24 and BC047481 were predicted to cis-regulate NM_014621. RP11-513G11.1 was predicted to cis-regulate LRRC15. RP11-153F5.1 was predicted to cis-regulate KRT8.
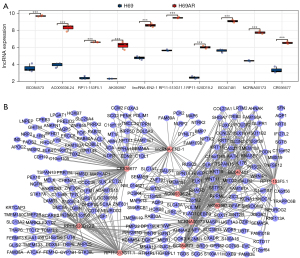
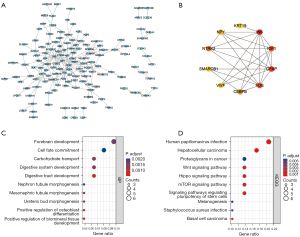
Table 3
DELs | Chr | Start | End | Strand | DEGs | Chr | Start | End | Strand | Up/down_stream | Distance (bp) |
---|---|---|---|---|---|---|---|---|---|---|---|
AC009336.24 | chr2 | 177042908 | 177043737 | + | NM_014621 | chr2 | 177017335 | 177017947 | + | UPSTREAM | 24,960 |
BC047481 | chr2 | 177037925 | 177042841 | – | NM_014621 | chr2 | 177017335 | 177017947 | + | UPSTREAM | 19,977 |
RP11-513G11.1 | chr3 | 194014253 | 194030493 | – | LRRC15 | chr3 | 194075976 | 194090472 | – | DOWNSTREAM | 45,482 |
RP11-153F5.1 | chr12 | 53279212 | 53280169 | + | KRT8 | chr12 | 53290970 | 53298868 | – | UPSTREAM | 10,800 |
DELs, differentially expressed lncRNAs; Chr, Chromosome; DEGs, differentially expressed genes.
Data set analysis of key genes
Given the importance of the mTOR signaling pathway, we focused on the co-expressed DEGs of the lncRNA-mRNA co-expression network enriched in the mTOR signaling pathway and identified IGF1/INS (among the hub genes in the top 10 DEL-co-expressed DEGs) and WNT6/WNT11/WNT2B/SESN2 (among the NCRNA00173-co-expressed DEGs). The expressions of these genes in our microarray analysis are shown in Figure 6A. Further, in the GEO database, we searched the SCLC data set GSE149507 (see Figure 6B) and noted the genes that were differently expressed (see Figure 6C). The paired t-test implied that the expression of NCRNA00173, INS, and WNT6 did not differ significantly between the 2 groups. However, IGF1, WNT2B, and SESN2 were under-expressed in the SCLC tumor tissues, while WNT11 was overexpressed in the tumor tissues (see Figure 6D-6J). We also compared the expression of the hub genes in the PPI analysis of the DEGs, and found that JUN and CHRM1 were downregulated in the tumor tissues, and STAT1 was upregulated in the tumor tissues (see Figure S2).
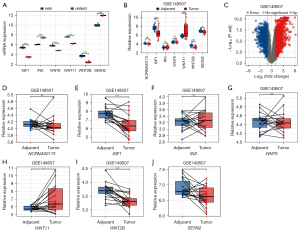
The results showed that IGF1, WNT2B, and JUN which were under-expressed in the SCLC chemoresistant cell line compared to the chemosensitive cell line, were also under-expressed in the SCLC tissues compared to the normal tissues. These results suggest that the low expression of IGF1, WNT2B, and JUN may not only be associated with chemoresistance but may also be related to the tumorigenesis of SCLC.
Validation of the key genes enriched in the mTOR signaling pathway
We further verified the expression of the key genes enriched in the mTOR signaling pathway. We performed qPCR on the H69 and H69AR cells. The results showed that IGF1, WNT6, WNT2B and WNT11 were downregulated, while INS, SESN2 and NCRNA00173 were upregulated in the H69AR cells (see Figure 7). These results were consistent with those of the microarray analysis, and thus confirmed the reliability of the expression profiling. The expression of the key genes was also validated in the other SCLC sensitive cell line H446 and the chemoresistant cell line H446DDP, the drug sensitivities of which were also analyzed by CCK8 assays (see Figure S3). Except for INS, the expression difference among the other genes was consistent with that of the H69 and H69AR cells.
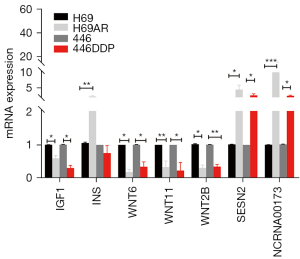
Discussion
As a neuroendocrine tumor, SCLC is characterized by aggressiveness, susceptibility to early hematologic and lymphatic metastasis, and high mortality (22), which is mainly attributed to the rapid development of patients’ resistance to chemotherapy. In this study, we performed a microarray analysis of H69 cells and H69AR cells to obtain the DEGs and DELs. The DEGs and DEGs co-expressed with the top 10 DELs were both enriched in the mTOR signaling pathway. Among the top 10 lncRNAs, NCRNA00173 has been reported in many tumors, and is involved in several cancer-related pathways, including the mTOR signaling pathway. Further, we found that in the enriched mTOR signaling pathway, IGF1 and INS were the hub genes in the top 10 DEL-co-expressed DEGs, and WNT6, WNT11, WNT2B, and SESN2 were the NCRNA00173-co-expressed DEGs. The key genes enriched in the mTOR signaling pathway were further analyzed using a data set from the GEO database and validated by 2 pairs of chemosensitive and chemoresistant SCLC cells by qPCR assays.
Through the KEGG enrichment analysis, we noted that the DEGs were enriched in various cancer-related pathways, including the PI3K-Akt signaling pathway, MAPK signaling pathway, mTOR signaling pathway, p53 signaling pathway, and FoxO signaling pathway. Additionally, some hub genes in the PPI network of the DEGs, such as JUN, INS, STAT1, CHRM1, and WNT1, were involved in the top 10 significant pathways. Among them, JUN was involved in the MAPK signaling pathway, INS in the FoxO/MAPK/mTOR/PI3K-Akt signaling pathways, STAT1 and CHRM1 in growth hormone synthesis, secretion, and action, and WNT1 in basal cell carcinoma and the mTOR signaling pathway. Recently, the activation of the MAPK signaling pathway (23) and the growth hormone-releasing hormone (24) were reported to be factors driving SCLC development. Further, the PI3K/Akt/mTOR pathway has been shown to play an important role in the progression and metastasis of SCLC (25). The activation of the PI3K/Akt/mTOR pathway contributes to the chemoresistance of SCLC cells; however, this chemoresistance could be largely overcome by combining chemotherapy with PI3K/Akt/mTOR pathway inhibitors (26). As a conserved serine/threonine protein kinase, mTOR is a key gene for upstream pathways that regulate cell growth, proliferation, motility, survival, and autophagy (27). Autophagy is a cellular degradation process conserved in biological evolution (28,29). Recent studies have shown that autophagy maintains cellular self-balance and provides energy and synthetic materials for cells to meet the metabolic needs of normal cells and even tumor cells and are essential in maintaining the balance of cellular bio-metabolism and the tumor microenvironment (30). Recently, the interaction between autophagy and drug resistance has become a topical issue (31,32).
As we know, mRNA expression profiles are regulated by lncRNAs at the epigenetic level. LncRNA, a general term for a class of RNA molecules >200 nucleotides, is one of the hottest frontier research areas in molecular biology today (33). Through the limma analysis, we identified the top 10 DELs. Among them, NCRNA00173 was found to be involved in the progression, of various tumors, such as lung squamous cell carcinoma (20), prostate cancer (21), glioma (34), and melanoma (35). In our previous study, we found that NCRNA00173 also contributes to the chemoresistance of colorectal cancer (19) and SCLC by acting as competing endogenous RNA (13). Tang et al. observed high levels of RP11-513G11.1 in the peripheral blood of diffuse large B-cell lymphoma patients and found that RP11-513G11.1 was correlated with a poor prognosis (36). To date, no research has examined the role of BC084573, AC009336.24, RP11-153F5.1, AK093987, lincRNA-EN2-1, RP11-520D19.2, BC047481, and CR936677 in cancers, which also need to be explored in relation to SCLC. As far as we know, this was the first study to conduct a microarray analysis to explore the roles of lncRNAs in the chemoresistance of SCLC.
LncRNA regulates adjacent or distant mRNAs in cis or trans ways (37). Our results revealed that 4 lncRNAs may cis-regulate adjacent mRNAs. Further, we predicted the trans-regulatory functions of the top 10 lncRNAs by constructing a lncRNA-mRNA co-expression network and performing enrichment analyses of all the co-expressed DEGs. We found that most of the co-expressed DEGs were enriched in the mTOR signaling pathway. To clarify the hub genes regulated by the top 10 DELs, a PPI analysis was performed, and we found that the hub genes of IGF1 and INS participate in the mTOR signaling pathway. Numerous reports have described the close association between these 2 genes and tumors (38-40). To further predict the functions of the top 10 lncRNAs and determine which lncRNA is most likely to regulate the mTOR signaling pathway, GO and KEGG analyses were performed on the co-expressed DEGs of each of the top 10 lncRNAs, and we found that the co-expressed DEGs of NCRNA00173 or RP11-153F5.1 were enriched in multiple tumor-related pathways, including the mTOR pathway.
Our results imply that these lncRNAs may promote SCLC chemoresistance by regulating the mTOR signaling pathway. We paid particular attention to NCRNA00173, as it has been reported to play important roles in several tumors (13,17-21). It has also been reported that lncRNAs modulate autophagy or drug resistance of SCLC; for example, the knockdown of HIF1A-AS2 increases the autophagy-related drug sensitivity of SCLC cells (41). Cancer-associated fibroblasts release exosomes capsuled with MEG3 to enhance CDDP chemoresistance in SCLC. Our previous studies suggest that KCNQ1OT1 inhibition induces apoptosis and drug sensitivity by inhibiting transforming growth factor beta 1 (42) or by activating the JAK2/STAT3 signaling pathway (43). TUG1 promotes the transcription of LIMK2b by binding to EZH2 to enhance cell growth and the chemoresistance of SCLC (14). HOTTIP (12) and NCRNA00173 (13) induce SCLC chemoresistance by promoting BCL-2 and Etk expression, respectively, by sponging microRNA. However, little is known about the relationship between NCRNA00173 and the mTOR signaling pathway and this needs to be further investigated in SCLC.
The expression of genes involved in the mTOR signaling pathway (i.e., NCRNA00173, IGF1, INS, WNT6, WNT11, WNT2B, and SESN2) and the hub genes of the DEGs PPI network (i.e., JUN, STAT1, CHRM1, and WNT1) were analyzed in the SCLC data set. We did not screen out chemoresistance-related data sets; however, we found that some of these genes differed in the normal and SCLC tissues in the GSE149507 data set. According to the results of the data set analysis, the low expression of IGF1, WNT2B, and JUN may be a biological characteristic of the occurrence and chemoresistance of SCLC. Finally, we verified the key genes enriched in the mTOR signaling pathway in vitro, and found that IGF1, WNT6, WNT11 and WNT2B were more lowly expressed, while SESN2, and NCRNA00173 were more highly expressed in the 2 chemoresistant cell lines than the chemosensitive cell lines; thus, NCRNA00173 may induce chemoresistance in SCLC by downregulating WNT6, WNT11, and WNT2B, or by upregulating SESN2, which means the NCRNA00173 and SESN2 inhibitors or the WNT6, WNT11, and WNT2B promoters would benefit for the treatment of SCLC. These genes might be used as chemoresistance biomarkers in SCLC, and further studies on their biological functions and mechanisms need to be conducted.
There are several limitations in our study that should be noted. Firstly, we analyzed lncRNA-mRNAs only indirectly using bioinformatics analysis and the key genes were verified by qPT-PCR in SCLC cell lines, further studies on the biological functions and mechanisms of the key genes need to be conducted in vitro and in vivo. Secondly, the DEGs and the DELs were screened out and lncRNA-mRNA co-expression network was also constructed in this study. Our research group had previously performed miRNA microarray (44) which could be combined with this study to construct the ceRNA network by integrating the relationships between lncRNAs and miRNAs, miRNAs and target genes, and the co-expression between differential mRNAs and lncRNAs (positive co-expression relationship) using the database such as TargetScan. The ceRNA analysis should be further performed. Thirdly, in this study , we conducted mRNA-lncRNA microarray analysis which is an important method to screen possible lncRNAs and mRNAs associated with specific diseases. Whole exome sequencing has high coverage and high accuracy. A recent study by Wagner et al. revealed that WNT pathway was activated in relapsed SCLC samples by whole-exome sequencing of paired SCLC tumor samples (45). The whole exome sequencing analysis should be further performed in the SCLC chemoresistant cell lines or clinical tissues to get more accurate information about SCLC chemoresistance.
Conclusions
Through a combination of bioinformatic analyses, we identified the top 10 DELs, including NCRNA00173, that may be involved in drug resistance in SCLC by regulating the genes related to the mTOR signaling pathway. Combining the results of microarray and data set analyses, we found that the low expression of IGF1, WNT2B, and JUN may not only indicate drug resistance, but may also indicate development of SCLC. Through qPCR assays of 2 pairs of sensitive and chemoresistant SCLC cell lines, we verified that IGF1, WNT6, WNT11, WNT2B, SESN2, and NCRNA00173 may serve as biomarkers of drug resistance in SCLC.
Acknowledgments
Funding: This work was supported by the National Natural Science Foundation of China (No. 81902311), the Natural Science Foundation of Guangdong Province (No. 2020A1515011454), the Project of the Featured Clinical Technique of Guangzhou (No. 2019TS28), and the Characteristic Innovation Project of Guangdong Universities (Natural Science) (No. 2021KTSCX093).
Footnote
Reporting Checklist: The authors have completed the MDAR reporting checklist. Available at https://atm.amegroups.com/article/view/10.21037/atm-22-3642/rc
Data Sharing Statement: Available at https://atm.amegroups.com/article/view/10.21037/atm-22-3642/dss
Conflicts of Interest: All authors have completed the ICMJE uniform disclosure form (available at https://atm.amegroups.com/article/view/10.21037/atm-22-3642/coif). All authors report that this work was supported by the National Natural Science Foundation of China (No. 81902311), the Natural Science Foundation of Guangdong Province (No. 2020A1515011454), the Project of the Featured Clinical Technique of Guangzhou (No. 2019TS28), and the Characteristic Innovation Project of Guangdong Universities (Natural Science) (No. 2021KTSCX093). The authors have no other conflicts of interest to declare.
Ethical Statement: The authors are accountable for all aspects of the work in ensuring that questions related to the accuracy or integrity of any part of the work are appropriately investigated and resolved. The study was conducted in accordance with the Declaration of Helsinki (as revised in 2013).
Open Access Statement: This is an Open Access article distributed in accordance with the Creative Commons Attribution-NonCommercial-NoDerivs 4.0 International License (CC BY-NC-ND 4.0), which permits the non-commercial replication and distribution of the article with the strict proviso that no changes or edits are made and the original work is properly cited (including links to both the formal publication through the relevant DOI and the license). See: https://creativecommons.org/licenses/by-nc-nd/4.0/.
References
- Sung H, Ferlay J, Siegel RL, et al. Global Cancer Statistics 2020: GLOBOCAN Estimates of Incidence and Mortality Worldwide for 36 Cancers in 185 Countries. CA Cancer J Clin 2021;71:209-49. [Crossref] [PubMed]
- Wang Z, Lu B, Sun L, et al. Identification of candidate genes or microRNAs associated with the lymph node metastasis of SCLC. Cancer Cell Int 2018;18:161. [Crossref] [PubMed]
- Koinis F, Kotsakis A, Georgoulias V. Small cell lung cancer (SCLC): no treatment advances in recent years. Transl Lung Cancer Res 2016;5:39-50. [PubMed]
- Mascaux C, Paesmans M, Berghmans T, et al. A systematic review of the role of etoposide and cisplatin in the chemotherapy of small cell lung cancer with methodology assessment and meta-analysis. Lung Cancer 2000;30:23-36. [Crossref] [PubMed]
- Waqar SN, Morgensztern D. Treatment advances in small cell lung cancer (SCLC). Pharmacol Ther 2017;180:16-23. [Crossref] [PubMed]
- Kumar MM, Goyal R. LncRNA as a Therapeutic Target for Angiogenesis. Curr Top Med Chem 2017;17:1750-7. [Crossref] [PubMed]
- Hu X, Sood AK, Dang CV, et al. The role of long noncoding RNAs in cancer: the dark matter matters. Curr Opin Genet Dev 2018;48:8-15. [Crossref] [PubMed]
- Yin X, Yang J, Wang H, et al. Non-coding genome in small cell lung cancer between theoretical view and clinical applications. Semin Cancer Biol 2022. [Epub ahead of print]. pii: S1044-579X(22)00080-3. doi:
10.1016/j.semcancer.2022.03.024 .10.1016/j.semcancer.2022.03.024 - Kumar S, Pandey M, Sharawat SK. Biological functions of long noncoding RNAs and circular RNAs in small-cell lung cancer. Epigenomics 2020;12:1751-63. [Crossref] [PubMed]
- Luo H, Zhang Y, Qin G, et al. LncRNA MCM3AP-AS1 sponges miR-148a to enhance cell invasion and migration in small cell lung cancer. BMC Cancer 2021;21:820. [Crossref] [PubMed]
- Yan Z, Yang Q, Xue M, et al. YY1-induced lncRNA ZFPM2-AS1 facilitates cell proliferation and invasion in small cell lung cancer via upregulating of TRAF4. Cancer Cell Int 2020;20:108. [Crossref] [PubMed]
- Sun Y, Hu B, Wang Q, et al. Long non-coding RNA HOTTIP promotes BCL-2 expression and induces chemoresistance in small cell lung cancer by sponging miR-216a. Cell Death Dis 2018;9:85. [Crossref] [PubMed]
- Zeng F, Wang Q, Wang S, et al. Linc00173 promotes chemoresistance and progression of small cell lung cancer by sponging miR-218 to regulate Etk expression. Oncogene 2020;39:293-307. [Crossref] [PubMed]
- Niu Y, Ma F, Huang W, et al. Long non-coding RNA TUG1 is involved in cell growth and chemoresistance of small cell lung cancer by regulating LIMK2b via EZH2. Mol Cancer 2017;16:5. [Crossref] [PubMed]
- Ritchie ME, Phipson B, Wu D, et al. limma powers differential expression analyses for RNA-sequencing and microarray studies. Nucleic Acids Res 2015;43:e47. [Crossref] [PubMed]
- Yu G, Wang LG, Han Y, et al. clusterProfiler: an R package for comparing biological themes among gene clusters. OMICS 2012;16:284-7. [Crossref] [PubMed]
- Yang Q, Kong S, Zheng M, et al. Long intergenic noncoding RNA LINC00173 as a potential serum biomarker for diagnosis of non-small-cell lung cancer. Cancer Biomark 2020;29:441-51. [Crossref] [PubMed]
- Fan H, Yuan J, Li X, et al. LncRNA LINC00173 enhances triple-negative breast cancer progression by suppressing miR-490-3p expression. Biomed Pharmacother 2020;125:109987. [Crossref] [PubMed]
- Yu Y, Lu X, Yang C, et al. Long Noncoding RNA LINC00173 Contributes to the Growth, Invasiveness and Chemo-Resistance of Colorectal Cancer Through Regulating miR-765/PLP2 Axis. Cancer Manag Res 2020;12:3363-9. [Crossref] [PubMed]
- Chen J, Liu A, Wang Z, et al. LINC00173.v1 promotes angiogenesis and progression of lung squamous cell carcinoma by sponging miR-511-5p to regulate VEGFA expression. Mol Cancer 2020;19:98. [Crossref] [PubMed]
- Hu CH, Yang XJ, Yu L, et al. Long non-coding RNA LINC00173 serves as sponge for miR-338-3p to promote prostate cancer progression via regulating Rab25. Eur Rev Med Pharmacol Sci 2020;24:9290-302. [PubMed]
- Xie D, Marks R, Zhang M, et al. Nomograms Predict Overall Survival for Patients with Small-Cell Lung Cancer Incorporating Pretreatment Peripheral Blood Markers. J Thorac Oncol 2015;10:1213-20. [Crossref] [PubMed]
- Caeser R, Hulton C, Costa E, et al. MAPK pathway activation selectively inhibits ASCL1-driven small cell lung cancer. iScience 2021;24:103224. [Crossref] [PubMed]
- Wang H, Zhang X, Vidaurre I, et al. Inhibition of experimental small-cell and non-small-cell lung cancers by novel antagonists of growth hormone-releasing hormone. Int J Cancer 2018;142:2394-404. [Crossref] [PubMed]
- Krencz I, Sztankovics D, Danko T, et al. Progression and metastasis of small cell lung carcinoma: the role of the PI3K/Akt/mTOR pathway and metabolic alterations. Cancer Metastasis Rev 2021;40:1141-57. [Crossref] [PubMed]
- Li X, Li C, Guo C, et al. PI3K/Akt/mTOR signaling orchestrates the phenotypic transition and chemo-resistance of small cell lung cancer. J Genet Genomics 2021;48:640-51. [Crossref] [PubMed]
- Wang Y, Zhang H. Regulation of Autophagy by mTOR Signaling Pathway. Adv Exp Med Biol 2019;1206:67-83. [Crossref] [PubMed]
- Levine B, Kroemer G. Biological Functions of Autophagy Genes: A Disease Perspective. Cell 2019;176:11-42. [Crossref] [PubMed]
- Levy JMM, Towers CG, Thorburn A. Targeting autophagy in cancer. Nat Rev Cancer 2017;17:528-42. [Crossref] [PubMed]
- Kenific CM, Debnath J. Cellular and metabolic functions for autophagy in cancer cells. Trends Cell Biol 2015;25:37-45. [Crossref] [PubMed]
- Chen C, Lu L, Yan S, et al. Autophagy and doxorubicin resistance in cancer. Anticancer Drugs 2018;29:1-9. [Crossref] [PubMed]
- Huang Y, Lin Y, Song X, et al. LINC00857 contributes to proliferation and lymphomagenesis by regulating miR-370-3p/CBX3 axis in diffuse large B-cell lymphoma. Carcinogenesis 2021;42:733-41. [Crossref] [PubMed]
- Peng WX, Koirala P, Mo YY. LncRNA-mediated regulation of cell signaling in cancer. Oncogene 2017;36:5661-7. [Crossref] [PubMed]
- Du Q, Liu J, Tian D, et al. Long Noncoding RNA LINC00173 Promotes NUTF2 Expression Through Sponging miR-765 and Facilitates Tumorigenesis in Glioma. Cancer Manag Res 2020;12:7211-7. [Crossref] [PubMed]
- Yang F, Lei P, Zeng W, et al. Long Noncoding RNA LINC00173 Promotes the Malignancy of Melanoma by Promoting the Expression of IRS4 Through Competitive Binding to microRNA-493. Cancer Manag Res 2020;12:3131-44. [Crossref] [PubMed]
- Tang JL, Li XM, Zhang L. Expression and Significance of LncRNA RP11-513G11.1 in Peripheral Blood of Patients with Diffuse Large B-Cell Lymphoma. Zhongguo Shi Yan Xue Ye Xue Za Zhi 2019;27:1515-21. [PubMed]
- Huarte M. The emerging role of lncRNAs in cancer. Nat Med 2015;21:1253-61. [Crossref] [PubMed]
- Liu L, Li X. A Review of IGF1 Signaling and IGF1-related Long Noncoding RNAs in Chemoresistance of Cancer. Curr Cancer Drug Targets 2020;20:325-34. [Crossref] [PubMed]
- Solarek W, Koper M, Lewicki S, et al. Insulin and insulin-like growth factors act as renal cell cancer intratumoral regulators. J Cell Commun Signal 2019;13:381-94. [Crossref] [PubMed]
- Werner H, Laron Z. Role of the GH-IGF1 system in progression of cancer. Mol Cell Endocrinol 2020;518:111003. [Crossref] [PubMed]
- Güçlü E, Eroğlu Güneş C, Kurar E, et al. Knockdown of lncRNA HIF1A-AS2 increases drug sensitivity of SCLC cells in association with autophagy. Med Oncol 2021;38:113. [Crossref] [PubMed]
- Li D, Tong Q, Lian Y, et al. Inhibition of lncRNA KCNQ1OT1 Improves Apoptosis and Chemotherapy Drug Response in Small Cell Lung Cancer by TGF-β1 Mediated Epithelial-to-Mesenchymal Transition. Cancer Res Treat 2021;53:1042-56. [Crossref] [PubMed]
- Zhu Y, Shen Y, Chen R, et al. KCNQ1OT1 lncRNA affects the proliferation, apoptosis, and chemoresistance of small cell lung cancer cells via the JAK2/STAT3 axis. Ann Transl Med 2021;9:891. [Crossref] [PubMed]
- Guo L, Liu Y, Bai Y, et al. Gene expression profiling of drug-resistant small cell lung cancer cells by combining microRNA and cDNA expression analysis. Eur J Cancer 2010;46:1692-702. [Crossref] [PubMed]
- Wagner AH, Devarakonda S, Skidmore ZL, et al. Recurrent WNT pathway alterations are frequent in relapsed small cell lung cancer. Nat Commun 2018;9:3787. [Crossref] [PubMed]