Screening of key pathogenic genes in advanced knee osteoarthritis based on bioinformatics analysis
Introduction
Knee osteoarthritis (KOA) is a degenerative disease (1). Middle-aged and elderly individuals are prone to KOA, which is characterized by progressive degeneration of articular cartilage and may be accompanied by subchondral bone sclerosis and hyperostosis (2-4). Age, sex, obesity, heredity, trauma, and joint deformity are pathogenic factors of KOA (5,6). These factors also interact with each other to jointly increase the risk of disease. For most patients, the knee joint function gradually decreases with KOA progression and they eventually undergo joint replacement (7). Long-term pain, high treatment costs, and knee joint dysfunction seriously affect the quality of life of patients (8).
A variety of inflammatory factors and inflammatory response-related pathways participate in the pathogenesis of KOA. Inflammatory reactions may degrade the cartilage extracellular matrix and reduce the number of chondrocytes. However, the progression mechanism of KOA has not yet been fully clarified. Previous studies have not identified the key pathogenic genes of KOA and the inflammatory response trigger point. The main purpose of early KOA treatment is to relieve pain and delay disease progression. Patients with advanced KOA require surgery. However, clinical staging of knee osteoarthritis is mainly based on clinical symptoms and imaging diagnosis, lacking biological markers. There are few related studies in the past. A study (9) screened the differentially expressed genes of early KOA and late KOA, but did not identify the key genes.
With the development of molecular biology technology, whole transcriptome sequencing technology has been applied to explore the pathogenesis of KOA. Susceptibility genes play a decisive role in the occurrence and progression of KOA. The screening of differential genes may provide a reference and basis for early diagnosis and treatment. The present study aims to analyze the sequencing results of early- and late-stage KOA synovial tissue and screen the key genes of late-stage KOA in combination with a machine learning algorithm. We present the following article in accordance with the STARD reporting checklist (available at https://atm.amegroups.com/article/view/10.21037/atm-22-3863/rc).
Methods
Data download
The sequencing results of the whole transcriptome of synovial tissue from KOA patients (GSE176223 and GSE32317) were downloaded from the gene expression omnibus (GEO) database. According to the Kellgren-Lawrence classification, grades 1 to 2 are early, and grades 3 to 4 are late. A total of 39 early KOA synovial tissue samples and 31 late KOA synovial tissue samples were included. The two data sets were combined into a data matrix. Furthermore, logarithmic transformation and batch correction were applied to eliminate the influence of the experimental background on the data. The diagnostic criteria and baseline data balance of early and late KOA were referred to the data source literature (9,10), and the two groups of data had good baseline data balance. The study was conducted in accordance with the Declaration of Helsinki (as revised in 2013).
Differential analysis
R software (V3.5.1) and R package (MathSoft, USA) were used to screen differentially expressed genes (DEGs) in early and late KOA synovial tissue samples. The screening criteria were set as: fold change (FC) > two times and P<0.05.
Enrichment analysis
The R software and R package were used to perform enrichment analysis in the Kyoto Encyclopedia of Genes and Genomes (KEGG) and Gene Ontology (GO) for the DEGs. This study selected the GO project and the KEGG pathway with P<0.05. Gene set enrichment analysis (GESA) was performed on DEGs, and the screening standard was set as P<0.05.
Construction of a protein interaction network
A differential gene interaction network was constructed using the String database. A hub gene interaction network was constructed using Cytoscape software and cytoHubba plug-in (https://cytoscape.org/).
Key gene screening
A penalty model was built through least absolute shrinkage and selection operator (LASSO) regression analysis to screen the early and late KOA key genes. When the binomial deviance was smallest, the minimum variable number gene was selected to characterize the overall sample characteristics. Weighted correlation network analysis (WGCNA) was applied to screen the early and late KOA co-expression genes. The gene module with |cor| >0.5 and P<0.01 was kept.
Diagnostic efficacy evaluation
The diagnostic efficacy of key genes for advanced KOA was evaluated using a receiver operating characteristic curve (ROC) curve. For areas under curve (AUC) >0.6, P<0.8 was considered to indicate that it has a good diagnostic model. Moreover, AUC values closer to 1 were considered to represent better gene diagnostic efficiency.
Statistical analysis
In this study, R software (v3.5.1) and related R packages were used for statistical analysis. A two-way P<0.05 was considered statistically significant.
Results
DEGs screening
This study screened 211 DEGs related to knee arthritis based on |log2FC| ≥2 and P<0.05. As shown in Figure 1, compared with the synovial tissue of early knee arthritis, 111 genes were upregulated and 100 genes were downregulated in the synovial tissue of late knee arthritis. Based on the knee arthritis DEGs, we constructed a hub gene interaction network, which is displayed in Figure 2.
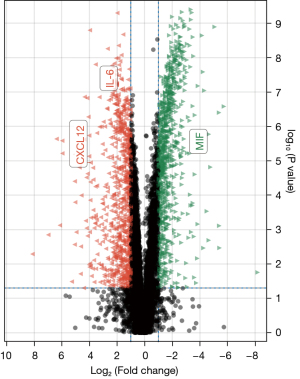
DEG enrichment analysis
GO enrichment analysis demonstrated that DEGs were significantly enriched in early and late knee arthritis in the following biological process (BP): positive regulation of the response to external stimulus, cell chemotaxis, leukocyte-mediated immunity, cellular response to lipopolysaccharide, and regulation of leukocyte chemotaxis. These items were related to the inflammatory response, as shown in Figure 3.
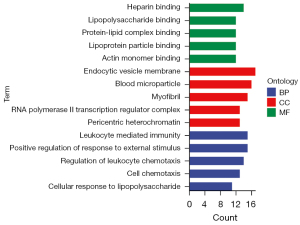
KEGG enrichment analysis indicated that DEGs were significantly enriched in pathways related to inflammatory response, including the interleukin 17 (IL-17) signaling pathway, antigen processing and presentation, the Toll-like receptor signaling pathway, the tumor necrosis factor (TNF) signaling pathway, viral protein interaction with cytokine and cytokine receptors, the PI3K-AKT signaling pathway, cytokine-cytokine receptor interaction, etc. (Figure 4).
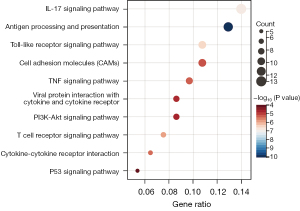
GSEA enrichment analysis showed that the DEGs were enriched in the chemicals of intelligence factors and immune response activation, as shown in Figure 5.
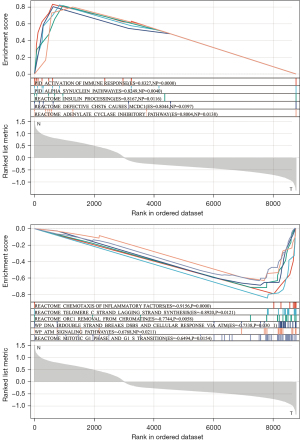
Key gene screening
Through WGCNA, we screened three co-expressive gene modules related to early and late knee arthritis with |Cor| >0.5 and P<0.01 as the screening criteria. As shown in Figures 6,7, these three modules contained 66 genes. LASSO analysis showed that when the binomial deviance was smallest, 34 key genes were screened (Figure 8). The genes obtained by the two algorithms were combined with three overlapping genes, including interleukin 6 (IL-6), chemokine (C-X-C motif) ligand 12 (CXCL12), and macrophage migration inventory factor (MIF), as shown in Figure 9.
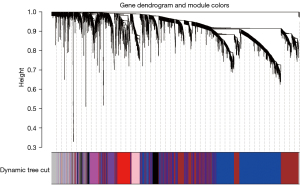
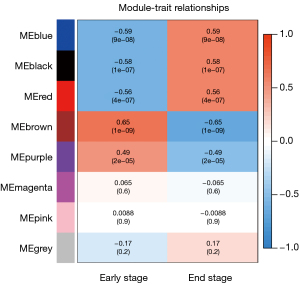
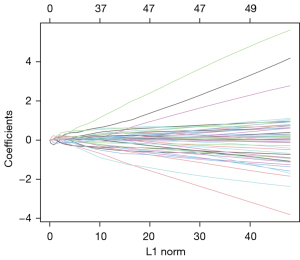
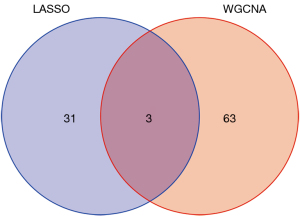
Evaluation of diagnostic efficiency
The areas under the ROC curves of CXCL12, IL-6, and MIF were 0.96, 0.944, and 0.961, respectively (P<0.001). CXCL12, IL-6, and MIF exhibited good diagnostic efficacy for advanced KOA, as shown in Figures 10-12.
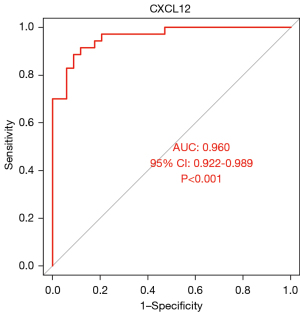
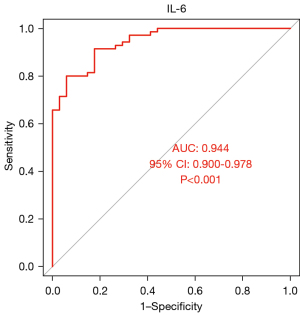
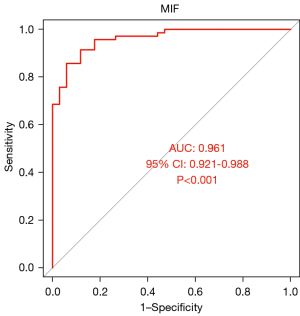
Discussion
In recent years, some studies have screened the key miRNAs and lncRNAs in the pathogenesis of KOA (11,12). In KOA chondrocytes, lncRNA interacts with miRNA to participate in the regulation of chondrocyte synthesis and catabolism. At the same time, lncRNAs and miRNAs also play an important role in the development of bone and cartilage. However, the number of identified functional lncRNAs and miRNAs is small, and the functional research on lncRNA and miRNA remains limited. The present study focused on screening the key pathogenic mRNAs and explored gene function through enrichment analysis.
We screened 211 DEGs by comparing the whole transcriptome sequencing results of early and late KOA synovial tissues. These genes were significantly enriched in the KEGG pathway of inflammatory response and the GO project. GSEA showed that the DEGs were associated with inflammatory chemokines and immune system activation. These results demonstrated that the screened differential genes were representative. Furthermore, we also screened three key genes (IL-6, CXCL12, and MIF) using two machine learning algorithms. IL-6 and CXCL12 were highly expressed in the late KOA synovium, while MIF was downregulated in the late KOA synovium. CXCL12, IL-6, and MIF showed good diagnostic efficacy for advanced KOA.
Some studies have explored the key pathogenic genes of KOA using bioinformatics analysis. Song et al. (11) suggested that susceptibility genes play a crucial regulatory role in the occurrence and development of osteoarthritis, which might impact the following cell functions and structural pathological changes: including chondrocyte function, cartilage matrix metabolism, synovial hyperplasia, neovascularization, etc. Compared with normal chondrocytes, the GAS5 gene was highly expressed in KOA chondrocytes. The GAS5 gene might participate in and regulate matrix metalloproteinases and affect soft cell secretion, apoptosis, and autophagy. Le et al. (12) found that MEG3 and VEGF were upregulated in KOA articular cartilage compared to normal cartilage. They suggested that downregulation of MEG3 expression could delay disease progression and relieve pain in patients.
Other studies have described the role of IL-6 in KOA. For instance, Ge et al. (13) found that kallikrein-related peptidase 6 (KLK6) was mutated in the blood samples of KOA patients through full exon sequencing. KLK6 is associated with the inflammatory response in various malignant diseases and may mediate extracellular matrix degradation. They also pointed out that KLK6 was closely related to IL-6, both of which are key pathogenic factors of KOA. Also, Wang et al. (14) showed that reducing the level of IL-6 in serum could inhibit inflammatory and oxidative stress responses in a constructed rat KOA model. Moreover, Yan et al. (15) showed that high fibular osteotomy could reduce the serum IL-6 level in a KOA rabbit model.
Researchers also found that lowering serum levels of inflammatory factors such as IL-6 could protect medial ventricular KOA. Singh et al. (16) showed that IL-6 was an independent risk factor for KOA. The AUC of IL-6 was 0.8, indicating that it could be used for disease prediction. In addition, researchers further constructed a KOA prediction model by combining IL-6, IL-1β, and clinically susceptible factors, including body mass index (BMI), triglyceride level, and poor sleep. These results are consistent with our findings. CXCL12 is a classical pro-inflammatory cytokine that can induce cells of the immune system to enter the inflammatory response site during an immune response (17-19). Our analysis demonstrated that CXCL12 mediated inflammatory homing in KOA. However, there is currently no literature confirming this. MIF can inhibit macrophage migration (20-23) and might be a protective factor of KOA, although this requires further verification.
In conclusion, IL-6, CXCL12, and MIF are key pathogenic genes of KOA and have good diagnostic efficacy for advanced KOA.
Acknowledgments
Funding: The study was supported by the Open Fund of Key Laboratory of Theory and Application of Viscera and Viscera in Traditional Chinese Medicine, Ministry of Education, Liaoning University of Traditional Chinese Medicine (No. zyzx1907); the Natural Science Foundation of Liaoning Province (No. 2018055083); Program of Natural Science Foundation of Liaoning Province (No. 2022-MS-286); and the Platform construction project of the Liaoning Academy of Traditional Chinese Medicine Sciences (No. 2019JH6/10100002).
Footnote
Reporting Checklist: The authors have completed the STARD reporting checklist. Available at https://atm.amegroups.com/article/view/10.21037/atm-22-3863/rc
Conflicts of Interest: All authors have completed the ICMJE uniform disclosure form (available at https://atm.amegroups.com/article/view/10.21037/atm-22-3863/coif). The authors have no conflicts of interest to declare.
Ethical Statement: The authors are accountable for all aspects of the work in ensuring that questions related to the accuracy or integrity of any part of the work are appropriately investigated and resolved. The study was conducted in accordance with the Declaration of Helsinki (as revised in 2013).
Open Access Statement: This is an Open Access article distributed in accordance with the Creative Commons Attribution-NonCommercial-NoDerivs 4.0 International License (CC BY-NC-ND 4.0), which permits the non-commercial replication and distribution of the article with the strict proviso that no changes or edits are made and the original work is properly cited (including links to both the formal publication through the relevant DOI and the license). See: https://creativecommons.org/licenses/by-nc-nd/4.0/.
References
- Goff AJ, Elkins MR. Knee osteoarthritis. J Physiother 2021;67:240-1. [Crossref] [PubMed]
- Lin KW. Treatment of Knee Osteoarthritis. Am Fam Physician 2018;98:603-6. [PubMed]
- Dantas LO, Salvini TF, McAlindon TE. Knee osteoarthritis: key treatments and implications for physical therapy. Braz J Phys Ther 2021;25:135-46. [Crossref] [PubMed]
- Nazari G. Knee osteoarthritis. J Physiother 2017;63:188. [Crossref] [PubMed]
- Hussain SM, Neilly DW, Baliga S, et al. Knee osteoarthritis: a review of management options. Scott Med J 2016;61:7-16. [Crossref] [PubMed]
- Landsmeer MLA, Runhaar J, van Middelkoop M, et al. Predicting Knee Pain and Knee Osteoarthritis Among Overweight Women. J Am Board Fam Med 2019;32:575-84. [Crossref] [PubMed]
- Imani F, Patel VB. Therapeutic Challenges for Knee Osteoarthritis. Anesth Pain Med 2019;9:e95377. [PubMed]
- Carlesso LC, Neogi T. Identifying pain susceptibility phenotypes in knee osteoarthritis. Clin Exp Rheumatol 2019;37:96-9. [PubMed]
- Nanus DE, Badoume A, Wijesinghe SN, et al. Synovial tissue from sites of joint pain in knee osteoarthritis patients exhibits a differential phenotype with distinct fibroblast subsets. EBioMedicine 2021;72:103618. [Crossref] [PubMed]
- Wang Q, Rozelle AL, Lepus CM, et al. Identification of a central role for complement in osteoarthritis. Nat Med 2011;17:1674-9. [Crossref] [PubMed]
- Song J, Ahn C, Chun CH, et al. A long non-coding RNA, GAS5, plays a critical role in the regulation of miR-21 during osteoarthritis. J Orthop Res 2014;32:1628-35. [Crossref] [PubMed]
- Le LT, Swingler TE, Crowe N, et al. The microRNA-29 family in cartilage homeostasis and osteoarthritis. J Mol Med (Berl) 2016;94:583-96. [Crossref] [PubMed]
- Ge Y, Zhou C, Xiao X, et al. A Novel Mutation of the KLK6 Gene in a Family With Knee Osteoarthritis. Front Genet 2021;12:784176. [Crossref] [PubMed]
- Wang S, Ding P, Xia X, et al. Bugan Rongjin decoction alleviates inflammation and oxidative stress to treat the postmenopausal knee osteoarthritis through Wnt signaling pathway. Biomed Eng Online 2021;20:103. [Crossref] [PubMed]
- Yan F, Zhao X, Duan S, et al. High fibular osteotomy ameliorates medial compartment knee osteoarthritis in a rabbit model. J Biomech 2021;128:110734. [Crossref] [PubMed]
- Singh M, Valecha S, Khinda R, et al. Multifactorial Landscape Parses to Reveal a Predictive Model for Knee Osteoarthritis. Int J Environ Res Public Health 2021;18:5933. [Crossref] [PubMed]
- Portella L, Bello AM, Scala S. CXCL12 Signaling in the Tumor Microenvironment. Adv Exp Med Biol 2021;1302:51-70. [Crossref] [PubMed]
- Kurowarabe K, Endo M, Kobayashi D, et al. CXCL12-stimulated lymphocytes produce secondary stimulants that affect the surrounding cell chemotaxis. Biochem Biophys Rep 2021;28:101128. [Crossref] [PubMed]
- Mousavi A. CXCL12/CXCR4 signal transduction in diseases and its molecular approaches in targeted-therapy. Immunol Lett 2020;217:91-115. [Crossref] [PubMed]
- Jankauskas SS, Wong DWL, Bucala R, et al. Evolving complexity of MIF signaling. Cell Signal 2019;57:76-88. [Crossref] [PubMed]
- Noe JT, Mitchell RA. MIF-Dependent Control of Tumor Immunity. Front Immunol 2020;11:609948. [Crossref] [PubMed]
- Kang I, Bucala R. The immunobiology of MIF: function, genetics and prospects for precision medicine. Nat Rev Rheumatol 2019;15:427-37. [Crossref] [PubMed]
- Yang J, Yuan Z, Ma J, et al. MIF gene polymorphism in cerebral infarction. Panminerva Med 2021; Epub ahead of print. [Crossref] [PubMed]
(English Language Editor: A. Kassem)