Identification and verification of a glycolysis-related gene signature for gastric cancer
Introduction
Gastric cancer (GC) is a very common disease, has the 2nd highest cancer-associated mortality rate and represents a serious threat to human health worldwide (1). GC is divided into many subtypes, including squamous cell carcinoma, adenocarcinoma, carcinoid, and adenosquamous carcinoma. Among them, gastric adenocarcinoma is the most common histological type of GC. Numerous treatment methods, including surgery, adjuvant chemotherapy and chemoradiation, may significantly improve the survival rate of GC patients however, the 5-year survival rate of GC patients remains unsatisfactory (2,3). The prognosis of GC patients is poor, as GC patients are often diagnosed at an advanced stage and effective treatments are limited. It has been reported that tissue type, biological behavior, pathological stage, location, and treatment are closely related to the prognosis of GC patients (4). An increasing number of potential biomarkers related to prognosis and survival of GC have been developed. However, there is still a lack of accurate prediction models and a single biomarker hardly achieves a good prediction effect for GC. Thus, effective models for predicting the prognosis and guiding the treatment of GC patients in clinical practice urgently need to be developed.
There is increasing evidence that metabolic reprogramming is a common hallmark of cancer cells, and plays an important role in the proliferation, invasion, and angiogenesis of cancer cells (5-7). Aerobic glycolysis, also known as the Warburg effect, is one of the most common metabolic reprogramming methods. Previous studies have shown that inhibiting aerobic glycolysis might effectively inhibit the growth and induce the apoptosis of cancer cells (8-10). A gene expression signature consisting with several genetic markers might improve the specificity and sensitivity of prediction for GC. Some studies using data from public databases have also shown that glycolysis-related genes can predict the prognosis of cancer patients, including those with clear cell renal cell carcinoma (11), lung adenocarcinoma (12), hepatocellular carcinoma (13), breast cancer (14,15), ovarian cancer (16), and colorectal cancer (17). Additionally, recent research has shown that glycolysis-related genes might be used to effectively assess the prognosis of GC patients (18,19). However, systematic studies on the relationship between glycolysis-related genes and the prognosis of GC patients are still lacking.
Thus, in this study, we analyzed the relationship between glycolysis-related genes and the prognosis of GC patients, and then established a novel 4 glycolysis-related gene signature to assess the prognosis of GC patients. Our results provide novel insights into how to predict the prognosis of GC patients. We present the following article in accordance with the TRIPOD reporting checklist (available at https://atm.amegroups.com/article/view/10.21037/atm-22-3980/rc).
Methods
Flowchart of study design
The study design is illustrated in Figure 1.
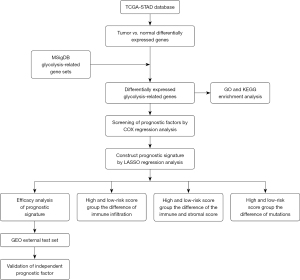
Tumor and clinical data collection
The clinical data and messenger ribonucleic acid (mRNA) expression profiles of GC were downloaded from The Cancer Genome Atlas (TCGA) database (https://xena.ucsc.edu/). In total, 350 GC samples and 31 normal control samples were obtained from TCGA database. The somatic mutation data of the GC samples were also downloaded from TCGA database. Gene Expression Omnibus (GEO) cohorts were used for the external validations. A total of 433 GC patient samples were retrieved and analyzed from the GEO (https://www.ncbi.nlm.nih.gov/geo) database (GSE84437). The GES84437 cohort obtained from the GEO database was analyzed using the GPL6947 platform. The probe was matched to the genes. If multiple probes were matched to the same gene, the highest expression level of the gene was annotated as the expression level of the gene. The clinicopathological characteristics of the GC patients from The Cancer Genome Atlas Stomach Adenocarcinoma (TCGA-STAD) cohort and the GSE84437 data set are set out in Table 1. The study was conducted in accordance with the Declaration of Helsinki (as revised in 2013).
Table 1
Characteristics | TCGA-STAD | GSE84437 |
---|---|---|
Number of samples | 350 | 433 |
Median survival time (days) | 475 | 2,040 |
Number of deaths, n (%) | 146 (41.71) | 209 (48.27) |
Average age (years) | 65.25 | 60.06 |
Gender, n (%) | ||
Male | 226 (64.57) | 296 (68.36) |
Female | 124 (35.43) | 137 (39.14) |
FIGO stage, n (%) | ||
I | 46 (13.14) | NA |
II | 110 (31.43) | NA |
III | 145 (41.43) | NA |
IV | 35 (10.00) | NA |
NA | 14 (4.00) | NA |
Grade, n (%) | ||
1 | 9 (2.57) | NA |
2 | 125 (35.71) | NA |
3 | 207 (59.14) | NA |
NA | 9 (2.57) | NA |
TCGA, The Cancer Genome Atlas; STAD, Stomach Adenocarcinoma; FIGO, International Federation of Gynecology and Obstetrics; NA, not applicable.
Identification of DEGs
R language (version 3.6.1) from the edge R package (20) was used to compare the differential expression profiles of the mRNAs in the GC and normal groups. Genes with a false discovery rate (FDR) <0.05 and a |log2 fold change (log2 FC)| >1 were identified as the differentially expressed genes (DEGs) (20).
Enrichment analysis of glycolysis-related genes
We applied Molecular Signatures Database (MSigDB) (http://software.broadinstitute.org/gsea/msigdb, version 7.1) to analyze the association of the DEGs between the GC samples and normal control samples and identify the glycolysis-related DEGs. Next, the glycolysis-related DEGs were analyzed using the Kyoto Encyclopedia of Genes and Genomes (KEGG) signaling pathways and Gene Ontology (GO) through the R language “clusterProfiler” package (21). An FDR value <0.05 indicated significant enrichment.
Differential expression analysis
Construction and validation of the prognostic model of GC
The samples obtained from TCGA were used as the training set to construct the model. A univariate Cox regression analysis was performed to screen the glycolysis-related DEGs whose expression levels were closely related to the overall survival (OS) of the GC patients using the “survival” R package. Subsequently, we further used least absolute shrinkage and selection operator (LASSO) regression to identify glycolysis-related genes for the prognostic signature through the R package “glmnet” (22,23) according to the results of the univariate Cox regression analysis (P<0.05). Based on the results of the LASSO regression analysis, a prognostic risk-score model was constructed. Finally, the risk scores of 350 GC samples obtained from TCGA were calculated according to the model. The GC patients in the training and testing sets were divided into the high- and low-risk groups based on the median risk score. The survival rates between the 2 groups were compared using the log-rank test.
Immune cell infiltration analysis
In this study, the differences between the high- and low-risk groups in terms of the mutation, immune cell infiltration, immune score, and stromal score for the 22 types of immune cells in the GC sample were performed using the R language (version 3.6.1). The mutations of the 22 immune cells in the GC sample were assessed using the “maftools” R package. The infiltration levels of the 22 immune cells in the GC sample were assessed based on CIBERSORT (http://cibersort.stanford.edu/) (24). The immune and stromal scores of the 22 immune cells in the GC sample were assessed using the “estimate” R package.
Statistical analysis
Univariate and multivariate Cox analysis were performed by using the “survival” R package. LASSO analysis was performed using the R package “glmnet”. The immune and stromal scores in the GC sample were assessed using the “estimate” R package. P value <0.05 was considered statistically significant.
Results
Identification analysis of DEGs in TCGA
The differential expression profiles of the GC and normal samples from TCGA were analyzed. Among the 381 samples of TCGA, 350 were GC samples and 31 were normal control samples. The criteria for the DEGs were an FDR value <0.05 and a |log2 FC| value >1. As Figure 2A shows, a total of 3,058 DEGs were identified, of which 1,304 were upregulated and 1,754 were downregulated. The top 100 DEGs genes were selected and a heatmap was drawn according to the |log2 FC| values (see Figure 2B).
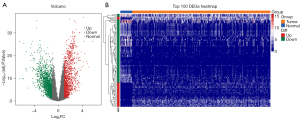
Enrichment analysis of glycolysis-related DEGs
We analyzed the glycolysis-related genes in GC using the MSigDB, and the MSigDB gene sets of 290 glycolysis-related genes were then acquired (see Appendix 1). We combined 3,058 DEGs and 290 glycolysis-related genes to verify the glycolysis-related genes that differed significantly between the GC and normal control samples. As Figure 3 shows, we identified a total of 55 glycolysis-related genes that differed significantly between the GC and normal control samples. To reveal the function of the glycolysis-related DEGs, GO and KEGG analyses, including analyses of the biological processes (BPs), molecular functions (MFs), and cellular components (CCs), were performed on the 55 glycolysis-related DEGs. The 55 glycolysis-related DEGs were significantly enriched in the following BPs and pathways: purine nucleoside monophosphate metabolism, carbohydrate catabolism, purine nucleoside monophosphate biosynthesis, adenosine diphosphate (ADP) metabolism, glucose metabolism, nucleotide phosphorylation, and gluconeogenesis (see Figure 4). Interestingly, none of the 55 glycolysis-related DEGs were enriched in terms of the CCs (see Figure 4).
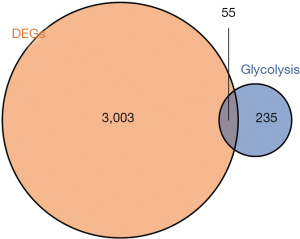
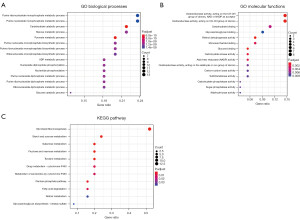
Construction and validation of the glycolysis-related gene prognostic signature
We used 350 GC samples obtained from TCGA as the training set to construct the model. We also conducted a univariate Cox regression analysis to examine the relationship between the 55 glycolysis-related DEGs and patients’ OS in the training set. The univariate Cox regression analysis showed that 4 glycolysis-related DEGs (i.e., VCAN, EFNA3, ADH4, and CLDN9) were significantly correlated with patients’ OS in the training set (see Figure 5). A Kaplan-Meier analysis revealed that the OS of the VCAN, ADH4, and CLDN9 high-expression groups was significantly worse than that of the VCAN, ADH4, and CLDN9 low-expression groups (see Figure 5A,5B,5D). The Kaplan-Meier analysis also showed that the OS of the EFNA3 high-expression group was significantly higher than that of the EFNA3 low-expression group (see Figure 5C).
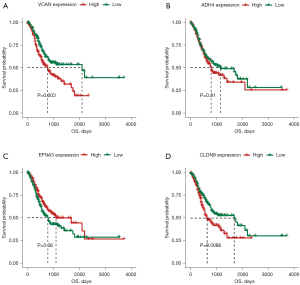
Next, the corresponding 4 glycolysis-related genes of VCAN, EFNA3, ADH4, and CLDN9 were selected for the LASSO regression analysis. Based on the results of the LASSO regression analyses, the 4 glycolysis-related genes of VCAN, EFNA3, ADH4, and CLDN9 were used to establish and validate the risk model for predicting GC patients’ outcomes and coefficients (see Figure 6 and Table 2). In the training set, the risk scores of the 350 GC samples obtained from TCGA were calculated using a LASSO regression analysis according to the predictive signature model of the 4 glycolysis-related genes. The following formula was used to calculate the risk scores of the 4 glycolysis-related genes: risk score = 0.013876966 × Expr (VCAN) – 0.016756713 × Expr (EFNA3) + 0.002457761 × Expr (ADH4) + 0.018168653 × Expr (CLDN9).
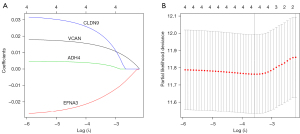
Table 2
No. | Gene | Coefficient |
---|---|---|
1 | VCAN | 0.013876966 |
2 | EFNA3 | −0.016756713 |
3 | ADH4 | 0.002457761 |
4 | CLDN9 | 0.018168653 |
LASSO, least absolute shrinkage and selection operator.
Next, the GC patients in TCGA-STAD training set were divided into high- and low-risk groups based on the median value of the risk score using the log-rank test (P=0.00074<0.001). As Figure 7 shows, in TCGA-STAD training group, the OS of the high-risk group was significantly worse than that of the low-risk group, and the median survival time of the low-risk patients was significantly prolonged. Additionally, the efficacy of the predictive signature model was further validated by the external independent GSE84437 testing set (n=433 samples) obtained from the GEO database (log-rank test P=0.022<0.05). The prediction efficiency of the GSE84437 testing set was consistent with the results of TCGA-STAD training set (see Figure 8).
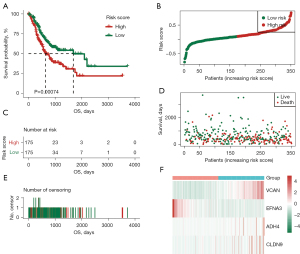
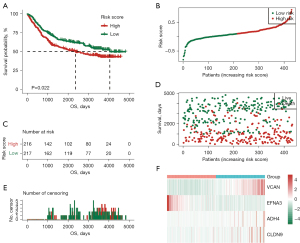
Identification of the risk scores of the 4 glycolysis-related genes correlated biological pathways
We also examined whether high-risk GC scores were correlated with specific mutations. As Figure 9 shows, the mutation rate of 5 genes was >19% in the high-risk score group, and the mutation rate of 25 genes was >19% in the low-risk score group. Notably, we did not find any significant correlations between the higher rates of gene mutations and low-risk scores.
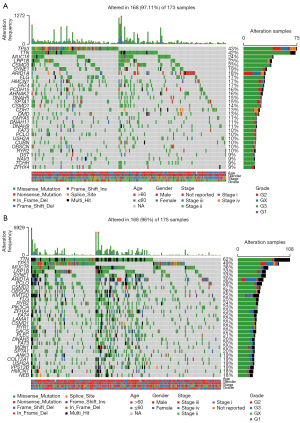
Estimation of the immune cell infiltration and immune infiltration scores in different risk groups
To further explore the correlations between immune cell infiltration and the 2 risk groups, we identified the infiltration of 22 types of immune cells in TCGA training set using CIBERSORT. As Figure 10 shows, in 22 types of immune infiltrating cells, the immune infiltration of naive B cells, plasma cells, T follicular helper cells, monocytes, M2 macrophages, resting dendritic cells, and resting Mast cells differed significantly between the high- and low-risk groups. The immune infiltrations of naive B cells (P=0.006), plasma cells (P=0.002), and T follicular helper cells (P=0.001) of the low-risk groups were much greater than those of the high-risk groups (see Figure 10). Moreover, the immune infiltrations of monocytes (P<0.001), M2 macrophages (P<0.001), resting dendritic cells (P=0.007), and resting Mast cells (P<0.0001) of high-risk groups were much greater than those of the low-risk groups (see Figure 10). To further examine the differences between the immune score and stromal score of the 2 risk groups, we used Estimation of Stromal and Immune cells in MAlignant Tumor tissues using Expression data (ESTIMATE) to evaluate the immune score and stromal score in TCGA training set. The ESTIMATE results showed that the immune and stromal scores of the high-risk groups were significantly higher than those of the low-risk groups (P=1.1e-09, P<2.2e-16) (see Figure 11).
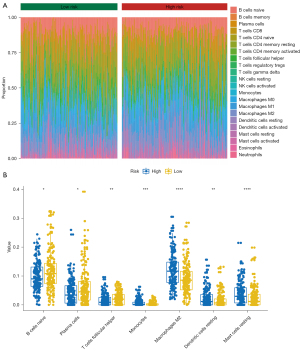
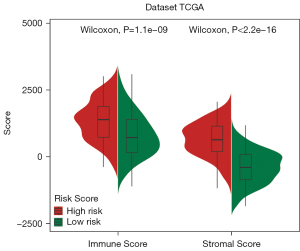
The 4 glycolysis-related gene signature as an independent prognostic factor
To explore whether the 4 glycolysis-related gene signature was an independent prognostic factor for GC, a univariate Cox regression analysis was conducted using the TCGA training set. The univariate analysis results indicated that risk score [hazards ratio (HR): 4.99; 95% confidence interval (CI): 2.55–9.77; P<0.001], age (HR: 1.02; 95% CI: 1.01–1.04; P=0.007), histologic grade (HR: 1.31; 95% CI: 0.98–1.75; P=0.068), gender (HR: 1.32; 95% CI: 0.93–1.89; P=0.068), and tumor stage (HR: 1.22; 95% CI: 1.02–1.46; P=0.028) were independent prognostic factors for GC (see Table 3). The multivariable analyses also indicated that risk score (HR: 5.42; 95% CI: 2.76–10.66; P<0.001), age (HR: 1.03; 95% CI: 1.02–1.05; P<0.001), and tumor stage (HR: 1.27; 95% CI: 1.05–1.53; P=0.012) remained independent prognostic factors for GC (see Table 3). These results demonstrated that risk score, age, and tumor stage were significantly correlated with the OS of the GC patients. After controlling for clinical features, including, age, histologic grade, gender, and tumor stage, the risk score of the 4 glycolysis-related gene signature was still an independent prognostic indicator for GC patients (see Table 3).
Table 3
Variable | Univariate analysis | Multivariate analysis | |||||
---|---|---|---|---|---|---|---|
HR | 95% CI | P value | HR | 95% CI | P value | ||
Risk score | 4.99 | 2.55–9.77 | <0.001 | 5.42 | 2.76–10.66 | <0.001 | |
Age | 1.02 | 1.01–1.04 | 0.007 | 1.03 | 1.02–1.05 | <0.001 | |
Histologic grade | 1.31 | 0.98–1.75 | 0.068 | – | – | – | |
Gender | 1.32 | 0.93–1.89 | 0.12 | – | – | – | |
Tumor stage | 1.22 | 1.02–1.46 | 0.028 | 1.27 | 1.05–1.53 | 0.012 |
HR, hazards ratio; CI, confidence interval.
Discussion
Due to the complicated molecular mechanisms and phenotypes of GC, the traditional prognostic systems, including Lauren classification, TNM staging and Borrmann classification, might be inaccurate at determining the prognosis of GC patients in clinical practice. Thus, specific prognostic signature genes for GC patients urgently need to be identified.
There is increasing evidence that glycolysis plays an important role in the development of GC (25,26). To explore the relationship between glycolysis-related genes and the prognosis of GC patients, we first identified a total of 55 glycolysis-related genes between the normal and GC samples. Next, 4 glycolysis-related genes (i.e., VCAN, EFNA3, ADH4, and CLDN9) were identified using univariate Cox and LASSO regression analyses, and a risk model for predicting GC patients was then established. The GC patients were divided into high- and low-risk groups in TCGA-STAD and the GSE84437 data sets according to the median risk score. We found that the OS of the high-risk group was significantly worse than that of the low-risk group, and the median survival time of the low-risk patients was significantly prolonged in TCGA-STAD and the GSE84437 data sets. The 4 glycolysis-related gene signature also provided insights into immune cell infiltration and immune infiltration scores in different risk groups. Additionally, we confirmed that the 4 glycolysis-related gene signature was an independent prognostic indicator for GC patients.
Aerobic glycolysis, which is a main energy source, provides ATP and nutrients for tumor cells, which contributes to the unlimited proliferation and distal metastasis of tumor cells (27-29). Recent studies have focused on clarifying the role of glycolysis-related genes in tumors. Zhang et al. found an 11-gene signature related to glycolysis for predicting the prognosis of breast cancer patients (14). Zhu et al. identified a 5 glycolysis-related gene signature for predicting the prognosis of colorectal cancer patients (17). Bi et al. constructed a 5 glycolysis-related gene signature for predicting the prognosis of ovarian cancer patients (30). Yu et al. also constructed a 7-gene signature for predicting the prognosis of GC patients (18).
There is increasing evidence that single gene features are poor reliable prognostic markers. Studies examining the relationship between glycolysis-related genes and the prognosis of GC patients are still lacking. In this study, we downloaded clinical materials from TCGA database to screen out a total of 55 glycolysis-related genes, which differed significantly between the GC and normal control samples. A total of 55 glycolysis-related genes were significantly enriched in the BPs and pathways of purine nucleoside monophosphate metabolism, carbohydrate catabolism, purine nucleoside monophosphate biosynthesis, ADP metabolism, glucose metabolism, nucleotide phosphorylation, and gluconeogenesis.
We also conducted univariate Cox and LASSO regression analyses to identify 4 glycolysis-related genes (i.e., VCAN, EFNA3, ADH4, and CLDN9). VCAN, which is a kind of chondroitin sulfate proteoglycan, is a component of the extracellular matrix (27932299). Some studies have shown that VCAN is positively correlated with a poor prognosis in GC patients (31-33). EFNA3 is expressed in a variety of tumors and is high in GC tissues, and thus might be used as a prognostic marker for GC patients (18,34). ADH4 is a member of the ADH family and can metabolize retinol and ethanol. Wei et al. reported that ADH4 can be used as a potential prognostic marker for hepatocellular carcinoma (35). There is increasing evidence that CLDN9 can be used as a potential prognostic marker for some cancer types, including esophageal adenocarcinoma, endometrial cancer, and GC (18,36,37).
In our study, the Kaplan-Meier analysis revealed that VCAN, EFNA3, ADH4, and CLDN9 were significantly associated with the OS of GC patients. VCAN, ADH4, and CLDN9 were positively correlated with the OS of GC patients. EFNA3 was negatively correlated with the OS of GC patients. Further, we developed and validated a glycolysis-related gene signature and risk-score model based on the expression of VCAN, EFNA3, ADH4, and CLDN9. The risk score model was divided into high- and low-risk groups. Our results showed the OS of the high-risk group was significantly worse than that of the low-risk group and the median survival time of the low-risk patients was significantly prolonged in TCGA-STAD and the GSE84437 data sets. Additionally, we found the risk score of the 4 glycolysis-related gene signature was an independent prognostic indicator for GC patients. Our results demonstrated that the 4 glycolysis-related gene signature was a reliable model for predicting the prognosis of GC patients.
Many studies have suggested that the immune microenvironment plays an important role in cancer development (38-40). The diverse clinical outcomes of cancer patients with the same histological type might be associated with different levels of immune infiltration. Zheng et al. show that EFNA3 is negatively correlated with the infiltration of immune cells in GC (34). Huang et al. demonstrated that VCAN is positively correlated with the high infiltration of immune cells in GC (31). Yu et al. also found that EFNA3 and CLDN9 are closely correlated to high immune infiltration in GC (18).
In this study, we identified the infiltration of 22 types of immune cells in TCGA training set using CIBERSORT. Our results suggest that immune infiltrations of naive B cells, plasma cells, and T follicular helper cells in the low-risk groups were much greater than those of the high-risk groups. Additionally, the immune infiltrations of monocytes, M2 macrophages, resting dendritic cells, and resting Mast cells in the high-risk groups were much greater than those of the low-risk groups. Additionally, we used ESTIMATE to calculate immune and stromal scores in TCGA training set. Our ESTIMATE results indicated that the immune and stromal scores of the high-risk groups were significantly higher than those of the low-risk groups. These results indicated that the 4 glycolysis-related gene signature was closely associated with immune cell infiltration in GC patients.
The present study had some limitations. First, the clinical information of GC patients was downloaded from public databases. Second, we need to further validate the prediction model in large-scale multicenter cohorts. Third, we need to verify our findings by conducting basic experiments at our hospital
In conclusion, a 4 glycolysis-related gene signature (comprising VCAN, EFNA3, ADH4, and CLDN9) was constructed and validated and found to be related to the prognosis of GC patients based on bioinformatics and biological validation studies. Our results indicate that a higher risk score indicates a poorer prognosis for GC patients. The 4 glycolysis-related gene signature could also provide novel insights into immunological biomarkers and the underlying mechanism of GC.
Acknowledgments
Funding: This work was financially supported by the National Natural Science Foundation of China—Youth Projects (grant No. 81402012), the Shaanxi Natural Science Foundation (grant No. 2019JM-547), the Shaanxi Innovative Talents Cultivate Program (grant No. 2017KCT-28), the Operating Expenses of Basic Scientific Research Project of Xi’an Jiaotong University (grant No. xzy012019112), the Science and Technology Project of Xi’an (grant No. 2019114613YX-001SF035[3]), the Shaanxi Province Key Industry Innovation Chain (Group) Project—Social Development Field (No. 2021ZDLSF01-07), the Scientific and Technological Talents Support Plan of Shaanxi Provincial People’s Hospital (Leading Talents) (No. 2021LJ-02), and the Scientific and Technological Talents Support Plan of Shaanxi Provincial People’s Hospital (Top Talent) (No. 2021BJ-01).
Footnote
Reporting Checklist: The authors have completed the TRIPOD reporting checklist. Available at https://atm.amegroups.com/article/view/10.21037/atm-22-3980/rc
Conflicts of Interest: All authors have completed the ICMJE uniform disclosure form (available at https://atm.amegroups.com/article/view/10.21037/atm-22-3980/coif). All authors report that this work was supported by the National Natural Science Foundation of China—Youth Projects (grant No. 81402012), the Shaanxi Natural Science Foundation (grant No. 2019JM-547), the Shaanxi Innovative Talents Cultivate Program (grant No. 2017KCT-28), the Operating Expenses of Basic Scientific Research Project of Xi’an Jiaotong University (grant No. xzy012019112), the Science and Technology Project of Xi’an (grant No. 2019114613YX-001SF035[3]), the Shaanxi Province Key Industry Innovation Chain (Group) Project—Social Development Field (No. 2021ZDLSF01-07), the Scientific and Technological Talents Support Plan of Shaanxi Provincial People’s Hospital (Leading Talents) (No. 2021LJ-02), and the Scientific and Technological Talents Support Plan of Shaanxi Provincial People’s Hospital (Top Talent) (No. 2021BJ-01). The authors have no other conflicts of interest to declare.
Ethical Statement: The authors are accountable for all aspects of the work in ensuring that questions related to the accuracy or integrity of any part of the work are appropriately investigated and resolved. The study was conducted in accordance with the Declaration of Helsinki (as revised in 2013).
Open Access Statement: This is an Open Access article distributed in accordance with the Creative Commons Attribution-NonCommercial-NoDerivs 4.0 International License (CC BY-NC-ND 4.0), which permits the non-commercial replication and distribution of the article with the strict proviso that no changes or edits are made and the original work is properly cited (including links to both the formal publication through the relevant DOI and the license). See: https://creativecommons.org/licenses/by-nc-nd/4.0/.
References
- Russo AE, Strong VE. Gastric Cancer Etiology and Management in Asia and the West. Annu Rev Med 2019;70:353-67. [Crossref] [PubMed]
- Camargo MC, Figueiredo C, Machado JC. Review: Gastric malignancies: Basic aspects. Helicobacter 2019;24:e12642. [Crossref] [PubMed]
- Miao RL, Wu AW. Towards personalized perioperative treatment for advanced gastric cancer. World J Gastroenterol 2014;20:11586-94. [Crossref] [PubMed]
- Fu M, Gu J, Jiang P, et al. Exosomes in gastric cancer: roles, mechanisms, and applications. Mol Cancer 2019;18:41. [Crossref] [PubMed]
- Hanahan D, Weinberg RA. Hallmarks of cancer: the next generation. Cell 2011;144:646-74. [Crossref] [PubMed]
- Crunkhorn S. Targeting cancer cell metabolism in glioblastoma. Nat Rev Cancer 2019;19:250. [Crossref] [PubMed]
- Rosario SR, Long MD, Affronti HC, et al. Pan-cancer analysis of transcriptional metabolic dysregulation using The Cancer Genome Atlas. Nat Commun 2018;9:5330. [Crossref] [PubMed]
- Hu Q, Qin Y, Ji S, et al. UHRF1 promotes aerobic glycolysis and proliferation via suppression of SIRT4 in pancreatic cancer. Cancer Lett 2019;452:226-36. [Crossref] [PubMed]
- Liu Y, Tong L, Luo Y, et al. Resveratrol inhibits the proliferation and induces the apoptosis in ovarian cancer cells via inhibiting glycolysis and targeting AMPK/mTOR signaling pathway. J Cell Biochem 2018;119:6162-72. [Crossref] [PubMed]
- Huang X, Hou Y, Weng X, et al. Diethyldithiocarbamate-copper complex (CuET) inhibits colorectal cancer progression via miR-16-5p and 15b-5p/ALDH1A3/PKM2 axis-mediated aerobic glycolysis pathway. Oncogenesis 2021;10:4. [Crossref] [PubMed]
- Lv Z, Qi L, Hu X, et al. Identification of a Novel Glycolysis-Related Gene Signature Correlates With the Prognosis and Therapeutic Responses in Patients With Clear Cell Renal Cell Carcinoma. Front Oncol 2021;11:633950. [Crossref] [PubMed]
- Zhang L, Zhang Z, Yu Z. Identification of a novel glycolysis-related gene signature for predicting metastasis and survival in patients with lung adenocarcinoma. J Transl Med 2019;17:423. [Crossref] [PubMed]
- Zhou W, Zhang S, Cai Z, et al. A glycolysis-related gene pairs signature predicts prognosis in patients with hepatocellular carcinoma. PeerJ 2020;8:e9944. [Crossref] [PubMed]
- Zhang D, Zheng Y, Yang S, et al. Identification of a Novel Glycolysis-Related Gene Signature for Predicting Breast Cancer Survival. Front Oncol 2021;10:596087. [Crossref] [PubMed]
- He M, Hu C, Deng J, et al. Identification of a novel glycolysis-related signature to predict the prognosis of patients with breast cancer. World J Surg Oncol 2021;19:294. [Crossref] [PubMed]
- Yu J, Liu TT, Liang LL, et al. Identification and validation of a novel glycolysis-related gene signature for predicting the prognosis in ovarian cancer. Cancer Cell Int 2021;21:353. [Crossref] [PubMed]
- Zhu J, Wang S, Bai H, et al. Identification of Five Glycolysis-Related Gene Signature and Risk Score Model for Colorectal Cancer. Front Oncol 2021;11:588811. [Crossref] [PubMed]
- Yu S, Hu C, Cai L, et al. Seven-Gene Signature Based on Glycolysis Is Closely Related to the Prognosis and Tumor Immune Infiltration of Patients With Gastric Cancer. Front Oncol 2020;10:1778. [Crossref] [PubMed]
- Zhao X, Zou J, Wang Z, et al. Identifying Novel Cell Glycolysis-Related Gene Signature Predictive of Overall Survival in Gastric Cancer. Biomed Res Int 2021;2021:9656947. [Crossref] [PubMed]
- Robinson MD, McCarthy DJ, Smyth GK. edgeR: a Bioconductor package for differential expression analysis of digital gene expression data. Bioinformatics 2010;26:139-40. [Crossref] [PubMed]
- Yu G, Wang LG, Han Y, et al. clusterProfiler: an R package for comparing biological themes among gene clusters. OMICS 2012;16:284-7. [Crossref] [PubMed]
- Friedman J, Hastie T, Tibshirani R. Regularization Paths for Generalized Linear Models via Coordinate Descent. J Stat Softw 2010;33:1-22. [Crossref] [PubMed]
- Wang H, Lengerich BJ, Aragam B, et al. Precision Lasso: accounting for correlations and linear dependencies in high-dimensional genomic data. Bioinformatics 2019;35:1181-7. [Crossref] [PubMed]
- Newman AM, Liu CL, Green MR, et al. Robust enumeration of cell subsets from tissue expression profiles. Nat Methods 2015;12:453-7. [Crossref] [PubMed]
- Nie X, Wang H, Wei X, et al. LRP5 Promotes Gastric Cancer via Activating Canonical Wnt/β-Catenin and Glycolysis Pathways. Am J Pathol 2022;192:503-17. [Crossref] [PubMed]
- Liu Y, Zhang Z, Wang J, et al. Metabolic reprogramming results in abnormal glycolysis in gastric cancer: a review. Onco Targets Ther 2019;12:1195-204. [Crossref] [PubMed]
- Li A, Hou S, Chen J, et al. Development and validation of a novel glycolysis-related risk signature for predicting survival in pancreatic adenocarcinoma. Clin Chim Acta 2021;518:156-61. [Crossref] [PubMed]
- Vaupel P, Schmidberger H, Mayer A. The Warburg effect: essential part of metabolic reprogramming and central contributor to cancer progression. Int J Radiat Biol 2019;95:912-9. [Crossref] [PubMed]
- Ganapathy-Kanniappan S. Molecular intricacies of aerobic glycolysis in cancer: current insights into the classic metabolic phenotype. Crit Rev Biochem Mol Biol 2018;53:667-82. [Crossref] [PubMed]
- Bi J, Bi F, Pan X, et al. Establishment of a novel glycolysis-related prognostic gene signature for ovarian cancer and its relationships with immune infiltration of the tumor microenvironment. J Transl Med 2021;19:382. [Crossref] [PubMed]
- Huang XY, Liu JJ, Liu X, et al. Bioinformatics analysis of the prognosis and biological significance of VCAN in gastric cancer. Immun Inflamm Dis 2021;9:547-59. [Crossref] [PubMed]
- Li W, Han F, Fu M, et al. High expression of VCAN is an independent predictor of poor prognosis in gastric cancer. J Int Med Res 2020;48:300060519891271. [Crossref] [PubMed]
- Zhu Z, Xu J, Li L, et al. Comprehensive analysis reveals CTHRC1, SERPINE1, VCAN and UPK1B as the novel prognostic markers in gastric cancer. Transl Cancer Res 2020;9:4093-110. [Crossref] [PubMed]
- Zheng P, Liu X, Li H, et al. EFNA3 Is a Prognostic Biomarker Correlated With Immune Cell Infiltration and Immune Checkpoints in Gastric Cancer. Front Genet 2022;12:796592. [Crossref] [PubMed]
- Wei RR, Zhang MY, Rao HL, et al. Identification of ADH4 as a novel and potential prognostic marker in hepatocellular carcinoma. Med Oncol 2012;29:2737-43. [Crossref] [PubMed]
- Kang H, Wang N, Wang X, et al. A glycolysis-related gene signature predicts prognosis of patients with esophageal adenocarcinoma. Aging (Albany NY) 2020;12:25828-44. [Crossref] [PubMed]
- Wang ZH, Zhang YZ, Wang YS, et al. Identification of novel cell glycolysis related gene signature predicting survival in patients with endometrial cancer. Cancer Cell Int 2019;19:296. [Crossref] [PubMed]
- Lei X, Lei Y, Li JK, et al. Immune cells within the tumor microenvironment: Biological functions and roles in cancer immunotherapy. Cancer Lett 2020;470:126-33. [Crossref] [PubMed]
- Zhang K, Ping L, Du T, et al. A Ferroptosis-Related lncRNAs Signature Predicts Prognosis and Immune Microenvironment for Breast Cancer. Front Mol Biosci 2021;8:678877. [Crossref] [PubMed]
- Huber M, Brehm CU, Gress TM, et al. The Immune Microenvironment in Pancreatic Cancer. Int J Mol Sci 2020;21:7307. [Crossref] [PubMed]
(English Language Editor: L. Huleatt)