Identification of key genes associated with cancer stem cell characteristics in Wilms’ tumor based on bioinformatics analysis
Introduction
Nephroblastoma, also known as Wilms’ tumor (WT), is the primary pathological type of renal tumor in children, accounting for 6.4% of childhood cancers (1). In recent years, significant achievements have been made in WT treatment, and more than 80% of children with WT can be cured by modern multisystemic treatment (2,3). However, 5~10% of patients still suffer from cancer metastasis or chemotherapeutic resistance, which seriously threatens their lives (4). Therefore, new and promising treatments are needed to improve the prognosis of these patients.
In recent years, significant progress has been made in understanding the relationship between stem cells and cancer (5,6). Cancer stem cells (CSCs) have been identified as the driving forces for tumor initiation, proliferation, and recurrence (7,8). Senanayake et al. reported that the pluripotent renal stem cell regulator SIX2 was activated in WT, influencing cellular proliferation and migration (9). Raved et al. found that CSCs were significantly associated with poor survival in WT and could be used for risk stratification (10). The discovery of specific biomarkers in CSC research would facilitate targeted treatment of the disease, which could significantly reduce tumor metastasis and drug resistance (7,11-13). Malta et al. defined stem cell characteristics using an innovative logistic regression algorithm based on mRNA expression analysis, which effectively evaluated the degree of dedifferentiation of cancer and provided a novel concept for targeted cancer treatment (14). However, in-depth studies of CSCs at the WT transcriptome level have not been conducted, and related targeted therapies are lacking. Therefore, a therapeutic target study of WT based on the mRNA stem index (mRNAsi) is warranted to extend the use of CSCs in WT therapy to provide new perspectives in treatment.
Weighted gene co-expression network analysis (WGCNA) is a systems biology method that describes the pattern of gene associations among disparate samples. This method obtains different gene modules with highly coordinated changes and can be used to construct gene co-expression networks, calculate the weighted correlation of genes, and perform hierarchical clustering analysis (15). Moreover, WGCNA can be used to identify alternate biomarker genes or therapeutic targets according to the intrinsic correlation of gene sets and the association between gene sets and phenotypes. Gene expression analysis is now widely applied in studies of various cancers, including esophageal squamous cell cancer, endometrial cancer, and myeloid leukemia (16-19). In this study, we aimed to use WGCNA to systematically investigate CSC characteristics based on the mRNA level in WT and identify related targeted genes in the hope of offering a foundation for targeted WT treatment.
Methods
Data download and pre-processing
The study was conducted in accordance with the Declaration of Helsinki (as revised in 2013). The fragments per kilobase of exon model per million mapped reads (FPKM) RNA-seq data and clinical data of 120 WT and six para-cancerous tissues were obtained from the UCSC Xena (http://xena.ucsc.edu) database (Table S1). The Ensemble IDs were converted to Human Gene Nomenclature Committee (HGNC) symbols based on the Ensemble database using the “biomeRt” R package (http://asia.ensembl.org/index.html). The Ensemble IDs with the highest expression value were retained when more than one Ensemble ID matched the same HGNC symbol. Using the R platform, we calculated the mRNAsi score of each tissue using the methodology outlined in previous reports (14).
Acquisition of differentially expressed genes (DEGs)
The identification of DEGs in WT and normal controls was established using the “edgeR” R package, with the cut-off threshold of the false discovery rate (FDR) set at <0.05 and |log2fold change| >1.5. Before analysis with the “edgeR” R package, the low-expression genes with <1 count per million (CPM) in less than a third of samples were filtered to improve the accuracy of the results.
WGCNA
The expression profiles of the DEGs were constructed to perform WGCNA using the “WGCNA” R package. Specifically, The RNA-FPKM data were firstly filtered to reduce outliers. The correlation matrices were performed for pairwise DEGs. Next, the weighted adjacency matrix was constructed using the power function amn= | cmn|β. Specifically, the β represented the exponential parameter for power law distribution, which was used to characterize the likeness to a scale-free network. We chose a suitable β to transform the adjacent matrix into a topological overlap matrix (TOM), which displayed the network sum connectivity of DEGs with other DEGs for the network generation. We calculated the average linkage hierarchical clustering based on the TOM-based dissimilarity measure to identify genes with similar expression profiles in the gene modules. The TOM-based dissimilarity measure with a minimum size of 50 was identified for the gene dendrogram. A cut line (<0.35) was chosen for merging the dendrogram module.
Identification of mRNAsi-related modules
Module eigengenes (MEs), gene significance (GS), and module significance (MS) were calculated to identify the significant mRNAsi-related modules. The MEs indicated the major component in the principal component analysis for each DEG module. The expression pattern of all DEGs could be condensed into a single characteristic expression with a given module. Moreover, the correlation between MEs and clinical characteristics was performed to identify the relevant module. The GS was identified as the log10 transformation of the P value (GS = lg P) in the linear regression between the gene expression and clinical information. In addition, MS indicated the average GS for all the genes in a module.
Selection of hub genes
The hub genes were identified by the correlation between expression profiles and MEs. Genes with an MM threshold >0.8 and a GS threshold >0.45 were defined as the hub genes.
Kyoto Encyclopedia of Genes and Genomes (KEGG) analysis
A KEGG analysis was performed for the key genes and key gene modules to explore the signaling pathways associated with the mRNAsi. The threshold was set to an adjusted P value <0.05.
Validation of key gene expressions
To validate the differentially expressed pattern of hub key genes associated with WT, expression profiles from the GSE66405 dataset, which included 28 WT samples and four non-tumor samples, were acquired using the “GEOquery” R package. The probes were converted to gene symbols based on the annotation file of GPL17077 platform. Next, we validated the expressions of key gene in GSE66405 validation dataset using the t-test.
Causal relationship and protein interactions
A DisNor (https://disnor.uniroma2.it/) analysis was performed to explore the protein interaction networks linking the disease genes with the causal interaction information annotated in SIGNOR database (https://signor.uniroma2.it/) and the protein interaction data in Mentha database (http://mentha.uniroma2.it/). We used STRING (https://www.string-db.org) analysis to validate the protein interaction relationships.
Gene co-expression analysis
According to the gene expression level, the co-expression relationship between the key gene and the upstream gene was calculated, and the expression levels of the key gene and the upstream gene were determined. The R package “corrplot” was used to calculate the Pearson correlation between the genes.
Statistical analysis
All statistical analyses were performed in R 3.6.1 (http://www.r-project.org/) and its corresponding R packages. The Kruskal-Wallis test was performed to determine differences in the mRNAsi between normal and tumor tissues. A Kaplan-Meier (KM) analysis was conducted using the “survival” R package, and a KEGG analysis was completed using the “clusterProfiler” R package. A gene co-expression analysis between the key genes and the upstream genes was performed based on the gene expression levels using Pearson’s correlation coefficient in the “corrplot” R package. The P value of less than 0.05 indicated a statistically significant difference in all analysis.
Results
Clinical characteristics of the mRNAsi in WT
The mRNAsi was defined as a quantitative representation of CSCs and indicated the degree of similarity between tumor cells and stem cells. The mRNAsi scores of normal and tumor tissues showed significant statistical differences (Figure 1A, P=7.2e-05). Moreover, based on the KM analysis, we observed that patients with lower mRNAsi scores had a better OS than those with higher mRNAsi scores (Figure 1B, P=0.0087).
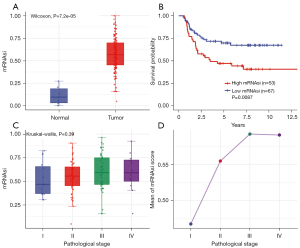
We further explored the relationship between the mRNAsi score and pathological stage. The results indicated that the average mRNAsi score tended to increase in line with the pathological stage, although no significant differences were shown between stages. The T3 stage had the highest mRNAsi score (Figure 1C,1D, P=0.39).
Identification of mRNAsi-related modules in WGCNA
We identified 2,408 DEGs between the normal and WT tissues (Figure 2A,2B) and used these to identify the mRNAsi-related modules. In our analysis, we chose β=3 (scale-free R =0.85) as a soft threshold to establish a scale-free network after filtering the outlier samples (Figure S1). Finally, 13 modules were built to identify the relationships between the modules and the mRNAsi (Figure 3A). We used MS as each module’s overall gene expression level to calculate the correlations between clinical phenotypes and the mRNAsi. Intriguingly, the blue module was most significantly associated with the mRNAsi, with a correlation close to 0.6 (Figure 3B). In addition, the brown, pink, green, yellow, and turquoise modules exhibited relatively high correlations with the mRNAsi (Figure 3B,3C).
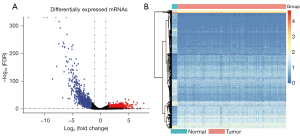
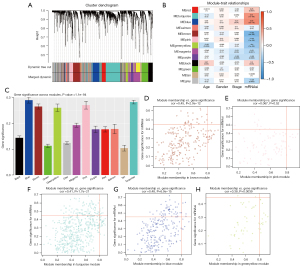
Identification of mRNAsi-related key genes
A total of 11 genes with a threshold of cor.MM >0.8 and cor.GS >0.45 were defined as the key genes associated with the mRNAsi, including CCNB1, CDK, MAD2L1, CENPK, ACSL1, TPX2, SPAG5, ARHGAP11A, FANCI, NCAPH, and BLM (Figure 3D-3H).
KEGG analysis
The KEGG analysis showed that cell proliferation-related signaling pathways were closely associated with the mRNAsi, including cell cycle, p53 signaling pathway, and cellular senescence (Figure 4A,4B).
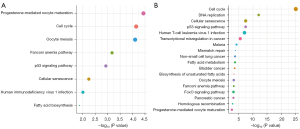
Validation of key gene expression
Nine genes overlapped with the above 11 key genes in the GSE66405 dataset, and the nine overlapped key genes were all verified as WT DEGs using the t-test (Figure 5, P<0.05).
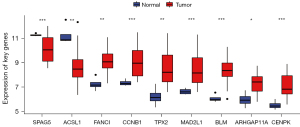
Causal relationships and protein interactions
The DisNor analysis revealed the first neighbor genes of six key genes, among which ATM and CDKN1A were identified as important upstream genes that directly or indirectly affected at least two key genes (Figure 6A). The protein interaction relationships between the key genes and upstream genes were also validated using STRING, which revealed that ATM and CDKN1A were significantly associated with most of the key genes (Figure 6B).
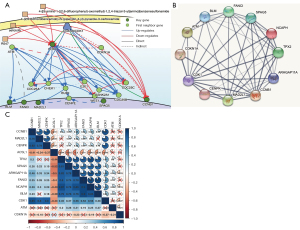
Co-expression of key genes and upstream genes
Most of the key genes and upstream genes were significantly related to each other, with a P value <0.05 in the co-expression analysis (Figure 6C). Our results indicated that the protein interaction relationship between the key genes and upstream genes was accurate and reliable. Further, the upstream gene ATM directly or indirectly upregulated the expression of the key genes, whereas CDKN1A downregulated the expression of the key genes. This finding was also validated by the co-expression analysis of the key genes and upstream genes (Figure 6C).
Discussion
Emerging stem cell theory has shown that CSCs are characterized by self-renewal and limitless proliferation, which significantly affects the diagnosis and treatment of cancer (20-23). Previous studies have also confirmed that CSCs are significantly associated with poor clinicopathological parameters and prognosis in WT (9,10). Our analysis also revealed significant changes in the mRNAsi score of WT samples, thus justifying our quest to elucidate the role of CSCs in WT.
Our KM analysis indicated that the mRNAsi was closely associated with OS in WT, and a higher mRNAsi score had a poorer OS. At the same time, through the observation of WT staging, we found that the mRNAsi score showed an upward trend in line with the pathological stage. This suggests that the mRNAsi score may serve as an important clinical parameter to guide clinical decision-making in WT. But further research is still needed.
As an unsupervised data mining method, WGCNA can closely link gene modules to clinical phenotypes (15), and this method has been used in several previous studies (24,25). In this study, we used WGCNA to identify 11 key genes associated with the mRNAsi, including CCNB1, CDK1, MAD2L1, CENPK, ACSL1, TPX2, SPAG5, ARHGAP11, FANCI, NCAP, and BLM. Jin et al. (26) reported that an increased expression of CDK1/CCNB1 could regulate the cell cycle, inhibit apoptosis, and promote the invasion of cancer cells, which is also consistent with our KEGG signaling analysis results of the close associations with CSC characteristics. In addition, another study reported that CCNB1 can provide a survival advantage and escape the host immune response in non-small cell lung cancer (27). Notably, CDK1-mediated phosphorylation of TFCP2L1 has been shown to be necessary for stem cell pluripotency and bladder carcinogenesis (28), and CDK1 can also inhibit ∆Np63α to promote epithelial-mesenchymal transformation and the migration of head and neck squamous cell carcinoma cells (29). MAD2L1 is a key component of the mitotic checkpoint complex protein and has been shown to play an important role in the proliferation of gastric cancer cells (30). CENPK is a cancer-promoting factor in ovarian cancer that is associated with poor prognosis (31) and promotes gastric cancer cell proliferation and migration by interacting with XRCC5 (32). Ma et al. indicated that ACSL1 promoted the development of prostate cancer by increasing the accumulation of lipids and enhancing fatty acid β oxidation (33). TPX2 has been shown to promote the epithelial-mesenchymal transition of prostate cancer by increasing the expression of CDK1 and the phosphorylation of the ERK/GSK3β/SNAIL pathway (34). Wang et al. found that the inhibition of NCAPH expression could inhibit the proliferation, in vitro migration, and in vivo xenograft tumor formation of cervical carcinoma cells. In addition, the knockdown of NCAPH also promoted apoptosis and cell cycle arrest in the G2/M phase (35). SPAG5 plays an essential role in anaphase mitosis and has been identified as a proliferation marker and predictor of chemotherapeutic sensitivity (36,37), playing a driving role in various cancers (38-41). Dai et al. showed that ARHGAP11A promotes the malignant progression of hepatocellular carcinoma through its interaction with RAC1B (42). It is also associated with the malignant progression of gastric adenocarcinoma and poor prognosis in lung adenocarcinoma (43,44). FANCI and BLM have also been implicated in the development of various cancers (45-48). NCAPH reportedly regulates the progression of gastric cancer through DNA damage response, promotes cell proliferation via the MEK/ERK signaling pathway, and inhibits apoptosis in bladder cancer cells (49,50). The above explanation indicates that these 11 genes play an important role in the occurrence and development of cancer. Most of them are closely related to cell proliferation and cell cycle, and this finding provides a foundation for identifying biomarkers related to CSC characteristics in WT.
Enrichment analysis is used to ascertain whether a set of genes is over-represented at a specific functional node (51). The KEGG signaling analysis was used to explore the enrichment nodes of 11 key genes and identify the biological processes most related to biological phenomena. The KEGG result showed that these biological processes were closely related to CSC characteristics, including cell cycle, cell senescence, p53 signaling pathway, etc. The cell cycle is a carefully regulated and highly organized process that ensures stable replication of genetic material and normal cell division (52). Disruption of the cell cycle is the basis of abnormal cell proliferation, and uncontrolled cell cycle checkpoints contribute to genetic instability (53). Cancer is characterized by a disturbance in the cell cycle, and many studies have shown that cell cycle disturbance drives the occurrence and progression of cancer (54,55). Cell senescence is a type of stable cell cycle arrest in diploid cells, which restricts the infinite proliferation of cells (56). Activation of oncogenes in mammalian cells induces senescence, thereby limiting tumor growth. However, recent studies have revealed that cellular senescence has dual roles in tumorigenesis and suppression. The induction of senescence in cancer cells may exert pro-tumorigenic effects and contribute to the processes of cancer invasion, metastasis, and disease recurrence, resulting in a negative impact. Thus, targeted drugs that selectively clear residual cancer cells after therapy-induced senescence are being developed (57). The transcription factor p53 is an important tumor suppressor that has important roles in apoptosis, growth inhibition, inhibition of cell cycle progression, differentiation and acceleration of DNA repair, genotoxicity, and senescence after cell stress (58,59). However, some studies have shown that p53 can promote tumor regeneration under the induction of chemotherapy drugs (60). In recent years, an increasing number of studies on p53 have revealed the complexity and connectivity of the p53 pathway, many of which have extended beyond cancer research into the field of stem cell biology and other diverse aspects of health and disease (61). Our KEGG analysis further elucidates the potential biological functions of these 11 key genes and verifies their close correlations with stem cells, cell proliferation, and other characteristics at the functional level.
Further, using Disnor and gene co-expression analyses, we found that ATM and CDKN1A were key upstream genes that directly or indirectly affected at least two key genes. Relevant studies have also shown the importance of these two genes in cancer. The mutation of ATM could cause ataxia telangiectasia, which is an autosomal recessive genetic disease with radiation sensitivity and cancer susceptibility, as well as mammary carcinoma and WT (62,63). In addition, ATM mutation carriers are predisposed to a higher risk of cancer, including breast cancer, pancreatic cancer, gastric cancer, and prostate cancer (64). Additionally, the ATM signal is enhanced in some advanced tumors and promotes the invasion and metastasis of cancer cells and increases the resistance of cancer cells to radiotherapy and chemotherapy (65). CDKN1A is a universal cell cycle inhibitor (66). Previous studies have shown that CDKN1A has an effect on proliferation, invasion, and metastasis in various types of tumors, including breast cancer (67,68), bladder cancer (69), thyroid cancer (70), and gastric cancer (71,72). In addition, CDKN1A also plays a role in regulating tumor response to treatment (22). Liu et al. have illustrated that downregulation of CDKN1A promotes cisplatin resistance in human lung adenocarcinoma (73), and Zamagni et al. have shown that CDKN1A silencing increased apoptosis and G1/S cell cycle arrest, thereby improving the efficacy of the cisplatin-pemetrexed combination (74). These studies indicate that these two upstream genes are closely related to the occurrence and development of cancers, providing a specific basis for WT CSC-targeted therapy.
Despite our specific key findings, this study has some limitations. First, in vitro or in vivo experiments are imperative to verify the reliability of our analytical results. Second, combining the mRNAsi index with multiple genomic data, such as mutant and methylation data, could improve its credibility. Therefore, a well-designed clinical trial to validate our observations is warranted, which will provide new insights into targeted therapies for WT patients.
Conclusions
In this study, we evaluated the CSC characteristics of WT at the transcriptome level and identified 11 key genes and two upstream genes related to CSCs. Our findings provide a foundation for identifying biomarkers related to CSC characteristics in WT.
Acknowledgments
Funding: The present study was supported by the Guangxi Natural Science Foundation (grant No. 2022GXNSFAA035641), the Basic Ability Enhancement Program for Young and Middle-aged Teachers of Guangxi (grant Nos. 2020KY03022 and 2021KY0084), the Scientific Research Project of Guangxi Provincial Health and Family Planning Commission (grant No. Z20180943), and the College Student Innovation and Entrepreneurship Project (No. x202210598321).
Footnote
Conflicts of Interest: All authors have completed the ICMJE uniform disclosure form (available at https://atm.amegroups.com/article/view/10.21037/atm-22-4477/coif). The authors have no conflicts of interest to declare.
Ethical Statement: The authors are accountable for all aspects of the work in ensuring that questions related to the accuracy or integrity of any part of the work are appropriately investigated and resolved. The study was conducted in accordance with the Declaration of Helsinki (as revised in 2013).
Open Access Statement: This is an Open Access article distributed in accordance with the Creative Commons Attribution-NonCommercial-NoDerivs 4.0 International License (CC BY-NC-ND 4.0), which permits the non-commercial replication and distribution of the article with the strict proviso that no changes or edits are made and the original work is properly cited (including links to both the formal publication through the relevant DOI and the license). See: https://creativecommons.org/licenses/by-nc-nd/4.0/.
References
- Miller RW, Young JL Jr, Novakovic B. Childhood cancer. Cancer 1995;75:395-405. [Crossref] [PubMed]
- Gatta G, Botta L, Rossi S, et al. Childhood cancer survival in Europe 1999-2007: results of EUROCARE-5--a population-based study. Lancet Oncol 2014;15:35-47. [Crossref] [PubMed]
- Green DM, Breslow NE, D'Angio GJ, et al. Outcome of patients with Stage II/favorable histology Wilms tumor with and without local tumor spill: a report from the National Wilms Tumor Study Group. Pediatr Blood Cancer 2014;61:134-9. [Crossref] [PubMed]
- Ghanem MA, van Steenbrugge GJ, Sudaryo MK, et al. Expression and prognostic relevance of vascular endothelial growth factor (VEGF) and its receptor (FLT-1) in nephroblastoma. J Clin Pathol 2003;56:107-13. [Crossref] [PubMed]
- Sirkisoon SR, Carpenter RL, Rimkus T, et al. TGLI1 transcription factor mediates breast cancer brain metastasis via activating metastasis-initiating cancer stem cells and astrocytes in the tumor microenvironment. Oncogene 2020;39:64-78. [Crossref] [PubMed]
- Gagliardi F, Narayanan A, Gallotti AL, et al. Enhanced SPARCL1 expression in cancer stem cells improves preclinical modeling of glioblastoma by promoting both tumor infiltration and angiogenesis. Neurobiol Dis 2020;134:104705. [Crossref] [PubMed]
- Clarke MF, Dick JE, Dirks PB, et al. Cancer stem cells--perspectives on current status and future directions: AACR Workshop on cancer stem cells. Cancer Res 2006;66:9339-44. [Crossref] [PubMed]
- Reya T, Morrison SJ, Clarke MF, et al. Stem cells, cancer, and cancer stem cells. Nature 2001;414:105-11. [Crossref] [PubMed]
- Senanayake U, Koller K, Pichler M, et al. The pluripotent renal stem cell regulator SIX2 is activated in renal neoplasms and influences cellular proliferation and migration. Hum Pathol 2013;44:336-45. [Crossref] [PubMed]
- Raved D, Tokatly-Latzer I, Anafi L, et al. Blastemal NCAM+ALDH1+ Wilms’ tumor cancer stem cells correlate with disease progression and poor clinical outcome: A pilot study. Pathol Res Pract 2019;215:152491. [Crossref] [PubMed]
- Jin L, Hope KJ, Zhai Q, et al. Targeting of CD44 eradicates human acute myeloid leukemic stem cells. Nat Med 2006;12:1167-74. [Crossref] [PubMed]
- Galmozzi E, Facchetti F, La Porta CA. Cancer stem cells and therapeutic perspectives. Curr Med Chem 2006;13:603-7. [Crossref] [PubMed]
- Huntly BJ, Gilliland DG. Leukaemia stem cells and the evolution of cancer-stem-cell research. Nat Rev Cancer 2005;5:311-21. [Crossref] [PubMed]
- Malta TM, Sokolov A, Gentles AJ, et al. Machine Learning Identifies Stemness Features Associated with Oncogenic Dedifferentiation. Cell 2018;173:338-354.e15. [Crossref] [PubMed]
- Langfelder P, Horvath S. WGCNA: an R package for weighted correlation network analysis. BMC Bioinformatics 2008;9:559. [Crossref] [PubMed]
- Wang W, Fu S, Lin X, et al. miR-92b-3p Functions As A Key Gene In Esophageal Squamous Cell Cancer As Determined By Co-Expression Analysis. Onco Targets Ther 2019;12:8339-53. [Crossref] [PubMed]
- Huo X, Sun H, Liu Q, et al. Clinical and Expression Significance of AKT1 by Co-expression Network Analysis in Endometrial Cancer. Front Oncol 2019;9:1147. [Crossref] [PubMed]
- Chen CT, Wang PP, Mo WJ, et al. Expression profile analysis of prognostic long non-coding RNA in adult acute myeloid leukemia by weighted gene co-expression network analysis (WGCNA). J Cancer 2019;10:4707-18. [Crossref] [PubMed]
- Zuo Y, Liang Y, Zhang J, et al. Transcriptome Analysis Identifies Piwi-Interacting RNAs as Prognostic Markers for Recurrence of Prostate Cancer. Front Genet 2019;10:1018. [Crossref] [PubMed]
- Horikoshi Y, Yan Y, Terashvili M, et al. Fatty Acid-Treated Induced Pluripotent Stem Cell-Derived Human Cardiomyocytes Exhibit Adult Cardiomyocyte-Like Energy Metabolism Phenotypes. Cells 2019;8:1095. [Crossref] [PubMed]
- MacDonagh L, Gray SG, Breen E, et al. Lung cancer stem cells: The root of resistance. Cancer Lett 2016;372:147-56. [Crossref] [PubMed]
- Xiao BD, Zhao YJ, Jia XY, et al. Multifaceted p21 in carcinogenesis, stemness of tumor and tumor therapy. World J Stem Cells 2020;12:481-7. [Crossref] [PubMed]
- Yang L, Shi P, Zhao G, et al. Targeting cancer stem cell pathways for cancer therapy. Signal Transduct Target Ther 2020;5:8. [Crossref] [PubMed]
- Tang J, Kong D, Cui Q, et al. Prognostic Genes of Breast Cancer Identified by Gene Co-expression Network Analysis. Front Oncol 2018;8:374. [Crossref] [PubMed]
- Chen EB, Qin X, Peng K, et al. HnRNPR-CCNB1/CENPF axis contributes to gastric cancer proliferation and metastasis. Aging (Albany NY) 2019;11:7473-91. [Crossref] [PubMed]
- Jin J, Xu H, Li W, et al. LINC00346 Acts as a Competing Endogenous RNA Regulating Development of Hepatocellular Carcinoma via Modulating CDK1/CCNB1 Axis. Front Bioeng Biotechnol 2020;8:54. [Crossref] [PubMed]
- Arora S, Singh P, Rahmani AH, et al. Unravelling the Role of miR-20b-5p, CCNB1, HMGA2 and E2F7 in Development and Progression of Non-Small Cell Lung Cancer (NSCLC). Biology (Basel) 2020;9:201. [Crossref] [PubMed]
- Heo J, Noh BJ, Lee S, et al. Phosphorylation of TFCP2L1 by CDK1 is required for stem cell pluripotency and bladder carcinogenesis. EMBO Mol Med 2020;12:e10880. [Crossref] [PubMed]
- Chen H, Hu K, Xie Y, et al. CDK1 Promotes Epithelial-Mesenchymal Transition and Migration of Head and Neck Squamous Carcinoma Cells by Repressing ∆Np63α-Mediated Transcriptional Regulation. Int J Mol Sci 2022;23:7385. [Crossref] [PubMed]
- Wang Y, Wang F, He J, et al. miR-30a-3p Targets MAD2L1 and Regulates Proliferation of Gastric Cancer Cells. Onco Targets Ther 2019;12:11313-24. [Crossref] [PubMed]
- Lee YC, Huang CC, Lin DY, et al. Overexpression of centromere protein K (CENPK) in ovarian cancer is correlated with poor patient survival and associated with predictive and prognostic relevance. PeerJ 2015;3:e1386. [Crossref] [PubMed]
- Tian H, Wang F, Deng Y, et al. Centromeric protein K (CENPK) promotes gastric cancer proliferation and migration via interacting with XRCC5. Gastric Cancer 2022;25:879-95. [Crossref] [PubMed]
- Ma Y, Zha J, Yang X, et al. Long-chain fatty acyl-CoA synthetase 1 promotes prostate cancer progression by elevation of lipogenesis and fatty acid beta-oxidation. Oncogene 2021;40:1806-20. [Crossref] [PubMed]
- Zhang B, Zhang M, Li Q, et al. TPX2 mediates prostate cancer epithelial-mesenchymal transition through CDK1 regulated phosphorylation of ERK/GSK3β/SNAIL pathway. Biochem Biophys Res Commun 2021;546:1-6. [Crossref] [PubMed]
- Wang M, Qiao X, Cooper T, et al. HPV E7-mediated NCAPH ectopic expression regulates the carcinogenesis of cervical carcinoma via PI3K/AKT/SGK pathway. Cell Death Dis 2020;11:1049. [Crossref] [PubMed]
- Thein KH, Kleylein-Sohn J, Nigg EA, et al. Astrin is required for the maintenance of sister chromatid cohesion and centrosome integrity. J Cell Biol 2007;178:345-54. [Crossref] [PubMed]
- Chu X, Chen X, Wan Q, et al. Nuclear Mitotic Apparatus (NuMA) Interacts with and Regulates Astrin at the Mitotic Spindle. J Biol Chem 2016;291:20055-67. [Crossref] [PubMed]
- Canu V, Donzelli S, Sacconi A, et al. Aberrant transcriptional and post-transcriptional regulation of SPAG5, a YAP-TAZ-TEAD downstream effector, fuels breast cancer cell proliferation. Cell Death Differ 2021;28:1493-511. [Crossref] [PubMed]
- Li Z, Li H, Chen J, et al. SPAG5 promotes osteosarcoma metastasis via activation of FOXM1/MMP2 axis. Int J Biochem Cell Biol 2020;126:105797. [Crossref] [PubMed]
- Dang L, Shi C, Zhang Q, et al. Downregulation of sperm-associated antigen 5 inhibits melanoma progression by regulating forkhead box protein M1/A disintegrin and metalloproteinase 17/NOTCH1 signaling. Bioengineered 2022;13:4744-56. [Crossref] [PubMed]
- Liu J, Zhang Y, Zeng H, et al. Fe-doped chrysotile nanotubes containing siRNAs to silence SPAG5 to treat bladder cancer. J Nanobiotechnology 2021;19:189. [Crossref] [PubMed]
- Dai B, Zhang X, Shang R, et al. Blockade of ARHGAP11A reverses malignant progress via inactivating Rac1B in hepatocellular carcinoma. Cell Commun Signal 2018;16:99. [Crossref] [PubMed]
- Zheng L, Cai X, Song J, et al. MicroRNA-30c-2-3p represses malignant progression of gastric adenocarcinoma cells via targeting ARHGAP11A. Bioengineered 2022;13:14534-44. [Crossref] [PubMed]
- Yan B, Han M, Liu R. Rho GTPase-activating protein 11A (ARHGAP11A) is up-regulated in lung adenocarcinoma and positively associated with poor prognosis. Xi Bao Yu Fen Zi Mian Yi Xue Za Zhi 2021;37:596-601. [PubMed]
- Zheng P, Li L. FANCI Cooperates with IMPDH2 to Promote Lung Adenocarcinoma Tumor Growth via a MEK/ERK/MMPs Pathway. Onco Targets Ther 2020;13:451-63. [Crossref] [PubMed]
- Birkbak NJ, Li Y, Pathania S, et al. Overexpression of BLM promotes DNA damage and increased sensitivity to platinum salts in triple-negative breast and serous ovarian cancers. Ann Oncol 2018;29:903-9. [Crossref] [PubMed]
- Li Y, Zhang Y, Yang Q, et al. Silencing of FANCI Promotes DNA Damage and Sensitizes Ovarian Cancer Cells to Carboplatin. Curr Cancer Drug Targets 2022;22:591-602. [Crossref] [PubMed]
- Zhang J, Wang J, Wu J, et al. UBE2T regulates FANCI monoubiquitination to promote NSCLC progression by activating EMT. Oncol Rep 2022;48:139. [Crossref] [PubMed]
- Wang Y, Li JQ, Yang ZL, et al. NCAPH regulates gastric cancer progression through DNA damage response. Neoplasma 2022;69:283-91. [Crossref] [PubMed]
- Li B, Xiao Q, Shan L, et al. NCAPH promotes cell proliferation and inhibits cell apoptosis of bladder cancer cells through MEK/ERK signaling pathway. Cell Cycle 2022;21:427-38. [Crossref] [PubMed]
- Yu G, Wang LG, Han Y, et al. clusterProfiler: an R package for comparing biological themes among gene clusters. OMICS 2012;16:284-7. [Crossref] [PubMed]
- Otto T, Sicinski P. Cell cycle proteins as promising targets in cancer therapy. Nat Rev Cancer 2017;17:93-115. [Crossref] [PubMed]
- Williams GH, Stoeber K. The cell cycle and cancer. J Pathol 2012;226:352-64. [Crossref] [PubMed]
- Gonzalez S, Klatt P, Delgado S, et al. Oncogenic activity of Cdc6 through repression of the INK4/ARF locus. Nature 2006;440:702-6. [Crossref] [PubMed]
- Malumbres M, Barbacid M. Cell cycle, CDKs and cancer: a changing paradigm. Nat Rev Cancer 2009;9:153-66. [Crossref] [PubMed]
- Calcinotto A, Kohli J, Zagato E, et al. Cellular Senescence: Aging, Cancer, and Injury. Physiol Rev 2019;99:1047-78. [Crossref] [PubMed]
- Karabicici M, Alptekin S, Fırtına Karagonlar Z, et al. Doxorubicin-induced senescence promotes stemness and tumorigenicity in EpCAM-/CD133- nonstem cell population in hepatocellular carcinoma cell line, HuH-7. Mol Oncol 2021;15:2185-202. [Crossref] [PubMed]
- Lane D, Levine A. p53 Research: the past thirty years and the next thirty years. Cold Spring Harb Perspect Biol 2010;2:a000893. [Crossref] [PubMed]
- Bieging KT, Mello SS, Attardi LD. Unravelling mechanisms of p53-mediated tumour suppression. Nat Rev Cancer 2014;14:359-70. [Crossref] [PubMed]
- Cho YH, Ro EJ, Yoon JS, et al. 5-FU promotes stemness of colorectal cancer via p53-mediated WNT/β-catenin pathway activation. Nat Commun 2020;11:5321. [Crossref] [PubMed]
- Vousden KH, Prives C. Blinded by the Light: The Growing Complexity of p53. Cell 2009;137:413-31. [Crossref] [PubMed]
- Pietrucha BM, Heropolitańska-Pliszka E, Wakulińska A, et al. Ataxia-telangiectasia with hyper-IgM and Wilms tumor: fatal reaction to irradiation. J Pediatr Hematol Oncol 2010;32:e28-30. [Crossref] [PubMed]
- Renwick A, Thompson D, Seal S, et al. ATM mutations that cause ataxia-telangiectasia are breast cancer susceptibility alleles. Nat Genet 2006;38:873-5. [Crossref] [PubMed]
- Hall MJ, Bernhisel R, Hughes E, et al. Germline Pathogenic Variants in the Ataxia Telangiectasia Mutated (ATM) Gene are Associated with High and Moderate Risks for Multiple Cancers. Cancer Prev Res (Phila) 2021;14:433-40. [Crossref] [PubMed]
- Phan LM, Rezaeian AH. ATM: Main Features, Signaling Pathways, and Its Diverse Roles in DNA Damage Response, Tumor Suppression, and Cancer Development. Genes (Basel) 2021;12:845. [Crossref] [PubMed]
- El-Deiry WS. p21(WAF1) Mediates Cell-Cycle Inhibition, Relevant to Cancer Suppression and Therapy. Cancer Res 2016;76:5189-91. [Crossref] [PubMed]
- Hu CC, Liang YW, Hu JL, et al. LncRNA RUSC1-AS1 promotes the proliferation of breast cancer cells by epigenetic silence of KLF2 and CDKN1A. Eur Rev Med Pharmacol Sci 2019;23:6602-11. [PubMed]
- Zaremba-Czogalla M, Hryniewicz-Jankowska A, Tabola R, et al. A novel regulatory function of CDKN1A/p21 in TNFα-induced matrix metalloproteinase 9-dependent migration and invasion of triple-negative breast cancer cells. Cell Signal 2018;47:27-36. [Crossref] [PubMed]
- Zhao J, Shi L, Zeng S, et al. Importin-11 overexpression promotes the migration, invasion, and progression of bladder cancer associated with the deregulation of CDKN1A and THBS1. Urol Oncol 2018;36:311.e1-311.e13. [Crossref] [PubMed]
- Lei ST, Shen F, Chen JW, et al. MiR-639 promoted cell proliferation and cell cycle in human thyroid cancer by suppressing CDKN1A expression. Biomed Pharmacother 2016;84:1834-40. [Crossref] [PubMed]
- Ma JX, Yang YL, He XY, et al. Long noncoding RNA MNX1-AS1 overexpression promotes the invasion and metastasis of gastric cancer through repressing CDKN1A. Eur Rev Med Pharmacol Sci 2019;23:4756-62. [PubMed]
- Bi M, Yu H, Huang B, et al. Long non-coding RNA PCAT-1 over-expression promotes proliferation and metastasis in gastric cancer cells through regulating CDKN1A. Gene 2017;626:337-43. [Crossref] [PubMed]
- Liu Z, Sun M, Lu K, et al. The long noncoding RNA HOTAIR contributes to cisplatin resistance of human lung adenocarcinoma cells via downregualtion of p21(WAF1/CIP1) expression. PLoS One 2013;8:e77293. [Crossref] [PubMed]
- Zamagni A, Pasini A, Pirini F, et al. CDKN1A upregulation and cisplatin pemetrexed resistance in non small cell lung cancer cells. Int J Oncol 2020;56:1574-84. [Crossref] [PubMed]