Identification of the molecular mechanism and candidate markers for diabetic nephropathy
Highlight box
Key findings
• COL1A2 may be a potential biomarker for DN.
What is known and what is new?
• Immune inflammation and oxidative stress were involved in DN.
• COL1A2 may affect T cells and aDC, then subsequently participate in DN.
What is the implication, and what should change now?
• Identifying new predictive biomarkers will provide opportunities for prevention and/or treatment interventions. Future research focusing on COL1A2 is necessary.
Introduction
Diabetes mellitus (DM) is a systemic disease characterized by the inability of the body to produce or effectively respond to the glucose regulatory hormone, insulin (1). The resulting hyperglycemia can disrupt hemodynamics and metabolize homeostasis enzymes, leading to serious complications (2). Diabetic nephropathy (DN), a kind of microvascular complication, is the most common cause of end-stage renal disease (3). It is characterized by continuous proteinuria, a gradual decrease of the glomerular filtration rate, and increased blood pressure (4), and its diagnosis has always been based on the presence of microalbuminuria (MA) (5). However, MA does not adequately predict DN, especially in young patients or non-albuminuria patients. Therefore, new biomarkers are needed to identify individuals who will develop this life-threatening disease.
Oxidative stress and inflammation caused by chronic elevation of blood glucose in diabetic patients have increasingly been considered risk factors for DN (6). Cell and molecular experiments have shown that mitochondrial dysfunction, endoplasmic reticulum stress, and abnormal activation of the intracellular signaling pathway are closely related to DN (7). Without adequate oxygen supply, hyperglycemia may impair the stability of the hypoxia-inducible factor (HIF) and promote renal fibrosis (8). In both animal models and DN patients, increased infiltration of circulating inflammatory mediators and immune cells into renal tissue has been observed (9). Inflammation also plays an important role in the pathogenesis of DN, such as through cell adhesion molecules, growth factors, chemokines, and proinflammatory cytokines (10,11). A low dose of interleukin-17A (IL-17A) can prevent DN in type 1 and type 2 diabetic models (12). Although several effective treatments can delay the occurrence and development of DN, its incidence rate remains high.
DN is characterized by glomerular and tubulointerstitial damage (13). This study aimed to investigate the molecular mechanism of DN by bioinformatics analysis of the transcriptome data of renal tubules and glomeruli in DN. Identifying new predictive biomarkers will provide opportunities for prevention and/or treatment interventions. We present the following article in accordance with the STREGA reporting checklist (available at https://atm.amegroups.com/article/view/10.21037/atm-22-5128/rc).
Methods
Data collection and difference analysis
The gene expression profiles of glomeruli and renal tubules samples from DN donors and normal controls in the GSE30122 dataset were obtained from the Gene Expression Omnibus (GEO) database (https://www.ncbi.nlm.nih.gov/geo). This dataset included nine glomerular DN samples, 26 glomerular normal samples, 10 tubular DN samples, and 24 tubular normal samples. The demographic characteristics of the donors were shown as in Woroniecka et al. (14). There were obvious differences in the clinical indexes between DN patients and controls. The Affymetrix human genome U133A 2.0 array (GPL571) platform was used. The limma package was used to obtain the differentially expressed genes (DEGs) between DN and controls, and the conditions of |log2 fold change (FC)| ≥0.5, P<0.05 were used to screen the DEGs.
Co-expression analysis
The co-expression of the two groups of DEGs was analyzed through weighted gene co-expression network analysis (WGCNA). The co-expression similarity matrix of the genes was calculated, and the soft threshold was used to maintain the correlation between genes. The topological overlap difference was calculated from the adjacency matrix, and the hierarchical clustering method was used to define gene clusters, with each cluster representing a module. Using Pearson correlation, the first mock exam was associated with the characteristics of each module. The highly correlated genes in each module were classified as hub genes. The receiver operating characteristic (ROC) curve was drawn using the pROC package (15), and the area under the ROC curve (AUC) value of the hub genes was calculated.
Enrichment and Gene Set Enrichment Analysis (GSEA)
The ClusterProfiler package was used to analyze the Gene Ontology (GO) function and the Kyoto Encyclopedia of Genes and Genomes (KEGG) signaling pathway. A threshold value P<0.05 was set. KEGG pathways involved in the expression of genes in glomeruli and renal tubules were determined using GSEA method (16), and P<0.05 was set as the threshold.
Assessment of immune levels
The expression profiles of 24 immune cells (17) in the DN and control groups were calculated by single sample gene set enrichment analysis (ssGSEA) using GSVA package (18). The correlation was calculated by Pearson correlation analysis.
Ethical statement
The study was conducted in accordance with the Declaration of Helsinki (as revised in 2013).
Statistical analysis
All analyses in this study were performed using the Bioinforcloud platform (http://www.bioinforcloud.org.cn).
Results
Co-expression of DEGs
We analyzed the DEGs between the DN samples and the controls in the GSE30122 dataset. A total of 1,778 DEGs were selected from the glomeruli and 1,996 DEGs from the renal tubules (Figure 1A). These DEGs may be related to the pathogenesis of DN. Through co-expression analysis of the union genes of the two DEGs groups, 3,130 genes were identified in nine modules (Figure 1B,1C). Among them, the brown module was most highly correlated with the glomerular and tubular DN samples (Figure 1D). Each module may represent different molecular maladjustment mechanisms, and the up- and down-regulated genes were distributed in each module (Figure 1E,1F). In addition, there was considerable up-regulation of the expression proportion of DEGs in the brown module in the glomeruli or renal tubules (Figure 1G,1H). The hub genes within each module were identified (Table 1).
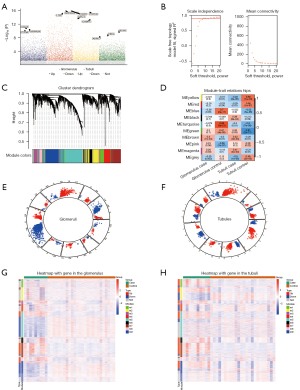
Table 1
Color | Hub genes | Module |
---|---|---|
Black | TM9SF2 | m6 |
Blue | NPTN | m9 |
Brown | COL1A2 | m7 |
Green | TRIM22 | m1 |
Magenta | TUBB | m3 |
Pink | SLC25A11 | m8 |
Red | SORD | m4 |
Turquoise | ARHGAP19 | m5 |
Yellow | ERF | m2 |
Biological functions and signaling pathways of the module genes
Enrichment analysis showed that the module genes participated in 6,769 biological processes (BP), 795 cell components (CC), 1,310 molecular functions (MF), and 212 KEGG signaling pathways (available online: https://cdn.amegroups.cn/static/public/atm-22-5128-1.xlsx). Among them, oxidative stress and autophagy were the main biological functions (Figure 2A). The phosphatidylinositol 3-kinase/protein kinase B (PI3K/AKT) and nucleotide-binding and oligomerization domain (NOD)-like receiver signaling pathways were the main signaling pathways (Figure 2B). In addition, by comparing the GSEA results, it was found that three signaling pathways were consistent with those in the renal tubules, and 15 signaling pathways were consistent with those in the glomeruli (Figure 2C,2D). These pathways may be closely related to the immune system.
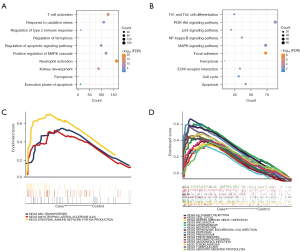
Module genes related to immune cells
In this study, the expression profiles of 24 immune cells were calculated by ssGSEA (Figure 3A,3B). Most of the immune cells were highly expressed in DN glomeruli, and B cells, activated dendritic cells (aDC), T cells, and T helper cells were highly expressed in the DN renal tubules. In addition, immune cells, especially T cells and aDC, were highly correlated (Figure 3C). T cells and B cells exhibited the strongest correlation in the glomeruli, while that between T cells and aDC was the strongest in the renal tubules (Figure 3D,3E). Among these, the brown module’s hub gene, collagen type I alpha 2 chain (COL1A2), was highly correlated with the glomerular T cells of DN and the tubular aDC (Figure 3F,3G). These results suggested that COL1A2 might affect T cells and aDC, and subsequently participate in DN.
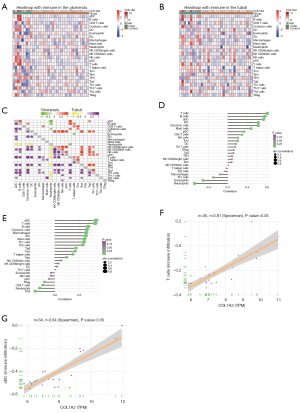
Biomarkers of DN
We found that COL1A2, SLC25A11, SORD, TRIM22, and TUBB exhibited the same expression trends in the glomeruli and renal tubules (Figure 4A,4B). To further identify the molecular markers of DN, we calculated the AUC values of the hub genes of the modules (Figure 4C). It was found that the AUC values of COL1A2 and TRIM22 were in the top 5 in both tissues (Figure 4D,4E). Based on our comprehensive analysis, we believed that COL1A2 exhibited better clinical diagnostic ability and may have potential as a biomarker of DN.
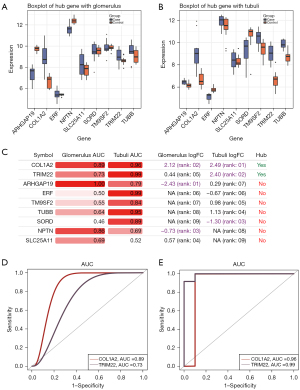
Discussion
This study explored the gene expression profiles of DN and control groups obtained from the GEO database and analyzed the DEGs. The number of DEGs in glomeruli and renal tubules was similar, and the distribution of modular genes in the two groups was also similar. It may be that both tissues have early damage in diabetes (19). We then performed WGCNA to investigate DN-associated co-expressed gene networks and identify the hub genes. Selective impairment of glomeruli and dysfunction of proximal renal tubule reabsorption contributes to the development of DN (20,21).
Enrichment analysis showed that most of the DEGs were enriched in the immune inflammatory response and oxidative stress. KEGG pathway analysis determined that the PI3K/Akt and NOD-like receiver signaling pathways were enriched. Inflammation played a key role in the progression of DN. Preclinical studies have identified several anti-inflammatory molecules that effectively reduce proteinuria and/or proteinuria (22). The study of a diabetic experimental animal model showed that oxidative stress was the main determinant of DN pathophysiology (23). Activation of the PI3K/Akt signaling pathway could promote the development of DN while blocking this pathway seemed to improve DN (24). The intracellular NOD-like receptor comprises an inflammatory body, which could activate and release caspase-1, IL-1β, and IL-18, thereby causing an inflammatory reaction, which is closely related to the occurrence and development of DN (25,26). Therefore, the DEGs in this study may participate in the DN progression through these biological functions and signaling pathways.
Numerous immune cells play an important role in DN, including key members of the innate and adaptive immune systems. In cases of severe DN, the renal infiltration of T cells, neutrophils, and macrophages increases (27). Moreover, the accumulation of macrophages or T cells has been confirmed in both a human DN model and a rodent model (28). Dendritic cells (DCs) are the key mediators of immune and antigen-specific immune tolerance (29). The change of specific DC subsets in the cornea may be an early indicator of immune activation in DM, and its function is also closely related to the DN progression (30,31). At present, the role of B cells in the pathogenesis of DN is not clear. The most likely mechanism for B cell participation in DN is through the production of antibodies, the formation of immune complexes, and depositing in the kidney via complement activation, leading to inflammation and glomerulonephritis (32).
Importantly, we identified nine pivotal genes, among which the brown module of COL1A2 was the most closely correlated with DN, T cells and aDC. COL1A2 showed a good diagnostic ability in the glomeruli and renal tubules. Previous studies have shown that COL1A2 is involved in renal tubulointerstitial injury in diabetes (33). Also, the high expression of COL1A2 promotes the differentiation of fibroblasts (34). In the process of tubulointerstitial fibrosis in DN, there is increased production of type I collagen, which is the main component of extracellular matrix (35). It has been suggested that the high expression of COL1A2 may promote the occurrence and development of DN through inflammation and fibrosis (36-38). In addition, TRIM22 affects the inflammation-related signaling pathway and participates in several diseases (39). The increased expression of TRIM22 in monocyte macrophages treated with high glucose may be related to DN induced by high glucose (40). Therefore, COL1A2 and TRIM22 are expected to be a new research target and provide a new direction for the diagnosis and treatment of DN.
Our study also has limitations that should be noted. Firstly, the sample size used for analyzing was too small to allow for generalization of the results, a larger number of samples will be used in future. Also, we should conduct relevant experimental tests to determine the potential biological role of the pathways in DN. Additionally, experiments to study the detailed molecular mechanism and biological function of the COL1A2 will performed in future. Finally, in follow-up studies, we should expand the experimental samples to verify the potential diagnostic effects of the hub genes on DN.
In conclusion, the biological functions through which DEGs participate may play a regulated role in DN. COL1A2, which was identified by the co-expression network, may be a potential biomarker of DN. Therefore, future research focusing on COL1A2 is necessary.
Conclusions
The molecular mechanisms of renal tubules and glomeruli in DN are similar and are related to immune inflammation and oxidative stress. T cells, B cells, and aDC play an important role in the immune process. Importantly, we determined that COL1A2 might serve as a potential biomarker of DN.
Acknowledgments
Funding: None.
Footnote
Reporting Checklist: The authors have completed the STREGA reporting checklist. Available at https://atm.amegroups.com/article/view/10.21037/atm-22-5128/rc
Conflicts of Interest: All authors have completed the ICMJE uniform disclosure form (available at https://atm.amegroups.com/article/view/10.21037/atm-22-5128/coif). The authors have no conflicts of interest to declare.
Ethical Statement: The authors are accountable for all aspects of the work in ensuring that questions related to the accuracy or integrity of any part of the work are appropriately investigated and resolved. The study was conducted in accordance with the Declaration of Helsinki (as revised in 2013).
Open Access Statement: This is an Open Access article distributed in accordance with the Creative Commons Attribution-NonCommercial-NoDerivs 4.0 International License (CC BY-NC-ND 4.0), which permits the non-commercial replication and distribution of the article with the strict proviso that no changes or edits are made and the original work is properly cited (including links to both the formal publication through the relevant DOI and the license). See: https://creativecommons.org/licenses/by-nc-nd/4.0/.
References
- Tomic D, Shaw JE, Magliano DJ. The burden and risks of emerging complications of diabetes mellitus. Nat Rev Endocrinol 2022;18:525-39. [Crossref] [PubMed]
- Ehtewish H, Arredouani A, El-Agnaf O. Diagnostic, Prognostic, and Mechanistic Biomarkers of Diabetes Mellitus-Associated Cognitive Decline. Int J Mol Sci 2022;23:6144. [Crossref] [PubMed]
- Erekat NS. Programmed Cell Death in Diabetic Nephropathy: A Review of Apoptosis, Autophagy, and Necroptosis. Med Sci Monit 2022;28:e937766. [Crossref] [PubMed]
- Saini DC, Kochar A, Poonia R. Clinical correlation of diabetic retinopathy with nephropathy and neuropathy. Indian J Ophthalmol 2021;69:3364-8. [Crossref] [PubMed]
- Heyman SN, Raz I, Dwyer JP, et al. Diabetic Proteinuria Revisited: Updated Physiologic Perspectives. Cells 2022;11:2917. [Crossref] [PubMed]
- Lindblom R, Higgins G, Coughlan M, et al. Targeting Mitochondria and Reactive Oxygen Species-Driven Pathogenesis in Diabetic Nephropathy. Rev Diabet Stud 2015;12:134-56. [Crossref] [PubMed]
- Lin YC, Chang YH, Yang SY, et al. Update of pathophysiology and management of diabetic kidney disease. J Formos Med Assoc 2018;117:662-75. [Crossref] [PubMed]
- Sun HJ, Wu ZY, Cao L, et al. Hydrogen Sulfide: Recent Progression and Perspectives for the Treatment of Diabetic Nephropathy. Molecules 2019;24:2857. [Crossref] [PubMed]
- Donate-Correa J, Luis-Rodríguez D, Martín-Núñez E, et al. Inflammatory Targets in Diabetic Nephropathy. J Clin Med 2020;9:458. [Crossref] [PubMed]
- Huang C, Zhang L, Shi Y, et al. The KCa3.1 blocker TRAM34 reverses renal damage in a mouse model of established diabetic nephropathy. PLoS One 2018;13:e0192800. [Crossref] [PubMed]
- Hu HC, Lei YH, Zhang WH, et al. Antioxidant and Anti-inflammatory Properties of Resveratrol in Diabetic Nephropathy: A Systematic Review and Meta-analysis of Animal Studies. Front Pharmacol 2022;13:841818. [Crossref] [PubMed]
- Mohamed R, Jayakumar C, Chen F, et al. Low-Dose IL-17 Therapy Prevents and Reverses Diabetic Nephropathy, Metabolic Syndrome, and Associated Organ Fibrosis. J Am Soc Nephrol 2016;27:745-65. [Crossref] [PubMed]
- Wilson PC, Wu H, Kirita Y, et al. The single-cell transcriptomic landscape of early human diabetic nephropathy. Proc Natl Acad Sci U S A 2019;116:19619-25. [Crossref] [PubMed]
- Woroniecka KI, Park AS, Mohtat D, et al. Transcriptome analysis of human diabetic kidney disease. Diabetes 2011;60:2354-69. [Crossref] [PubMed]
- Robin X, Turck N, Hainard A, et al. pROC: an open-source package for R and S+ to analyze and compare ROC curves. BMC Bioinformatics 2011;12:77. [Crossref] [PubMed]
- Subramanian A, Tamayo P, Mootha VK, et al. Gene set enrichment analysis: a knowledge-based approach for interpreting genome-wide expression profiles. Proc Natl Acad Sci U S A 2005;102:15545-50. [Crossref] [PubMed]
- Bindea G, Mlecnik B, Tosolini M, et al. Spatiotemporal dynamics of intratumoral immune cells reveal the immune landscape in human cancer. Immunity 2013;39:782-95. [Crossref] [PubMed]
- Hänzelmann S, Castelo R, Guinney J. GSVA: gene set variation analysis for microarray and RNA-seq data. BMC Bioinformatics 2013;14:7. [Crossref] [PubMed]
- Van JA, Scholey JW, Konvalinka A. Insights into Diabetic Kidney Disease Using Urinary Proteomics and Bioinformatics. J Am Soc Nephrol 2017;28:1050-61. [Crossref] [PubMed]
- Liu F, Brezniceanu ML, Wei CC, et al. Overexpression of angiotensinogen increases tubular apoptosis in diabetes. J Am Soc Nephrol 2008;19:269-80. [Crossref] [PubMed]
- Bonventre JV. Can we target tubular damage to prevent renal function decline in diabetes? Semin Nephrol 2012;32:452-62. [Crossref] [PubMed]
- Moreno JA, Gomez-Guerrero C, Mas S, et al. Targeting inflammation in diabetic nephropathy: a tale of hope. Expert Opin Investig Drugs 2018;27:917-30. [Crossref] [PubMed]
- Sagoo MK, Gnudi L. Diabetic nephropathy: Is there a role for oxidative stress? Free Radic Biol Med 2018;116:50-63. [Crossref] [PubMed]
- Ni WJ, Guan XM, Zeng J, et al. Berberine regulates mesangial cell proliferation and cell cycle to attenuate diabetic nephropathy through the PI3K/Akt/AS160/GLUT1 signalling pathway. J Cell Mol Med 2022;26:1144-55. [Crossref] [PubMed]
- Wada J, Makino H. Innate immunity in diabetes and diabetic nephropathy. Nat Rev Nephrol 2016;12:13-26. [Crossref] [PubMed]
- Jin J, Zhou TJ, Ren GL, et al. Novel insights into NOD-like receptors in renal diseases. Acta Pharmacol Sin 2022;43:2789-806. [Crossref] [PubMed]
- Wang X, Yao B, Wang Y, et al. Macrophage Cyclooxygenase-2 Protects Against Development of Diabetic Nephropathy. Diabetes 2017;66:494-504. [Crossref] [PubMed]
- Shahzad K, Bock F, Dong W, et al. Nlrp3-inflammasome activation in non-myeloid-derived cells aggravates diabetic nephropathy. Kidney Int 2015;87:74-84. [Crossref] [PubMed]
- Obregon C, Kumar R, Pascual MA, et al. Update on Dendritic Cell-Induced Immunological and Clinical Tolerance. Front Immunol 2017;8:1514. [Crossref] [PubMed]
- Angulo JA, Davis LG, Burkhart BA, et al. Reduction of striatal dopaminergic neurotransmission elevates striatal proenkephalin mRNA. Eur J Pharmacol 1986;130:341-3. [Crossref] [PubMed]
- Zheng Z, Zheng F. Immune Cells and Inflammation in Diabetic Nephropathy. J Diabetes Res 2016;2016:1841690. [Crossref] [PubMed]
- Smith MJ, Simmons KM, Cambier JC. B cells in type 1 diabetes mellitus and diabetic kidney disease. Nat Rev Nephrol 2017;13:712-20. [Crossref] [PubMed]
- Zeng M, Liu J, Yang W, et al. Multiple-microarray analysis for identification of hub genes involved in tubulointerstial injury in diabetic nephropathy. J Cell Physiol 2019; Epub ahead of print. [Crossref] [PubMed]
- Papaioannou I, Xu S, Denton CP, et al. STAT3 controls COL1A2 enhancer activation cooperatively with JunB, regulates type I collagen synthesis posttranscriptionally, and is essential for lung myofibroblast differentiation. Mol Biol Cell 2018;29:84-95. [Crossref] [PubMed]
- Xu H, Wu X, Qin H, et al. Myocardin-Related Transcription Factor A Epigenetically Regulates Renal Fibrosis in Diabetic Nephropathy. J Am Soc Nephrol 2015;26:1648-60. [Crossref] [PubMed]
- Das F, Ghosh-Choudhury N, Maity S, et al. Oncoprotein DJ-1 interacts with mTOR complexes to effect transcription factor Hif1α-dependent expression of collagen I (α2) during renal fibrosis. J Biol Chem 2022;298:102246. [Crossref] [PubMed]
- Chen J, Luo SF, Yuan X, et al. Diabetic kidney disease-predisposing proinflammatory and profibrotic genes identified by weighted gene co-expression network analysis (WGCNA). J Cell Biochem 2022;123:481-92. [Crossref] [PubMed]
- Riser BL, Najmabadi F, Garchow K, et al. Treatment with the matricellular protein CCN3 blocks and/or reverses fibrosis development in obesity with diabetic nephropathy. Am J Pathol 2014;184:2908-21. [Crossref] [PubMed]
- Zhang L, Zhang B, Wei M, et al. TRIM22 inhibits endometrial cancer progression through the NOD2/NF-κB signaling pathway and confers a favorable prognosis. Int J Oncol 2020;56:1225-39. [Crossref] [PubMed]
- Miao F, Chen Z, Zhang L, et al. RNA-sequencing analysis of high glucose-treated monocytes reveals novel transcriptome signatures and associated epigenetic profiles. Physiol Genomics 2013;45:287-99. [Crossref] [PubMed]
(English Language Editor: A. Kassem)