Effects of short-term waterfall forest aerosol air exposure on rat lung proteomics
Highlight box
Key findings
• The aerosol environment in the forest of Huangguoshu Waterfall has the presence of negative oxygen ions that are beneficial to human health.
What is known and what is new?
• Short-term (≤10 days) exposure to the Huangguoshu Waterfall forest environment can reduce lung microinflammation and excessive oxidative stress;
• Longer (≥15 days) exposure to the Huangguoshu Waterfall forest environment can balance the immune environment of the lungs and reduce the chance of allergies.
What is the implication, and what should change now?
• Uqcrfs1, Tufm and Rpl4 may be biological targets relevant to the evaluation of ambient air quality.
Introduction
Environmental particulate matter (PM) pollution has been proven to lead to several adverse cardiopulmonary effects, for instance, inflammation, reduced lung function, disruption of cardiac autonomic function, and increased oxidation (1,2). Many nations, including China, have made great measures to control emissions from a wide range of sources due to the significant negative health effects of ambient particulate pollution. As a result, ambient air pollutant levels in China have been significantly reduced over the past few years (3). However, air pollution levels in urban areas remain high, leading to an increased incidence of chronic diseases in the population. High-efficiency particulate air (HEPA) purifiers are regarded as an effective tool to reduce indoor PM levels and improve the cardiorespiratory health of occupants. This effect is achieved mainly through the production of negative oxygen ions by the apparatus (4,5). A study has suggested that negative air ions have “mysterious” health benefits, such as promoting physiological health and boosting the immune system (6). Indeed, a study has indicated significant health benefits associated with short-term exposure to high levels of negative ions (NIs) (7). Humidity is higher in natural environments compared to urban environments. In a forest environment with large waterfalls, the water molecules are cleaved by the waterfall drop to produce more abundant negative oxygen ions, which interact with the phytocide released from the surrounding vegetation to create a specific aerosol environment. Large waterfalls and the surrounding unique microenvironment have been shown to increase the levels of interleukin (IL)-10 and regulate the role of T cells, which can assist in the treatment of allergic asthma and improve the body’s immune function (8,9). Nevertheless, the protective mechanism of the waterfall forest aerosol environment on the lungs remains to be fully elucidated.
To date, no relevant publications have assessed whether a protective effect on the lungs can be achieved by short-term recuperation vacations in the waterfall forest environment. Therefore, the overall proteomic changes in the lungs associated with short-term waterfall forest aerosol environment exposure should be investigated. Data-independent acquisition mass spectrometry (DIA-MS) has become a crucial technique for quantitative proteomics in decades (10,11). Data-independent acquisition (DIA) provides more in-depth data coverage in a shorter analysis time compared to shotgun proteomics in data-dependent acquisition (DDA) mode. The data acquired through this approach represent higher precision, fewer missing values, and better reproducibility.
In the current study, 8-week-old male Sprague Dawley (SD) rats were placed in a waterfall forest aerosol environment or a conventional urban environment, with identical feeding conditions. A comprehensive proteomic analysis of the lungs of the different groups of rats was performed using the DIA-MS method. Time course and pairwise comparative analysis of protein abundance was performed for all samples at each time point. The physiological or biological processes (BPs) of the differentially abundant proteins (DAPs) were examined to screen for significantly altered proteins. This study is the first to explore the mechanisms involved in the regulation of proteins in rat lungs by short-term exposure to excellent air quality. We present the following article in accordance with the ARRIVE reporting checklist (available at https://atm.amegroups.com/article/view/10.21037/atm-22-4813/rc).
Methods
Experimental groups
Healthy adult male SD rats (weighing 180–200 g) were purchased from the Animal Research Center of Central South University. After 1 week of acclimatization feeding, rats were randomly divided into the following 6 groups: a normal environment 5-day group (N5; n=10), a normal environment 10-day group (N10; n=10), a normal environment 15-day group (N15; n=10), a waterfall forest aerosol 5-day group (W5; n=10), a waterfall forest aerosol 10-day group (W10; n=10), and a waterfall forest aerosol 15-day group (W15; n=10). The rats in each group were housed in 2 cages, with 5 rats per cage. Rats in cage 1 were numbered 1 to 5 and rats in cage 2 were numbered 6 to 10 (by applying picric acid markings on the rat tails). Animal experiments were performed under a project license (No. 1901001) granted by the Experimental Animal Ethics Committee of Guizhou Provincial People’s Hospital, in compliance with institutional guidelines for the care and use of animals. A protocol was prepared before the study without registration. All rats were given free access to food and water. At the end of the experimental time points, rats were anesthetized with 10% chloral hydrate (0.5 mL/mg), the thoracic cavity was opened, and the lung tissues were harvested. Figure 1 shows the specific experimental procedure. The lung samples were frozen in liquid nitrogen and stored at −80 ℃ for subsequent analyses.
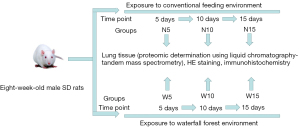
Study sites
Experiments on control rats were conducted in Guiyang City, Guizhou Province, China (106°17′E, 26°11′N). Experimental group rats were kept in the core scenic area of Huangguoshu Waterfall, Anshun, Guizhou Province, China (150°40′E, 25°59.5′N). The experiments were conducted between August 9 and August 25, 2019. The different environmental conditions are shown in Figure 2. Environmental measurements were performed using a multi-pollutant concentration logger (QDM1, Beijing Green Building Environment Technology Co., Ltd.) to measure temperature, relative humidity, real-time indoor concentrations of PM2.5 and carbon dioxide (CO2). NI concentrations were measured by using a NI detector (ONETEST KEC900, Wanyi Technology Co., Ltd.). The validity period for these monitors, which were calibrated before the first filtration, encompassed the whole study period. Indoor concentrations of total volatile organic compounds (TVOC), benzene, and toluene were measured by off-line gas chromatography-mass spectrometry (MS) analysis via a GSP-400 FT sampling pump, collecting air samples onto Tenax sorbent tubes. Real-time concentrations of air pollutants, including PM2.5, PM10, carbon monoxide (CO), sulfur dioxide (SO2), nitrogen dioxide (NO2), ozone (O3), and weather parameter data (ambient air temperature, relative humidity, atmospheric pressure, and wind speed) are detailed for each environment (Table 1).
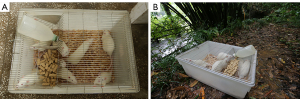
Table 1
Environmental parameters | Laboratory environment | Waterfall forest environment | P value |
---|---|---|---|
PM2.5, µg/m3 | 37.27±12.18 | 13.06±6.39 | <0.001 |
PM10, µg/m3 | 56.67±11.96 | 19.47±5.53 | <0.001 |
Negative ions, count/cm3 | 281.20±78.56 | 23,284.13±8,506.69 | <0.001 |
O3, µg/m3 | 57.20±11.53 | 44.08±8.23 | 0.0012 |
CO, µg/m3 | 1.08±0.23 | 0.61±0.31 | <0.001 |
SO2, µg/m3 | 4.52±1.42 | 2.30±1.10 | <0.001 |
NO2, µg/m3 | 35.20±7.36 | 21.00±6.00 | <0.001 |
Temperature, ℃ | 26.55±1.95 | 26.32±2.17 | 0.76 |
Relative humidity, % | 77.60±4.12 | 78.87±6.02 | 0.51 |
Wind speed, m/s | 1.94±0.39 | 2.10±0.42 | 0.37 |
Data are presented as mean ± standard error. PM, particulate matter.
Methods of prepared rat lung protein samples
Lung tissue samples were sonicated and boiled for 15 minutes. After centrifugation at 14,000 ×g for 40 minutes, total protein concentration in the supernatant was quantified using a BCA protein analysis kit (Bio-Rad, California, USA). Samples were stored at −80 ℃ until further analyses. Aliquots of each rat lung sample from this experiment were mixed into one sample for DDA library generation and quality control.
Protein digestion was conducted based on the filter-assisted sample preparation (FASP) procedure. Briefly, 200 mg of protein was incorporated into 30 mL of SDT [4% sodium dodecyl sulfate (SDS), 100 mmol/L, Tris-HCl, pH7.6] buffer. Detergents, dithiothreitol (DTT), and other low molecular weight components were removed by repeated ultrafiltration (Microcon device, 30 kDa) urea (UA) buffer (8 M urea, 150 mM Tris-HCl pH 8.0). Iodoacetamide (0.05 M, 100 mL) was added to UA buffer to block the reduced cysteine residues. Samples were then incubated in the dark for 30 minutes. The filters were washed three times with 100 mL of UA buffer, followed by two washes with 100 mL 25 mM NH4HCO3. The protein solution was then digested with 2 mg of trypsin (Promega) in 40 L of 100 mM NH4HCO3 buffer overnight at 37 ℃. Filtrates were collected from the produced peptides. The peptide content was estimated by UV (8 M urea, 150 mM Tris-HCl, pH 8.0) spectral density at 280 nm. By using a high pH reverse phase peptide fractionation kit (Thermo-Scientific), the digestion pool peptides were subsequently fractionated into 10 fractions. Each fraction was vacuum centrifuged for concentration, then reconstituted in 15 µL of 0.1% (v/v) formic acid. Collected peptides were desalted with a C18 cartridge (Empore) on a strong cation exchange solid-phase extraction (SPE) column C18 standard density, bed ID 7 mm, volume 3 mL, sigma) and reconstituted in 40 µL 0.1% (v/v) formic acid. The iRT kit (Zurich, Switzerland, Biognosys) was added to correct for the relative retention time difference between the iRT standard peptide and the sample peptide in a volume ratio of 1:3 for the run.
DDA MS analysis
All fractions generated from the DDA library were injected into a Thermo Scientific Q Extractive HF mass spectrometer connected to an Easy nLC 1200 chromatography system (Massachusetts, USA, Thermo Scientific). The peptides (2 mg) were loaded onto an EASY SprayTM C18 trap column (Thermo Scientific, P/N 164946, 3 mm, 75 mm × 2 cm), and then separated on an EASYSprayTM C18 LC analytical column (Thermo Scientific, ES803, 2 mm, 75 mm × 50 cm) with a gradient flow rate of 250 nL/min over 120 minutes using buffer B (80% acetonitrile and 0.1% formic acid). MS detection was positive ion with a scan range of 300–1,650 m/z, MS1 scan resolution of 60,000 at 200 m/z, automatic gain control (AGC) target of 3e6 with a maximum value of 25 MS, and a dynamic exclusion of 30.0 seconds. Each complete mass spectrometry-selected ion monitoring (MS-SIM) scan was followed by 20 ddMS2 scans. The MS2 scan had a resolution of 15,000, an AGC target of 5e4, a maximum resolution of 25 ms, and a normalized collision energy of 27 eV. Mass spectrometric analysis of DIA sample peptides was performed by liquid chromatograph-mass spectrometer (LC-MS)/MS in DIA mode (Shanghai Applied Protein Technology Co., Ltd.). Each DIA cycle consisted of one full MS-SIM scan with 30 DIA scans covering the mass range of 350–1,650 m/z with the following settings: full SIM scan resolution of 60,000 at 200 m/z, AGC 3e6, maximum IT 50 MS, profile mode. DIA scan resolution was set to 30,000, AGC target 3e6, maximum IT auto, and normalized collision energy of 30 eV. The running time was set as 120 minutes with a linear gradient of buffer B (80% acetonitrile and 0.1% formic acid) and a flow rate of 250 nL/min. At the beginning of the MS study and after every fifth injection throughout the experiment, quality control (QC) samples (from each of the experimental aliquot of the sample collected) were injected to monitor the MS performance in DIA mode.
MS data analysis
For the DDA library data, the FASTA sequence database was employed by using MaxQuant software (version 1.5.3.17). The database was downloaded from http://www.uniprot.org and the iRT peptide sequences were added (>Biognosys/iRT Kit/Sequence/Fusion). The parameters were set as follows: enzyme was trypsin, maximum number of deletion breaks was 2, fixed modification was aminomethyl (C), and dynamic modification was oxidative modification (M) and acetyl modification (protein N-terminus). All reported data are based on false discovery rate [FDR = N(decoy)*2/(N(decoy) + N(target)] determined with 99% confidence ≤1% for protein identification. Spectral libraries were constructed by inputting the original raw files and DDA search results into Spectronaut Pulsar XTM_12.0.20491.4 (Biognosys). DIA data were analyzed by searching the above-constructed spectral libraries through Spectronaut Pulsar XTM. The primary program settings were shown as follows: retention time prediction type was dynamic iRT, interference with MS2 level correction was enabled, and cross-run normalization was enabled. All results were filtered according to a Q cutoff value of 0.01 (corresponding to FDR <1%). All samples were classified by hierarchical clustering analysis of differentially expressed proteins (http://www.uniprot.org). The differentially expressed proteins were then analyzed for Gene Ontology (GO) by Blast2GO (https://www.blast2go.com/) and matched to the Kyoto Encyclopedia of Genes and Genomes (KEGG) database using the KEGG Automatic Annotation Server (KAAS) (https://www.genome.jp/tools/kaas/) to determine their relationship to the KEGG database. A P value <0.05 obtained with the Fisher’s exact test was considered statistically significant.
Weighted gene co-expression network analysis (WGCNA) co-expression module analysis
The different environmental exposures associated with different number of days-related weighted co-expression network was constructed using the “WGCNA” package of R (6), and samples with expression less than 0.5 were removed, then the scale-free distribution topology matrix was calculated, and the optimal soft threshold β was selected using the “pickSoftThreshold” function. The best soft threshold β was selected using the “pickSoftThreshold” function, and the Pearson correlation coefficients of each gene were calculated, and the weighted correlation coefficients were used to construct the adjacency matrix. The adjacency matrix was then converted into a topological overlap matrix, which was used to construct a clustering tree. The modules with the number of genes greater than 50 were retained, and the modules with similarity greater than 0.25 were merged. The optimal module was selected according to the expression differences of the normal group in the SANFH group. Using the grouping as a trait, the association between co-expression modules and trait was assessed. The proteins in the selected modules of interest were subjected to GO/KEGG enrichment analysis to determine the functional/pathway enrichment of the co-expressed proteins in the module, reflecting the protein functional/pathway level characteristics of the module.
Hematoxylin-eosin (HE) staining
Rat lung tissues were fixed in 10% formaldehyde solution, placed in embedding boxes, paraffin-embedded, and cut into blocks with 1.5 cm × 1.5 cm × 4 µm. Slides were stained with hematoxylin and eosin, and images were visualized and analyzed.
Immunohistochemical (IHC) staining
Rat lung tissues were placed in an oven at 60 ℃ for 24 hours. Sections were deparaffinized, dehydrated in alcohol, and washed three times with phosphate-buffered saline. Thereafter, sections were boiled with 0.01 M sodium citrate buffer for antigen retrieval and blocked with 5% bovine serum albumin (BSA). The following primary polyclonal antibodies were used: rabbit anti-rat ubiquinol-cytochrome C reductase iron-sulfur subunit 1 (Uqcrfs1) antibody (1:50; Abcam, MA, USA), rabbit anti-rat mitochondrial Tu translation elongation factor (Tufm) antibody (1:100; Abcam, MA, USA), and rabbit anti-rat ribosomal protein L4 (Rpl4) antibody (1:100; Abcam, MA, USA). Sections were then incubated with the corresponding secondary antibody (1:100; Abcam, MA, USA) for 1 hour at room temperature. Finally, DAB (3,3'-diaminobenzidine) and hematoxylin staining were performed. Uqcrfs1, Tufm, and Rpl4 positive regions were observed using an Olympus BX53 fluorescence microscope (Tokyo, Japan).
Statistical analysis
The SPSS (IBM, version 23.0, USA) software was used for statistical analysis and one-way analysis of variance (ANOVA) was performed. All data are expressed as mean ± standard error. Differences were assumed to be statistically significant when P<0.05.
Results
Histological changes in rat lung tissues in different environments
HE staining revealed that there was little difference in the lung tissues of rats in the urban environment and those in the waterfall forest aerosol environment (Figure 3), suggesting that different environmental exposures did not significantly affect the lung tissue structure in the short term.
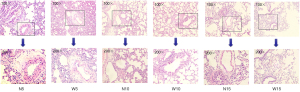
Identification of rat lung proteomes
All rat lung samples were used to build the DIA library database for this project using the DDA MS data acquisition method. A total of 9,398 proteins were identified in 18 samples. Follow-up biological and statistical analyses were performed to ensure validity and accuracy. The correlation coefficients of the QC samples indicated the stability of the overall experimental operation and the reliability of the test results. In this study, proteins that were found to be at constant levels in >50% of the samples were selected for subsequent statistical and bioinformatic analysis. Each sample in the project was prepared independently, proteolyzed, and then subjected to separate DIA analysis. The resulting DIA raw files were imported into Spectronaut Pulsar X for analysis, yielding a total qualitative and quantitative number of 5,355 proteins and 32,735 peptides for all samples. The number of differential proteins in the different environments for varying durations was compared (Figure 4).
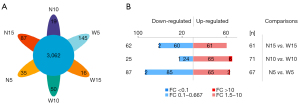
Differential protein analysis
The Hierarchy Cluster algorithm (HCA) was used to cluster the analysis of different groups of differentially expressed proteins and the data are displayed as heatmaps shown in Figure 4. Hierarchical clustering analysis of proteins in different real environments at the same time points detected the greatest number of differential proteins between the N5 and W5 groups. This suggested that short-term exposure to a waterfall aerosol environment may have modulatory effects on protein expression in the rat lung.
Heat maps of proteins at different exposure times
GO functional annotation was performed to elucidate the protein function in this study. The differentially expressed proteins were most enriched in defense responses, enzyme inhibitor activity, and extracellular space in the BP, molecular functions (MFs), and cellular components (CCs) categories, respectively.
The differentially expressed proteins between the N5 and W5 groups were enriched in the mitochondrial respiratory chain, mitochondrial cytosol, and other components in BPs. The differentially expressed proteins between the N10 and W10 groups were mainly involved in the contractile activity of myosin, while the differentially expressed proteins between the N15 and W15 groups were enriched in phosphatase activity and ATPase binding in the MFs category (Figure 5).
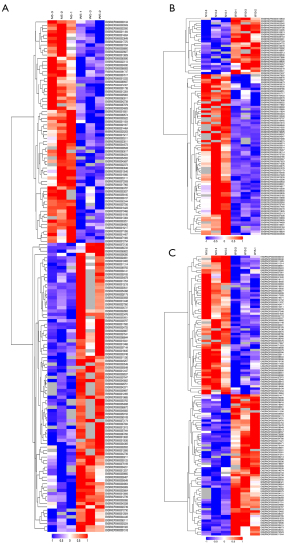
GO analysis and enrichment analysis
KEGG analysis of the differentially expressed proteins revealed that the differential proteins between the N5 and W5 groups are mainly involved in signaling pathways such as oxidative phosphorylation, endocrine signaling, and glycan degradation (Figure 6). The differential proteins between the N10 and W10 groups are mainly involved in sphingolipid signaling pathways and hormone signaling pathways. The differential proteins between the N15 and W15 groups are mainly involved in the immune-related high-affinity IgE receptor pathway (Figure 7).
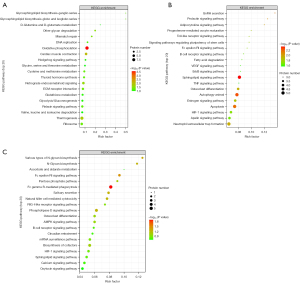
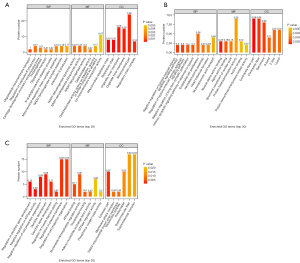
Protein-protein interaction (PPI) analysis of the differentially expressed proteins
In the N5 vs. W5 group, there were 91 proteins involved in protein interactions in rat lung proteins.48 of the differentially expressed proteins in the N10 vs. W10 group were involved in protein interactions. 64 of the proteins in the N15 vs. W15 group were involved in protein interactions The node with ≥10 degrees was selected as the reference condition to identify the key core targets in the network. Uqcrfs1, Tufm, and Rpl4 were obtained as possible biological targets related to the evaluation of air quality (Figure 8).
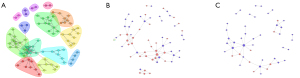
The expression and distribution of screening proteins in the rat lung as detected by IHC
Uqcrfs1 and Rpl4 were expressed in bronchial epithelial cells in the N15 group and stained weakly positive, while Uqcrfs1 expression was decreased in the waterfall forest environment. The expression of Tufm was elevated after exposure to the waterfall forest environment (Figure 9).
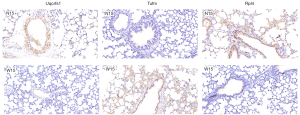
WGCNA
Hierarchical clustering analysis based on a weighting algorithm was performed and the clustering results were divided according to a set soft threshold and different groupings to obtain different genetic modules. The WGCNA analysis module phenotypes have been correlated by different groupings (Figure 10A).

The greatest number of protein differences was observed on day 5 after exposure to the different environments. WGCNA focused on the phenotypic modules after 5 days of environmental exposure. A total of 161 genes in these 2 modules were compared and 203 reciprocal targets and 20 duplicates were found. Interaction networks were constructed through the STRING database (Figure 10B). Enrichment analysis of the KEGG pathways revealed that the targets were mainly enriched in the ribosomal signaling pathway. Therefore, it is possible that these 20 targets may be involved in the short-term protection of the lungs by waterfall forest aerosols.
Discussion
With the rapid development of the global economy, the population is gradually concentrated in medium and large cities, and the urbanization process is accelerating (12). This has led to a series of problems that threaten the health of urban dwellers, the most prominent of which is air pollution (13). PM, such as fossil fuel, diesel exhaust particles, and carbon black (CB) from incomplete combustion from diesel vehicles and cars, are the main components that make up urban air pollution. Fine PM air pollution poses an even greater threat to human health than dust storms (14). Outside of the human nose, particles with a particle size of 10 µm or more will be prevented. However, particles between 2.5 and 10 µm in size can get into the upper respiratory tract. There is relatively little harm to human health from some of the particles because they can be eliminated through sputum or blocked by the villi in the nasal cavity. Fine particles with a particle size below 2.5 µm (PM2.5), such as aerosols with an aerodynamic equivalent diameter ≤2.5 µm, are difficult to block since their diameter is equivalent to one-tenth of a human hair. These can be very harmful to human health and the environment (15), and have been associated with an increased risk of depression in exposed populations (16). In addition, PM2.5 can absorb a large amount of heavy metal, polycyclic aromatic hydrocarbons, and other harmful chemicals on its surface. It can enter the deep lung tissues with breathing, and the water-soluble components or insoluble nanoparticles it carries can reach other extra-pulmonary organs through blood circulation via capillaries in the lungs (17-19). Epidemiological reports have shown that for every 10 µg/m2 increase in PM2.5 in the real environment, there is an 11% increase in cardiovascular disease-related mortality in the exposed population (20). Furthermore, the survival rate of liver cancer patients has been shown to be significantly shortened when they are exposed to environments with air pollution (21,22). Therefore, reduced air quality may lead to an increased incidence of chronic diseases.
Current research has found that increased levels of NIs in the air can significantly reduce the PM2.5 concentration (23,24) and there is a positive correlation between NI content and air humidity (25). The relative abundance of NIs near forests and waterfalls has long been attributed to its “mysterious” health benefits. NIs can sink heavy metals in the air. It has also been found that trees, shrubs, and mosses in forest environments produce volatile sterols that diffuse into the air, creating a continuous and stable aerosol environment (26,27). Whether this natural aerosol environment may exert a protective effect on the lungs has not been reported in detail. In this study, we investigated the proteomic changes in rat lungs under short-term environmental aerosol exposure in a waterfall forest by using LC-MS (28). LC-MS is the primary analytical technique for identifying and quantifying peptides and proteins in biological samples nowadays, which is crucial to identify biomarkers and compare between samples (28). These analyses could be obtained following specific signals after the fragmentation of peptides with high specificity, accuracy, and reproducibility. Besides, LC-MS can be utilized to measure the amount of post-translationally modified forms and isoforms of proteins. Through LC-MS detection, a total of 9,398 differentially expressed proteins were identified in this study. The highest number of differentially expressed proteins between the urban environment and the waterfall environment was found on day 5 of exposure compared to day 10 and day 15. This suggested that short-term exposure to different environments can affect proteomic changes in the lungs. Exposure to waterfall forest aerosols for 5 days downregulated the expression of the classical inflammatory nuclear factor κB (NF-κB) signaling pathway. A study has shown that air pollution can lead to the activation of toll-like receptor 4 (TLR4) and pro-inflammatory transcription factor NF-κB, and the expression of pro-inflammatory cytokines, such as tumor necrosis factor α (TNF-α) and IL-8, by increasing the levels of reactive oxygen species (ROS) in the body (29). In contrast, the present study demonstrated that waterfall forest aerosol exposure attenuated the activity of the NF-κB signaling pathway and reduced the occurrence of microinflammation in the lungs. GO and KEGG analyses revealed that the waterfall forest aerosol environment initially affected the oxidative phosphorylation of lung cells, followed by hormone signaling and the immune-related high-affinity IgE receptor pathway. A previous study has also demonstrated that the waterfall aerosol environment can alleviate asthma exacerbations, but the effect can only be observed after a longer period of exposure (≥30 days) (9). In contrast, the present proteomics study suggested that the effect of the waterfall forest aerosol environment on immune-related signaling pathways in the lung can be produced after 15 days of environmental exposure.
Protein interaction analysis identified Uqcrfs1, Tufm, and Rpl4 as possible biological targets related to the evaluation of air quality. Uqcrfs1 is a core component of the mitochondrial respiratory chain (30,31). It transmits electrons to cytochrome C. Elevation of Uqcrfs1 expression leads to increased levels of cytochrome C1, resulting in volumetric hyperglycemia and metabolic abnormalities (32). Weng et al. showed that abnormal mitochondrial respiratory chain metabolism also leads to impairment of invariant natural killer T cell (iNKT) preactivation and reduced T cell receptor (TCR) signaling (33). This is consistent with the reports of neonatal immune deficiency due to air pollution exposure (34). The current study demonstrated that Uqcrfs1 protein expression was diminished after environmental exposure to waterfall forest aerosols, further weakening the electron transport activity. This increased the stability of mitochondrial complex III, thereby reducing the release of large amounts of ROS from the mitochondrial complex to both sides of the inner cell membrane and inhibiting the oxidative stress associated with air pollution.
Tufm is a key factor encoded by nuclear genes that regulate mitochondrial protein translation, and it is involved in amino acid elongation and codon-anticodon and amino acid-tRNA pairing proofreading during the translation of mitochondrial gene proteins (35). The expression level of Tufm in lung cancer tissues has been shown to be positively correlated with epithelial cell marker protein E-cadherin and negatively correlated with the progression of lung cancer. Knockdown of Tufm inhibited the expression of mitochondrial gene-encoded proteins in lung cancer cells, causing impairment of mitochondrial function and promoting ROS production (36). The cellular energy and oxidative stress caused by Tufm downregulation can lead to the activation of AMP-activated protein kinase (AMPK). Activated AMPK further phosphorylates glycogen synthase kinase-3 beta (GSK3β), which in turn promotes nuclear translocation of b-catenin and the expression of cytosolic genes. This ultimately induces endothelial mesenchymal transition (EMT) in lung cancer cells and promotes migration, infiltration, and anti-apoptosis in lung cancer cells. Thus, downregulation of nuclear gene protein Tufm suppresses mitochondrial gene expression, which in turn contributes to the activation of the AMPK-GSK3β-β-catenin pathway, ultimately affecting nuclear gene expression and inducing EMT and related cellular function changes in lung cancer cells. In addition, has been demonstrated that the phosphorylated state of Tufm can alter its pro-mitochondrial autophagy function to an inhibitory function. The increased expression of Tufm in the lungs of rats in the waterfall forest aerosol group prevented excessive clearance of mitochondria and stabilized organelle function, further suggesting that the risk of lung cancer may be reduced by long-term exposure to this environment (37).
To date, Rpl4 has only been associated with hepatocellular carcinoma progression, and no correlation has been reported for lung disease. Further in-depth studies are warranted to examine the intrinsic link between air quality and Rpl4 (38).
There were some limitations to this study. First, it is not clear what major component of the waterfall forest aerosol environment modulated the protein alterations in rat lungs. We hypothesized that the aerosol exerts a compound protective effect on the lungs. Second, the effects of the waterfall forest aerosol environment on the proteomics of other organs, such as the heart, liver, and kidneys, remain to be elucidated. Future experiments should examine the specific mechanisms and biological markers of the effect of air quality on the proteins in different organs. Third, the lung tissue in this study was obtained from SD rats, whose genetic background is somewhat different from that of the population. An investigation of relevant medical data should be conducted to verify the health benefits of short-term exposure to waterfall forest aerosols in the environment.
Conclusions
LC-MS is an effective method for studying the effects of short-term exposure to waterfall forest aerosols in the environment. Exposure to waterfall forest aerosols for 5 days reduced the microinflammation of lung tissues and after 15 days of exposure, the allergen response was reduced in lung tissues. Uqcrfs1, Tufm, and Rpl4 may be biological targets relevant to the evaluation of air quality, while ribosomal signaling pathways may play an important role in the protective mechanism of waterfall forest aerosols on the lung.
Acknowledgments
The authors would like to thank the Ecological Meteorology and Satellite Remote Sensing Center of Guizhou Meteorological Bureau and the Education Affairs Management Center of Huangguoshu Tourism District, Anshun, Guizhou.
Funding: This study was supported by the Project of Science and Technology Society (No. 2018 [4], Anshun City, Guizhou Province, China).
Footnote
Reporting Checklist: The authors have completed the ARRIVE reporting checklist. Available at https://atm.amegroups.com/article/view/10.21037/atm-22-4813/rc
Data Sharing Statement: Available at https://atm.amegroups.com/article/view/10.21037/atm-22-4813/dss
Conflicts of Interest: All authors have completed the ICMJE uniform disclosure form (available at https://atm.amegroups.com/article/view/10.21037/atm-22-4813/coif). The authors have no conflicts of interest to declare.
Ethical Statement: The authors are accountable for all aspects of the work in ensuring that questions related to the accuracy or integrity of any part of the work are appropriately investigated and resolved. Animal experiments were performed under a project license (No. 1901001) granted by the Experimental Animal Ethics Committee of Guizhou Provincial People’s Hospital, in compliance with institutional guidelines for the care and use of animals.
Open Access Statement: This is an Open Access article distributed in accordance with the Creative Commons Attribution-NonCommercial-NoDerivs 4.0 International License (CC BY-NC-ND 4.0), which permits the non-commercial replication and distribution of the article with the strict proviso that no changes or edits are made and the original work is properly cited (including links to both the formal publication through the relevant DOI and the license). See: https://creativecommons.org/licenses/by-nc-nd/4.0/.
References
- Miller MR. The cardiovascular effects of air pollution: Prevention and reversal by pharmacological agents. Pharmacol Ther 2022;232:107996. [Crossref] [PubMed]
- Zhang Y, Liu D, Liu Z. Fine Particulate Matter (PM2.5) and Chronic Kidney Disease. Rev Environ Contam Toxicol 2021;254:183-215. [PubMed]
- Zhang M, Ding S, Pang J, et al. The effect of indirect household energy consumption on PM 2.5 emission in China: An analysis based on CLA method. J Environ Manage 2021;279:111531. [Crossref] [PubMed]
- Riederer AM, Krenz JE, Tchong-French MI, et al. Effectiveness of portable HEPA air cleaners on reducing indoor endotoxin, PM10, and coarse particulate matter in an agricultural cohort of children with asthma: A randomized intervention trial. Indoor Air 2021;31:1926-39. [Crossref] [PubMed]
- Tamana SK, Gombojav E, Kanlic A, et al. Portable HEPA filter air cleaner use during pregnancy and children's body mass index at two years of age: The UGAAR randomized controlled trial. Environ Int 2021;156:106728. [Crossref] [PubMed]
- Zhang JB, Rong YM, Yin QF, et al. Spatiotemporal Variation and Influencing Factors of TSP and Anions in Coastal Atmosphere of Zhanjiang City, China. Int J Environ Res Public Health 2022;19:2030. [Crossref] [PubMed]
- Kim M, Jeong GJ, Hong JY, et al. Negative Air Ions Alleviate Particulate Matter-Induced Inflammation and Oxidative Stress in the Human Keratinocyte Cell Line HaCaT. Ann Dermatol 2021;33:116-21. [Crossref] [PubMed]
- Grafetstätter C, Gaisberger M, Prossegger J, et al. Does waterfall aerosol influence mucosal immunity and chronic stress? A randomized controlled clinical trial. J Physiol Anthropol 2017;36:10. [Crossref] [PubMed]
- Gaisberger M, Šanović R, Dobias H, et al. Effects of ionized waterfall aerosol on pediatric allergic asthma. J Asthma 2012;49:830-8. [Crossref] [PubMed]
- Weke K, Kote S, Faktor J, et al. DIA-MS proteome analysis of formalin-fixed paraffin-embedded glioblastoma tissues. Anal Chim Acta 2022;1204:339695. [Crossref] [PubMed]
- Sato H, Inoue Y, Kawashima Y, et al. In-Depth Serum Proteomics by DIA-MS with In Silico Spectral Libraries Reveals Dynamics during the Active Phase of Systemic Juvenile Idiopathic Arthritis. ACS Omega 2022;7:7012-23. [Crossref] [PubMed]
- Chaudhuri S, Kumar A. Urban greenery for air pollution control: a meta-analysis of current practice, progress, and challenges. Environ Monit Assess 2022;194:235. [Crossref] [PubMed]
- Yao L, Li X, Zheng R, et al. The Impact of Air Pollution Perception on Urban Settlement Intentions of Young Talent in China. Int J Environ Res Public Health 2022;19:1080. [Crossref] [PubMed]
- Bodor K, Szép R, Bodor Z. The human health risk assessment of particulate air pollution (PM2.5 and PM10) in Romania. Toxicol Rep 2022;9:556-62. [Crossref] [PubMed]
- Czwojdzińska M, Terpińska M, Kuźniarski A, et al. Exposure to PM2.5 and PM10 and COVID-19 infection rates and mortality: A one-year observational study in Poland. Biomed J 2021;44:S25-36. [Crossref] [PubMed]
- Gao X, Jiang W, Liao J, et al. Attributable risk and economic cost of hospital admissions for depression due to short-exposure to ambient air pollution: A multi-city time-stratified case-crossover study. J Affect Disord 2022;304:150-8. [Crossref] [PubMed]
- Joshi SS, Miller MR, Newby DE. Air pollution and cardiovascular disease: the Paul Wood Lecture, British Cardiovascular Society 2021. Heart 2022;108:1267-73. [Crossref] [PubMed]
- Huuskonen MT, Liu Q, Lamorie-Foote K, et al. Air Pollution Particulate Matter Amplifies White Matter Vascular Pathology and Demyelination Caused by Hypoperfusion. Front Immunol 2021;12:785519. [Crossref] [PubMed]
- Liu Q, Shkirkova K, Lamorie-Foote K, et al. Air Pollution Particulate Matter Exposure and Chronic Cerebral Hypoperfusion and Measures of White Matter Injury in a Murine Model. Environ Health Perspect 2021;129:87006. [Crossref] [PubMed]
- Brauer M, Davaakhuu N, Escamilla Nuñez MC, et al. Clean Air, Smart Cities, Healthy Hearts: Action on Air Pollution for Cardiovascular Health. Glob Heart 2021;16:61. [Crossref] [PubMed]
- Pritchett N, Spangler EC, Gray GM, et al. Exposure to Outdoor Particulate Matter Air Pollution and Risk of Gastrointestinal Cancers in Adults: A Systematic Review and Meta-Analysis of Epidemiologic Evidence. Environ Health Perspect 2022;130:36001. [Crossref] [PubMed]
- Wu ZH, Zhao M, Yu H, et al. The impact of particulate matter 2.5 on the risk of hepatocellular carcinoma: a meta-analysis. Int Arch Occup Environ Health 2022;95:677-83. [Crossref] [PubMed]
- Su TH, Lin CS, Lin JC, et al. Dry deposition of particulate matter and its associated soluble ions on five broadleaved species in Taichung, central Taiwan. Sci Total Environ 2021;753:141788. [Crossref] [PubMed]
- Liu S, Huang Q, Wu Y, et al. Metabolic linkages between indoor negative air ions, particulate matter and cardiorespiratory function: A randomized, double-blind crossover study among children. Environ Int 2020;138:105663. [Crossref] [PubMed]
- Guerra LP, Andrade LMV, Joner DC, et al. Home measures against low air humidity which may alleviate health problems. Einstein (Sao Paulo) 2021;19:eAO5484. [Crossref] [PubMed]
- Scungio M, Crognale S, Lelli D, et al. Characterization of the bioaerosol in a natural thermal cave and assessment of the risk of transmission of SARS-CoV-2 virus. Environ Geochem Health 2022;44:2009-20. [Crossref] [PubMed]
- Haque MM, Verma SK, Deshmukh DK, et al. Seasonal and temporal variations of ambient aerosols in a deciduous broadleaf forest from northern Japan: Contributions of biomass burning and biological particles. Chemosphere 2021;279:130540. [Crossref] [PubMed]
- Kulyyassov A, Fresnais M, Longuespée R. Targeted liquid chromatography-tandem mass spectrometry analysis of proteins: Basic principles, applications, and perspectives. Proteomics 2021;21:e2100153. [Crossref] [PubMed]
- Zhang Z, Weichenthal S, Kwong JC, et al. A Population-Based Cohort Study of Respiratory Disease and Long-Term Exposure to Iron and Copper in Fine Particulate Air Pollution and Their Combined Impact on Reactive Oxygen Species Generation in Human Lungs. Environ Sci Technol 2021;55:3807-18. [Crossref] [PubMed]
- Chen F, Bai J, Zhong S, et al. Molecular Signatures of Mitochondrial Complexes Involved in Alzheimer's Disease via Oxidative Phosphorylation and Retrograde Endocannabinoid Signaling Pathways. Oxid Med Cell Longev 2022;2022:9565545. [Crossref] [PubMed]
- Sánchez E, Lobo T, Fox JL, et al. LYRM7/MZM1L is a UQCRFS1 chaperone involved in the last steps of mitochondrial Complex III assembly in human cells. Biochim Biophys Acta 2013;1827:285-93. [Crossref] [PubMed]
- Sato T, Chang HC, Bayeva M, et al. mRNA-binding protein tristetraprolin is essential for cardiac response to iron deficiency by regulating mitochondrial function. Proc Natl Acad Sci U S A 2018;115:E6291-300. [Crossref] [PubMed]
- Weng X, Kumar A, Cao L, et al. Mitochondrial metabolism is essential for invariant natural killer T cell development and function. Proc Natl Acad Sci U S A 2021;118:e2021385118. [Crossref] [PubMed]
- García-Serna AM, Martín-Orozco E, Jiménez-Guerrero P, et al. Cytokine profiles in cord blood in relation to prenatal traffic-related air pollution: The NELA cohort. Pediatr Allergy Immunol 2022;33:e13732. [Crossref] [PubMed]
- Choi CY, Vo MT, Nicholas J, et al. Autophagy-competent mitochondrial translation elongation factor TUFM inhibits caspase-8-mediated apoptosis. Cell Death Differ 2022;29:451-64. [Crossref] [PubMed]
- Ashrafizadeh M, Mirzaei S, Hushmandi K, et al. Therapeutic potential of AMPK signaling targeting in lung cancer: Advances, challenges and future prospects. Life Sci 2021;278:119649. [Crossref] [PubMed]
- He K, Guo X, Liu Y, et al. TUFM downregulation induces epithelial-mesenchymal transition and invasion in lung cancer cells via a mechanism involving AMPK-GSK3β signaling. Cell Mol Life Sci 2016;73:2105-21. [Crossref] [PubMed]
- Yang X, Sun L, Wang L, et al. LncRNA SNHG7 accelerates the proliferation, migration and invasion of hepatocellular carcinoma cells via regulating miR-122-5p and RPL4. Biomed Pharmacother 2019;118:109386. [Crossref] [PubMed]
(English Language Editor: J. Teoh)