Web-based study on Chinese dermatologists’ attitudes towards artificial intelligence
Introduction
It was at the Dartmouth conference of 1956 that “artificial intelligence” (AI) was first formally proposed as a general term, and defined as the ability of technology to model intelligent behavior with minimal human intervention. Currently, AI is widely used in many fields, including in medical areas and healthcare systems (1-3). As dermatology is a medical specialty in which numerous clinical images are used, and the visual characteristics of observable image features are a key focus, it seems to be a field highly suited for the integration of AI training. Indeed, preliminary studies have concluded that the application of AI in dermatology has the potential advantages of being able to potentially automate repetitive tasks, optimize time-consuming tasks, extend access to limited medical resources, improve interobserver reliability issues, and expand the diagnostic toolbox of dermatologists (4). Thus far, many well-known and meaningful AI studies have been conducted which have focused on the recognition and classification of skin diseases, including skin cancers (5-13), atopic dermatitis (14), psoriasis (15,16), and onychomycosis (17).
In China, the development of AI has recently garnered more interest, and, as it makes inroads into dermatology, the interest in it from dermatologists has risen in kind. A library of skin images known as the Chinese Skin Image Database (CSID) was established in 2017 (18) and has developed into a system platform for skin imaging research, education, and application in China. CSID provides a large amount of skin imaging data for AI training and has facilitated the development of a series of clinical AI projects. AI research has also become more popular in dermatology in China; for example, AI methods were used to classify melanoma using 2,200 dermoscopy images in the Chinese population (19). However, there is currently no research or summary review of the attitudes that Chinese dermatologists’ hold towards AI. Therefore, the aim of this study was to evaluate the awareness that Chinese dermatologists have AI through a web-based questionnaire.
Methods
Study design
This study was initiated and sponsored by the National Institute of Hospital Administration, the National Health Commission of the People’s Republic of China, and the China Digital Medicine Magazine. We designed a web-based questionnaire (Supplementary file at http://fp.amegroups.cn/cms/e869712a2753bdd10cd7cf5f686dc12e/atm.2019.12.102-1.pdf) by reviewing the literatures (20-24) and consulting the CSID expert committee. Technicians from UMER Doctor (an online learning platform for dermatologists developed by Shanghai Wheat Color Intelligent Technology Company, China) provided technological support. Groups from CSID and UMER Doctor were responsible for conducting this survey.
Online survey platform
We published the questionnaire on the UMER Doctor platform (http://www.umer.com.cn) for two weeks (from November 16 to 30, 2018). The UMER Doctor application is the largest online learning platform for dermatologists in China, and over 20,000 dermatologists (approximately 24,000 dermatologists in China) have registered with the platform. The UMER Doctor platform provided access to an ample number of Chinese dermatologists to complete this survey. Dermatologists from the UMER Doctor platform were recruited to fill in the questionnaire online and were prompted to complete the questionnaire in 20 minutes. The participated dermatologists also provided their demographic information, professional title, and the hospital name and level.
Division of observations
In this questionnaire, we classified age groups along with the following guidelines: 18–44-year-old as the younger population, 45–59-year-old as the middle-aged population, and ≥60-year-old as the senior population. Hospitals in China are mainly divided into a 3-tier system (primary, secondary, and tertiary hospitals) based on the ability to provide medical care, medical education, and conduct medical research, along with additional services such as community health service stations, private clinics, and village clinics. The professional titles of dermatologists in China include chief physician, associate chief physician, attending physician, resident physician, and other (with a research title such as research fellow, associate research fellow, assistant research fellow, or without a professional title). In order to measure the attention levels of dermatologists towards AI information, we divided the levels of attention into four categories. These categories were active attention (actively search and concern for AI-related information), passive-active attention (reading AI-related information when seen), general attention (sometimes reading AI-related AI information), and unconcerned (ignoring AI-related information). Furthermore, we classified the dermatologists’ attitudes and thoughts regarding AI impact into the following four categories: replace dermatologists’ daily work, assisting the daily diagnosis and treatment activities for dermatologists, useless, and unconcerned.
Statistical analysis
The continuous variables data were shown as mean ± standard deviation (SD), and the categorical variables data were shown in numbers and percentages. Attention levels to AI information and the beliefs about the roles AI from the perspective of Chinese dermatologists were regarded as ordered categorical data in this study. In instances where the objective was to analyze the differences of dermatologists' attitudes towards AI among different groups (stratified by age, gender, hospital level, education degree, professional title, and hospital ownership), the datasets were regarded as one-way ordered categorical data; Mann-Whitney U test was used in the datasets were from two-independent samples, and Kruskal-Wallis H test was used in the datasets were from K-independent samples (K>2). When we attempted to calculate the correlations between stratified factors and dermatologists’ attitudes towards AI or the thought of AI roles from the perspective of Chinese dermatologists, these datasets were regarded as two-way ordered categorical data. In these cases, Spearman’s rank correlation test was used in this study. Statistical package for social science (SPSS), version 22.0 (IBM Corp., Armonk, NY, USA) was used for all analyses. A two-sided P value <0.05 was considered statistically significant in all analyses.
Results
Basic characteristics of participated dermatologists
There were 1,228 valid questionnaires that were completed by Chinese dermatologists from 30 provinces, autonomous regions, municipalities, and other regions (including Hong Kong, Macau, and Taiwan) (Figure 1). The average age of the participated dermatologists was 36.84 (SD =8.86). Of the 1,228 Chinese dermatologists, the male-to-female ratio was 476:752. Regarding the education of participants, 508 (41.4%) dermatologists held a bachelor’s degree, 485 (39.5%) dermatologists held a master’s degree, 105 (8.6%) dermatologists held a doctorate degree, and 130 (10.6%) dermatologists held other degrees or had no degree. Regarding the professional titles of the participants, 366 (29.8%) dermatologists were resident physicians, 473 (38.5%) were attending physicians, 206 (16.8%) were associate chief physicians, 89 (7.2%) were chief physicians, and 94 (7.7%) had other professional titles or had no professional title. The hospital distribution of dermatologists included 47 (3.8%) dermatologists from primary hospitals, 309 (25.2%) from secondary hospitals, 745 (60.7%) from tertiary hospitals, and 127 (10.3%) from other medical institutions (such as hospitals without a level of determination, or clinics). A total of 1,112 (90.65%) dermatologists were affiliated with public medical institutions, 84 (6.8%) were affiliated with private medical institutions, and 32 (2.6%) were affiliated with other medical institutions (such as a combination of public and private ownership).
Approaches to obtain AI information by Chinese dermatologists
We investigated how Chinese dermatologists acquire information about AI throughout their day-to-day work and life. The survey results showed that Chinese dermatologists obtained information about AI in a variety of approaches (Table 1), mainly through the internet (83.47%) and meetings or forums (73.70%). It is especially interesting that 70.51% of participated dermatologists acquired information about AI by two or more approaches, and that there were some other sources, including advertisements, brochure, course, etc.
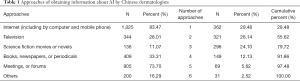
Full table
Attention levels about AI information by Chinese dermatologists
In total, 99.51% of participated dermatologists indicated that they pay attention (general, passive-active, and active attention) to information about AI. We stratified and analyzed the attention levels among Chinese dermatologists by age, gender, hospital level, education degree, professional title, and hospital ownership. Interestingly, stratified analyses showed statistically significant differences in their attention levels (unconcerned, general, passive-active, and active attention) to AI information by gender, hospital level, education degree, and professional title (P values ≤1.79E−02). There were correlations between stratified factors and the levels of attention, and although all P values were significant, but the relationships were extremely weak (Table 2).
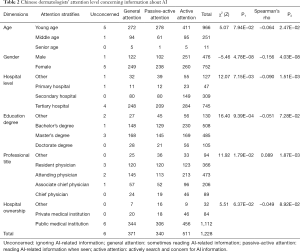
Full table
The roles of AI from the perspective of Chinese dermatologists
In total, 95.36% of the participated dermatologists believed the role of AI to be in “assisting the daily diagnosis and treatment activities for dermatologists”, whereas only 3.42% thought AI would replace dermatologists’ daily work, 0.98% thought AI is useless, and 0.24% were unconcerned about its role. We also stratified and analyzed the beliefs about AI roles (unconcerned, useless, assist, and replace) from participants of the survey by age, gender, hospital level, education degree, professional title, and hospital ownership. The results show that there was no statistically significant difference except the hospital level (P value =4.09E−03). The correlations between stratified factors and the beliefs about AI roles were weak and without significance (Table 3).
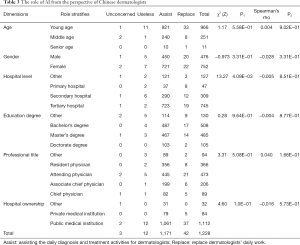
Full table
Application of AI in hospitals from the perspective of Chinese dermatologists
We investigated the implementation of AI in different levels of the hospital from the perspective of Chinese dermatologists to help determine which level of hospitals might benefit more from AI. The ratio shows that participants believed all levels of hospitals need AI in the clinic, while 788 (64.17%), 734 (59.77%), 593 (48.29%), and 596 (48.53%) dermatologists who participated thought that the secondary hospitals, tertiary hospitals, primary hospitals, and others (community health service stations, private clinics, and village clinics) needed AI application, respectively. These results will help prioritize the future direction of AI application in dermatology.
Application of AI in skin diseases from the perspective of Chinese dermatologists
We investigated the application of AI in skin diseases from the perspective of Chinese dermatologists to determine which kinds of skin diseases would benefit most from AI in the clinic. There were 1,127 (91.78%) participating dermatologists who thought AI should be applied for skin tumors. Additionally, 814 (66.29%), 777 (63.27%), 765 (62.3%), 702 (57.17%), 579 (47.15%), 474 (38.6%), and 351 (28.58%) of participated dermatologists thought AI should be applied to melanonychia, alopecia disease, vitiligo, psoriasis, allergic dermatitis, acne, and other skin diseases (freckle, tinea corporis, and cruris, keratosis pilaris, etc.), respectively. These results indicate the interests and attitudes of Chinese dermatologists in applying AI in skin diseases.
Discussion
To our knowledge, this study is the first web-based survey about Chinese dermatologists’ attitudes towards AI. There were 1,228 Chinese dermatologists from 30 provinces, autonomous regions, municipalities, and other regions (including Hong Kong, Macau, and Taiwan) who participated in this survey. The ratio of female to male was 1.58:1, which is consistent with the general belief that there are more female than male dermatologists in China. Of the participants, most dermatologists had obtained bachelor and graduate degrees, were resident physicians and attending physicians, and came from secondary hospitals and tertiary hospitals. The majority of most of the dermatologists’ hospitals were owned by public medical institutions. These phenomena are all consistent with the demographic distribution of Chinese dermatologists and hospitals in China.
In an era of information explosion, the modern world allows information to be quickly transmitted; therefore, there are various approaches to obtain information about AI. In a multicenter survey, around 52% of medical students were aware of the ongoing discussion about AI in radiology; however, 68% of them stated that they were unaware of the specific technologies involved (23). How Chinese dermatologists find information about AI, and their level of attention to information about AI is still understudied. Because of this, we conducted this research survey. According to the survey results, Chinese dermatologists obtained information about AI mainly through the Internet. Most importantly, 70.51% of dermatologists acquired information about AI using two or more approaches, which indicates that Chinese dermatologists are interested in AI from multiple sources. It is interesting to note that 99.51% of participated dermatologists pay attention (general, passive-active, and active attention) to AI information. Stratified analyses showed statistically significant differences in their attention levels to AI information by gender, hospital level, education degree, and professional title. We hypothesized that the stratified factors affect the Chinese dermatologists’ attention levels to AI-related information, but the correlations between stratified factors and attention were extremely weak. These findings suggest that it is not necessary to classify information about AI using dermatologist-related factors.
AI products have a broad application in dermatology, which will significantly improve doctor ability in diagnosis and treatment. Additionally, AI may also change the doctor's medical behavior patterns, and enable the physicians in primary hospitals to diagnose and treat skin diseases using skin lesion images. What are the AI roles in clinical treatment? We surveyed the beliefs of Chinese dermatologists about the role of AI, except for the hospital level, we found no statistically significant difference concerning beliefs on AI roles among the stratified groups. In addition, correlations between stratified factors and the beliefs on AI roles were weak correlations without significance. There are, however, two noteworthy observations from our study: 95.36% of participated dermatologists thought the role of AI to be in “assisting the daily diagnosis and treatment activities for dermatologists”, and only 3.42% of participated dermatologists thought AI would replace dermatologists’ daily work.
Will AI then replace dermatologists in the near future? In fact, the answer is not necessarily affirmative. Accurate diagnoses are important in clinical patient care, and approximately 10% of patient death and adverse events in the hospital are caused by diagnostic errors (25). Usually, clinicians must manage their patients before making a definitive diagnosis, and the diagnosis often hinges on management decisions (26). Diagnosis is a complex task and clinicians usually must perform a thorough examination of the patient, and not make a conclusion from a single lesion. When making a diagnosis, clinicians will consider additional information, such as the age of the patient, the duration of the disease, medical history, family history, and other symptoms beyond the skin lesion alone. Although AI has reached a performance above their human counterparts in many aspects, the higher diagnostic accuracy with digital images does not necessarily translate into better clinical performance or patient management (20).
Recently, an open, web-based, international, diagnostic study showed that state-of-the-art machine-learning classifiers outperformed human experts in the diagnosis of pigmented skin lesions, and it was recommended that these classifiers should have a more important role in clinical practice (20). The authors stated that a possible limitation of the algorithms that were implemented was due to out-of-distribution images, showing that there is room for improvement in the AI of the future. We investigated the role of AI technology in the clinic from the perspective of Chinese dermatologists. Regarding the dermatologists' understanding of the role of AI, 95.36% of participated dermatologists thought the role of AI to be in “assisting the daily diagnosis and treatment activities for dermatologists”, and only 3.42% of participated dermatologists thought AI would replace the work of dermatologists. In the field of dermatology, there are various kinds of skin diseases, and there are a vast number of patients with skin diseases in each country around the world. Dermatologists have a high patient volume, as described colorfully by Dr. Zhang in “A Day in the Life of a Chinese Dermatologist” (27). We believe AI will be widely applied in medical institutions to assist doctors’ daily work. As Yi et al. stated, the key relationship between AI and Radiology is collaboration (28), and we also recognize that the ideal pattern between AI and dermatologists is mutual cooperation.
As to the question of which level of the hospital most needs AI, our investigation showed that all levels of hospitals would benefit from the assistance of AI in clinical work. From the survey results on AI applications in hospitals, the top two choices from Chinese dermatologists were secondary hospitals and tertiary hospitals. It is speculated that AI is needed in these hospitals due to the large volume of patients. Our survey also examined the kinds of skin diseases that would benefit most from integrating AI into clinical work. We found that the most mentioned diseases for AI applications, as indicated by a dermatologist, were skin tumors, melanonychia, alopecia diseases, vitiligo, psoriasis, allergic dermatitis, and acne. The priorities for the application of AI indicated by the survey correlate closely with the importance and incidence of skin diseases. Skin tumors are exceedingly common and with increasing incidence across the globe, and there is a significant cost burden in the care of skin tumors in many countries (29). Melanonychia and other skin diseases (alopecia diseases, vitiligo, psoriasis, allergic dermatitis, and acne) also show high incidence, especially in China. These survey results will direct our future research about the application of AI in dermatology.
Our study is the first to investigate Chinese dermatologists’ attitudes towards AI. However, there are some limitations to this study. First, most of the participants were from the tertiary hospitals and were young dermatologists. It would have been beneficial to have more responses from secondary and primary hospitals, and more senior dermatologists should have been recruited to participate in this survey. Second, additional questions should have been included in this questionnaire in order to better understand the thoughts of AI from dermatologists in China. Third, though the number of participants in this survey represents a relatively large sample size, a larger scale study should be conducted in the future.
There was a survey conducted among the European Society of Radiology members from November to December 2018 regarding the expectations of AI in 5–10 years, responders showed a generally favorable attitude towards AI (30). With the current speed of technological innovation, AI in dermatology will become one of the most advanced and promising clinical technologies in the medical field. It is necessary that more resources, including government attention, funding input, expertise, and enterprise resource pooling, be integrated in order to further promote the rapid development and application of AI in dermatology.
Conclusions
Our study showed that most Chinese dermatologists are interested in information about AI and obtain this information through a variety of approaches. Almost all dermatologists pay attention to AI-related information, and the majority believe the role of AI to be in “assisting the daily diagnosis and treatment activities for dermatologists”. In the future, AI should be implemented in secondary hospitals and in skin tumors. We believe that AI in dermatology will better assist dermatologists in the diagnosis and treatment of skin diseases and will provide better healthcare to patients in the future.
Acknowledgments
We would like to acknowledge the support of the Chinese Skin Image Database, and Shanghai Wheat Color Intelligent Technology Company ltd.; we also would like to thank all those who participated in this study.
Funding: This work is supported by grants from the Milstein Medical Asian American Partnership Foundation Research Project, “Establishment and application of digital image database for skin Diseases in Chinese population” (Grant No.: MMAAP2016023); the China Digital Medicine Funding “Research on present situation and future development of artificial medical intelligence in China”; the Open Research Funding of China Skin Image Database (Grant no.: CSID-ORF-201711 and CSID-ORF-201918); and the scholarship from China Scholarship Council (Grant No.: 201806210430).
Footnote
Provenance and Peer Review: This article was commissioned by the Guest Editors (Haotian Lin and Limin Yu) for the series “Medical Artificial Intelligent Research” published in Annals of Translational Medicine. The article was sent for external peer review organized by the Guest Editors and the editorial office.
Conflicts of Interest: All authors have completed the ICMJE uniform disclosure form (available at http://dx.doi.org/10.21037/atm.2019.12.102). The series “Medical Artificial Intelligent Research” was commissioned by the editorial office without any funding or sponsorship. The authors have no other conflicts of interest to declare.
Ethical Statement: The authors are accountable for all aspects of the work in ensuring that questions related to the accuracy or integrity of any part of the work are appropriately investigated and resolved.
Open Access Statement: This is an Open Access article distributed in accordance with the Creative Commons Attribution-NonCommercial-NoDerivs 4.0 International License (CC BY-NC-ND 4.0), which permits the non-commercial replication and distribution of the article with the strict proviso that no changes or edits are made and the original work is properly cited (including links to both the formal publication through the relevant DOI and the license). See: https://creativecommons.org/licenses/by-nc-nd/4.0/.
References
- Magrabi F, Ammenwerth E, McNair JB, et al. Artificial Intelligence in Clinical Decision Support: Challenges for Evaluating AI and Practical Implications. Yearb Med Inform 2019;28:128-34. [Crossref] [PubMed]
- Wong NC, Shayegan B. Patient centered care for prostate cancer-how can artificial intelligence and machine learning help make the right decision for the right patient? Ann Transl Med 2019;7:S1. [Crossref] [PubMed]
- Cabitza F, Zeitoun JD. The proof of the pudding: in praise of a culture of real-world validation for medical artificial intelligence. Ann Transl Med 2019;7:161. [Crossref] [PubMed]
- Schlessinger DI, Chhor G, Gevaert O, et al. Artificial intelligence and dermatology: opportunities, challenges, and future directions. Semin Cutan Med Surg 2019;38:E31-7. [Crossref] [PubMed]
- Han SS, Kim MS, Lim W, et al. Classification of the Clinical Images for Benign and Malignant Cutaneous Tumors Using a Deep Learning Algorithm. J Invest Dermatol 2018;138:1529-38. [Crossref] [PubMed]
- Nasr-Esfahani E, Samavi S, Karimi N, et al. Melanoma detection by analysis of clinical images using convolutional neural network. Conf Proc IEEE Eng Med Biol Soc 2016;2016:1373-6. [PubMed]
- Esteva A, Kuprel B, Novoa RA, et al. Dermatologist-level classification of skin cancer with deep neural networks. Nature 2017;542:115-8. [Crossref] [PubMed]
- Fujisawa Y, Otomo Y, Ogata Y, et al. Deep-learning-based, computer-aided classifier developed with a small dataset of clinical images surpasses board-certified dermatologists in skin tumour diagnosis. Br J Dermatol 2019;180:373-81. [Crossref] [PubMed]
- Brinker TJ, Hekler A, Enk AH, et al. A convolutional neural network trained with dermoscopic images performed on par with 145 dermatologists in a clinical melanoma image classification task. Eur J Cancer 2019;111:148-54. [Crossref] [PubMed]
- Brinker TJ, Hekler A, Enk AH, et al. Deep learning outperformed 136 of 157 dermatologists in a head-to-head dermoscopic melanoma image classification task. Eur J Cancer 2019;113:47-54. [Crossref] [PubMed]
- Brinker TJ, Hekler A, Hauschild A, et al. Comparing artificial intelligence algorithms to 157 German dermatologists: the melanoma classification benchmark. Eur J Cancer 2019;111:30-7. [Crossref] [PubMed]
- Chuchu N, Takwoingi Y, Dinnes J, et al. Smartphone applications for triaging adults with skin lesions that are suspicious for melanoma. Cochrane Database Syst Rev 2018;12:CD013192. [PubMed]
- Marchetti MA, Codella NCF, Dusza SW, et al. Results of the 2016 International Skin Imaging Collaboration International Symposium on Biomedical Imaging challenge: Comparison of the accuracy of computer algorithms to dermatologists for the diagnosis of melanoma from dermoscopic images. J Am Acad Dermatol 2018;78:270-7.e1. [Crossref] [PubMed]
- Hekler A, Utikal JS, Enk AH, et al. Pathologist-level classification of histopathological melanoma images with deep neural networks. Eur J Cancer 2019;115:79-83. [Crossref] [PubMed]
- Shrivastava VK, Londhe ND, Sonawane RS, et al. Computer-aided diagnosis of psoriasis skin images with HOS, texture and color features: A first comparative study of its kind. Comput Methods Programs Biomed 2016;126:98-109. [Crossref] [PubMed]
- Lu J, Kazmierczak E, Manton JH, et al. Automatic segmentation of scaling in 2-D psoriasis skin images. IEEE Trans Med Imaging 2013;32:719-30. [Crossref] [PubMed]
- Han SS, Park GH, Lim W, et al. Deep neural networks show an equivalent and often superior performance to dermatologists in onychomycosis diagnosis: Automatic construction of onychomycosis datasets by region-based convolutional deep neural network. PLoS One 2018;13:e0191493. [Crossref] [PubMed]
- Zheng Y, Lin Y, Cui Y. Teledermatology in China: History, Current Status, and the Next Step. J Investig Dermatol Symp Proc 2018;19:S71-3. [Crossref] [PubMed]
- Cui X, Wei R, Gong L, et al. Assessing the effectiveness of artificial intelligence methods for melanoma:A retrospective review. J Am Acad Dermatol 2019;81:1176-80. [Crossref] [PubMed]
- Tschandl P, Codella N, Akay BN, et al. Comparison of the accuracy of human readers versus machine-learning algorithms for pigmented skin lesion classification: an open, web-based, international, diagnostic study. Lancet Oncol 2019;20:938-47. [Crossref] [PubMed]
- Radtke T, Ostergaard M, Cooke R, et al. Web-Based Alcohol Intervention: Study of Systematic Attrition of Heavy Drinkers. J Med Internet Res 2017;19:e217. [Crossref] [PubMed]
- Jun JH, Lee HW, Choi J, et al. Perceptions of using herbal medicines for managing menopausal symptoms: a web-based survey of Korean medicine doctors. Integr Med Res 2019;8:229-33. [Crossref] [PubMed]
- Pinto Dos Santos D, Giese D, Brodehl S, et al. Medical students' attitude towards artificial intelligence: a multicentre survey. Eur Radiol 2019;29:1640-6. [Crossref] [PubMed]
- Nadarzynski T, Miles O, Cowie A, et al. Acceptability of artificial intelligence (AI)-led chatbot services in healthcare: A mixed-methods study. Digit Health 2019;5:2055207619871808. [Crossref] [PubMed]
- Balogh EP, Miller BT, Ball JR. editors. National Academies of Sciences, Engineering, and Medicine. Improving Diagnosis in Health Care. Washington, DC: National Academies Press, 2015.
- Cook DA, Sherbino J, Durning SJ. Management Reasoning: Beyond the Diagnosis. JAMA 2018;319:2267-8. [Crossref] [PubMed]
- Zhang CF, Ren J. A Day in the Life of a Chinese Dermatologist. J Investig Dermatol Symp Proc 2015;17:9-10. [Crossref] [PubMed]
- Yi PH, Hui FK, Ting DSW. Artificial Intelligence and Radiology: Collaboration Is Key. J Am Coll Radiol 2018;15:781-3. [Crossref] [PubMed]
- Gordon LG, Rowell D. Health system costs of skin cancer and cost-effectiveness of skin cancer prevention and screening: a systematic review. Eur J Cancer Prev 2015;24:141-9. [Crossref] [PubMed]
- European Society of Radiology (ESR). Impact of artificial intelligence on radiology: a EuroAIM survey among members of the European Society of Radiology. Insights Imaging 2019;10:105. [Crossref] [PubMed]