High-level gain of mesenchymal-epithelial transition factor (MET) copy number using next-generation sequencing as a predictive biomarker for MET inhibitor efficacy
Introduction
The discovery of actionable gene mutations has revolutionized the therapeutic landscape and significantly improved the survival outcomes of patients with advanced non-small cell lung cancer (NSCLC) harboring oncogenic driver mutations (1-6). Oncogenic driver mutation in genes encoding tyrosine kinases including epidermal growth factor receptor (EGFR), anaplastic lymphoma kinase (ALK), and mesenchymal-epithelial transition factor (MET) predicts clinical response to various types of tyrosine kinase inhibitors (TKI) (1-6). Molecular testing to evaluate the mutation status of these oncogenic driver mutations are thus crucial in identifying patients who could benefit from the TKI therapy.
Gain-of-function of MET signaling has been demonstrated in oncogenic processes across multiple tumor types, including lung cancer through protein overexpression, gene amplification, mutations, or abnormal gene splicing (7-11). MET signaling is activated when hepatocyte growth factor (HGF) ligand binds to the MET receptor, inducing homodimerization and phosphorylation, which subsequently leads to the activation of the tyrosine kinase domain. This activation mediates the downstream signaling pathways including the mitogen-activated protein kinase (MAPK), phosphoinositide 3-kinase/protein kinase B (PI3K/AKT), signal transducer and activator of transcription (STAT), and nuclear factor-κB (NF-κB) pathways. This promotes cytoplasmic and nuclear processes, leading to a variety of cellular functions, including proliferation, survival, and migration (7).
Alterations in MET have been associated with poor prognosis and hence, it holds promise as a therapeutic target (8-10,12). A number of drugs, including inhibitors and monoclonal antibodies, have been developed to support MET signaling repression through targeting either MET or its ligand, HGF (8,13-17). Multi-target MET TKIs, crizotinib and cabozantinib, have been approved for the treatment of ALK-rearranged advanced NSCLC (18), and their efficacy has been demonstrated in advanced NSCLC patients with MET amplifications and exon 14 alterations (13-15,19,20). Meanwhile, a combination of EGFR TKI and MET TKI are being explored for those with concurrent EGFR mutation and MET amplification (20,21).
Conventionally, MET copy number (CN) gains or amplifications are quantified by fluorescence in situ hybridization (FISH) based on two methods: one method scores the CN of MET per cell (absolute CN), and the other quantifies the proportion of MET relative to other areas on the same chromosome such as the chromosome 7 centromere (MET/CEP7) (14,22). A high-level of MET amplification has been associated with poor prognosis and better response to MET TKIs when either method is used (9,14,23). Despite efforts to standardize the interpretation criteria for MET amplification status, there is a lack of consensus surrounding the cut-off values for the optimal classification of the MET-amplified tumors that would be most likely to respond to MET TKIs. As a result, treatment responses have been inconsistent. One possible explanation for this inconsistency is the presence of concurrent alterations in oncogenic drivers, such as KRAS amplification and KRAS G12D mutation (15,24-26), which results in tumors that are not solely driven by MET amplification.
In recent years, clinical oncology has seen targeted next-generation sequencing (NGS) become integral to the routine molecular diagnostics repertoire (27-29). Compared with traditional screening methods such as FISH, targeted capture-based NGS is advantageous due to its ability to simultaneously assess multiple alterations in oncogenic genes and provide a more comprehensive mutational profile, making this technology a superior option for molecular diagnosis. However, NGS-generated data are typically reported as absolute CN alteration with no established CN cut-off value for defining the subset of patients who would most likely benefit from MET-TKI treatment. The key to successful and effective targeted therapy lies in the selection of appropriate patients who will respond to the treatment. Hence, it is critical to establish the optimal criteria so that the subset of patients who would be most likely to have tumors that are primarily dependent on MET amplification as the sole oncogenic driver (and therefore would respond to MET TKI treatment) can be defined. In this study, we aim to identify the criteria to define MET amplification derived from NGS data that could potentially serve as a biomarker for MET TKI efficacy in NSCLC patients.
Methods
Patient recruitment
A total of 597 MET-amplified NSCLC patients with various stages and histological types were enrolled from 5 hospitals in this retrospective study. For the initial feasibility steps for the normalization of MET CN, sequencing data for matching tissue and plasma samples from 40 MET-amplified NSCLC patients were retrieved and analyzed with the patients’ consent.
To further identify the appropriate criteria for defining MET CN with benefit from MET TKI, the survival outcomes of 18 MET-amplified patients who were treated with MET TKI were assessed. Tumor histology was confirmed by examinations conducted independently by two pathologists. Tumors were staged according to the American Joint Committee on Cancer seventh edition tumor, node, metastasis (TNM) staging system of NSCLC (30). Detailed clinical and pathological information including age at diagnosis, tumor histopathology, clinical stage, and family history was collected from each patient. The decision of which MET TKI would be administered to the patients (either crizotinib or cabozantinib) was made by the attending physician. The study received approval from the ethics committee of Jiangsu Cancer Hospital and was conducted in accordance with the standards of the 1964 Declaration of Helsinki. Prior written informed consent for the use of plasma and/or tissue samples in further molecular studies was obtained from each of the patients.
Tissue and cell-free DNA isolation
Tissue DNA was extracted from formalin-fixed, paraffin-embedded (FFPE) tumor tissues using QIAamp DNA FFPE tissue kit (Qiagen, Hilden, Germany). The QIAamp Circulating Nucleic Acid kit (Qiagen, Hilden, Germany) was used to recover circulating cell-free DNA (cfDNA) from 4 to 5 mL of plasma.
Capture-based targeted DNA sequencing
A minimum of 50 ng of DNA is required for NGS library construction. Tissue DNA was sheared using Covaris M220 (Covaris, MA, USA), followed by end repair, phosphorylation, and adaptor ligation. An Agencourt AMPure XP Kit (Beckman Coulter, CA, USA) was used to purify fragments between 200–400 bp from the cfDNA and sheared tissue DNA, before hybridization with capture probe baits, hybrid selection with magnetic beads, and PCR amplification. The quality and the size of the fragments were assessed using Qubit 2.0 Fluorimeter with the dsDNA high-sensitivity assay kit (Life Technologies, Carlsbad, CA, USA). Indexed samples were sequenced on NextSeq 500 (Illumina, Inc., USA) with paired-end reads and an average sequencing depth of 1,000× and 10,000× for tissue and plasma samples, respectively. A panel of 168 genes including 68 lung cancer-related genes and 100 other genes related to cancer development, spanning 0.273 megabases (Mb) of the human genome, were used for targeted sequencing.
Sequence data analysis
Sequence data were mapped to the reference human genome (hg19) using the Burrows-Wheeler Aligner v.0.7.10 software package (31). Local alignment optimization, duplication marking and variant calling were performed using the Genome Analysis Tool Kit v.3.2 (Broad Institute, Cambridge, MA, USA) and VarScan v.2.4.3 (Genome Institute, Washington University, St. Louis, MO, USA) (32). Each plasma sample was compared against its own white blood cell control to identify somatic variants. Variants were filtered using the VarScan fpfilter pipeline, loci with a depth of less than 100 were filtered out. Base-calling in plasma and tissue samples required at least eight supporting reads for single nucleotide variations (SNV) and five supporting reads for insertion-deletion variations (INDEL), respectively. Variants with a population frequency over 0.1% in the ExAC, 1,000 Genomes, dbSNP or ESP6500SI-V2 databases were grouped as single nucleotide polymorphisms (SNP) and excluded from further analysis. Remaining variants were annotated with ANNOVAR (2016-02-01 release) (33) and SnpEff v.3.6 (34). Factera v.1.4.3 was used to carry out analysis of DNA translocation (35). Copy number variations (CNV) were analyzed on the basis of the depth of coverage data of capture intervals. Coverage data were corrected against sequencing bias stemming from GC content and probe design. The average coverage of all captured regions was used to normalize the coverage of different samples to comparable scales. CN was calculated based on the ratio between the depth of coverage in tumor samples and average coverage of an adequate number (n>50) of samples without CNV as references per capture interval. CNV is called if the coverage data of the gene region was quantitatively and statistically significant from its reference control. For CNVs, the limit of detection is 1.5 for CN deletions and 2.64 for CN amplifications.
Normalization of MET CN alterations
For each sample, adjusted copy number (adCN) was calculated as the absolute CN subtracted by 2, which was defined as the normal CN, divided by tumor purity, and the addition of 2 from the resulting quotient using the following formula [Eq. 1]:
| [1] |
Absolute or non-adjusted copy number (non-adCN) was defined as the CN output from the segmentation algorithm used to call CN alterations. Tumor purity for each tissue sample was calculated using the maximum mutant allele fraction (maxMAF) multiplied by 2 based on the assumption that mutations are heterogeneous. MaxMAF was defined as the highest mutant allele fraction among all the mutations detected from a single sample. The maxMAF for tissue samples should meet the following criteria: not higher than 50%, and no CN alterations on the genes selected. If the maxMAF could not be determined, the tumor cell percentage determined by hematoxylin-eosin staining of the FFPE tissue samples evaluated by trained histologists were used. Tissue samples not meeting the criteria for tumor purity, tumor percentage and maxMAF were excluded from the analysis. Tumor purity in plasma cfDNA was determined by the maxMAF multiplied by 2. The maxMAF for cfDNA should meet following criteria: greater than 2% and not exceeding 50%, and no CN alterations on the genes selected in the paired tissue sample. Plasma samples that did not meet the criteria for tumor purity and maxMAF were excluded from the analysis.
Statistical analysis
Software packages including Prism 8 (GraphPad, CA, USA) and R statistics package (R version 3.4.0; R: The R-Project for Statistical Computing, Vienna, Austria). Survival data were analyzed by Kaplan-Meier, and log-rank test was used to compare the difference between survival groups, while the Mantel-Cox proportional hazard model was used in the calculation of hazard ratios (HRs). Differences between groups were calculated and presented using paired, two-tailed Student’s t-test in P value. In all statistical tests, P<0.05 was considered statistically significant.
Results
Normalization of MET CN alteration
Retrospective analysis of the sequencing data from 40 MET-amplified patients with matched tissue and plasma samples was carried out to test the feasibility of normalizing MET CN alterations. Table S1 summarizes the mutation profile of the matched tissue and plasma samples of this cohort.
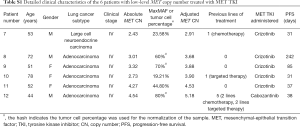
Full table
Using the data for non-adCN, a very weak correlation was observed between the paired plasma and tissue samples (R2=0.26, Figure 1A). On the other hand, the correlation improved after adjusting the absolute CN per sample relative to its corresponding tumor purity (adCN, R2=0.73, Figure 1B).
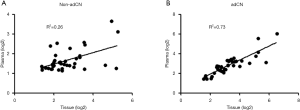
These correlation data suggest that normalizing CN with tumor purity to account for tumor heterogeneity with each sample allows either the tissue or plasma samples to be utilized for the reliable detection of CN alterations by NGS.
The relationship between adjusted MET CN alteration and concurrent somatic mutation in driver genes
As a potent oncogenic driver, MET amplification has been shown to be mutually exclusive of other lung cancer drivers (9,11,36). To validate the use of adjusted MET CN in either tissue or plasma, we further interrogated the mutation profiles of 127 tissue samples and 61 plasma samples from MET-amplified patients. Through this, we revealed the relationship between MET CN and concurrent oncogenic drivers including activating mutations in KRAS, ALK, RET, ROS1, BRAF genes, ERBB2 exon 20 insertion, MET exon 14 skipping, and amplifications in EGFR, ERBB2, and FGFR1. Since MET amplification is considered to be one of the mechanisms in developing resistance to EGFR TKI therapy (37-42), patients with concurrent EGFR sensitizing mutations who received EGFR TKI therapy were excluded from this analysis.
Based on the CN data obtained using tissue samples, adjusted MET CN data reflected the role of MET as a sole driver when compared to non-adCN data (adCN: P=0.015 vs. non-adCN: P=0.16, Figure 2A,B). Using the adjusted MET CN in the tissue samples, we further used the median adCN of the group as a cut-off value to categorize CNs as high or low. The patients with 4.8 adCN and less (adCN ≤4.8) were categorized as having low CN, while those with CNs exceeding 4.8 adCN (adCN >4.8) were categorized as high. Consistent with the relationship between MET amplification and the concurrence of alterations in other oncogenic drivers, our data also revealed this inverse relationship, in which a majority (7/11, 63.6%) of the MET-amplified patients with high CN had no other alteration in driver genes, while a majority (18/29, 62.1%) of the MET-amplified patients with low CN had concurrent alteration in other oncogenic drivers (P=0.12, Figure 2C).
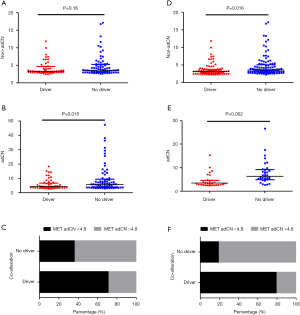
On the other hand, based on the CN data obtained using plasma samples, both the non-adCN and adCNs reflected the relationship between MET amplification and concurrent alterations in other oncogenic genes (Figure 2D,E). However, compared to the non-adjusted data, greater statistical significance was seen with the adCN (adCN: P=0.002 vs. non-adCN: P=0.016, Figure 2D,E). Moreover, the adjusted MET CN from the plasma samples even better reflected the mutual exclusivity of MET amplification and concurrent alterations in other oncogenic genes. A majority (9/11, 81.8%) of the MET-amplified patients with high CN had no other driver gene alterations, while a majority (23/29, 79.3%) of the MET-amplified patients with low CN had concurrent alterations in oncogenic drivers (P=2.469×10−6, Figure 2F).
These data suggest that the relationship between MET amplification and concurrent oncogenic drivers was reflected by the adCNs derived from data using either tissue or plasma samples.
The relationship between MET CN and survival outcome of MET-amplified patients after treatment with MET TKI
To demonstrate the MET CN that will have a better response to MET TKI, we analyzed the survival data from 18 MET-amplified patients with no concurrent alterations in other oncogenic genes.
Of these 18 MET TKI-treated patients with MET as the sole oncogenic driver, 78% (14/18) were male, and the rest (22%, 4/18) were female. The median age of the patients was 53 years, ranging from 36 to 80 years. The predominant histology of the patients in this cohort was adenocarcinoma (88.9%, 16/18), while 1 patient had large cell neuroendocrine carcinoma and 1 had an undetermined subtype. Most of the patients were clinically diagnosed with stage IV (94.4%, 17/18), and the remaining patient had stage IIIB. Among these patients, 16 patients received crizotinib and 2 patients received cabozantinib. The patient characteristics are summarized in Table 1.
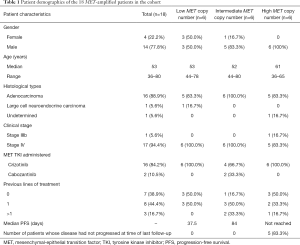
Full table
The non-adjusted and adjusted MET CNs of the patients were stratified accordingly into tertiles and patients categorized into low-, intermediate- and high-level amplification for further analysis. Based on the progression-free survival (PFS) data, non-adjusted MET CN did not reflect a benefit of MET TKI among all the groups regardless of groupings (P=0.25, Figure 3A). According to Cox proportional analysis for non-adCN, only a cut-off of 3 CNs could stratify the patients to demonstrate differences in PFS [P=0.004, HR =0.07, 95% confidence interval (CI): 0.01–0.41; Figure 3B]. On the contrary, tertile stratification of the adjusted data revealed that adCN could directly reflect the benefit of MET TKI among the patients. In relation to this, the patients with adCN of <5.5, categorized as low-level amplification, had the shortest median PFS of 37.5 days (Table S1). The patients with intermediate-level amplification and adCN between >5.5 to <13 had a median PFS of 84 days (Table S2). Meanwhile, the patients with adCN of >13, categorized as high-level amplification, showed longer PFS, with a median PFS that has not yet been reached (P=0.009, Figure 3C, Table 2). Cox proportional analysis for adCN revealed that a cut-off of 5 and above can allow effective stratification of patients based on adCN and reveal differences in PFS (cut-off =5, P=0.045, HR =0.3, 95% CI: 0.1–0.97; Figure 3D).
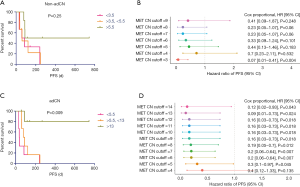
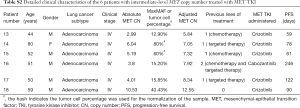
Full table
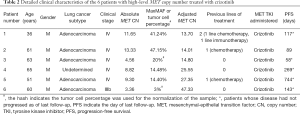
Full table
Each of the 6 patients with high-level MET CN amplification was male, with a median age of 61 years (range, 36 to 65 years). A majority of the patients (83.3%, 5/6) were diagnosed with adenocarcinoma and 1 patient had an undetermined subtype, and most of them (83.3%, 5/6) had stage IV, although 1 patient had stage IIIb. Three patients (50%) were treatment-naïve, two patients (33%) had previously received chemotherapy, and one patient had previously received immunochemotherapy. All of the patients with high-level amplification received crizotinib. Furthermore, 83% (5/6) of the patients with high-level MET CNs had not experienced disease progression at the time of the last follow-up in comparison with the other groups (Table 1). Detailed patient information is summarized in Table 2.
These data suggest that adjusted MET CN can be used as a standard for identifying the subset of patients with MET-dependent NSCLC who can benefit from MET TKI.
Discussion
Gene amplification is the most frequent genetic alteration associated with cancer (43). Traditionally, FISH was used to quantify gene amplifications; however, single-gene tests are time-consuming and can become costly when several tests are ordered at once. Targeted NGS, owing to its ability to simultaneously test multiple cancer-related genes and mutation types, has become the preferred choice for comprehensive mutational profiling in cancer diagnosis in recent years. A well-designed panel for targeted NGS, which includes oncogenic driver genes and key genes that are involved in pathways leading to carcinogenesis, can identify targetable as well as concurrent mutations in patients and can inform their treatment approach. Targeted NGS can also be used to monitor the course of treatment to gain molecular insight into the mechanism driving disease progression and guide subsequent treatment approaches (27,28). However, the DNA samples used for detection and quantification in NGS are extracted from a combination of tumor cells and non-tumor cells including normal stromal, epithelial and lymphoid cells. With the inherent genetic intratumor heterogeneity, interpreting absolute CN would incur bias and increase false-positive rates. The normalization method derived in this study takes into account tumor heterogeneity by adjusting the absolute CN derived from NGS data with tumor purity based on maxMAF. Based on our findings, this method could identify the MET CN to define the patients whose tumors are primarily driven by MET and reflect the response to MET TKIs. Additionally, this normalization method could be utilized for CN data obtained from either tissues or plasma samples. For tumor samples, adCNs normalized with tumor purity were highly correlated (Pearson coefficient 0.6533, P<0.0001; Figure S1); this suggests tumor purity using either NGS-based maxMAF or H&E-based tumor percentage could reliably normalize the NGS-derived CNs. Similarly, FISH-based assessment of MET amplification involves counting of MET/CEP7 ratio in at least 50 nuclei of tumor cells per specimen (22,39), indicating that it is critical to estimate CNs from only the tumor cell component of the sample to reflect relative CNs from NGS-derived CNs. The exclusion of the proportion of non-tumor cells from NGS data using our normalization method reflected the actual MET CNs from the tumor cell component of the patient samples and enabled the identification of patients with high MET CNs who benefitted from crizotinib therapy. Consistent with highly efficient targeted inhibition of oncogenically-addicted cells (23,44), our findings demonstrate that the higher the relative MET CN, the better the treatment outcomes with MET TKI.
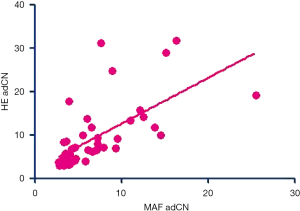
Alterations in MET are associated with higher metastatic potential due to its involvement with pathways including proliferation, survival, and motility (7-11). Several reports have also revealed that MET amplifications are mutually exclusive of other lung cancer drivers, suggesting they represent true oncogenic driver state (9,11). Reflecting the dependency of the tumor to MET amplifications, high-level MET amplification has been associated with dramatic responses to MET TKI. This assertion was successfully demonstrated with the use of the adCNs in our study when MET TKI-treated patients with adCN of more than 13, who were categorized as high-level amplification, had significantly more durable responses to MET TKI, reflected by a longer PFS in comparison with patients in the other categories. On the contrary, lower amplification levels relate to MET as a coincident event (14). Consistently, the data derived from our normalization method reflected the mutually exclusive relationship between MET amplification and concurrent oncogenic drivers. However, a certain percentage of patients with MET CNs >4.8 also had concurrent oncogenic gene alterations, highlighting the essentiality of molecular profiling in understanding the patients’ mutational landscape and the design of combination therapy for this subset of patients. Combination therapy has recently gained an increasing amount of attention and is a promising strategy to synergistically address concurrent oncogenic alterations (20,21,45).
Due to its retrospective nature, our study has limitations that should be discussed. These include the small number of patients involved in the validation cohort, and the lack of several types of clinical data including MET amplification evaluated by FISH, maximum tumor reduction, and the objective responses of the MET TKI-treated patients for correlation studies. Well-designed prospective studies which take into consideration a larger cohort are necessary to further validate the use of adjusted MET CN as a predictive biomarker. It would also be interesting to explore the application of adjusted MET CN in predicting clinical benefit with more selective MET TKIs including capmatinib, and tepotinib. It is also possible to incorporate adjusted MET CN as a biomarker in clinical trials of other MET TKIs that are currently under investigation. Moreover, the comparison of adjusted MET CN with traditional methods of assessing MET amplifications or overexpression including FISH and immunohistochemistry would also be important in establishing adjusted MET CN as a predictive biomarker.
Conclusions
Overall, we derived a normalization method that could reflect the relative CN and distinguish MET-amplified NSCLC patients with high-level amplification who were sensitive to crizotinib. These findings highlight the importance of factoring in intratumor heterogeneity in the interpretation of CN data generated from NGS. Moreover, our study paves the way for the potential use of adjusted MET high CN gain as a predictive biomarker for MET TKI response in NSCLC patients.
Acknowledgments
The authors thank all the patients who participated in this study and their families. We also thank the investigators, study coordinators, operation staff and the whole project team who worked on this study.
Funding: None.
Footnote
Data Accessibility: The datasets analyzed for this study are available in the Sequence Read Archive (SRA), hosted by the National Center for Biotechnology Information (NCBI), available as accession number PRJNA565453.
Conflicts of Interest: All authors have completed the ICMJE uniform disclosure form (available at http://dx.doi.org/10.21037/atm-20-2741). JX, AL, and JY are employees of Burning Rock Biotech. The other authors have no conflicts of interest to declare.
Ethical Statement: The authors are accountable for all aspects of the work in ensuring that questions related to the accuracy or integrity of any part of the work are appropriately investigated and resolved. The study received approval from the ethics committee of Shanghai Chest Hospital (No. KS(Y)2046) and was conducted in accordance with the standards of the 1964 Declaration of Helsinki. Prior written informed consent for the use of plasma and/or tissue samples in further molecular studies was obtained from each of the patients.
Open Access Statement: This is an Open Access article distributed in accordance with the Creative Commons Attribution-NonCommercial-NoDerivs 4.0 International License (CC BY-NC-ND 4.0), which permits the non-commercial replication and distribution of the article with the strict proviso that no changes or edits are made and the original work is properly cited (including links to both the formal publication through the relevant DOI and the license). See: https://creativecommons.org/licenses/by-nc-nd/4.0/.
References
- Velez MA, Burns TF. Is the game over for PD-1 inhibitors in EGFR mutant non-small cell lung cancer? Transl Lung Cancer Res 2019;8:S339-S342. [Crossref] [PubMed]
- Koivunen JP, Mermel C, Zejnullahu K, et al. EML4-ALK fusion gene and efficacy of an ALK kinase inhibitor in lung cancer. Clin Cancer Res 2008;14:4275-83. [Crossref] [PubMed]
- Maemondo M, Inoue A, Kobayashi K, et al. Gefitinib or chemotherapy for non-small-cell lung cancer with mutated EGFR. N Engl J Med 2010;362:2380-8. [Crossref] [PubMed]
- Spitaleri G, Passaro A, de Marinis F. Ensartinib (X-396) a novel drug for anaplastic lymphoma kinase-positive non-small cell lung cancer patients: we need smart trials to avoid wasting good bullets. Chin Clin Oncol 2019;8:S1. [Crossref] [PubMed]
- Fukuoka M, Wu YL, Thongprasert S, et al. Biomarker analyses and final overall survival results from a phase III, randomized, open-label, first-line study of gefitinib versus carboplatin/paclitaxel in clinically selected patients with advanced non-small-cell lung cancer in Asia (IPASS). J Clin Oncol 2011;29:2866-74. [Crossref] [PubMed]
- Zaman A, Bivona TG. Emerging application of genomics-guided therapeutics in personalized lung cancer treatment. Ann Transl Med 2018;6:160. [Crossref] [PubMed]
- Van Der Steen N, Pauwels P, Gil-Bazo I, et al. cMET in NSCLC: Can We Cut off the Head of the Hydra? From the Pathway to the Resistance. Cancers (Basel) 2015;7:556-73. [Crossref] [PubMed]
- Smyth EC, Sclafani F, Cunningham D. Emerging molecular targets in oncology: clinical potential of MET/hepatocyte growth-factor inhibitors. Onco Targets Ther 2014;7:1001-14. [Crossref] [PubMed]
- Tong JH, Yeung SF, Chan AW, et al. MET Amplification and Exon 14 Splice Site Mutation Define Unique Molecular Subgroups of Non-Small Cell Lung Carcinoma with Poor Prognosis. Clin Cancer Res 2016;22:3048-56. [Crossref] [PubMed]
- Dimou A, Non L, Chae YK, et al. MET gene copy number predicts worse overall survival in patients with non-small cell lung cancer (NSCLC); a systematic review and meta-analysis. PLoS One 2014;9:e107677. [Crossref] [PubMed]
- Frampton GM, Ali SM, Rosenzweig M, et al. Activation of MET via diverse exon 14 splicing alterations occurs in multiple tumor types and confers clinical sensitivity to MET inhibitors. Cancer Discov 2015;5:850-9. [Crossref] [PubMed]
- Kim JH, Kim HS, Kim BJ. Prognostic value of MET copy number gain in non-small-cell lung cancer: an updated meta-analysis. J Cancer 2018;9:1836-45. [Crossref] [PubMed]
- Paik PK, Drilon A, Fan PD, et al. Response to MET inhibitors in patients with stage IV lung adenocarcinomas harboring MET mutations causing exon 14 skipping. Cancer Discov 2015;5:842-9. [Crossref] [PubMed]
- Noonan SA, Berry L, Lu X, et al. Identifying the Appropriate FISH Criteria for Defining MET Copy Number-Driven Lung Adenocarcinoma through Oncogene Overlap Analysis. J Thorac Oncol 2016;11:1293-304. [Crossref] [PubMed]
- Schrock AB, Frampton GM, Suh J, et al. Characterization of 298 Patients with Lung Cancer Harboring MET Exon 14 Skipping Alterations. J Thorac Oncol 2016;11:1493-502. [Crossref] [PubMed]
- Spigel DR, Edelman MJ, O'Byrne K, et al. Results From the Phase III Randomized Trial of Onartuzumab Plus Erlotinib Versus Erlotinib in Previously Treated Stage IIIB or IV Non-Small-Cell Lung Cancer: METLung. J Clin Oncol 2017;35:412-20. [Crossref] [PubMed]
- Tarhini AA, Rafique I, Floros T, et al. Phase 1/2 study of rilotumumab (AMG 102), a hepatocyte growth factor inhibitor, and erlotinib in patients with advanced non-small cell lung cancer. Cancer 2017;123:2936-44. [Crossref] [PubMed]
- Kazandjian D, Blumenthal GM, Chen HY, et al. FDA approval summary: crizotinib for the treatment of metastatic non-small cell lung cancer with anaplastic lymphoma kinase rearrangements. Oncologist 2014;19:e5-11. [Crossref] [PubMed]
- Ou SH, Kwak EL, Siwak-Tapp C, et al. Activity of crizotinib (PF02341066), a dual mesenchymal-epithelial transition (MET) and anaplastic lymphoma kinase (ALK) inhibitor, in a non-small cell lung cancer patient with de novo MET amplification. J Thorac Oncol 2011;6:942-6. [Crossref] [PubMed]
- Zhang T, Li J. A c-met inhibitor in the treatment of metastatic non small cell lung cancer with c-met amplification. J Clin Oncol 2016;34:abstr e20524.
- Li A, Yang JJ, Zhang XC, et al. Acquired MET Y1248H and D1246N Mutations Mediate Resistance to MET Inhibitors in Non-Small Cell Lung Cancer. Clin Cancer Res 2017;23:4929-37. [Crossref] [PubMed]
- Cappuzzo F, Marchetti A, Skokan M, et al. Increased MET gene copy number negatively affects survival of surgically resected non-small-cell lung cancer patients. J Clin Oncol 2009;27:1667-74. [Crossref] [PubMed]
- Caparica R, Yen CT, Coudry R, et al. Responses to Crizotinib Can Occur in High-Level MET-Amplified Non-Small Cell Lung Cancer Independent of MET Exon 14 Alterations. J Thorac Oncol 2017;12:141-4. [Crossref] [PubMed]
- Cepero V, Sierra JR, Corso S, et al. MET and KRAS gene amplification mediates acquired resistance to MET tyrosine kinase inhibitors. Cancer Res 2010;70:7580-90. [Crossref] [PubMed]
- Liu SY, Gou LY, Li AN, et al. The Unique Characteristics of MET Exon 14 Mutation in Chinese Patients with NSCLC. J Thorac Oncol 2016;11:1503-10. [Crossref] [PubMed]
- Rotow J, Wu W, Blakely CM, et al. Concurrent genomic alterations in lung adenocarcinoma with a MET exon 14 skipping mutation. J Clin Oncol 2018;36:abstr 9083.
- Horak P, Frohling S, Glimm H. Integrating next-generation sequencing into clinical oncology: strategies, promises and pitfalls. ESMO Open 2016;1:e000094. [Crossref] [PubMed]
- Mullauer L. Next generation sequencing: clinical applications in solid tumours. Memo 2017;10:244-7. [Crossref] [PubMed]
- Freedman AN, Klabunde CN, Wiant K, et al. Use of Next-Generation Sequencing Tests to Guide Cancer Treatment: Results From a Nationally Representative Survey of Oncologists in the United States. JCO Precis Oncol 2018. [Crossref]
- Goldstraw P, Chansky K, Crowley J, et al. The IASLC Lung Cancer Staging Project: Proposals for Revision of the TNM Stage Groupings in the Forthcoming (Eighth) Edition of the TNM Classification for Lung Cancer. J Thorac Oncol 2016;11:39-51. [Crossref] [PubMed]
- Li H, Durbin R. Fast and accurate short read alignment with Burrows-Wheeler transform. Bioinformatics 2009;25:1754-60. [Crossref] [PubMed]
- Koboldt DC, Zhang Q, Larson DE, et al. VarScan 2: somatic mutation and copy number alteration discovery in cancer by exome sequencing. Genome Res 2012;22:568-76. [Crossref] [PubMed]
- Wang K, Li M, Hakonarson H. ANNOVAR: functional annotation of genetic variants from high-throughput sequencing data. Nucleic Acids Res 2010;38:e164. [Crossref] [PubMed]
- Cingolani P, Platts A. A program for annotating and predicting the effects of single nucleotide polymorphisms, SnpEff: SNPs in the genome of Drosophila melanogaster strain w1118; iso-2; iso-3. Fly (Austin) 2012;6:80-92. [Crossref] [PubMed]
- Newman AM, Bratman SV, Stehr H, et al. FACTERA: a practical method for the discovery of genomic rearrangements at breakpoint resolution. Bioinformatics 2014;30:3390-3. [Crossref] [PubMed]
- The Cancer Genome Atlas Research N. Comprehensive molecular profiling of lung adenocarcinoma. Nature 2014;511:543. [Crossref]
- Bean J, Brennan C, Shih JY, et al. MET amplification occurs with or without T790M mutations in EGFR mutant lung tumors with acquired resistance to gefitinib or erlotinib. Proc Natl Acad Sci U S A 2007;104:20932-7. [Crossref] [PubMed]
- Engelman JA, Zejnullahu K, Mitsudomi T, et al. MET amplification leads to gefitinib resistance in lung cancer by activating ERBB3 signaling. Science 2007;316:1039-43. [Crossref] [PubMed]
- Cappuzzo F, Janne PA, Skokan M, et al. MET increased gene copy number and primary resistance to gefitinib therapy in non-small-cell lung cancer patients. Ann Oncol 2009;20:298-304. [Crossref] [PubMed]
- Turke AB, Zejnullahu K, Wu YL, et al. Preexistence and clonal selection of MET amplification in EGFR mutant NSCLC. Cancer Cell 2010;17:77-88. [Crossref] [PubMed]
- Yu HA, Arcila ME, Rekhtman N, et al. Analysis of tumor specimens at the time of acquired resistance to EGFR-TKI therapy in 155 patients with EGFR-mutant lung cancers. Clin Cancer Res 2013;19:2240-7. [Crossref] [PubMed]
- Planchard D, Loriot Y, Andre F, et al. EGFR-independent mechanisms of acquired resistance to AZD9291 in EGFR T790M-positive NSCLC patients. Ann Oncol 2015;26:2073-8. [Crossref] [PubMed]
- Albertson DG. Gene amplification in cancer. Trends Genet 2006;22:447-55. [Crossref] [PubMed]
- Sharma SV, Settleman J. Oncogene addiction: setting the stage for molecularly targeted cancer therapy. Genes Dev 2007;21:3214-31. [Crossref] [PubMed]
- Yang Z, Tam KY. Combination Strategies Using EGFR-TKi in NSCLC Therapy: Learning from the Gap between Pre-Clinical Results and Clinical Outcomes. Int J Biol Sci 2018;14:204-16. [Crossref] [PubMed]