Perfusion MR prior to radiotherapy is a strong predictor of survival in high-grade gliomas after proton and carbon ion radiotherapy
Introduction
High-grade (i.e., WHO III or IV class) glioma, characterized by high angiogenesis, is the most frequent and lethal primary brain malignancy in adults (1). Progression is universal after standard treatment with resection, radiotherapy and temozolomide (TMZ) chemotherapy. The median survival remains dismal at approximately 15 months for glioblastoma patients treated with standard photon-based radiotherapy and TMZ after resection (2). In contrast to photon, charged particle (e.g., proton and carbon ion) beams allow sharper dose deposition and higher relative biological effectiveness (RBE), potentially providing therapeutic advantages of efficacy and toxicity profiles (3,4).
Particle beam radiotherapy (PBRT), as the current most advanced radiation technique, has been placed with expectation to reverse the poor outcome of high-grade glioma (HGG). Previous results of a collaborative research from Germany and Japan showed that carbon ion boost plus photon radiation improved survival of HGG patients in comparison to photon radiotherapy alone (4). Moreover, our early experience indicated an encouraging treatment efficacy of PBRT in HGG (5). PBRT has been increasingly spread worldwide to treat cancer, identifying predictors of treatment efficacy is a critical need for future development of PBRT in HGG.
Numerous demographic and tumor-related factors, particularly molecular characteristics including IDH gene, MGMT and TERT promoter status (1,6), have been identified as strong survival predictors in HGG. However, the nature of high heterogeneity within glioma tissue can induce significant sampling error of accurate pathologic and molecular assessment for the whole tumor area (7). Besides, intra-tumor cell populations with distinct molecular markers can react diverse treatment response (8,9). Radiomic, a novel imaging technique that providing a comprehensive and dynamic view of the whole tumor tissue, has been used with the combination of traditional markers to help deliver more accurate prognosis information.
Perfusion-weighted MR imaging, beyond conventional anatomical imaging methods, offers sub-structural micro-vascular information, such as angiogenesis and vascularity, for the entire brain tissue. In terms of angiogenesis, this highly typical character of HGG defines the invasiveness. Moreover, angiogenesis in HGG generally presents newly formed molecular microvessels with aberrant function, and mainly induces the radio-resistance of HGG by hypoxia (10). From these views, angiogenesis may be highly corresponding to HGG patients’ survival. With perfusion MR imaging, angiogenesis or microvessel hyperplasia is particularly reflected in cerebral blood volume (CBV).
For patients treated with photon-based radiotherapy, several studies indicated that CBV at a variety of time points was predictive of clinical outcome in HGG (11-17), but there are two other researches showed that CBV was not associated with survival in glioma (18,19). In the condition of PBRT, the value of CBV in predicting the survival of HGG patients is unknown. Besides, there are significant differences among tumor cells under hypoxia responding to different radiation beams (20,21). Therefore, it is essential to explore the relationship between angiogenesis and survival in HGG treated with PBRT, which may benefit improving survival predictability for informing clinical and personal decision-making, as well as distinguishing level of radio-resistance within tumor tissue for further improvement of PBRT in HGG. In the present study, we retrospectively documented the character of perfusion MR prior to PBRT, analyzed the effect of CBV on HGG patients’ clinical outcome, and further constructed nomograms to predict individual survival.
Methods
Patients and pretreatment workups
This retrospective study was approved by the institutional review board (IRB) of the Shanghai Proton and Heavy Ion Center (SPHIC), and patients’ informed consent was waived. The study was conducted in accordance with the Declaration of Helsinki (as revised in 2013). All patients were required to be presented and discussed in the multidisciplinary tumor clinic for their diagnosis, indication, and eligibility for the particle therapy protocol prior to registration and treatment at SPHIC.
Required pretreatment evaluation included a complete history and physical (H&P) examination, complete blood count, hepatic and renal function tests, electrocardiogram, and conventional MR with T1-weighted (T1W), T2-weighted (T2W), FLAIR and contrast-enhanced T1W imaging. Perfusion MR was encouraged and further required after the latest clinical trials of carbon-ion escalating boost in HGG initiated.
Particle radiotherapy and chemotherapy
Doses of PBRT were measured by gray relative biological equivalent [Gy (RBE)] to account for the RBE differences compared to photon beam. The clinical target volume (CTV) for high risk (CTV-hr) was defined as gross-tumor volume (GTV) in residual lesion detected on imaging studies and surgical bed plus 5 mm expansion, and the CTV for lower risk (CTV-lr) consisted of GTV plus 15 mm margin and edema area. The standard protocol of PBRT for all patients was CTV-hr with proton beam to 60 Gy (RBE), and CTV-lr with proton beam to 50 Gy (RBE). For patients with incomplete resection, dose escalating trials using proton of various does/fractions followed by carbon-ion escalating boost were encouraged to target residual disease.
Concurrent TMZ were planned to be used for all patients of <65 years of age, and ≥65 years with methylation of the MGMT promoter. Adjuvant TMZ was administered for at least six cycles in the absence of death or irreversible blood toxicity. Concurrent TMZ was started on first day of particle radiotherapy at 75 mg/m2 (oral), 7 days a week. Adjuvant TMZ was followed at 150–200 mg/m2 for 5 days during each 28-day cycle.
Perfusion MR and postprocessing
All MR imaging studies were performed on a 3.0T superconducting imaging unit (Magnetom Skyra; Siemens Medical Solutions, Germany). Conventional MR images, including T1W, T2W, contrast-enhanced T1W images, and dynamic susceptibility-weighted contrast-enhanced (DSC) perfusion MR images, were obtained during the same examination. DSC was with a gradient-echo echo-planar imaging sequence during the first pass of a standard-dose (0.1 mmol/kg) bolus of gadopentetate dimeglumine.
The normalized CBV (nCBV) map was generated using FDA-approved commercially available software (NordicBrainEx, NordicNeuroLab, Norway), and was presented as color overlays on structural images in semiautomatic method. The generation of nCBV map consisted of automatic/semiautomatic steps of leakage correction and normalization.
The method used for leakage correction is described by Bjornerud et al. (22). Leakage is estimated by a tail of the residual function equation
where C is the measured concentration, AIF is the arterial input function and R is the residual function. Basically, if the residual function does not approach 0 after a long time, there is leakage. The leakage correction is done by finding the mean value of the residual after a preset time Tc and multiplying it with the time that has passed after Tc.
Normalization in this study is done by Gaussian method (23,24). In brief, the value of CBV is normalized to the standard deviation of CBV throughout the whole normal brain, as shown in the equation
where σCBVWhole normal brain is standard deviation of the whole normal brain. The whole normal tissue is found by segmenting the whole brain using a clustering algorithm to exclude lesion and blood vessels.
The measurements of maximum nCBV (nCBVmax) within/adjacent to surgical bed and/or residual lesion were obtained using the ‘hot spot’ method (areas of peak CBV by visual inspection of the nCBV color map), as illustrated in Figure 1.
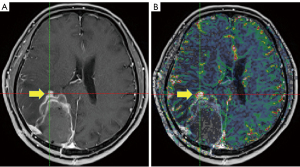
Treatment response assessments and follow-up
The patients were generally followed-up according to the institutional follow-up protocol of SPHIC after the completion of PBRT. The first follow-up was at 4 weeks after the completion of PBRT, then was planned to be followed-up with MRI scans and basic laboratory work every 2–3 months. The Response Assessment in Neuro-Oncology (RANO) criteria (25) with interpretation modifications (26), including parameters for changes in T1W enhancing lesion and non-enhancing T2/FLAIR, were used to determine disease progression.
Statistical analysis
Overall survival (OS) time is calculated as the duration between pathologic diagnosis and the date of death. Progression-free survival (PFS) time is defined as the duration between the time of diagnosis and the date of progression. Associations between nCBVmax [as continuous variable (nCBVmax-C)] and categorical variables (age, KPS, IDH, MGMT, tumor grade and extent of resection) were analyzed by Mann-Whitney test. Survival curves were constructed using the Kaplan-Meier method, and survival differences were evaluated by the log-rank test. Multivariate survival analysis was done using Cox proportional hazards (CPH) model. Receiver operator characteristic (ROC) analysis identified optimized cut-offs of nCBVmax stratified for PFS and OS. Nomograms in this study were created using information obtained from multivariable CPH analyses. Predictive performance of nomograms was assessed using the concordance index (C-index). Calibration curves of the nomograms were derived to evaluate the consistency between predicted survival and observed survival. All the statistical analyses were performed using the R software.
Results
Characteristics of patients and treatment
Between 6/2015 and 3/2019, the first 61 consecutive and non-selected patients with histology confirmed HGG were treated with standardized proton radiation or proton radiation plus carbon-ion boost at the SPHIC. Of these patients, 45 cases underwent perfusion MR imaging pre radiotherapy. The median age of 45 patients was 52 years old, and there were 28 males and 17 females. All patients had maximal possible surgical intervention, and concurrent particle radiotherapy and TMZ of the Stupp protocol. The characteristics of the patients, their condition, as well as PBRT are detailed in Table 1.
Table 1
Characteristics | No. of patients (N=45, %) |
---|---|
Gender | |
Male | 28 (62.2) |
Female | 17 (37.8) |
Age (years) | |
Median [range] | 52 [22–76] |
<50 | 22 (44.4) |
≥50 | 28 (55.6) |
KPS before radiotherapy | |
>80 | 37 (75.6) |
≤80 | 13 (24.4) |
Histology grade (WHO grade) | |
Grade IV | 29 (64.4) |
Grade III | 16 (35.6) |
IDH mutation | |
Wild type | 34 (75.6) |
Mutant type | 11 (24.4) |
Surgical intervention | |
PR/biopsy | 8 (17.8) |
STR | 21 (46.7) |
Total resection | 16 (35.5) |
MGMT promoter | |
Methylated | 14 (31.1) |
Un-methylated | 18 (40.0) |
NA | 13 (28.9) |
Doses of particle radiation (GyE/fractions) | |
Proton-60 GyE/30 | 25 (55.6) |
Proton-50 GyE/25 + C-ion-10–12 GyE/4–5* | 4 (8.9) |
Proton-60 GyE/30 + C-ion boost to 9–15 GyE/3 | 12 (26.7) |
Proton-34 GyE/10 + C-ion boost 9 GyE/3* | 2 (44.4) |
*, for patients ≥65 years only. PR, partial resection; STR, subtotal resection; GyE, gray-equivalent.
Correlations of nCBVmax with clinic-pathological factors
The median value of nCBVmax for the entire cohort was 6.332 (range, 1.600–13.180). The correlation between perfusion parameter and other characteristics were analyzed (detailed in Table 2). There was a trend toward correlation between nCBVmax and resection completeness (P=0.077). Interestingly, patients with IDH-wild type tend to have higher nCBVmax than those with IDH-mutant type after surgery (P=0.066). There was no statistically relation of nCBVmax to age, KPS, MGMT or histology grade.
Table 2
Characteristics | nCBVmax | P value |
---|---|---|
Age (years) | 0.848 | |
<50 | 6.128 | |
≥50 | 6.340 | |
KPS before radiotherapy | 0.397 | |
>80 | 5.985 | |
≤80 | 7.572 | |
Histology grade (WHO grade) | 0.341 | |
Grade IV | 6.332 | |
Grade III | 5.911 | |
IDH mutation | 0.066 | |
Wild type | 6.963 | |
Mutant type | 5.481 | |
Surgical intervention | 0.077 | |
Biopsy/PR/STR | 6.932 | |
GTR | 5.080 | |
MGMT promoter | 0.539 | |
Methylated | 6.340 | |
Un-methylated/NA | 6.189 |
nCBVmax, maximum normalized cerebral blood volume; PR, partial resection; STR, subtotal resection; GTR, gross total resection.
Survival analysis of nCBVmax with median value as cut-off
The median follow-up period of patients was 13.9 months. At the time of this analysis, 13 patients (2 grade III, 4 grade IV) had tumor progression, and 9 patients (2 grade III, 7 grade IV) died. The 12- and 18-month OS rates were 88.1% (95% CI, 77.1–99.1%) and 74.9% (95% CI, 58.2–91.6%), respectively for the entire cohort; and those of the PFS were 74.3% (95% CI, 59.6–89.0%) and 57.3% (95% CI, 38.7–75.9%), respectively.
Perfusion parameter was further analyzed for association with PFS and OS in both uni- and multi-variable analysis. Kaplan-Meier curves documented significant differences of OS and PFS between patients with median nCBVmax (nCBVmax-M) value above and below median cut-off of 6.332 (Figure 2). In multi-variable CPH models after adjusting for age, KPS, WHO grade, surgical intervention, IDH gene and MGMT status, nCBVmax-M remains independently predictive of OS and PFS (Figure 3).
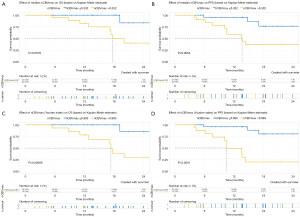
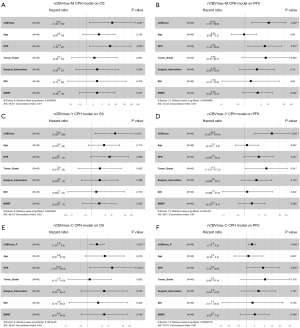
Survival analysis of nCBVmax using You-index method as cut-off
Beyond median value, ROC analysis of 2-year OS and 1-year PFS was conducted to achieve Youden index-derived cut-off values for nCBVmax. The values of area under the curve (AUC) were 0.778 and 0.848 assessed as for nCBVmax on OS and PFS (Figure 4), respectively. Both ROC models showed the same value of 6.993 as optimal cut-off point of nCBVmax.
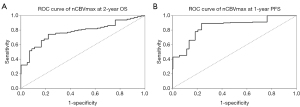
In conjunction with the ROC curve analysis, nCBVmax with the cut-off value derived from Youden index method (nCBVmax-Y) was assessed with the correlation of survival. Kaplan-Meier curves showed that nCBVmax-Y was statistically associated with reduced PFS and OS (Figure 2). Additionally, as illustrated in Figure 3, nCBVmax-Y remained robustly correlated with both OS and PFS in multivariate analysis.
Survival analysis of nCBVmax-C
Another CPH model was built consisted of nCBVmax-C, age, KPS, WHO grade, surgical intervention, IDH gene and MGMT status. Strong independently predictive value of nCBVmax-C was also indicated (Figure 3). All CPH models from nCBVmax-M, nCBVmax-Y and nCBVmax-C identified nCBVmax as an independent predictor of both OS and PFS in HGG patients underwent PBRT.
Analysis of individual survival prediction
To individualize the predicted OS and PFS probability for HGG patients underwent PBRT, nomograms on basis of the results of the above CPH models (Figure 5). The individual survival prediction performance was quantified with respect to discrimination (C-index plot) and calibration (calibration curves), as shown in Figure 6. All CPH models present similar performance in predicting individual OS and PFS.
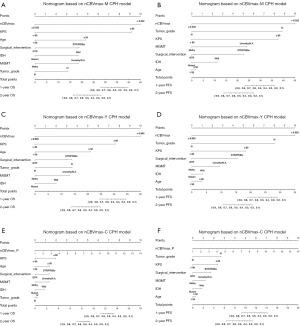
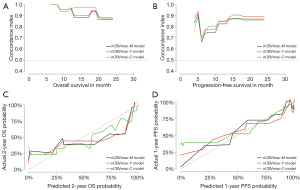
Discussion
High CBV values have been shown to be associated with shorter survival of glioma in numerous studies (11-17,27-29), while some studies indicated that CBV was not predictive of prognosis (18,19). Indeed, traditional CBV estimates vary with postprocessing algorithm, particularly referring to the measurement of CBV ratio of lesion to normal brain tissue (normalization) and leakage correction. Typically, normalization utilizing white matter method is performed whereby nCBV is divided by the value in a manually selected region of interest (ROI) of the contralateral normal-appearing white matter (NAWM); while a roughly 25–30% variation has been reported in NAWM CBV measurement in glioma (23,30). Leakage correction occupies another important role affecting the accurate estimates of CBV. During perfusion MR scan, extravasation of low concentration of contrast agent is inevitable due to severely compromised blood-brain barrier, and is associated with underestimation of nCBV in HGG (14).
In this study, the nCBV was generated with automatic normalization and leakage correction. In brief, the process of leakage correction used a tissue residual function method to eliminate the confounding effect of contrast agent extravasation (22); normalization was done by dividing all pixels on the value of the corresponding normal tissue, which was found by segmenting the brain using a clustering algorithm. This image post-processing procedure is highly automated without manual selection of reference (normal) tissue. Conventional manual methods generally have limitations concerning observer-dependent measurement of nCBV value with objectivity and reproducibility. In contrast, the method in this study could significantly reduce the variability in the assessment, making it more attractive and feasible in a clinical setting (24).
Selective analysis of perfusion parameters within non-enhancing and/or enhancing area partially or entirely on structural MR images is usually performed in conventional approach, which is concerning with bias in nature. Hot-spot measurement without regard to whether nCBVmax displayed in the enhancing or non-enhancing area was chosen in this study. The reasons are: (I) since resection level in HGG is mainly judged by removal of enhancing tumor component, and nCBVmax is not always within the enhancing part; (II) this approach does not need subjective definition of a ROI area for assessing nCBVmax, thereby is more reproducible and less time-consuming.
The most important finding of this study is that nCBVmax prior to PBRT help to predict OS and PFS in both univariate and multivariate analysis. Noticeably, the relevance outperformed clinical parameters and molecular markers. The result is consistent with the study reported by Akgoz et al. (14), in which multivariate analysis demonstrated that nCBV before initiating photon-based radiotherapy was the most predictive factor for both OS and PFS in glioblastoma rather than MGMT status, surgical intervention and age. Indeed, for low-grade gliomas, Law et al. also showed that CBV correlated more accurately with PFS than tumor grade (31). We suggest that nCBVmax areas may represent the site of the most malignancy and radio-resistance within heterogeneous HGG. Preliminary evidence supports this hypothesis that components of glioblastoma with high CBV have elevated expression of angiogenesis-related genes with corresponding to invasiveness (32). If it proves to be true, more aggressive treatment designed to target such area, such as radiation boost with higher dose, may be indicated. In this term, a phase I/III trial is ongoing in our center to assess the efficacy of carbon-ion boost plus standard proton radiation strategy guided by multi-modal imagines, including perfusion MR, 18F-FET-PET, MRS (33).
In looking at the clinical parameters and molecular markers with statistically relation to survival in our analysis, KPS was the independent predictors for both PFS and OS in all CPH models; histological grade and MGMT had a trend toward correlation with PFS in nCBVmax-M and/or nCBVmax-Y CPH models; age was indicated of a trend relation to OS in the nCBVmax-C CPH model; neither IDH nor surgical intervention had a correlation with PFS or OS in any CPH model. Our limited sample size probably underestimated the predictive value of these known prognostic factors. However, we have little doubt with the predictive value of resection completeness in the condition of our treatment strategy of delivering carbon-ion boost for tumor residual for incomplete removal of tumor. Again, the future results of our ongoing phase I/III trial can provide valuable information for this issue (33).
We also developed nomograms, based on nCBVmax-M, nCBVmax-Y, and nCBVmax-C CPH models, for obtaining individual survival estimates for HGG patients. Rather than a group estimate on specific patient-level factors, nomogram is provided as an accessible tool for predicting individual survival. Survival-related nomograms for glioblastoma patients have been constructed in previous studies (34-39). These nomograms have got some achievements in survival analysis, but none applied perfusion MR imaging parameters. In our study, fundamental clinical and pathological factors with possible correlation to survival have been included in each nomogram. All these outcome predictive models showed similar performance of excellent concordance and calibration with actual survival. The present study is, to our knowledge, the first study providing nomograms for HGG patients underwent PBRT.
A number of limitations of this study need to be discussed. First, this retrospective study in nature was based on a single institution experience, and the sample size of 45 cases is relatively small. At present, it is hard for us to perform validation with an independent-internal or external data set to address the issues of probably misestimating the nCBV’s prediction power and a possible overfitting of the CPH models’ performance. Hence, our result was limited in a research tool to explore the prediction role of perfusion MR. As with large scale sample of prospective trial going in our institution (33), future results may improve the generalizability of utilizing nCBV as a prognostic marker for the decision-making for PBRT in HGG. Second, despite all HGG patients in our institutional protocol were treated prospectively to 60 Gy (RBE) with or without boost to tumor residual guided by multi-modal images, 2 elderly cases of our 45 patients were treated with a hypo-fractionated strategy (proton beam to 34 Gy (RBE) in 10 fractions followed by carbon-ion boost); nevertheless, the treatments on the entire cohort level were homogenous. Third, there is possibility of histopathologic misdiagnosis attributable to small tissue sample in the condition of patients underwent biopsy/partial resection (PR). However, because only 15 of our 45 patients (33.3%) whose tumor were biopsied or partially removed, we postulate that the incidence of histopathologic misdiagnosis was low in our series. Fourth, for our nomograms, more dedicated models that include other modalities of imaging parameters are needed in the future; and these nomograms need to be validated in a prospective cohort in the next step prior to being formally applied in clinical practice.
Conclusions
The presented retrospective analysis of MR perfusion imaging prior to proton and heavy ion radiation showed that nCBVmax is a strong predictor of survival in HGG. The novel proposed perfusion MR-based nomogram with prospective validation could potentially be formally used in future clinical practice to individual survival probability.
Acknowledgments
Funding: This work was supported by The National Key Research and Development Program of China (Project No. 2017YFC0108603); The Shanghai Academic/Technology Research Leader Program (Project Nos. 19XD1432900 and 18XD1423000); Science and Technology Commission of Shanghai Municipality (Project No. 19411951000); Science and Technology Development Fund of Shanghai Pudong New Area (Project Nos. PKJ2018-Y51, PKJ2017-Y54, PKJ2017-Y50 and PKJ2017-Y49).
Footnote
Conflicts of Interest: All authors have completed the ICMJE uniform disclosure form (available at https://atm.amegroups.com/article/view/10.21037/atm-20-1646/coif). The authors have no conflicts of interest to declare.
Ethical Statement: The authors are accountable for all aspects of the work in ensuring that questions related to the accuracy or integrity of any part of the work are appropriately investigated and resolved. The study was conducted in accordance with the Declaration of Helsinki (as revised in 2013). The study was approved by the ethics committee at Shanghai Proton and Heavy Ion Center [approval number: SPHIC-HNCNS-2019-09 (RS)]. Written informed consent was obtained from all patients.
Open Access Statement: This is an Open Access article distributed in accordance with the Creative Commons Attribution-NonCommercial-NoDerivs 4.0 International License (CC BY-NC-ND 4.0), which permits the non-commercial replication and distribution of the article with the strict proviso that no changes or edits are made and the original work is properly cited (including links to both the formal publication through the relevant DOI and the license). See: https://creativecommons.org/licenses/by-nc-nd/4.0/.
References
- Louis DN, Perry A, Reifenberger G, et al. The 2016 World Health Organization Classification of Tumors of the Central Nervous System: a summary. Acta Neuropathol 2016;131:803-20. [Crossref] [PubMed]
- Stupp R, Mason WP, van den Bent MJ, et al. Radiotherapy plus concomitant and adjuvant temozolomide for glioblastoma. N Engl J Med 2005;352:987-96. [Crossref] [PubMed]
- Schlaich F, Brons S, Haberer T, et al. Comparison of the effects of photon versus carbon ion irradiation when combined with chemotherapy in vitro. Radiat Oncol 2013;8:260. [Crossref] [PubMed]
- Combs SE, Bruckner T, Mizoe JE, et al. Comparison of carbon ion radiotherapy to photon radiation alone or in combination with temozolomide in patients with high-grade gliomas: explorative hypothesis-generating retrospective analysis. Radiother Oncol 2013;108:132-5. [Crossref] [PubMed]
- Kong L, Gao J, Qiu X, et al. Proton and carbon-ion radiotherapy for high grade glioma: early results of the Shanghai Proton and Heavy Ion Center (SPHIC). Int J Radiat Oncol Biol Phys 2019;103:E8. [Crossref]
- Nguyen HN, Lie A, Li T, et al. Human TERT promoter mutation enables survival advantage from MGMT promoter methylation in IDH1 wild-type primary glioblastoma treated by standard chemoradiotherapy. Neuro Oncol 2017;19:394-404. [PubMed]
- Gutt-Will M, Murek M, Schwarz C, et al. Frequent diagnostic under-grading in isocitrate dehydrogenase wild-type gliomas due to small pathological tissue samples. Neurosurgery 2019;85:689-94. [Crossref] [PubMed]
- Sottoriva A, Spiteri I, Piccirillo SG, et al. Intratumor heterogeneity in human glioblastoma reflects cancer evolutionary dynamics. Proc Natl Acad Sci U S A 2013;110:4009-14. [Crossref] [PubMed]
- Verhaak RG, Hoadley KA, Purdom E, et al. Integrated genomic analysis identifies clinically relevant subtypes of glioblastoma characterized by abnormalities in PDGFRA, IDH1, EGFR, and NF1. Cancer Cell 2010;17:98-110. [Crossref] [PubMed]
- Leimgruber A, Hickson K, Lee ST, et al. Spatial and quantitative mapping of glycolysis and hypoxia in glioblastoma as a predictor of radiotherapy response and sites of relapse. Eur J Nucl Med Mol Imaging 2020;47:1476-85. [Crossref] [PubMed]
- Mangla R, Singh G, Ziegelitz D, et al. Changes in relative cerebral blood volume 1 month after radiation-temozolomide therapy can help predict overall survival in patients with glioblastoma. Radiology 2010;256:575-84. [Crossref] [PubMed]
- Cao Y, Tsien CI, Nagesh V, et al. Survival prediction in high-grade gliomas by MRI perfusion before and during early stage of RT Int J Radiat Oncol Biol Phys 2006;64:876-85. [corrected]. [Crossref] [PubMed]
- Li Y, Lupo JM, Polley MY, et al. Serial analysis of imaging parameters in patients with newly diagnosed glioblastoma multiforme. Neuro Oncol 2011;13:546-57. [Crossref] [PubMed]
- Akgoz A, Rahman R, You H, et al. Spin-echo echo-planar perfusion prior to chemoradiation is a strong independent predictor of progression-free and overall survival in newly diagnosed glioblastoma. J Neurooncol 2014;119:111-9. [Crossref] [PubMed]
- Hirai T, Murakami R, Nakamura H, et al. Prognostic value of perfusion MR imaging of high-grade astrocytomas: long-term follow-up study. AJNR Am J Neuroradiol 2008;29:1505-10. [Crossref] [PubMed]
- Kim JH, Choi SH, Ryoo I, et al. Prognosis prediction of measurable enhancing lesion after completion of standard concomitant chemoradiotherapy and adjuvant temozolomide in glioblastoma patients: application of dynamic susceptibility contrast perfusion and diffusion-weighted imaging. PLoS One 2014;9:e113587. [Crossref] [PubMed]
- Burth S, Kickingereder P, Eidel O, et al. Clinical parameters outweigh diffusion- and perfusion-derived MRI parameters in predicting survival in newly diagnosed glioblastoma. Neuro Oncol 2016;18:1673-9. [Crossref] [PubMed]
- Mills SJ, Patankar TA, Haroon HA, et al. Do cerebral blood volume and contrast transfer coefficient predict prognosis in human glioma? AJNR Am J Neuroradiol 2006;27:853-8. [PubMed]
- Oh J, Henry RG, Pirzkall A, et al. Survival analysis in patients with glioblastoma multiforme: predictive value of choline-to-N-acetylaspartate index, apparent diffusion coefficient, and relative cerebral blood volume. J Magn Reson Imaging 2004;19:546-54. [Crossref] [PubMed]
- Antonovic L, Lindblom E, Dasu A, et al. Clinical oxygen enhancement ratio of tumors in carbon ion radiotherapy: the influence of local oxygenation changes. J Radiat Res 2014;55:902-11. [Crossref] [PubMed]
- Strigari L, Torriani F, Manganaro L, et al. Tumour control in ion beam radiotherapy with different ions in the presence of hypoxia: an oxygen enhancement ratio model based on the microdosimetric kinetic model. Phys Med Biol 2018;63:065012. [Crossref] [PubMed]
- Bjornerud A, Sorensen AG, Mouridsen K, et al. T1- and T2*-dominant extravasation correction in DSC-MRI: part I--theoretical considerations and implications for assessment of tumor hemodynamic properties. J Cereb Blood Flow Metab 2011;31:2041-53. [Crossref] [PubMed]
- Ellingson BM, Zaw T, Cloughesy TF, et al. Comparison between intensity normalization techniques for dynamic susceptibility contrast (DSC)-MRI estimates of cerebral blood volume (CBV) in human gliomas. J Magn Reson Imaging 2012;35:1472-7. [Crossref] [PubMed]
- Qin L, Li X, Li A, et al. Clinical validation of automatable gaussian normalized CBV in brain tumor analysis: superior reproducibility and slightly better association with survival than current standard manual normal appearing white matter normalization. Transl Oncol 2018;11:1398-405. [Crossref] [PubMed]
- Wen PY, Macdonald DR, Reardon DA, et al. Updated response assessment criteria for high-grade gliomas: response assessment in neuro-oncology working group. J Clin Oncol 2010;28:1963-72. [Crossref] [PubMed]
- Ellingson BM, Wen PY, Cloughesy TF. Modified criteria for radiographic response assessment in glioblastoma clinical trials. Neurotherapeutics 2017;14:307-20. [Crossref] [PubMed]
- Deike K, Wiestler B, Graf M, et al. Prognostic value of combined visualization of MR diffusion and perfusion maps in glioblastoma. J Neurooncol 2016;126:463-72. [Crossref] [PubMed]
- Rau MK, Braun C, Skardelly M, et al. Prognostic value of blood flow estimated by arterial spin labeling and dynamic susceptibility contrast-enhanced MR imaging in high-grade gliomas. J Neurooncol 2014;120:557-66. [Crossref] [PubMed]
- Wahl DR, Kim MM, Aryal MP, et al. Combining perfusion and high B-value diffusion MRI to inform prognosis and predict failure patterns in glioblastoma. Int J Radiat Oncol Biol Phys 2018;102:757-64. [Crossref] [PubMed]
- Wetzel SG, Cha S, Johnson G, et al. Relative cerebral blood volume measurements in intracranial mass lesions: interobserver and intraobserver reproducibility study. Radiology 2002;224:797-803. [Crossref] [PubMed]
- Law M, Oh S, Babb JS, et al. Low-grade gliomas: dynamic susceptibility-weighted contrast-enhanced perfusion MR imaging--prediction of patient clinical response. Radiology 2006;238:658-67. [Crossref] [PubMed]
- Jain R, Poisson L, Narang J, et al. Correlation of perfusion parameters with genes related to angiogenesis regulation in glioblastoma: a feasibility study. AJNR Am J Neuroradiol 2012;33:1343-8. [Crossref] [PubMed]
- Kong L, Gao J, Hu J, et al. Carbon ion radiotherapy boost in the treatment of glioblastoma: a randomized phase I/III clinical trial. Cancer Commun (Lond) 2019;39:5. [Crossref] [PubMed]
- Gittleman H, Lim D, Kattan MW, et al. An independently validated nomogram for individualized estimation of survival among patients with newly diagnosed glioblastoma: NRG Oncology RTOG 0525 and 0825. Neuro Oncol 2017;19:669-77. [PubMed]
- Zhang X, Lu H, Tian Q, et al. A radiomics nomogram based on multiparametric MRI might stratify glioblastoma patients according to survival. Eur Radiol 2019;29:5528-38. [Crossref] [PubMed]
- Peeken JC, Goldberg T, Pyka T, et al. Combining multimodal imaging and treatment features improves machine learning-based prognostic assessment in patients with glioblastoma multiforme. Cancer Med 2019;8:128-36. [Crossref] [PubMed]
- Molitoris JK, Rao YJ, Patel RA, et al. Multi-institutional external validation of a novel glioblastoma prognostic nomogram incorporating MGMT methylation. J Neurooncol 2017;134:331-8. [Crossref] [PubMed]
- Gittleman H, Cioffi G, Chunduru P, et al. An independently validated nomogram for isocitrate dehydrogenase-wild-type glioblastoma patient survival. Neurooncol Adv 2019;1:vdz007. [Crossref] [PubMed]
- Cheng W, Zhang C, Ren X, et al. Treatment strategy and IDH status improve nomogram validity in newly diagnosed GBM patients. Neuro Oncol 2017;19:736-8. [Crossref] [PubMed]