Comparative evaluation of peptidome and microbiota in different types of saliva samples
Introduction
Recent advances in salivary diagnostics indicate that saliva holds promise for the screening of oral and systemic health problems as a favorable diagnostic medium (1-7). Saliva has the potential to serve as a first-line diagnostic medium since it offers analytes alternative to other traditional body fluids, directing an easy and noninvasive collection procedure with little risk of cross-infection (8,9). The development of novel technologies helps researchers and clinicians unravel the complex interactions between salivary biomolecules (e.g., DNA, mRNA, microRNA, proteins, metabolites and microbes) and the human body, making saliva available for early diagnosis, risk assessment, and prediction for prognosis (1-7).
Among salivary analytes, the analysis of low-molecular-weight-fraction proteome (peptidome) (10) and microbiota (11) are becoming more and more important for the oral cavity, and even for the whole body. Salivary peptidome, which contains endogenous bioactive peptides, shed proteins and protein fragments (12) and has the ability to enter the circulation as the products of cell differentiation, neurohormone transmitter regulation, inflammation, tumorigenesis, and immune cell infiltration, is carrying a lot of disease-related information of physiologic and pathologic events taking place in all perfused tissues (12-14). For this reason, salivary peptidome is now acknowledged as a novel reservoir for candidate biomarkers with advanced methodology (e.g., mass spectrometry) introduced (15), which endows saliva with great potentiality to be used for clinical purposes as an alternative to other body fluids such as blood and urine. Meanwhile, saliva samples have been regarded as a representative reflection of the entire ecosystem of the oral cavity (16), which contains a number of different habitats and harbors the second most abundant microbiota. Dysbiosis or imbalance of the oral microbiota contributes to oral and systematic diseases, and these diseases could shape the oral microbial community in a counteractive manner (17). Currently, the whole genome sequences of numerous oral species have been decoded, 70% of which are proved to be cultivable in vitro (18). Based on the above, the oral cavity turns into a leading model for studying the mechanisms of microbe-host and microbe-microbe interactions. As the technologies and relevant studies keep ever-developing, salivary peptidome and microbiota has caught more and more attentions from researchers in this field.
The three major salivary glands (parotid, submandibular and sublingual glands), in conjunction with numerous minor glands, are capable to produce more than 95% of the saliva (19). The mixed whole saliva, including those collected under unstimulated or stimulated conditions, is thought to be the best option which is practically feasible in clinical circumstances for saliva collection on a patient basis (20-22). On account of its significantly faster and more comfortable sampling procedure, stimulated saliva become more frequently used, especially favorable for the mass screening programs (20-22). For certain populations with insufficient saliva flow rate, such as the geriatric people (23) and patients with xerostomia (24), stimulated methods are regarded as the optimal choice for saliva sampling, which has the advantage of easier laboratory process by virtue of its lower mucin content and capability to offer more accurate analysis (25).
Generally, saliva can be stimulated in response to either masticatory or gustatory stimulants (22), among which flavorless paraffin wax and citric acid productions are the most frequently used triggers (22,26,27). Besides, the strategy to augment saliva flow using the smell of food, namely the olfactory stimulant (28), is considered as an approach with certain advantages given its sampling procedures interfere less with the oral cavity. Although stimulated saliva sampling has received growing clinical and research attentions, currently there are no studies systematically addressing the similarities and differences of salivary peptidome and microbiota in different types of samples.
Therefore, the purpose of this study was to directly compare salivary peptidome and microbial profiles in four types of saliva samples collected under unstimulated condition and in response to olfactory, gustatory, and masticatory triggers from systematically and orally healthy individuals, with the techniques of matrix-assisted laser desorption/ionization-time of flight-mass spectrometry (MALDI-TOF MS) and 16S rDNA amplicon high-throughput sequencing employed. We present the following article in accordance with the STROBE reporting checklist (available at http://dx.doi.org/10.21037/atm-20-393).
Methods
Ethics approval and informed consent
This study was approved by the Peking University School and Hospital of Stomatology Ethics Committee (PKUSSIRB-201944061) and conformed to the STROBE guidelines, with the informed consent signed by all the participants before it commenced.
Study population and design
Subjects were recruited from the Peking University School and Hospital of Stomatology from May to June 2018. After the review of medical history and oral clinical examination, 10 individuals (5 females and 5 males) were enrolled in the present study. The donors were enrolled according to the following criteria: (I) more than 18 years old; (II) no reported systematic diseases; (III) no history of antibiotic therapy within the last 3 months; (IV) currently not pregnant or lactating; (V) no smoking and alcohol-drinking habits; (VI) no evidence of untreated dental caries, periodontal diseases, or other oral diseases. The flow chart of this study was shown in Figure 1.
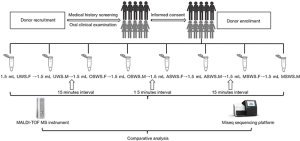
Sampling and processing of saliva
Donors were requested not to eat, brush teeth, drink, exercise, or chew gum for at least 2 hours before saliva sampling to minimize the probable risk of contamination (29). The participants were instructed to rinse their mouth thoroughly with deionized water and then rest for at least 10 minutes (sitting upright) before saliva collection at 9:00–10:00 a.m. Whole saliva samples were collected into a graduated polypropylene tube (Eppendorf, Hamburg, Germany) through a funnel (Zhenqi, Shanghai, China) with the four selected collection methods as follows: First, 1.5 mL unstimulated forepart whole saliva was collected by passive drooling (UWS.F), followed immediately by 1.5 mL unstimulated midstream whole saliva (UWS.M); Second, after a fifteen-minute interval for rest, 1.5 mL olfaction stimulated forepart whole saliva was collected using fresh lemon juice as the stimulant (OSWS.F), while 1.5 mL olfaction stimulated midstream whole saliva was collected straight after the forepart segment (OSWS.M); then, after another fifteen-minute interval for rest, 1.5 mL acid stimulated forepart whole saliva was collected using a citric acid swab (0.4 mol/L) as the stimulant (ASWS.F), followed immediately by 1.5 mL acid stimulated midstream whole saliva collected with the same stimulant (ASWS.M). Last, after a fifteen-minute interval for rest again, 1.5 mL mastication stimulated forepart whole saliva was collected using a paraffin gum as the stimulant (MSWS.F), followed immediately by 1.5 mL mastication stimulated midstream whole saliva collected with the same stimulant (MSWS.M). Most previous studies used the volume of 1–2 mL for saliva sampling. Thus, the 1.5 mL-forepart and 1.5 mL-midstream sampling under the same condition was regarded as a representative repeated collection process.
The flow rate was calculated by measuring the actual volume of a saliva sample and dividing it by the time taken to collect the sample. The saliva samples were immediately placed on ice and transferred to the laboratory for processing as soon as possible (within 2 hours). Saliva samples were centrifuged at 10,000 ×g for 10 minutes at 4 °C. The pellets were stored at −80 °C before DNA extraction. The supernatants were divided into 150 µL aliquots. Two separate aliquots were supplemented with a proteome stabilization treatment (30) and all the aliquots were stored at −80 °C until further analysis.
Peptidome detection and data processing
All samples were fractioned using a weak cation exchange magnetic bead (WCX MB) kit (Bioyong Tech, Beijing, China). Samples were isolated and purified by the following steps: (I) 150 µL of WCX MB binding solution, 20 µL of beads, and 10 µL of sample were mixed carefully and incubated for 5 min at room temperature; (II) the tubes were placed on the WCX MB separation device for 1 min to collect the beads onto the tube wall, and then the supernatant was removed; (III) the beads were washed by 150 µL washing solution. 2 min later, the tubes were placed on the separation device for 1 min; (IV) step 3 was repeated and all the supernatant was removed; (V) 10 µL of WCX MB elution solution was added, and the beads were allowed to gather on the tube wall in the separation device for 2 min; (VI) The clear supernatant was transferred to a new tube, and the peptides were analyzed immediately on a MALDI-TOF MS instrument (Bioyong Tech).
The matrix solution was 8 mg/mL CHCA in 50% acetonitrile/0.1% TFA/49.9% deionized water. First, 1 µL of the purified peptide solution was spotted onto a MALDI-TOF MS target and then dried at room temperature. Then 1 µL of the matrix solution was spotted to cover the sample and then dried again. Before analysis, we employed a three-peptide mixture (monoisotopic molecular weight of 1,533.8582, 2,465.1989 and 5,730.6087 Da; Sigma product numbers P2613, A8346 and I6279, respectively) to calibrate the mass spectrometry. Profile spectra were obtained from an average of 400 laser shots per sample. The mass-to-charge ratio (m/z) values in the range of 1,000–10,000 were collected. Each sample was analyzed in triplicate to acquire the average spectra for each sample. All the experiments were conducted by the same technician without changing reagent lots, standards, or control materials to minimize analytical variation. The above methodology has been utilized and validated successfully in the previous studies by our research group (31-34).
All the spectra obtained from the samples were analyzed by BioExplorer 1.0 (Bioyong Tech). The mean intensity of technical repetitions was determined and chemical/electrical noises was subtracted. Then, the spectra was normalized by applying the total ion current, then peak m/z values and intensities were determined in the mass range of 1,000–10,000. A signal-to-noise ratio >5 was required. To align the spectra, a mass shift of no more than 0.1% was determined.
Microbiota detection and data processing
The microbial DNA extraction was performed using the QIAamp DNA Microbiome Kit (Qiagen, Hilden, Germany) according to the manufacturer’s instructions. Concentration and purity testing of the DNA was performed using a Qubit 4 Fluorometer (Invitrogen, Thermo Scientific, Wilmington, DE, USA). The integrity of bacterial genomic DNA was checked by 1% agarose gel electrophoresis and a negative control only with buffer was used. The DNA samples were stored at −80 °C until further use.
Universal primers for 16S rDNA double V3-V4 region were used to conduct PCR amplification (338F 5'-ACTCCTACGGGAGGCAGCA-3', 806R 5'-GGACTACHVGGGTWTCTAAT-3'). The cycling conditions were carried out as follows: initial denaturation at 94 °C for 5 minutes, 30 cycles consisting of denaturation at 95 °C for 30 seconds, annealing at 56 °C for 30 seconds, elongation at 72 °C for 40 seconds, and final extension at 72 °C for 10 minutes. A negative control only with buffer was enrolled during DNA amplification to eliminate interference. The quality of the amplified PCR products was detected by 2% agarose gel electrophoresis. The purification of PCR products was performed using a QIAquick Gel Extraction Kit (QIAGEN, Hilden, Germany). The purified PCR products were quantified using a real-time PCR system. Illumina MiSeq 300 bp paired-end (PE) sequencing platform (Illumina, San Diego, CA, USA) was used for amplified products according to standard operating protocols.
The Quantitative Insights Into Microbial Ecology (QIIME, v1.8.0) pipeline was employed to process the sequencing data, as previously described (35). Briefly, raw sequencing reads with exact matches to the barcodes were assigned to the respective samples and identified as valid sequences. The low-quality sequences were filtered in accordance with the following criteria (36,37): sequences that had a length of <150 bp, sequences that had average Phred scores of <20, sequences that contained ambiguous bases, and sequences that contained mononucleotide repeats of >8 bp. Paired-end reads were assembled using FLASH (38). After chimera detection, the remaining high-quality sequences were clustered into operational taxonomic units (OTUs) at 97% sequence identity by UCLUST (39). A representative sequence was selected from each OTU using default parameters. OTU taxonomic classification was conducted by BLAST searching the representative sequences set against the HOMD database as reference (40). An OTU table was further generated to record the abundance of each OTU in each sample and the taxonomy of these OTUs. OTUs containing less than 0.001% of total sequences across all samples were discarded. To minimize the difference of sequencing depth across samples, an averaged, rounded rarefied OTU table was generated by averaging 100 evenly resampled OTU subsets under the 90% of the minimum sequencing depth for further analysis.
Sequence data analyses were mainly performed using QIIME and R packages (v3.2.0). OTU-level alpha diversity indices, such as the Shannon index, were calculated using the OTU table in QIIME. The taxonomy compositions and abundances were visualized using GraPhlAn (41). Venn diagram was generated to visualize the shared and unique OTUs among samples or groups using R package “VennDiagram”, based on the occurrence of OTUs across samples/groups regardless of their relative abundance.
Statistical analysis
For the salivary peptidome analysis, the Bray-Curtis distance matrix was calculated based on the peak intensity profile. The comparisons represented for the variation introduced by the change of segment within each saliva type (evaluation for repeated collection) and the variation introduced by the change of sampling condition (evaluation for determined stimulants) were visualized via principal coordinate analysis (PCoA) and hierarchical clustering analysis by unweighted pair-group method with arithmetic means (UPGMA) based on the Bray-Curtis distance matrix. The intra-individual distances introduced by the change of stimulants and repeated collection were extracted and compared using the paired t-test. For the salivary microbiota analysis, the weighted UniFrac distance metrics were generated (42,43), the subsequent analysis process is the same as the peptidome except the distance algorithm was different. P<0.05 was regarded as the threshold for statistical significance (two-sided).
Data availability
The raw sequencing data of this study are available in the NCBI Sequence Read Archive with accession number SRP227657, and the raw mass spectrometry data were uploaded as a supplementary material.
Results
Comparison of flow rate for different types of saliva samples
A total of 10 systematically and orally healthy donors were enrolled in this study, whose socio-demographic background and oral health status were shown in Table S1.
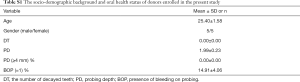
Full table
The comparative analysis of salivary flow rates was conducted using the paired t-test. As shown in Figure S1A, no significant difference was found in the flow rates between the forepart and midstream segments under the same sampling conditions (N=10 vs. 10; P=0.551, 0.691, 0.084, 0.105, respectively). Significant differences were found in the flow rates when compared between the stimulated and unstimulated conditions (P<0.001 for olfactory stimulant, P=0.002 for gustatory stimulant, P<0.001 for masticatory stimulant, respectively) (Figure S1B). Of the three specific stimulants, olfaction exhibited the weakest capability to augment saliva flow.
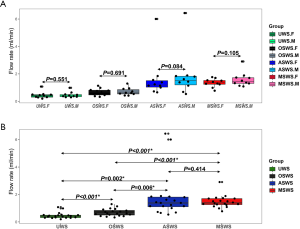
Comparison of peptidome profiles in different types of saliva samples
Salivary supernatants were analyzed by MALDI-TOF MS, and peptide fingerprints were obtained in the m/z range of 1,000–10,000. The visual differences of salivary peptidome were displayed in the virtual gel electrophoresis (Figure S2) and peptide fingerprints (Figure S3). The signals in OSWS was similar to that in UWS, and ASWS exhibited simpler signals than UWS, while MSWS showed more complicated signals than UWS. Meanwhile, it was observed that the peptidome components were diluted under the three stimulated conditions, especially for MSWS.
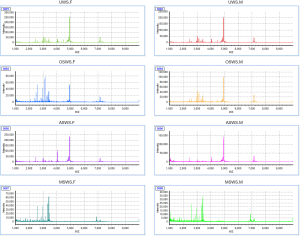
The holistic comparisons on salivary peptidome profiles among different types of saliva samples were conducted based on the Bray-Curtis distance and the results were visualized via PCoA (Figure S4), which indicated that repeated collections under the same sampling condition presented an unseparated tendency, while the four types of saliva samples exhibited different levels of separation. The UPGMA hierarchical clustering analyses based on the Bray-Curtis distance were then employed for comparisons between the repeated collections for each type of saliva samples (Figure 2A) and among different sampling conditions for each segment (Figure 2B). The results demonstrated that salivary peptidome tended to aggregate in terms of the donor (Figure 2A), indicating that the variations introduced by repeated collection contributed little to the composition of salivary peptidome for each type of saliva sample. However, the cluster trees shown in Figure 2B indicated that the variations brought by different sampling conditions would significantly affect the composition of salivary peptidome.
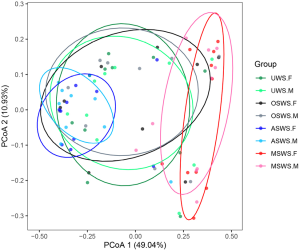
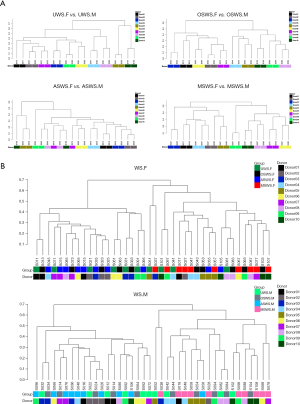
Based on the present findings, a schema diagram showing the intra-individual distances of salivary peptidome profiles between different segments within the same sampling condition (Distance 1–4) and between different sampling conditions within the same segment (Distance 5–10 for forepart segment and Distance 11–16 for midstream segment) was established (Figure 3A). The intra-individual Bray-Curtis distances under the circumstances of different sampling conditions and repeated collections were sorted and compared using the paired t-test (Figure 3B). The results of statistical analyses demonstrated the variations brought by different sampling conditions were significantly higher than those introduced by repeated collections, except for the comparison between OSWS and UWS.
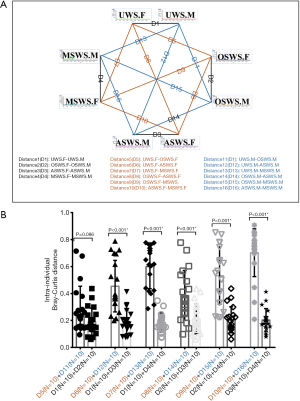
Comparison of microbial profiles in different types of saliva samples
The sequencing method generated a total of 2,820,236 sequences after quality filtering, with an average of 35,253 (ranged from 27,789 to 53,479) sequences per sample. The species richness of the salivary microbiota of each sample was estimated by rarefaction analysis (Figure S5). The shape of the rarefaction curves evidenced the complete achievement of a plateau, indicating that the sequencing depth of all the samples was reasonable. The number of shared and specific OTUs among the four sampling conditions for the forepart and midstream segments were shown in Figure S6.
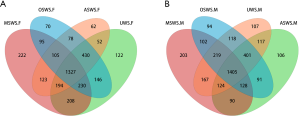
With the analyses of salivary microbiota in all the samples, a total of 11 phyla, 20 classes, 34 orders, 72 families, 148 genera and 379 species were detected. The overall structure of the salivary microbiota from phylum to species levels in different types of saliva samples was shown in Figure S7, which legends exhibited the top-20 taxa in terms of the mean relative abundance. Comparative evaluation of saliva sampling conditions for microbial diversity index (Shannon index referring to both richness and evenness) was shown in Figure S8, which indicated that saliva samples collected without mechanical chewing (UWS, OSWS and ASWS) had almost equivalent Shannon index, whereas a higher level of microbial diversity was found in saliva samples in response to mechanical chewing stimulation.
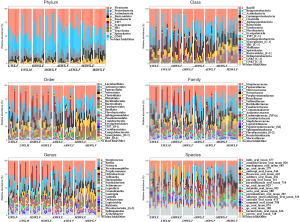
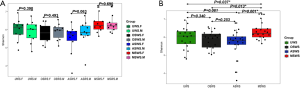
Similar with the procedures we have performed on salivary peptidome profiles, the holistic comparisons on salivary microbial profiles among different types of saliva samples were conducted based on the weighted UniFrac distance and the results were visualized via PCoA as well (Figure S9), which indicated that repeated collections under the same sampling condition presented an unseparated tendency, while the four types of saliva samples exhibited different levels of separation. The UPGMA hierarchical clustering analyses based on the weighted UniFrac distance were then employed for comparisons between the repeated collections for each type of saliva samples (Figure 4A) and among different sampling conditions for each segment (Figure 4B). Similarly with the findings of salivary peptidome, we demonstrated that salivary microbiota tended to aggregate in terms of the donor (Figure 4A), indicating that repeated saliva sampling under the same sampling condition had comparable microbial profiles. Nevertheless, the cluster trees shown in Figure 4B indicated that sampling conditions had a greater influence than the individual specificity, which resulted in significant changes of salivary microbial profiles.
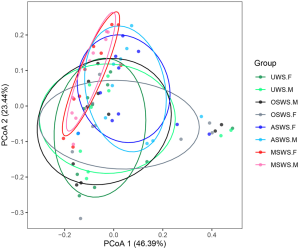
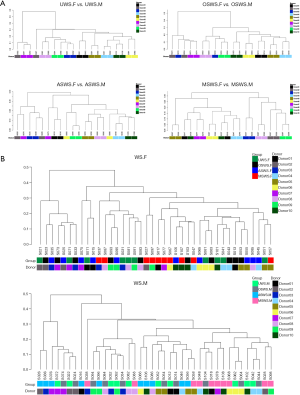
Similarly, a schema diagram showing the intra-individual distances of salivary microbial profile between repeated collections within the same sampling condition (Distance 1–4) and between different sampling conditions within the same segment (Distance 5–10 for forepart segment and Distance 11–16 for midstream segment) was established (Figure 5A). The intra-individual weighted UniFrac distances under the circumstances of different sampling conditions and repeated collections were sorted and compared using the paired t-test (Figure 5B). The results of statistical analyses demonstrated the variations brought by different sampling conditions were significantly higher than those introduced by repeated collections, except for the comparison between OSWS and UWS. These findings indicated that interference within the mouth, like acid and chewing triggers, could lead to a perturbation of the salivary microbial structure.
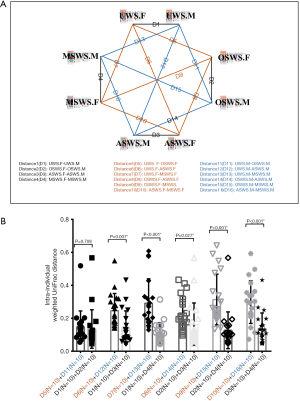
Discussion
Although challenges remain ahead, the rise of salivary diagnostics appears to hold promise to revolutionize the next generation of diagnostics (1-7). Clinical interest of salivary peptidome and microbiota has been growing in the areas of diagnosis and prognosis since they were suggested as candidate biomarkers associated with both oral and systemic health status (10,11,44). A number of salivary diagnostic studies have regarded the stimulated saliva as a representative reflection of the entire ecosystem of the oral cavity for its certain advantages. As we know, the most important pre-analytical step for successful measurement of salivary analytes is to choose the optimal sampling procedures. In this context, sampling variability introduced by collection methods should be kept at a minimum level or interpreted explicitly for reproducible analyses of salivary analytes. However, there were few comparative studies addressing the variations of peptidome and microbiota among different types of saliva samples. Hence, the significance of this study was to investigate the variations of peptidome and microbial profiles for the four different types of saliva samples (unstimulated versus olfaction, gustation and mastication stimulated saliva) using high-throughput techniques.
Considering the characteristics of interference within the mouth caused by selected sampling conditions, the order of sampling conditions adopted in the present study was set as UWS, OSWS, ASWS and MSWS. Olfactory stimulation had no direct interference to the oral environment, by which collection method the salivary peptidome was also comparable with unstimulated condition (UWS) in the present study. The most evident drawback of ASWS was the lower pH value which might affect some parameters such as its buffering capacity (45), resulting in the manifestation of simpler signals than the other three groups, downregulation of enzymatic activity of proteolysis, and finally the reduction of peptidomic richness. Since the acid load would be diluted as saliva flowed out, a constant flow rate was hard to elicit for ASWS, which is also confirmed in our findings that repeated collections led to the most obvious variations in salivary peptidome of ASWS (Figure 2A). With more complicated signals, peptidome in MSWS was also quite different from its counterparts in both UWS and ASWS, which is consistent with a previous study (Golatowski et al. 2013) reported that the composition of salivary proteins depended on mastication effect (46).
In the present study, the mean Shannon index in the sequencing analysis for salivary microbiota was around 6.0, which magnitude was significantly higher than previously reported in other sequencing studies conducted in healthy adults (around 4.0–5.0) (47-49). This was most likely owing to the QIAamp DNA Microbiome kit, which could optimize mechanical and chemical cell lysis to improve the ability of bacterial DNA isolation (50). Based on our findings, it could be inferred that chewing stimulation does increase the level of microbial diversity in saliva samples, whereas the median level of ASWS was lower than the other three groups but no statistical differences presented (Figure S8). We think the shorter collection time and the still flow path might contribute to the decrease in the median level of ASWS. As for OSWS, both the level of microbial diversity and community structure were comparable with that of UWS, which could also be attributed to the similar outflowing way of saliva and no interference with the oral cavity in the sampling procedures. Our findings indicated interference within the mouth could lead to a perturbation of the salivary microbial structure, which coincided with Gomar-Vercher et al.’s findings that masticatory stimulated and unstimulated saliva samples have significantly different bacterial profiles (51), but seemed contradictory to another previous study by Belstrøm et al. which verified that masticatory stimulated saliva was an adequate surrogate of unstimulated saliva for microbiome-related studies (52). This conflict of conclusion could be ascribed to certain shortcomings such as the low throughput of detection methods and underdeveloped analyzing methodologies in these previous studies, hence we are more confident with our present findings that saliva samples collected with and without masticatory stimulation would have appreciable differences in microbiota profiles.
Nevertheless, our findings must be interpreted in the context of study limitations as well, which may provide certain enlightenments for future research directions. First, as the present findings were all based on healthy persons, cautions are necessary for further extrapolation, particularly for those with systemic or oral diseases. For diseases that do not affect the secretion and filtration of salivary glands, it can be analogized that the characteristics of peptidome and microbiota in selected types of saliva samples should be consistent with the findings in our present study. For diseases that do affect the function of salivary glands, which site is affected and its severity will have influence on the selection procedure for saliva type. Further studies will be needed to verify the similarities and differences of peptidome and microbiota for different types of saliva samples, and explore which type of saliva is appropriate for detecting potential biomarkers under these circumstances of diseases. Additionally, though it is supposed that these findings in participants aged 20 years and over could be analogized to the population of other ages, e.g., the geriatric people, further studies are still needed to confirm this assumption. Second, though MALDI-TOF MS is an appropriate platform for the rapid screening test in peptidome studies, there was a lack of absolute quantification and identification of peptide sequences. Even so, we believe the comparisons of overall peptidome were still of clinical significance for the selection of salivary sampling methods since the panel of m/z values had the potentiality to serve as disease indicators (53). The introduction of high-throughput tandem mass spectrometry based on data-independent acquisition to obtain standard quantitative information (54-56) would yield more in-depth results in the future. Third, the sequencing analysis in this study was based on the 16S rDNA double V3-V4 region amplicon sequencing library and Illumina MiSeq PE300 platform, which had difficulty to provide absolute taxa abundance. It is expected that further studies utilizing high-throughput absolute abundance quantification techniques could detect the salivary microbiota at the species level or provide more explicit details in the future (57,58).
In summary, our comparative evaluation of peptidome and microbiota in unstimulated saliva and samples collected with triggers (olfactory, gustatory and masticatory stimulants) has made the following supplementary to the current knowledge: (I) saliva collected in response to olfactory stimulation is an appropriate alternative to unstimulated saliva for peptidome and microbiota-related studies; (II) the gustatory and masticatory stimulants could lead to appreciable variations of salivary peptidome and microbiota when comparing with the unstimulated condition; (III) with regards to the significantly different peptidome and microbial profiles among different types of saliva samples, it is suggested that only one type of stimulating method should be used throughout one peptidome/microbiome research.
Acknowledgments
The authors acknowledge all participants in this study.
Funding: The work was supported by the National Natural Science Foundation of China (grant number: 81801037), Beijing Municipal Science and Technology Commission (grant number: Z181100001618015), and the Ministry of Science and Technology of the People’s Republic of China (grant number: 2018FY101005).
Footnote
Reporting Checklist: The authors have completed the STROBE reporting checklist. Available at http://dx.doi.org/10.21037/atm-20-393
Data Sharing Statement: Available at http://dx.doi.org/10.21037/atm-20-393
Conflicts of Interest: All authors have completed the ICMJE uniform disclosure form (available at http://dx.doi.org/10.21037/atm-20-393). SGZ reports grants from Beijing Municipal Science and Technology Commission, grants from the Ministry of Science and Technology of the People’s Republic of China, outside the submitted work. The other authors have no conflicts of interest to declare.
Ethical Statement: The authors are accountable for all aspects of the work in ensuring that questions related to the accuracy or integrity of any part of the work are appropriately investigated and resolved. Peking University School and Hospital of Stomatology Ethics Committee approved the ethics of this study (PKUSSIRB-201944061). All participants had informed consent signed.
Open Access Statement: This is an Open Access article distributed in accordance with the Creative Commons Attribution-NonCommercial-NoDerivs 4.0 International License (CC BY-NC-ND 4.0), which permits the non-commercial replication and distribution of the article with the strict proviso that no changes or edits are made and the original work is properly cited (including links to both the formal publication through the relevant DOI and the license). See: https://creativecommons.org/licenses/by-nc-nd/4.0/.
References
- Pfaffe T, Cooper-White J, Beyerlein P, et al. Diagnostic potential of saliva: current state and future applications. Clin Chem 2011;57:675-87. [Crossref] [PubMed]
- Spielmann N, Wong DT. Saliva: diagnostics and therapeutic perspectives. Oral Dis 2011;17:345-54. [Crossref] [PubMed]
- Zhang Y, Sun J, Lin CC, et al. The emerging landscape of salivary diagnostics. Periodontol 2000 2016;70:38-52. [Crossref] [PubMed]
- Dawes C, Wong DTW. Role of Saliva and Salivary Diagnostics in the Advancement of Oral Health. J Dent Res 2019;98:133-41. [Crossref] [PubMed]
- Schipper RG, Silletti E, Vingerhoeds MH. Saliva as research material: biochemical, physicochemical and practical aspects. Arch Oral Biol 2007;52:1114-35. [Crossref] [PubMed]
- Kaczor-Urbanowicz KE, Wei F, Rao SL, et al. Clinical validity of saliva and novel technology for cancer detection. Biochim Biophys Acta Rev Cancer 2019;1872:49-59. [Crossref] [PubMed]
- Samaranayake L. Saliva as a diagnostic fluid. Int Dent J 2007;57:295-9. [Crossref] [PubMed]
- Chiappin S, Antonelli G, Gatti R, et al. Saliva specimen: a new laboratory tool for diagnostic and basic investigation. Clin Chim Acta 2007;383:30-40. [Crossref] [PubMed]
- Lima DP, Diniz DG, Moimaz SA, et al. Saliva: reflection of the body. Int J Infect Dis 2010;14:e184-8. [Crossref] [PubMed]
- Amado F, Lobo MJ, Domingues P, et al. Salivary peptidomics. Expert Rev Proteomics 2010;7:709-21. [Crossref] [PubMed]
- Gao L, Xu T, Huang G, et al. Oral microbiomes: more and more importance in oral cavity and whole body. Protein Cell 2018;9:488-500. [Crossref] [PubMed]
- Geho DH, Liotta LA, Petricoin EF, et al. The amplified peptidome: the new treasure chest of candidate biomarkers. Curr Opin Chem Biol 2006;10:50-5. [Crossref] [PubMed]
- Villanueva J, Shaffer DR, Philip J, et al. Differential exoprotease activities confer tumor-specific serum peptidome patterns. J Clin Invest 2006;116:271-84. [Crossref] [PubMed]
- Boonen K, Landuyt B, Baggerman G, et al. Peptidomics: the integrated approach of MS, hyphenated techniques and bioinformatics for neuropeptide analysis. J Sep Sci 2008;31:427-45. [Crossref] [PubMed]
- Baumann S, Ceglarek U, Fiedler GM, et al. Standardized approach to proteome profiling of human serum based on magnetic bead separation and matrix-assisted laser desorption/ionization time-of-flight mass spectrometry. Clin Chem 2005;51:973-80. [Crossref] [PubMed]
- Aas JA, Paster BJ, Stokes LN, et al. Defining the normal bacterial flora of the oral cavity. J Clin Microbiol 2005;43:5721-32. [Crossref] [PubMed]
- Krishnan K, Chen T, Paster BJ. A practical guide to the oral microbiome and its relation to health and disease. Oral Dis 2017;23:276-86. [Crossref] [PubMed]
- Escapa IF, Chen T, Huang Y, et al. New Insights into Human Nostril Microbiome from the Expanded Human Oral Microbiome Database (eHOMD): a Resource for the Microbiome of the Human Aerodigestive Tract. mSystems 2018. [Crossref] [PubMed]
- Zhang CZ, Cheng XQ, Li JY, et al. Saliva in the diagnosis of diseases. Int J Oral Sci 2016;8:133-7. [Crossref] [PubMed]
- Dawes C. Salivary flow patterns and the health of hard and soft oral tissues. J Am Dent Assoc 2008;139 Suppl:18S-24S. [Crossref] [PubMed]
- Dodds MW, Johnson DA, Yeh CK. Health benefits of saliva: a review. J Dent 2005;33:223-33. [Crossref] [PubMed]
- Navazesh M. Methods for collecting saliva. Ann N Y Acad Sci 1993;694:72-7. [Crossref] [PubMed]
- Turner MD, Ship JA. Dry mouth and its effects on the oral health of elderly people. J Am Dent Assoc 2007;138 Suppl:15S-20S. [Crossref] [PubMed]
- Kassan SS, Moutsopoulos HM. Clinical manifestations and early diagnosis of Sjogren syndrome. Arch Intern Med 2004;164:1275-84. [Crossref] [PubMed]
- Humphrey SP, Williamson RT. A review of saliva: normal composition, flow, and function. J Prosthet Dent 2001;85:162-9. [Crossref] [PubMed]
- Neyraud E, Sayd T, Morzel M, et al. Proteomic analysis of human whole and parotid salivas following stimulation by different tastes. J Proteome Res 2006;5:2474-80. [Crossref] [PubMed]
- Bhattarai KR, Kim HR, Chae HJ. Compliance with Saliva Collection Protocol in Healthy Volunteers: Strategies for Managing Risk and Errors. Int J Med Sci 2018;15:823-31. [Crossref] [PubMed]
- Peres JC, Rouquette JL, Miocevic O, et al. New techniques for augmenting saliva collection: bacon rules and lozenge drools. Clin Ther 2015;37:515-22. [Crossref] [PubMed]
- Hansen AM, Garde AH, Persson R. Sources of biological and methodological variation in salivary cortisol and their impact on measurement among healthy adults: a review. Scand J Clin Lab Invest 2008;68:448-58. [Crossref] [PubMed]
- Xiao H, Wong DT. Method development for proteome stabilization in human saliva. Anal Chim Acta 2012;722:63-9. [Crossref] [PubMed]
- Ao S, Sun X, Shi X, et al. Longitudinal investigation of salivary proteomic profiles in the development of early childhood caries. J Dent 2017;61:21-7. [Crossref] [PubMed]
- Sun X, Huang X, Tan X, et al. Salivary peptidome profiling for diagnosis of severe early childhood caries. J Transl Med 2016;14:240. [Crossref] [PubMed]
- Tong P, Yuan C, Sun X, et al. Identification of salivary peptidomic biomarkers in chronic kidney disease patients undergoing haemodialysis. Clin Chim Acta 2019;489:154-61. [Crossref] [PubMed]
- Tang H, Yuan C, Ma Z, et al. The potentiality of salivary peptide biomarkers for screening patients with periodontal diseases by mass spectrometry. Clin Chim Acta 2019;495:278-86. [Crossref] [PubMed]
- Caporaso JG, Kuczynski J, Stombaugh J, et al. QIIME allows analysis of high-throughput community sequencing data. Nat Methods 2010;7:335-6. [Crossref] [PubMed]
- Gill SR, Pop M, Deboy RT, et al. Metagenomic analysis of the human distal gut microbiome. Science 2006;312:1355-9. [Crossref] [PubMed]
- Chen H, Jiang W. Application of high-throughput sequencing in understanding human oral microbiome related with health and disease. Front Microbiol 2014;5:508. [Crossref] [PubMed]
- Magoč T, Salzberg SL. FLASH: fast length adjustment of short reads to improve genome assemblies. Bioinformatics 2011;27:2957-63. [Crossref] [PubMed]
- Edgar RC. Search and clustering orders of magnitude faster than BLAST. Bioinformatics 2010;26:2460-1. [Crossref] [PubMed]
- Chen T, Yu WH, Izard J, et al. The Human Oral Microbiome Database: a web accessible resource for investigating oral microbe taxonomic and genomic information. Database (Oxford) 2010;2010:baq013. [Crossref] [PubMed]
- Asnicar F, Weingart G, Tickle TL, et al. Compact graphical representation of phylogenetic data and metadata with GraPhlAn. PeerJ 2015;3:e1029. [Crossref] [PubMed]
- Lozupone CA, Hamady M, Kelley ST, et al. Quantitative and qualitative beta diversity measures lead to different insights into factors that structure microbial communities. Appl Environ Microbiol 2007;73:1576-85. [Crossref] [PubMed]
- Lozupone C, Knight R. UniFrac: a new phylogenetic method for comparing microbial communities. Appl Environ Microbiol 2005;71:8228-35. [Crossref] [PubMed]
- Wang K, Zhou X, Li W, et al. Human salivary proteins and their peptidomimetics: Values of function, early diagnosis, and therapeutic potential in combating dental caries. Arch Oral Biol 2019;99:31-42. [Crossref] [PubMed]
- Ericson S, Sjoback I. Salivary factors in children with recurrent parotitis. Part 1: Salivary flow rate, buffering capacity and inorganic components. Swed Dent J 1996;20:121-32. [PubMed]
- Golatowski C, Salazar MG, Dhople VM, et al. Comparative evaluation of saliva collection methods for proteome analysis. Clin Chim Acta 2013;419:42-6. [Crossref] [PubMed]
- Wang L, Yin G, Guo Y, et al. Variations in Oral Microbiota Composition Are Associated With a Risk of Throat Cancer. Front Cell Infect Microbiol 2019;9:205. [Crossref] [PubMed]
- Piccolo M, De Angelis M, Lauriero G, et al. Salivary Microbiota Associated with Immunoglobulin A Nephropathy. Microb Ecol 2015;70:557-65. [Crossref] [PubMed]
- Yang J, Mu X, Wang Y, et al. Dysbiosis of the Salivary Microbiome Is Associated With Non-smoking Female Lung Cancer and Correlated With Immunocytochemistry Markers. Front Oncol 2018;8:520. [Crossref] [PubMed]
- Stinson LF, Keelan JA, Payne MS. Comparison of Meconium DNA Extraction Methods for Use in Microbiome Studies. Front Microbiol 2018;9:270. [Crossref] [PubMed]
- Gomar-Vercher S, Simon-Soro A, Montiel-Company JM, et al. Stimulated and unstimulated saliva samples have significantly different bacterial profiles. PLoS One 2018;13:e0198021. [Crossref] [PubMed]
- Belstrøm D, Holmstrup P, Bardow A, et al. Comparative analysis of bacterial profiles in unstimulated and stimulated saliva samples. J Oral Microbiol 2016;8:30112. [Crossref] [PubMed]
- Hajduk J, Matysiak J, Kokot ZJ. Challenges in biomarker discovery with MALDI-TOF MS. Clin Chim Acta 2016;458:84-98. [Crossref] [PubMed]
- Li Y, Zhong CQ, Xu X, et al. Group-DIA: analyzing multiple data-independent acquisition mass spectrometry data files. Nat Methods 2015;12:1105-6. [Crossref] [PubMed]
- Vidova V, Spacil Z. A review on mass spectrometry-based quantitative proteomics: Targeted and data independent acquisition. Anal Chim Acta 2017;964:7-23. [Crossref] [PubMed]
- Meyer JG, Schilling B. Clinical applications of quantitative proteomics using targeted and untargeted data-independent acquisition techniques. Expert Rev Proteomics 2017;14:419-29. [Crossref] [PubMed]
- Tourlousse DM, Yoshiike S, Ohashi A, et al. Synthetic spike-in standards for high-throughput 16S rRNA gene amplicon sequencing. Nucleic Acids Res 2017;45:e23. [PubMed]
- Bender JM, Li F, Adisetiyo H, et al. Quantification of variation and the impact of biomass in targeted 16S rRNA gene sequencing studies. Microbiome 2018;6:155. [Crossref] [PubMed]