Assessing the genetic relationships between osteoarthritis and human plasma proteins: a large scale genetic correlation scan
Introduction
Osteoarthritis (OA) is a degenerative and chronic joint disease, mainly characterized by the breakdown of articular cartilage and underlying bone. OA was estimated to affect more than 20.7 million people in the United States, and to make the leading cause of disability across the whole world (1). The knee, hip, and hand are the areas most affected by OA, ultimately leading to joint failure with pain, stiffness and disability (2). The health economic burden of OA is increasing due to the current rapid ageing and increasing obesity evident in recent years, especially in the Asian region (3). Accumulated studies showed that genetic factors contributed greatly to the pathogenesis and disease progression of OA (4,5). Classic twin studies indicated primary OA had a strong hereditary component (5), with heritability estimating 30–46% according to the different sites (6). However, the genetic mechanism of OA is complicated and remain largely unknown now.
Human plasma proteins have been demonstrated to be involved in many biological processes, including signaling, transport, repair, and defense against infection (7). They play a major role as signals or biological markers in disease diagnosis as well as therapeutic monitoring (8). Recently, the impact of plasma proteins on the development of OA has also been reported. Researchers found multiple plasma proteins, which could activate macrophages via the innate immune receptor TLR-4, contributing to the low-level grade inflammation in OA patients (9,10). Another study found that human C-reactive protein-transgenic mice developed more severe OA compared with wild-type controls under the same high-fat diet (HFD) regime, implicating the role of CRP as a trigger to aggravate HFD-induced OA (11). Screening of the plasma proteome is considered as a promising approach for the identification of metabolic and disease processes. However, limited efforts were paid to explore the functional relevance of plasma proteome with OA. Recently, Sun et al. conducted a large scale GWAS of human plasma proteome in 3,301 healthy participants, and identified 1,927 genotype-protein associations (pQTLs), providing new clues for understanding of the genetic regulation of plasma proteins (7).
Genetic correlation serves as a critical population parameter in describing the genetic correlation of complex traits and diseases. Linkage disequilibrium score regression (LD score regression) is one of the most popular approach for genetic correlation scan. A far more important use of LD Score regression is the estimation and correction of confounding, for which is robust to the confounding biases caused by cryptic relatedness and population stratification (12). If the genetic correlation is statistically and quantitatively significant, then total phenotypic correlations are not entirely attributable to confounders of complete environmental nature (13). The more genetic variation an index variant tags, the higher the probability that this index variant will tag a causal variant (14). The great power of LD score regression has been demonstrated in previous studies. For example, Treur et al. identified the genetic correlation between smoking behaviors and schizophrenia via comparing the genome-wide association study (GWAS) meta-analysis results from five smoking phenotypes and the GWAS results of schizophrenia using LD score regression (15).
In this study, utilizing the large scale GWAS datasets of OA and plasma proteome, for the first time, we conducted a genetic correlation scan of OA and plasma proteome to explore the functional relevance of plasma proteins with OA. Our study holds the potential to provide new insights into the genetic mechanism of OA. We present the following article in accordance with the STREGA reporting checklist (available at http://dx.doi.org/10.21037/atm-19-4643).
Methods
The GWAS summary data of hip OA and knee OA
The GWAS summary data sets of hip OA and knee OA were obtained from the published studies (16). Briefly, 2,396 hospital-diagnosed hip OA and 9,593 controls, 4,462 hospital-diagnosed knee OA and 17,885 controls were included in the GWAS, separately. The genotyping of samples was conducted either using the UK BiLEVE array or the UK Biobank Axiom array. Imputation was performed using UK10K/1000 Genomes Project haplotype reference panel by the IMPUTE3 program. After quality control, there are 16,122,076 SNPs for hip OA and 16,309,199 SNPs for knee OA were used in following analysis, respectively. Detailed description of sample characteristics, experimental design, and statistical analysis can be shown in the published study (16).
The GWAS summary data of hand OA
The GWAS summary of hand OA was derived from the UK Biobank (UK Biobank fields: 20002). Briefly, a total of 37,782 hand OA cases and 414,482 controls in UK Biobank participants of European ancestry were enrolled in our study. The UK Biobank participants were genotyped using the Affymetrix UK BiLEVE Axiom or UK Biobank Axiom array and augmented by imputation of ~90 million genetic variants from the Haplotype Reference Consortium, 1,000 Genomes and UK 10K projects (17). After filtering, this dataset Contains 9,113,133 imputed variants. Detailed information about samples, imputation, genotyping can be found in the published study (18).
The GWAS data of human plasma proteome
The GWAS summary data of human plasma proteome was obtained from the published study (7). Briefly, a total of 3,622 plasma proteins were quantified in 3,301 healthy participants from 25 centers across England (19). Genotyping was performed using Affymetrix Axiom UK Biobank genotyping array (Santa Clara, California, USA). Totally, 654,966 high-quality variants were used for imputation. Using a combined 1,000 Genomes Phase 3-UK10K reference panel, imputation was performed via the Sanger Imputation Server (https://imputation.sanger.ac.uk) and resulted in 87,696,888 imputed variants.
Genetic correlation analysis
Following the standard approach recommended by the developers, LD score regression software (https://github.com/bulik/ldsc) was used here to evaluate the genetic correlation between OA and each of the human plasma proteins. LD score regression is capable of estimating genetic correlation by using GWAS summary statistics rather than individual level genotype data (14,20,21). The idea of LD score regression is that, for a polygenic trait, LD to an annotation that is enriched for heritability will increase the χ2 statistic of a SNP more than LD to an annotation that is not enriched for heritability. And SNPs with lower LD have significantly larger per-SNP heritability. Consequently, the LD score regression is capable of distinguishing genuine polygenicity from the confounding bias (such as cryptic relatedness and population stratification) and more powerful than λGC, especially at large sample sizes (14). After correcting for multiple testing, the significant threshold of this study should be P<1.38×10-5.
Results
For GWAS analysis of OA, the significant SNPs associated with hand OA, hip OA and knee OA were summarized in (http://cdn.amegroups.cn/static/application/8d28e643d1bc2432d8a90b76a79aa85b/atm-19-4643-1.pdf, http://cdn.amegroups.cn/static/application/0822f6b41bee76029ee949f7606d60c5/atm-19-4643-2.pdf, http://cdn.amegroups.cn/static/application/978f9e622fab5e1e48a905c326c36893/atm-19-4643-3.pdf) (P value <5×10-8).
Genetic correlation analysis between knee OA and plasma proteome
For knee OA, 12 suggestive plasma proteins were detected (Figure 1A, Table 1), such as Elafin (coefficient =−0.5562, P value =0.0092), Interleukin-16 (coefficient =0.3949, P value =0.0435), leukotriene A-4 hydrolase (coefficient =0.8366, P value =0.0114), muscle and skeletal receptor tyrosine-protein kinase (coefficient =0.434, P value =0.0219), fructose-1,6-bisphosphatase 1 (coefficient =0.8139, P value =0.0344).
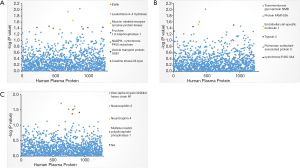
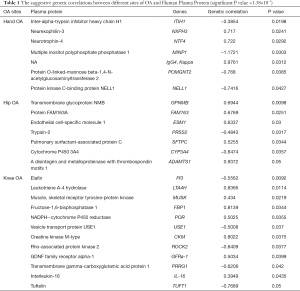
Full table
Genetic correlation analysis between hip OA and plasma proteome
For hip OA, we also detected 7 suggestive plasma proteins (Figure 1B, Table 1), such as transmembrane glycoprotein NMB (coefficient =0.6944, P value =0.0098), endothelial cell-specific molecule 1 (coefficient =0.6337, P value =0.03) and Cytochrome P450 3A4 (coefficient =−0.8474, P value =0.0357).
Genetic correlation analysis between hand OA and plasma proteome
For hand OA, 7 suggestive plasma proteins were detected (Figure 1C, Table 1), such as inter-alpha-trypsin inhibitor heavy chain H1 (coefficient =−0.3854, P value =0.0198), multiple inositol polyphosphate phosphatase 1 (coefficient =−1.1721, P value =0.0303), protein kinase C-binding protein NELL1 (coefficient =−0.7416, P value =0.0427), neurexophilin-3 (coefficient =0.717, P value =0.0241), neurotrophin-4 (coefficient =0.722, P value =0.0292).
Discussion
Recent studies observed the impact of plasma proteins on the development of OA. However, there was no systematic study online investigating the genetic correlation among plasma proteins and different sites of OA. In this study, utilizing the large scale GWAS datasets of OA and plasma proteome, LD score regression scan was conducted to investigate the functional relevance between OA and plasma proteome. We identified several candidate plasma proteins for hip OA, knee OA and hand OA, respectively. Our study results hold great potential in promoting the understanding of the genetic pathophysiology of OA and paving the way towards the development of effective therapies against OA given the different genetic mechanisms of different sites.
Inter-alpha-trypsin inhibitor heavy chain H1 showed the strongest correlation evidence with hand OA in this study. Inter-alpha-trypsin inhibitor heavy chain H1 (ITIH1), is encoded by ITIH1 gene, acting as a carrier of hyaluronan in serum or as a binding protein between hyaluronan and other matrix protein. ITIH1 has been defined as an important protein biomarker for OA in the previous studies (22). An existing study revealed the protein level of ITIH1 in serum was significantly elevated in OA patients compared with the control subjects (23). A large scale GWAS of OA identified ITIH1 as a susceptibility gene for OA (24), which was consistent with our finding.
Protein kinase C-binding protein, also known as NEL-like protein 1 (NELL1) or Nel-related protein 1, was identified for hand OA in our study. The important role of NELL-1 in driving differentiation and growth of bone and cartilage tissue has been well documented in recent years (25). A recent study showed that adding NELL1 to the human perivascular stem cells (hPSCs) could increase the gene and protein expression of chondrogenic markers, suggesting the role of NELL1 in enhancing and accelerating cartilage repair (26). Another study suggested that NELL1 was a novel osteogenic factor that transduced the osteogenic signals through the activation of certain Tyr-kinases, leading to the osteogenic differentiation (27). Desai, Shannon et al. observed that NELL1-deficient mice showed reduced expression of extracellular matrix proteins (ECM), cell adhesion and communication proteins, causing cranial and vertebral defects (28). Another animal study suggested the significant improvement of NELL1 in treating diverse pathologies of cartilage defects and degeneration (29).
Transmembrane glycoprotein NMB (GPNMB), a protein involved in the bone formation, showed the strongest correlation evidence with Hip OA. GPNMB was found to expressed in various cell types, including melanocytes, osteoclasts, osteoblasts, dendritic cells. A previous study reported that the expressional level of GPNMB is significantly higher in OA cartilage compared with normal donor cartilage (4). Moreover, Jiang et al. found the expressional level of GPNMB was associated with bone loss and the pathology of OA (30). GPNMB is expressed in normal articular chondrocytes, and expression level is higher in damaged cartilage, suggesting the anti-inflammatory role of GPNMB in OA. Furthermore, data also suggested that GPNMB inhibits pERK and pNFkB-65 proteins through the CD44 receptor (31). GPNMB was also known as osteoactivin due to the role in osteoblast differentiation and bone remodeling. Previous literatures have shown that osteoactivin (OA/GPNMB) inhibited RANKL-induced osteolysis in vivo and this process was regulated through CD44 and ERK activation (32). The same conclusion about the role of osteoactivin as a positive regulator of osteoblastogenesis was also drawn by another finding (33).
Another notable protein identified in hip OA was A disintegrin and metalloproteinase with thrombospondin motifs 1 (ADAMTS1). ADAMTS1, a member of the ADAMTS family of proteases, is a multifunctional metalloproteinase necessary for the development and function of a variety of organs and pathological processes. ADAMTS families, including ADAMTS4, ADAMTS5, ADAM9, ADAM10, ADAM12 and so on, are found to be expressed in cartilage and several of them showed significantly altered expression in OA (34). Wachsmuth et al. confirmed the expression and presence of ADAMTS1 in normal and OA human cartilage, and found it was the first matrix-degrading enzyme downregulated by the catabolic factor IL-1beta (IL-β) in vitro (35). Interesting, different conclusions were also drawn by some other studies. Data from previous study suggested there was no increasement expression of ADAMTS1 after destabilization of the medial meniscus (DMM) in mice (36). A comparative analysis found that ADAMTS1 was downregulated in both cartilage and synovial tissues of OA compared with the fracture to the neck of femur samples (37). Further biological researches on the specific role of ADAMTS1 on OA are needed.
As is known to all, OA is a highly heterogeneous disease, which can also be seen from the clinical presentation. Several stratification methods of OA have been reported, including mechanism of onset, stage of disease progression, involved joints, inflammatory levels, and effusion (38). Specifically, OA is stratified by several subgroups considering the involved joints, such as generalized OA (39), bilateral OA, specific location OA (e.g., hand, hip, knee, and ankle) (16,40). Genetic evidence also confirms the heterogeneity of OA. Previous studies have identified many risk loci for OA (41). Nevertheless, most of these significant variants were not shared among different OA sites (42). Another study conducted in 992 monozygotic and dizygotic female twin participants showed that genetic influence on OA differs by anatomical site, indicating OA is a joint-specificity disease (43). Except from genetic loci, the causal effects of some phenotypes on OA are also site-specific, including lumbar spine bone mineral density and height (16).
Many reasons accounted for these observations. For example, studies showed there were higher serum concentrations of cartilage degradation markers [e.g., cartilage oligomeric matrix protein (COMP), hyaluronan (HA)] while lower serum concentrations of cartilage formation markers (e.g., a collagen II synthesis marker) in patients with hand OA compared with other joints OA (44,45). In addition, bone shape might be a risk factor for the occurrence or development of OA (46). Haverkamp et al. investigated the role of joint shape in knee OA by using a statistical shape model, it turned out that knees with OA were wider, more extended during radiography, and had an elevated lateral tibial plateau (47). This kind change of bone shape could serve as a biomarker to predict the development or progression of knee OA (47). Furthermore, there might be histological differences in articular cartilage between joints. Biochemical properties of chondrocytes differed from knee and ankle joints, the ankle chondrocytes synthesized more proteoglycans (PGs) and collagens than knee chondrocytes (48).
In our study, we performed a LD score regression analysis to explore the association between different sites of OA and plasma proteins via repurposing the previous GWAS data of OA and pQTL data of human plasma proteome. we found 12, 7, 7 suggestive proteins associated with knee OA, hip OA and hand OA, respectively. In line with previous studies, there was no common plasma protein shared among these three sites of OA. Our results provide novel evidence that OA is a heterogeneous disease. In addition, the association of plasma proteins and OA was discussed from the perspective of genetics, which was not easily affected by the environmental confounding factors. Moreover, the large sample sizes of the GWAS data ensured the accuracy of our study results, which were consistent with previous study results. Finally, given the great power of LD score regression in estimating genetic correlation, our finding holds great potential in providing novel clues for the genetic mechanism of OA.
One issue should be noted in our study. After correcting for multiple testing, the significant threshold should be P<1.38×10−5. Unfortunately, there was no significant genetic correlation under this threshold based on our results. Since all the plasma proteins identified in the present study were suggestive, our study results should be interpreted with caution. Additionally, given the complexity of OA, we cannot sure the specific role of these identified plasma proteins in disease progression, which needs more mechanism-based experiments to further confirm the biological rationality and to clarify the biological mechanism of the identified plasma proteins involving in the development of OA. Also, more analysis with larger, and more homogeneous populations may help untangle the genetic architecture of OA. In our future study, we will conduct further studies to explore the functional roles of these proteins in the development of OA via biological functional analysis such as cells as well as animal experiments. Then we can confirm whether these proteins play a direct or indirect role in the development of OA.
Taken together, we investigated the genetic correlations between plasma proteins and different sites of OA in a systematic way by using the large summary GWAS datasets, respectively. And we found several suggestive plasma proteins on the different sites of OA in our study. Our results provide a novel clue for validating the heterogeneity of OA.
Acknowledgments
Funding: This study is supported by the National Natural Scientific Foundation of China (81472925, 81673112), the Key projects of international cooperation among governments in scientific and technological innovation (2016YFE0119100), the Natural Science Basic Research Plan in Shaanxi Province of China (2017JZ024), and the Fundamental Research Funds for the Central Universities.
Footnote
Reporting Checklist: The authors have completed the STREGA reporting checklist. Available at http://dx.doi.org/10.21037/atm-19-4643
Conflicts of Interest: All authors have completed the ICMJE uniform disclosure form (available at http://dx.doi.org/10.21037/atm-19-4643). The authors have no conflicts of interest to declare.
Ethical Statement: The authors are accountable for all aspects of the work in ensuring that questions related to the accuracy or integrity of any part of the work are appropriately investigated and resolved.
Open Access Statement: This is an Open Access article distributed in accordance with the Creative Commons Attribution-NonCommercial-NoDerivs 4.0 International License (CC BY-NC-ND 4.0), which permits the non-commercial replication and distribution of the article with the strict proviso that no changes or edits are made and the original work is properly cited (including links to both the formal publication through the relevant DOI and the license). See: https://creativecommons.org/licenses/by-nc-nd/4.0/.
References
- Ollivier JE. Advances in the management of osteoarthritis and rheumatoid arthritis. JAAPA 2001;14:22-5. [PubMed]
- Glyn-Jones S, Palmer AJR, Agricola R, et al. Osteoarthritis. Lancet 2015;386:376-87. [PubMed]
- Fransen M, Bridgett L, March L, et al. The epidemiology of osteoarthritis in Asia. Int J Rheum Dis 2011;14:113-21. [Crossref] [PubMed]
- Karlsson C, Dehne T, Lindahl A, et al. Genome-wide expression profiling reveals new candidate genes associated with osteoarthritis. Osteoarthritis Cartilage 2010;18:581-92. [Crossref] [PubMed]
- Valdes AM, Spector TD. The Contribution of Genes to Osteoarthritis. Med Clin North Am 2009;93:45-66. x. [Crossref] [PubMed]
- Kirk KM, Bellamy N, O'Gorman LE, et al. The validity and heritability of self-report osteoarthritis in an Australian older twin sample. Twin Res 2002;5:98-106. [Crossref] [PubMed]
- Sun BB, Maranville JC, Peters JE, et al. Genomic atlas of the human plasma proteome. Nature 2018;558:73-9. [Crossref] [PubMed]
- Johann DJ Jr, McGuigan MD, Patel AR, et al. Clinical proteomics and biomarker discovery. Ann N Y Acad Sci 2004;1022:295-305. [Crossref] [PubMed]
- Goldring SR, Scanzello CR. Plasma proteins take their toll on the joint in osteoarthritis. Arthritis Res Ther 2012;14:111. [Crossref] [PubMed]
- Sohn DH, Sokolove J, Sharpe O, et al. Plasma proteins present in osteoarthritic synovial fluid can stimulate cytokine production via Toll-like receptor 4. Arthritis Res Ther 2012;14:R7. [Crossref] [PubMed]
- Kozijn AE, Tartjiono MT, Ravipati S, et al. Human C-reactive protein aggravates osteoarthritis development in mice on a high-fat diet. Osteoarthritis Cartilage 2019;27:118-28. [Crossref] [PubMed]
- Bulik-Sullivan BK, Loh PR, Finucane HK, et al. LD Score regression distinguishes confounding from polygenicity in genome-wide association studies. Nat Genet 2015;47:291-5. [Crossref] [PubMed]
- Lee JJ, McGue M, Iacono WG, et al. The accuracy of LD Score regression as an estimator of confounding and genetic correlations in genome-wide association studies. Genet Epidemiol 2018;42:783-95. [Crossref] [PubMed]
- Bulik-Sullivan BK, Loh PR, Finucane HK, et al. LD Score regression distinguishes confounding from polygenicity in genome-wide association studies. Nat Genet 2015;47:291-5. [Crossref] [PubMed]
- Treur JL, Taylor AE, Ware JJ, et al. Smoking and caffeine consumption: a genetic analysis of their association. Addict Biol 2017;22:1090-102. [Crossref] [PubMed]
- Zengini E, Hatzikotoulas K, Tachmazidou I, et al. Genome-wide analyses using UK Biobank data provide insights into the genetic architecture of osteoarthritis. Nat Genet 2018;50:549-58. [Crossref] [PubMed]
- Abecasis GR, Auton A, Brooks LD, et al. An integrated map of genetic variation from 1,092 human genomes. Nature 2012;491:56-65. [Crossref] [PubMed]
- Canela-Xandri O, Rawlik K, Tenesa A. An atlas of genetic associations in UK Biobank. Nat Genet 2018;50:1593-9. [Crossref] [PubMed]
- Di AE, Thompson SG, Kaptoge S, et al. Efficiency and safety of varying the frequency of whole blood donation (INTERVAL): a randomised trial of 45 000 donors. Lancet 2017;390:2360-71. [Crossref] [PubMed]
- Lee JJ, McGue M, Iacono WG, et al. The accuracy of LD Score regression as an estimator of confounding and genetic correlations in genome-wide association studies. Genetic Epidemiology 2018. [Crossref] [PubMed]
- Ni G, Moser G, Wray NR, et al. Estimation of genetic correlation using linkage disequilibrium score regression and genomic restricted maximum likelihood. bioRxiv 2018:194019.
- Reynard LN, Loughlin J. Insights from human genetic studies into the pathways involved in osteoarthritis. Nat Rev Rheumatol 2013;9:573-83. [Crossref] [PubMed]
- Lourido L, Ayoglu B, Fernández-Tajes J, et al. Discovery of circulating proteins associated to knee radiographic osteoarthritis. Sci Rep 2017;7:137. [Crossref] [PubMed]
- Consortium A, Collaborators A, Zeggini E, et al. Identification of new susceptibility loci for osteoarthritis (arcOGEN): a genome-wide association study. Lancet 2012;380:815-23. [Crossref] [PubMed]
- Shen MJ, Wang GG, Wang YZ, et al. Nell-1 Enhances Osteogenic Differentiation of Pre-Osteoblasts on Titanium Surfaces via the MAPK-ERK Signaling Pathway. Cell Physiol Biochem 2018;50:1522-34. [Crossref] [PubMed]
- Li CS, Zhang X, Peault B, et al. Accelerated Chondrogenic Differentiation of Human Perivascular Stem Cells with NELL-1. Tissue Eng Part A 2016;22:272-85. [Crossref] [PubMed]
- Bokui N, Otani T, Igarashi K, et al. Involvement of MAPK signaling molecules and Runx2 in the NELL1-induced osteoblastic differentiation. FEBS Lett 2008;582:365-71. [Crossref] [PubMed]
- Desai J, Shannon ME, Johnson MD, et al. Nell1-deficient mice have reduced expression of extracellular matrix proteins causing cranial and vertebral defects. Hum Mol Genet 2006;15:1329-41. [Crossref] [PubMed]
- Siu RK, Zara JN, Hou Y, et al. NELL-1 promotes cartilage regeneration in an in vivo rabbit model. Tissue Eng Part A 2012;18:252-61. [Crossref] [PubMed]
- Jiang SS, Chen CH, Tseng KY, et al. Gene expression profiling suggests a pathological role of human bone marrow-derived mesenchymal stem cells in aging-related skeletal diseases. Aging 2011;3:672-84. [Crossref] [PubMed]
- Al-Adlaan, Asaad "A NOVEL ANTI-INFLAMMATORY ROLE OF OSTEOACTIVIN/GPNMB IN POST-TRAUMATIC OSTEOARTHRITIS." Electronic Thesis or Dissertation. Kent State University, 2017. Available online: https://etd.ohiolink.edu/
- Sondag GR, Mbimba TS, Moussa FM, et al. Osteoactivin inhibition of osteoclastogenesis is mediated through CD44-ERK signaling. Exp Mol Med 2016;48:e257. [Crossref] [PubMed]
- Abdelmagid SM, Barbe MF, Rico MC, et al. Osteoactivin, an anabolic factor that regulates osteoblast differentiation and function. Exp Cell Res 2008;314:2334-51. [Crossref] [PubMed]
- Yang CY, Chanalaris A, Troeberg L. ADAMTS and ADAM metalloproteinases in osteoarthritis - looking beyond the 'usual suspects'. Osteoarthritis Cartilage 2017;25:1000-9. [Crossref] [PubMed]
- Wachsmuth L, Bau B, Fan Z, et al. ADAMTS-1, a gene product of articular chondrocytes in vivo and in vitro, is downregulated by interleukin 1beta. J Rheumatol 2004;31:315-20. [PubMed]
- Gardiner MD, Vincent TL, Driscoll C, et al. Transcriptional analysis of micro-dissected articular cartilage in post-traumatic murine osteoarthritis. Osteoarthritis Cartilage 2015;23:616-28. [Crossref] [PubMed]
- Davidson RK, Waters JG, Kevorkian L, et al. Expression profiling of metalloproteinases and their inhibitors in synovium and cartilage. Arthritis Res Ther 2006;8:R124. [Crossref] [PubMed]
- Driban JB, Sitler MR, Barbe MF, et al. Is osteoarthritis a heterogeneous disease that can be stratified into subsets? Clin Rheumatol 2010;29:123-31. [Crossref] [PubMed]
- Kraus VB, Jordan JM, Doherty M, et al. The Genetics of Generalized Osteoarthritis (GOGO) study: study design and evaluation of osteoarthritis phenotypes. Osteoarthritis Cartilage 2007;15:120-7. [Crossref] [PubMed]
- Kloppenburg M, Kwok WY. Hand osteoarthritis—a heterogeneous disorder. Nat Rev Rheumatol 2011;8:22-31. [Crossref] [PubMed]
- Styrkarsdottir U, Helgason H, Sigurdsson A, et al. Whole-genome sequencing identifies rare genotypes in COMP and CHADL associated with high risk of hip osteoarthritis. Nat Genet 2017;49:801-5. [Crossref] [PubMed]
- Styrkarsdottir U, Thorleifsson G, Helgadottir HT, et al. Severe osteoarthritis of the hand associates with common variants within the ALDH1A2 gene and with rare variants at 1p31. Nat Genet 2014;46:498-502. [Crossref] [PubMed]
- MacGregor AJ, Li Q, Spector TD, et al. The genetic influence on radiographic osteoarthritis is site specific at the hand, hip and knee. Rheumatology (Oxford) 2009;48:277-80. [Crossref] [PubMed]
- Chiu C, Moss CF. The role of the external ear in vertical sound localization in the free flying bat, Eptesicus fuscus. J Acoust Soc Am 2007;121:2227-35. [Crossref] [PubMed]
- Aslam I, Perjar I, Shi XA, et al. Associations between biomarkers of joint metabolism, hand osteoarthritis, and hand pain and function: the Johnston County Osteoarthritis Project. J Rheumatol 2014;41:938-44. [Crossref] [PubMed]
- Baker-LePain JC, Lane NE. Role of bone architecture and anatomy in osteoarthritis. Bone 2012;51:197-203. [Crossref] [PubMed]
- Haverkamp DJ, Schiphof D, Bierma-Zeinstra SM, et al. Variation in joint shape of osteoarthritic knees. Arthritis Rheum 2011;63:3401-7. [Crossref] [PubMed]
- Huch K. Knee and ankle: human joints with different susceptibility to osteoarthritis reveal different cartilage cellularity and matrix synthesis in vitro Arch Orthop Trauma Surg 2001;121:301-6. [published correction appears in Arch Orthop Trauma Surg 2002 Apr;122(3):191]. [Crossref] [PubMed]