RAB5C, SYNJ1, and RNF19B promote male ankylosing spondylitis by regulating immune cell infiltration
Introduction
Ankylosing spondylitis (AS) is a common rheumatic disorder characterized by inflammation and new bone formation (1). AS often affects the sacroiliac joints and spine, but it can also affect the surrounding joints, especially the hip joints. Extra-articular lesions mainly manifest as acute uveitis, psoriasis, aortic root, and intestinal inflammation (2). AS can result in limited motion and gradual joint stiffness, so the disability rate is extremely high (3), and the patient’s quality of life and workability can be severely impacted. The onset of AS usually occurs around the age of 30 years. Males are more likely to be affected than females, with the male-to-female ratio being about 2–3/1 (4). Although great progress has been made in AS treatment in recent years, the therapeutic effect is still not completely satisfactory.
In recent years, an increasing number of studies have shown that immune cell infiltration plays a critical role in the occurrence, development, and treatment of AS. For instance, dendritic cells (DCs), macrophages, natural killer (NK) cells, T helper 1 (Th1) cells, and T helper 17 (Th17) cells all have important involvement in the development of AS (5). Hallett et al. observed a large number of infiltrating DCs, CD4+ T cells, monocytes, and NK cells in the spinal region in a mouse model of AS; similarly, they reported that DCs and CD4+ T cells were infiltrated in the synovial tissue of human patients with AS (6). Liu et al. found that myeloid-derived suppressor cells (MDSCs), which are involved in the regulation of immunity, are significantly increased in patients with AS, and this process is regulated by the signal transducer and activator of transcription 3 (STAT3)/arginase-I signaling pathway (7). Tumor necrosis factor-alpha (TNF-α) inhibitors can improve the immune imbalance of CD4+ T cells and negative regulatory cells, thereby inhibiting AS activity and achieving the goal of treating the disease (8). Therefore, evaluation of immune cell infiltration and determination of the differences in infiltrating immune cell components are of great value for elucidating the molecular mechanism of AS progression and developing new immunotherapeutic targets.
CIBERSORT is an analytical tool that uses microarray data or RNA-sequencing (RNA-Seq) data to assess the expression of immune cells in samples and to obtain various immune cell ratios (9). As an analytical tool, CIBERSORT has been widely applied to analyze immune infiltration in cancer and non-cancerous diseases, including colorectal cancer (10), renal cell carcinoma (11), and osteoarthritis (12). However, to our knowledge, no study to date has used CIBERSORT to analyze the characteristics of sex-specific AS immune cell infiltration.
In this study, we innovatively used bioinformatics techniques to analyze the role of immune infiltration in sex-specific AS and screen out possible key genes. We first downloaded the AS microarray dataset from the Gene Expression Omnibus (GEO) database and then extracted the data of male patients with AS (M_AS) and male healthy controls (M_HC). Differentially expressed genes (DEGs) were identified, and the key genes were screened out through protein-protein interaction (PPI) analysis and weighted correlation network analysis (WGCNA). Then, using CIBERSORT, we analyzed the differences in immune cell infiltration between the M_AS group and the M_HC group. To better understand the immune mechanism and related genes in AS occurrence and development, the differences in immune cell infiltration in groups separated by the expression of key genes were analyzed using the same method. We present the following article in accordance with the MDAR reporting checklist (available at https://dx.doi.org/10.21037/atm-21-2721).
Methods
Data download
The GEO database (https://www.ncbi.nlm.nih.gov/geo/) (13) was searched using “spondylitis, ankylosing” [Medical Subject Headings Terms] OR “Ankylosing Spondylitis” [All Fields], and a total of 167 entries were obtained. Then, we used “Homo sapiens” [porgn] AND “Expression profiling by array” [Filter] as the filtering conditions and obtained 8 datasets. After checking the details of each dataset, we finally selected GSE73754 as the research object. The dataset was downloaded for subsequent analyses using the “GEOquery” package of R software (version 3.6.3) (14). The dataset is based on the GPL10558 Illumina HumanHT-12 V4.0 expression beadchip, which contains 72 samples across four groups. The M_AS group and female AS patients (F_AS) group comprised 27 and 25 patients, respectively, while the M_HC and female healthy controls (F_HC) groups each contained 10 patients (15). The data of the M_AS and M_HC groups were extracted. There were 37 samples, and the microarray data of each sample were preprocessed; the missing values were completed, and when the gene corresponded to multiple probes, the maximum value was taken (Figure 1A). The study was conducted in accordance with the Declaration of Helsinki (as revised in 2013). The data included in this study were downloaded from a public database, so the requirements to obtain institutional review board approval and patients’ written informed consent did not apply. The workflow is shown in Figure 1B.
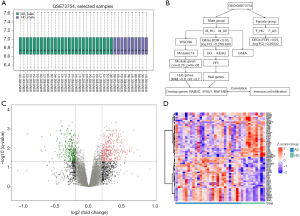
Differential expression analysis
The “limma” package (an R software package based on Bioconductor specifically for processing expression profile microarray data) was used to standardize and analyze differences between the M_AS and M_HC data (16). DEGs were screened out using the Benjamini-Hochberg adjusted false discovery rate (FDR) <0.05, |log fold change (FC)| >0.263 as the standard (17).
Functional annotation of DEGs
The “clusterProfiler” and “pathview” packages were used to perform Gene Ontology (GO) and Kyoto Encyclopedia of Genes and Genomes (KEGG) analyses on the selected DEGs (18), GO terms fall under three categories: biological process (BP), cellular component (CC), and molecular function (MF). The FDR <0.05 was considered to be significantly enriched.
PPI network construction
All of the DEGs were imported into the STRING online database (https://string-db.org/) (19) for PPI analysis, and Cytoscape software (3.7.3) was employed to visualize the PPI network. The Molecular COmplex DEtection (MCODE) plug-in in Cytoscape was used for further analysis of the interaction network (20). The standard settings were: Node score cut-off value =0.2, K-Core value (K-Core) =2, and degree value cut-off =2. The score value of MCODE was calculated, and MCODE score >5 was used as the screening criteria for significant clusters.
Gene set enrichment analysis (GSEA)
GSEA is an analytical method for genome-wide expression profile microarray data (21), The “clusterProfiler” package was used to perform GSEA, with “c2.cp.kegg.v7.0.symbols.gmt” selected as the reference gene set. An FDR <0.25 and P<0.05 were considered to indicate significant enrichment.
WGCNA and screening of hub genes
WGCNA is a systems biology method used to describe gene association patterns between different samples. It can be used for the identification of highly synergistic gene sets and alternative biomarker genes or therapeutic targets based on their connectivity and association between gene sets and phenotypes (22). We started by using the “hclust” function to perform a hierarchical clustering analysis. After that, we filtered the soft threshold in the module construction process by using “pickSoftThreshold”, selected the appropriate power value, and used the “WGCNA” package to construct a co-expression network. Each module was given a unique color, and the minimum value was set to 30; then, the module most closely associated with the phenotype was screened out. The “clusterProfiler” package was used to perform GO and KEGG analysis on the genes in the module. Module membership (MM) >0.8 and gene significance (GS) >0.7 were used as the criteria for screening hub genes (23). The hub genes obtained through WGCNA were intersected with the hub genes obtained from the PPI network, and the key genes were finally obtained.
Immune infiltration analysis
Gene expression profile data were uploaded to CIBERSORT (https://cibersort.stanford.edu/) to obtain the immune cell infiltration matrix (9). The “FactoMineR” and “factoextra” packages were employed to perform principal component analysis (PCA) on the immune cell infiltration matrix. The software package “ggplot2” was used to draw a two-dimensional PCA cluster diagram. A heat map of 22 types of infiltrating immune cells was drawn using the “corrplot” software package. Violin diagrams were made to visualize differences in immune cell infiltration between the M_HC and M_AS groups using the “ggplot2” package. Finally, according to the median expression levels of the key genes, the samples were divided into two groups: the high expression group and the low expression group. The differences in immune cell infiltration between the two groups were analyzed, and the results were visualized using the “ggplot2” package.
Statistical analysis
Statistical analyses were performed with R software (3.6.3). All data were expressed as means ± standard deviations (SDs). The DEGs between the M_AS and M_HC groups were determined using the limma package.
Results
DEGs between the M_HC and M_AS groups
The expression profile data of the M_HC group and M_AS group were compared and screened with FDR <0.05 and |log FC| >0.263 as the standard. A total of 689 DEGs were obtained, among which 395 genes were up-regulated and 294 were down-regulated (Figure 1C). The 30 most significantly up-regulated genes and the 30 most significantly down-regulated genes were selected, and a heat map was drawn (Figure 1D).
Functional annotation of DEGs
The GO analysis results showed that the DEGs were predominantly enriched in BPs. The significantly enriched BP terms for the DEGs included neutrophil degranulation, neutrophil activation involved in immune response, mast cell degranulation, and mast cell activation involved in immune response. The significantly enriched CC terms for the DEGs included ribonucleoprotein granule, ribosomal subunit, and immunological synapse. The significantly enriched MF terms for the DEGs included small GTPase binding, Ras GTPase binding, and MHC protein complex binding (Figure 2A,B,C). The KEGG results showed that the up-regulated and down-regulated genes were enriched in different pathways. The up-regulated genes were mainly enriched in leukocyte transendothelial migration, the TNF signaling pathway, and the chemokine signaling pathway, while the down-regulated genes were mainly enriched in ribosome, oxidative phosphorylation, and pathways of neurodegeneration-multiple diseases (Figure 2D,E).
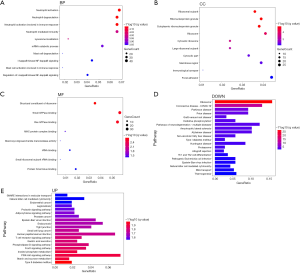
DEG PPI network
The STRING online database was used to construct the PPI network of the common DEGs, and the obtained results were imported into Cytoscape for visualization and cluster screening. The “Network Analyser” tool in Cytoscape was used to calculate the score of each node in the PPI network without direction, and the degree value of each node was obtained. The node size represents the degree value; red and blue represent up-regulated and down-regulated genes, respectively; and the width of the edge represents the combined score value of the edge. The nodes with degree ≥15 were screened out and visualized (Figure 3A). The MCODE plug-in was used to analyze all nodes. Cluster association analysis was carried out with the default parameters of node score cut-off value =0.2, K-Core value (K-Core) =2, and degree value cut-off =2. A total of 23 clusters were obtained, and the 5 clusters with the highest scores were visualized and further analyzed. The 5 sub-clusters were cluster 1 (40 nodes, 421 edges, score 21.59, Figure 3B), cluster 2 (20 nodes, 107 edges, score 11.26, Figure 3C), cluster 3 (12 nodes, 54 edges, score 9.82, Figure 3D), cluster 4 (8 nodes, 28 edges, score 7.43, Figure 3E), and cluster 5 (19 nodes, 50 edges, score 5.56, Figure 3F).
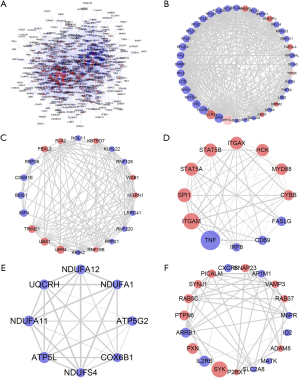
GSEA
We used “c2.cp.kegg.v7.0.symbols.gmt” as the reference gene set to perform GSEA on all microarray profile data. Compared with the M_HC group, enriched BPs in the M_AS group were mainly related to the regulation of immune response, innate immune response, and negative regulation of cell death. The enriched CC terms mainly included secretory granule membrane, tertiary granule, and integrin complex (Figure 4A,B). The enriched MF terms mainly included Toll-like receptor (TLR) binding, phosphatidylinositol-3,4,5-trisphosphate binding, and protein domain-specific binding. The enriched signaling pathways mainly included the nuclear factor-kappa B (NF-κB) signaling pathway, sphingolipid signaling pathway, FoxO signaling pathway, and Rap1 signaling pathway. The top pathways were visualized, the abscissa represents GeneRatio, and the ordinate represents different pathways; the significance of enrichment gradually increases from blue to red, and the size of the dot represents the number of genes contained in the corresponding pathway (Figure 4C). The enrichment plot shows the running enrichment scores of 4 representative pathways (Figure 4D). The results were observed to be similar to those of the GO and KEGG analysis of the DEGs.
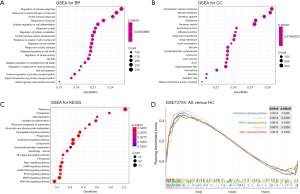
WGCNA
All the microarray data were used for WGCNA. A sample dendrogram showed that 2 samples deviated greatly; these samples were subsequently removed, and 35 samples were finally included for analysis (Figure 5A). Through the construction of the co-expression matrix, 14 gene modules were obtained (Figure 5B). Correlation analysis of the 14 gene modules and phenotypes (M_HC or M_AS) was carried out. Among them, the green module showed the highest correlation with M_AS (cor =0.76, P=9e–08) (Figure 5C). Further BP analysis of the genes in the green modules revealed that the BP were mainly in the neutrophil activation, neutrophil degranulation, regulation of cell morphogenesis, and neutrophil activation involved in immune response (Figure 5D). Further KEGG analysis of the genes in the green modules revealed that the pathways were mainly enriched in the T-cell receptor signaling pathway, the B-cell receptor signaling pathway, osteoclast differentiation, leukocyte transendothelial migration, and platelet activation (Figure 5E). The results showed that most of the genes in the green module were enriched in pathways related to immune response.
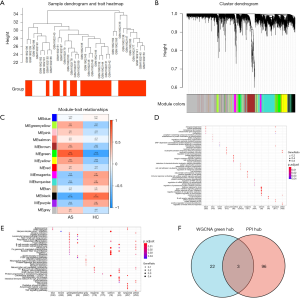
Determining the key genes
The hub genes screened by WGCNA and the genes in the first 5 clusters of the PPI network were intersected. Finally, three overlapping genes, RAB5C, SYNJ1, and RNF19B were obtained, and were identified as key genes (Figure 5F). The expression of RAB5C, SYNJ1, and RNF19B differed significantly between the M_HC and M_AS groups (Figure S1).
Immune infiltration analysis
Immune infiltration analysis through the CIBERSORT website found that 17 types of immune cells were expressed in the tested samples. The proportions of infiltrating immune cells in each sample was shown in Figure 6A. Correlation analysis of these 17 immune cells revealed positive correlations between regulatory T cells (Tregs) and memory B cells, activated memory CD4 T cells and CD8 T cells, and resting mast cells and activated memory CD4 T cells. Meanwhile, negative correlations were found between neutrophils and CD8 T cells, Tregs and activated memory CD4 T cells, and activated and resting NK cells (Figure 6B). Through PCA, we uncovered differences in immune cell infiltration between the M_HC group and M_AS group (Figure 6C). Similar results were also obtained after grouping of the samples according to the expression levels of RAB5C, SYNJ1, and RNF19B (Figure S2). Subsequently, through the violin plot of differences in immune cell infiltration, we observed an increase in monocytes and a decrease in gamma delta T cells in the M_AS group compared with the M_HC group (Figure 6D). After grouping according to the expression levels of the key genes, we found that activated NK cells, activated DCs, and M2 macrophages were decreased, whereas monocytes and M0 macrophages were increased in the RAB5C high expression group compared with the RAB5C low expression group. In the SYNJ1 high expression group, monocytes and M0 macrophages were increased, while M2 macrophages and activated DCs were decreased compared with the SYNJ1 low expression group. Furthermore, CD8 T cells were decreased, while monocytes, M0 macrophages, and neutrophils were increased in the high RNF19B expression group compared with the low RNF19B expression group (Figure 7A,B,C).
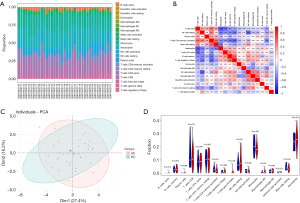
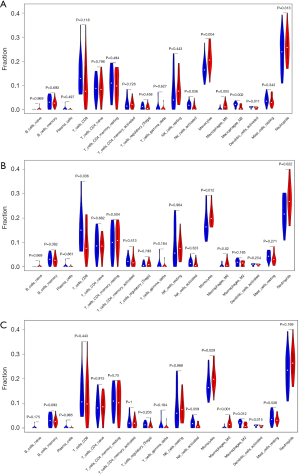
Discussion
AS is a refractory and highly disabling autoimmune disease affecting the spine and joints (24), and it places a massive burden on patients’ families and society. Therefore, it is of great importance to clarify the pathogenesis of AS and discover a therapeutic target. AS is more common among young people than older people. According to the literature, the ratio of male to female patients of AS varies between 10/1 and 3/1, anyhow there are more male patients than female patients (25,26). Therefore, in this study, we extracted the male cases from the dataset for analysis and used FDR <0.05 and |log FC| >0.263 as the standard to screen the DEGs. A total of 689 DEGs were obtained, of which 395 genes were up-regulated and 294 genes were down-regulated. Interestingly, we also extracted the data of female cases with the intention of screening out DEGs according to the same standards, but no DEGs were obtained. This indicates that the DEGs between patients with AS and healthy individuals are sex-specific. These DEGs may be related to the difference in the incidence of AS between men and women. Some research has also supported this view. For instance, Th17 has been found to be the key factor in determining the sexual dimorphism of AS, and can be activated by STAT3 and STAT5 (15,27); STAT3 and STAT5 appear in our DEG list. However, more data is needed to confirm this conclusion.
GO enrichment analysis showed that the DEGs were mainly related to neutrophil degranulation, regulation of I kappaB kinase/NF-κB signaling, and mast cell degranulation. These results suggest that immune response plays an important role in AS. A meta-analysis of 10 studies involving 765 patients with AS and 701 healthy individuals showed that the neutrophil lymphocyte ratio (NLR) of the AS group was significantly higher than that of the healthy control group (28). Studies have also found that compared with healthy controls, the expression levels of I kappaB alpha (IκB-α), NF-κB, and mitogen-activated protein kinase 14 (MAPK14) genes in patients with AS are significantly higher, and M2000 can treat AS by inhibiting the TLR/NF-κB signaling pathway (29). Mast cell hyperplasia is a feature of rheumatoid arthritis and osteoarthritis; however, in a variety of autoimmune disorders, including AS, mast cells can regulate the pathophysiological process of the disease by expressing interleukin (IL)-17A (30,31). The above reports give strong support to the results of our GO enrichment analysis, and we also obtained similar results through GSEA. The GSEA results showed that the enriched pathways mainly included platelet activation and the TNF signaling pathway. Wang et al. used flow cytometry to detect the number of CD62P- and CD63-positive cells, and found that the proportions of these cells in patients with AS were much higher than those in the normal control group. They therefore concluded that platelet activation may be a sign of AS aggravation (32). Sode et al. found that high activation of the TNF-α, IL-23/IL-17, and NF-κB pathways was associated with an increased risk of AS (33). The above research results are consistent with our data, which indicates that the results of the present study are accurate.
WGCNA is a method that can be used to search for co-expressed gene modules, explore the relationship between gene networks and phenotypes of interest, and determine hub genes. Since the advent of the “WGCNA” package, this method has been widely used for the analysis of gene co-expression networks in various tumors and non-neoplastic diseases, and has been universally recognized (22,34-36). In this study, we obtained 14 modules through WGCNA. Through correlation analysis, we found that the green module showed the highest correlation with AS. After analyzing the genes in the green module by function and pathway enrichment analysis, we found that the genes in this module are mainly enriched in immune response-related functions and pathways. This result is extremely similar to those of the DEG functional enrichment analysis and GSEA. Therefore, we infer that the green module is an important module that regulates AS through immune response.
To further study the interrelationship of genes in the module, we set a threshold (MM >0.8 and GS >0.7) and screened out the hub genes. We performed correlation analysis of the hub genes and found that all of them were positively correlated. The hub genes analyzed through WGCNA were intersected with the genes in the first 5 clusters of the PPI network. Finally, three overlapping genes were obtained: RAB5C, SYNJ1, and RNF19B. RAB5C is a member of the RAB5 family. RAB5 protein is a guanosine triphosphatase related to endosomal classification which participates in endosomal membrane fusion (37,38). Studies have shown that RAB5C can promote the replication of respiratory syncytial virus by regulating vesicle transport (39). RAB5C acts as a key regulator in the process of endothelial cell adhesion and migration (40), and also plays a vital regulatory role in a variety of immune and inflammatory responses (41). In view of the important role played by immune response and inflammatory response in the occurrence and development of AS (42), we believe that RAB5C may participate in the pathophysiological process of AS by regulating the immune response or immune cell migration and adhesion. The protein encoded by the SYNJ1 gene is a lipid phosphatase that is abundant in brain tissue and plays an important role in synaptic vesicle circulation and phosphatidylinositol metabolism (43). Previous studies have shown that SYNJ1 performs a critical regulatory role in the occurrence and development of various neurological diseases such as Alzheimer’s disease and Parkinson’s disease (44,45). Moreover, SYNJ1 also has an important regulatory effect on autophagy, and studies have shown that it is a regulatory factor with crucial involvement in the maturation of presynaptic terminal autophagosomes in Alzheimer’s (46). Autophagy is also a key player in the pathophysiological process of AS (47). Thus, we speculate that SYNJ1 can affect the occurrence and development of AS via regulating autophagy. RNF19B, also called NK lysozyme-related molecule, is a unique member of the E3 ubiquitin ligase small family, which has vital involvement in innate immunity, mainly through its regulation of NK cells and macrophages (48). In our immune cell infiltration analysis, we found that the levels of monocytes and M0 macrophages in the RNF19B high expression group were elevated, which is consistent with the results reported in the literature. Similarly, in a mouse model of viral pneumonia, the absence of RNF19B led to a decrease in the production of inflammatory factors, which indicates that RNF19B regulates the production of pro-inflammatory cytokines during viral infection (49). Since innate immunity is critical to the pathophysiological process of AS (50), we speculate that RNF19B may promote AS occurrence and development by regulating NK cells and macrophages. However, many more clinical and experimental studies are needed to verify this.
To further explore the role of immune cell infiltration in AS, CIBERSORT was used to comprehensively evaluate AS immune infiltration. Compared with the M_HC group, monocytes were increased in the M_AS group, while gamma delta T cells were decreased. According to previous research, monocytes can promote the development of AS, and monocyte-to-lymphocyte ratio is significantly increased in patients with AS (51,52), which is consistent with our findings. Regarding gamma delta T cells, some reports have suggested that the proportions of these cells are reduced in patients with AS (53), which is also consistent with our results. However, some studies have reported that the proportions of these cells in patients with AS are increased (54), which contradicts our results. Therefore, more clinical and experimental data are needed. In the immune infiltration analysis of groups with high and low expression of the three key genes (RAB5C, SYNJ1, and RNF19B), we found that monocytes and M0 macrophages were elevated in the three high expression groups. Therefore, it is speculated that RAB5C, SYNJ1, and RNF19B may promote the development of AS by regulating monocytes and macrophages. In the future, further research is needed to clarify the complex interactions between genes and immune cells to test this hypothesis.
Conclusions
In conclusion, differences exist in immune infiltration between M_AS and M_HC, and monocytes and gamma delta T cells may play a key role in this process. Through differential analysis, PPI network analysis, and WGCNA, we identified RAB5C, SYNJ1, and RNF19B as key genes. Further analysis revealed that these genes are associated with increases in monocytes and macrophages. Therefore, we speculate that RAB5C, SYNJ1, and RNF19B may promote AS by regulating monocytes and macrophages. Our analysis provides a certain reference for further clarification of the mechanisms of AS development and the discovery of possible therapeutic targets.
Acknowledgments
Funding: This study was supported by the Science and Technology Program of Guangzhou, China (201707010089), Medical Science and Technology Research Foundation of Guangdong Province, Guangzhou, China (A2021371), and Funding of Basics and Application Basics of Guangzhou, China (202102020096).
Footnote
Reporting Checklist: The authors have completed the MDAR reporting checklist. Available at https://dx.doi.org/10.21037/atm-21-2721
Conflicts of Interest: All authors have completed the ICMJE uniform disclosure form (available at https://dx.doi.org/10.21037/atm-21-2721). The authors have no conflicts of interest to declare.
Ethical Statement: The authors are accountable for all aspects of the work in ensuring that questions related to the accuracy or integrity of any part of the work are appropriately investigated and resolved. The study was conducted in accordance with the Declaration of Helsinki (as revised in 2013).
Open Access Statement: This is an Open Access article distributed in accordance with the Creative Commons Attribution-NonCommercial-NoDerivs 4.0 International License (CC BY-NC-ND 4.0), which permits the non-commercial replication and distribution of the article with the strict proviso that no changes or edits are made and the original work is properly cited (including links to both the formal publication through the relevant DOI and the license). See: https://creativecommons.org/licenses/by-nc-nd/4.0/.
References
- Simone D, Al Mossawi MH, Bowness P. Progress in our understanding of the pathogenesis of ankylosing spondylitis. Rheumatology (Oxford) 2018;57:vi4-9. [Crossref] [PubMed]
- Smith JA. Update on ankylosing spondylitis: current concepts in pathogenesis. Curr Allergy Asthma Rep 2015;15:489. [Crossref] [PubMed]
- Liu CH, Raj S, Chen CH, et al. HLA-B27-mediated activation of TNAP phosphatase promotes pathogenic syndesmophyte formation in ankylosing spondylitis. J Clin Invest 2019;129:5357-73. [Crossref] [PubMed]
- Sieper J, Poddubnyy D. Axial spondyloarthritis. Lancet 2017;390:73-84. [Crossref] [PubMed]
- Rezaiemanesh A, Abdolmaleki M, Abdolmohammadi K, et al. Immune cells involved in the pathogenesis of ankylosing spondylitis. Biomed Pharmacother 2018;100:198-204. [Crossref] [PubMed]
- Hallett RM, Chew T. Immune cell transcript modules reveal leukocyte heterogeneity in synovial biopsies of seronegative spondylarthropathy patients. BMC Musculoskelet Disord 2014;15:446. [Crossref] [PubMed]
- Liu YF, Zhuang KH, Chen B, et al. Expansion and activation of monocytic-myeloid-derived suppressor cell via STAT3/arginase-I signaling in patients with ankylosing spondylitis. Arthritis Res Ther 2018;20:168. [Crossref] [PubMed]
- Yang M, Lv Q, Wei Q, et al. TNF-α inhibitor therapy can improve the immune imbalance of CD4+ T cells and negative regulatory cells but not CD8+ T cells in ankylosing spondylitis. Arthritis Res Ther 2020;22:149. [Crossref] [PubMed]
- Newman AM, Liu CL, Green MR, et al. Robust enumeration of cell subsets from tissue expression profiles. Nat Methods 2015;12:453-7. [Crossref] [PubMed]
- Ye L, Zhang T, Kang Z, et al. Tumor-infiltrating immune cells act as a marker for prognosis in colorectal cancer. Front Immunol 2019;10:2368. [Crossref] [PubMed]
- Zhang S, Zhang E, Long J, et al. Immune infiltration in renal cell carcinoma. Cancer Sci 2019;110:1564-72. [Crossref] [PubMed]
- Cai W, Li H, Zhang Y, et al. Identification of key biomarkers and immune infiltration in the synovial tissue of osteoarthritis by bioinformatics analysis. PeerJ 2020;8:e8390 [Crossref] [PubMed]
- Barrett T, Wilhite SE, Ledoux P, et al. NCBI GEO: archive for functional genomics data sets--update. Nucleic Acids Res 2013;41:D991-5. [Crossref] [PubMed]
- Davis S, Meltzer PS. GEOquery: a bridge between the Gene Expression Omnibus (GEO) and BioConductor. Bioinformatics 2007;23:1846-7. [Crossref] [PubMed]
- Gracey E, Yao Y, Green B, et al. Sexual Dimorphism in the Th17 Signature of Ankylosing Spondylitis. Arthritis Rheumatol 2016;68:679-89. [Crossref] [PubMed]
- Ritchie ME, Phipson B, Wu D, et al. limma powers differential expression analyses for RNA-sequencing and microarray studies. Nucleic Acids Res 2015;43:e47 [Crossref] [PubMed]
- Fan X, Qi B, Ma L, et al. Screening of underlying genetic biomarkers for ankylosing spondylitis. Mol Med Rep 2019;19:5263-74. [Crossref] [PubMed]
- Yu G, Wang LG, Han Y, et al. clusterProfiler: an R package for comparing biological themes among gene clusters. Omics 2012;16:284-7. [Crossref] [PubMed]
- Liu J, Zhou S, Li S, et al. Eleven genes associated with progression and prognosis of endometrial cancer (EC) identified by comprehensive bioinformatics analysis. Cancer Cell Int 2019;19:136. [Crossref] [PubMed]
- Deng JL, Xu YH, Wang G. Identification of Potential Crucial Genes and Key Pathways in Breast Cancer Using Bioinformatic Analysis. Front Genet 2019;10:695. [Crossref] [PubMed]
- Tilford CA, Siemers NO. Gene set enrichment analysis. Methods Mol Biol 2009;563:99-121. [Crossref] [PubMed]
- Langfelder P, Horvath S. WGCNA: an R package for weighted correlation network analysis. BMC Bioinformatics 2008;9:559. [Crossref] [PubMed]
- Zou D, Li R, Huang X, et al. Identification of molecular correlations of RBM8A with autophagy in Alzheimer's disease. Aging (Albany NY) 2019;11:11673-85. [Crossref] [PubMed]
- Jiang N, Liu HX, Liang HY, et al. Osteogenic differentiation characteristics of hip joint capsule fibroblasts obtained from patients with ankylosing spondylitis. Ann Transl Med 2021;9:331. [Crossref] [PubMed]
- Moll JM, Haslock I, Macrae IF, et al. Associations between ankylosing spondylitis, psoriatic arthritis, Reiter's disease, the intestinal arthropathies, and Behcet's syndrome. Medicine (Baltimore) 1974;53:343-64. [Crossref] [PubMed]
- Will R, Edmunds L, Elswood J, et al. Is there sexual inequality in ankylosing spondylitis? A study of 498 women and 1202 men. J Rheumatol 1990;17:1649-52. [PubMed]
- Zhao P, Li J, Tian Y, et al. Restoring Th17/Treg balance via modulation of STAT3 and STAT5 activation contributes to the amelioration of chronic obstructive pulmonary disease by Bufei Yishen formula. J Ethnopharmacol 2018;217:152-62. [Crossref] [PubMed]
- Xu S, Ma Y, Wu M, et al. Neutrophil lymphocyte ratio in patients with ankylosing spondylitis: A systematic review and meta-analysis. Mod Rheumatol 2020;30:141-8. [Crossref] [PubMed]
- Roozbehkia M, Mahmoudi M, Aletaha S, et al. The potent suppressive effect of β-d-mannuronic acid (M2000) on molecular expression of the TLR/NF-kB Signaling Pathway in ankylosing spondylitis patients. Int Immunopharmacol 2017;52:191-6. [Crossref] [PubMed]
- Patel DD, Lee DM, Kolbinger F, et al. Effect of IL-17A blockade with secukinumab in autoimmune diseases. Ann Rheum Dis 2013;72:ii116-23. [Crossref] [PubMed]
- Buckley MG, Walters C, Wong WM, et al. Mast cell activation in arthritis: detection of alpha- and beta-tryptase, histamine and eosinophil cationic protein in synovial fluid. Clin Sci (Lond) 1997;93:363-70. [Crossref] [PubMed]
- Wang F, Yan CG, Xiang HY, et al. The significance of platelet activation in ankylosing spondylitis. Clin Rheumatol 2008;27:767-9. [Crossref] [PubMed]
- Sode J, Bank S, Vogel U, et al. Genetically determined high activities of the TNF-alpha, IL23/IL17, and NFkB pathways were associated with increased risk of ankylosing spondylitis. BMC Med Genet 2018;19:165. [Crossref] [PubMed]
- Liang W, Sun F, Zhao Y, et al. Identification of susceptibility modules and genes for cardiovascular disease in diabetic patients using WGCNA analysis. J Diabetes Res 2020;2020:4178639 [Crossref] [PubMed]
- Niemira M, Collin F, Szalkowska A, et al. Molecular signature of subtypes of non-small-cell lung cancer by large-scale transcriptional profiling: identification of key modules and genes by weighted gene co-expression network analysis (WGCNA). Cancers (Basel) 2019;12:37. [Crossref] [PubMed]
- Tian Z, He W, Tang J, et al. Identification of Important Modules and Biomarkers in Breast Cancer Based on WGCNA. Onco Targets Ther 2020;13:6805-17. [Crossref] [PubMed]
- Woodman PG. Biogenesis of the sorting endosome: the role of Rab5. Traffic 2000;1:695-701. [Crossref] [PubMed]
- Chen PI, Kong C, Su X, et al. Rab5 isoforms differentially regulate the trafficking and degradation of epidermal growth factor receptors. J Biol Chem 2009;284:30328-38. [Crossref] [PubMed]
- Li J, Li M, Wang X, et al. Long noncoding RNA NRAV promotes respiratory syncytial virus replication by targeting the microRNA miR-509-3p/Rab5c axis to regulate vesicle transportation. J Virol 2020;94:e00113-20. [Crossref] [PubMed]
- Barbera S, Nardi F, Elia I, et al. The small GTPase Rab5c is a key regulator of trafficking of the CD93/Multimerin-2/β1 integrin complex in endothelial cell adhesion and migration. Cell Commun Signal 2019;17:55. [Crossref] [PubMed]
- Prashar A, Schnettger L, Bernard EM, et al. Rab GTPases in immunity and inflammation. Front Cell Infect Microbiol 2017;7:435. [Crossref] [PubMed]
- Pedersen SJ, Maksymowych WP. The pathogenesis of ankylosing spondylitis: an update. Curr Rheumatol Rep 2019;21:58. [Crossref] [PubMed]
- Wenk MR, Pellegrini L, Klenchin VA, et al. PIP kinase Igamma is the major PI(4,5)P(2) synthesizing enzyme at the synapse. Neuron 2001;32:79-88. [Crossref] [PubMed]
- Xie F, Chen S, Cen ZD, et al. A novel homozygous SYNJ1 mutation in two siblings with typical Parkinson's disease. Parkinsonism Relat Disord 2019;69:134-7. [Crossref] [PubMed]
- Ando K, Ndjim M, Turbant S, et al. The lipid phosphatase Synaptojanin 1 undergoes a significant alteration in expression and solubility and is associated with brain lesions in Alzheimer's disease. Acta Neuropathol Commun 2020;8:79. [Crossref] [PubMed]
- Vanhauwaert R, Kuenen S, Masius R, et al. The SAC1 domain in synaptojanin is required for autophagosome maturation at presynaptic terminals. Embo j 2017;36:1392-411. [Crossref] [PubMed]
- Feng Y, Li B, Li XY, et al. The Role of Autophagy in Rheumatic Disease. Curr Drug Targets 2018;19:1009-17. [Crossref] [PubMed]
- Lawrence DW, Willard PA, Cochran AM, et al. Natural killer lytic-associated molecule (NKLAM): An E3 ubiquitin ligase with an integral role in innate immunity. Front Physiol 2020;11:573372 [Crossref] [PubMed]
- Lawrence DW, Shornick LP, Kornbluth J. Mice deficient in NKLAM have attenuated inflammatory cytokine production in a Sendai virus pneumonia model. PLoS One 2019;14:e0222802 [Crossref] [PubMed]
- Vanaki N, Aslani S, Jamshidi A, et al. Role of innate immune system in the pathogenesis of ankylosing spondylitis. Biomed Pharmacother 2018;105:130-43. [Crossref] [PubMed]
- Huang Y, Deng W, Zheng S, et al. Relationship between monocytes to lymphocytes ratio and axial spondyloarthritis. Int Immunopharmacol 2018;57:43-6. [Crossref] [PubMed]
- Ciccia F, Guggino G, Zeng M, et al. Proinflammatory CX3CR1+CD59+tumor necrosis factor-like molecule 1A+interleukin-23+ monocytes are expanded in patients with ankylosing spondylitis and modulate innate lymphoid cell 3 immune functions. Arthritis Rheumatol 2018;70:2003-13. [Crossref] [PubMed]
- Toussirot É, Laheurte C, Gaugler B, et al. Increased IL-22- and IL-17A-producing mucosal-associated invariant T cells in the peripheral blood of patients with ankylosing spondylitis. Front Immunol 2018;9:1610. [Crossref] [PubMed]
- Al-Mossawi MH, Chen L, Fang H, et al. Unique transcriptome signatures and GM-CSF expression in lymphocytes from patients with spondyloarthritis. Nat Commun 2017;8:1510. [Crossref] [PubMed]
(English Language Editor: J. Reynolds)