Screening and prognostic value of potential biomarkers for ovarian cancer
Introduction
Ovarian cancer is the most common cause of death among the gynecologic malignancies, and mainly occurs in women aged over 50 years. This disease is frequently diagnosed at the late stage because patients are often asymptomatic, which seriously affects prognosis (1). Approximately 90% of ovarian cancers are epithelial tumors, which are classified into five histotypes: serous, endometrioid, mucinous, clear cells and undifferentiated. Advanced serous cancer is the most common and fatal of the ovarian cancers. In North America, advanced serous cancer accounts for 70% of all ovarian cancers and 90% of deaths from ovarian cancer (2,3). Endometrial carcinoma accounts for ~10% of ovarian cancers, and is usually low stage and low grade. Undifferentiated serous ovarian cancer has a high mortality rate, and is often associated with metastasis and drug resistance (4). Mucinous ovarian cancer is a rare tumor, accounting for ~3% of all epithelial ovarian cancers (5,6). Each type of ovarian cancer has its own molecular phenotype and unique treatment, so it is challenging to diagnose and treat this disease. To control the progression of ovarian cancer, its pathogenesis must be understood, which will help to better screen the populations at high risk for ovarian cancer, and the identification of potentially changeable pathological factors provides an opportunity to reduce morbidity (7). At present, the molecular mechanism of ovarian cancer remains unclear, which hinders prognosis and the development of treatment strategies. Therefore, it is an urgent task to provide new biomarkers or biological targets for ovarian cancer.
We present the following article in accordance with the REMARK reporting checklist (available at https://dx.doi.org/10.21037/atm-21-2627).
Methods
Data collection
Ovarian cancer-related clinical information and gene expression information were downloaded from the TCGA. All samples were ovarian cancer. Only samples of severe ovarian cancer are kept in the GEO dataset. The study was conducted in accordance with the Declaration of Helsinki (as revised in 2013).
Data processing
Trimmed mean of M values (TMM) was used to normalize the TCGA sequencing data (8), and Robust multi-array average (RMA) was used to process the GEO data (9). After removing low-expression samples, the following criteria were used: for sequencing data, reads per kb per million (rpkm) ≥1 in at least one-third of the samples; for chip data, normalized expression value ≥ median value of overall expression in at least one-third of the samples.
Survival analysis
To further screen the genes greatly associated with ovarian cancer survival, we first analyzed the TCGA and GEO datasets using univariable Cox proportional hazards regression analysis. The threshold value was set to P<0.05. The key genes in the two datasets were screened, and key genes co-expressed by the two datasets were selected. The genes with common effect on tumor survival in the two datasets [log2 (HR) values >0 or <0] were further selected. The LASSO method was used to set 300 calculations (10). The genes with frequency ≥150 were defined as potentially highly related to survival. Finally, the P values of the Cox regression model of these potential genes highly related to survival were analyzed and compared between the TCGA and GEO datasets.
Risk model construction
A multivariate Cox regression model was used to analyze the genes that were potentially highly related to survival. Stepwise regression analysis was used to select the smallest akaike information criteria (AIC) information statistics for gene deletion. The remaining genes were used for risk model construction. The risk formula was generated according to the Cox regression results. The risk coefficient of each patient was calculated according to the formula. The patients were classified according to the median risk value: low-risk group (low median risk value) and high-risk group (high median risk value).
GSEA analysis
The log2 difference folds (high vs. low) of the genes detected in the datasets were analyzed. The genes were sequenced according to log2 difference folds (from high to low). The database used for GSEA analysis was the KEGG dataset (data from https://www.gsea-msigdb.org/gsea/msigdb). The pathways with Padj <0.05 and absolute value of normalized enrichment score (NES) ≥1 were considered as enriched pathways (11). The first six of them (arranged by P value from low to high) were selected to create the distribution curve of the enrichment score.
Immune cell infiltration analysis
To analyze the differences in the proportion of immune cells between samples of high and low risk for ovarian cancer, immune cell infiltration analysis of the TCGA datasets was performed by the Timer (tumor immune estimation resource; https://cistrome.shinyapps.io/timer) (12) and Cibersort (https://cibersort.stanford.edu/) (13), respectively. The Wilcoxon test was used to analyze the differences in cell proportions between samples of high- and low-risk ovarian cancer (14).
Statistical analysis
To evaluate the predictive accuracy of the risk score model, the receiver operating characteristic (ROC) curve was plotted and the area under the ROC (AUC) was calculated. The effects of age, grade, stage, risk status and other potential prognostic factors in the TCGA and GEO datasets were analyzed using univariate and multivariate Cox regression analyses.
Results
Principal component analysis (PCA)
To investigate the feasibility of subsequent study, PCA was applied to the TCGA and GEO datasets (Figure 1). There were no outliers in the PCA results, indicating that sample homogeneity was good.
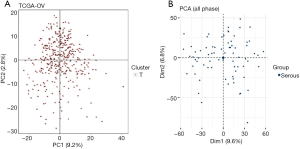
Functional analysis of co-expressed genes
Univariate Cox proportional hazards regression was used to analyze the ovarian cancer data in the TCGA and GEO datasets and screen out co-expressed genes. According to the log2(HR) value, 111 co-expressed genes with common effects on ovarian cancer survival were screened (Table 1). The function of these co-expressed genes was analyzed based on the GO and KEGG datasets. Based on the results of biological process (BP), a type of GO analysis, these 111 co-expressed genes mainly participated in metabolism, protein phosphorylation, and other functions. According to the KEGG results, these genes were involved in immune-related signaling pathways (Figure 2A,B).
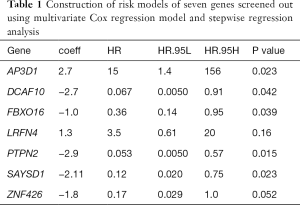
Full table
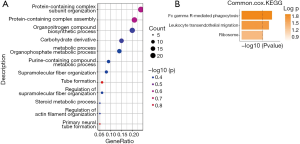
Screening of ovarian cancer survival-related genes
To investigate the relationship between ovarian cancer-related genes and survival, the LASSO method was used to further analyze the 111 co-expressed genes from the TCGA and GEO datasets. A total of 42 and 18 genes with a frequency ≥150 that were highly related to ovarian cancer survival were selected from the TCGA and CEO datasets, respectively (Figure 3A,B). Interestingly, nine common genes were found (Figure 3C). Finally, the P value of the Cox proportional hazards regression of these nine genes was compared between the TCGA and GEO datasets (Figure 3D). The results showed that AP3D1, DCAF10, FBXO16, LRFN4, PTPN2, SAYSD1, SMU1, WAC.AS1 and ZNF426 were highly related to ovarian cancer survival. Subsequent study of ovarian cancer biomarkers should be performed based on these nine genes.
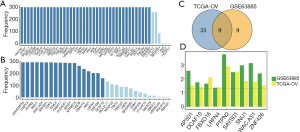
Prognostic value of ovarian cancer markers
To investigate the prognostic value of ovarian cancer markers, the nine genes were analyzed using a multivariate Cox regression model, and seven risk genes for ovarian cancer were finally screened out using stepwise regression analysis (Table 1). According to the Cox regression results, the risk formula was constructed as below: 2.7090 * AP3D1-2.6960 * DCAF10-1.0232 * FBXO16 +1.2613 * LRFN4-2.9331 * PTPN2-2.1094 * SAYSD1-1.7618 * ZNF426. According to the median risk value, patients in the TCGA and GEO datasets were assigned to low-risk and high-risk groups, and the ovarian cancer survival curve was plotted (Figure 4), which showed that patients at a low risk of ovarian cancer had good survival status. The heat map (Figure 5) shows the expression level of risk genes in the low-risk and high-risk groups from the TCGA dataset and the distribution of clinical pathological features. Accordingly, AP3D1 and LRFN4 are highly expressed in patients with low survival and are considered risk genes for ovarian cancer. DCAF10, FBXO16, PTPN2, SAYSD1, SMU1, WAC.AS1 and ZNF426 are considered protective genes. Univariate and multivariate Cox regression analyses were performed to analyze the effects of age, stage, grade, and risk status on ovarian cancer survival based on the TCGA and CEO datasets (Tables 2,3). Both univariate and multivariate Cox regression analysis results revealed that risk status was related to survival. These findings demonstrate that risk status may be an independent prognostic factor. To analyze the predictive value of a risk score for ovarian cancer prognosis, a time-dependent ROC curve was plotted according to the risk score of seven genes screened out of the TCGA and CEO datasets (Figure 6). In the TCGA dataset, the AUC for 1-, 3- and 5-year prognosis models was 0.6, 0.628 and 0.667, respectively, and 0.741, 0.9 and 0.955 in the GEO dataset. Therefore, in the two datasets, the predictive power of 1-, 3- and 5-year prognosis models was very high. Generally speaking, the AUC values were mostly >0.6, and therefore, the 1-, 3- and 5-year prognosis models had high predictive value.
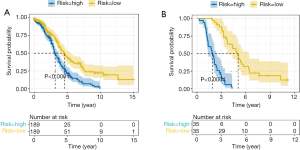
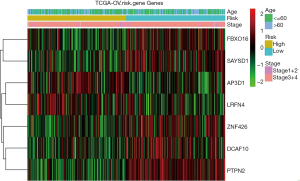
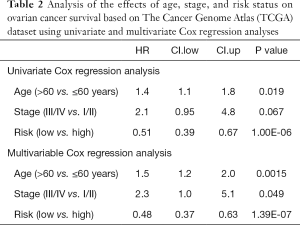
Full table
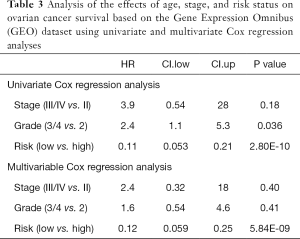
Full table
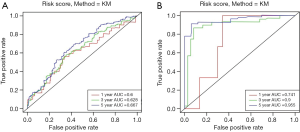
Investigation of the signaling pathways involved in ovarian cancer using GSEA
The GSEA results revealed that in the samples from patients at high risk of ovarian cancer the immune-related pathways were upregulated and ribosomal pathway was downregulated (Figure 7). The first six pathways (arranged by P values from low to high) were used to plot the distribution curves of the enrichment score (Figure 8), which showed that the genes in “complement and coagulation cascades”, “ECM-receptor interaction”, “Hematopoietic cell lineage”, “Th1 and Th2 cell differentiation” and “Toll-like receptor signaling pathway” were clustered in the high-expression region, and the genes in the ribosomal pathway were clustered in the low-expression region. Generally speaking, the biological functions of the signaling pathways involved in ovarian cancer in high-risk patients were mostly related to the patient’s immune function.
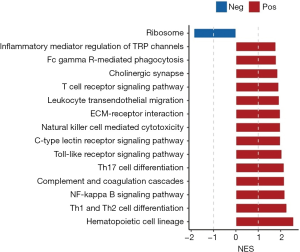
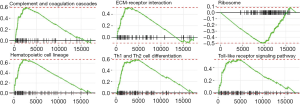
Immune cell infiltration analysis using Timer and Cibersort
Immune cell infiltration analysis using Timer and Cibersort (Figures 9,10) revealed that high-risk status samples tended to have high abundance of most immune cells. B cells, mDC activated cells and NK(natural killer)-activated cells were less expressed in high-risk status samples, and CD4+ T cells, macrophages, mDC cells and neutrophils were highly expressed in high-risk status samples.
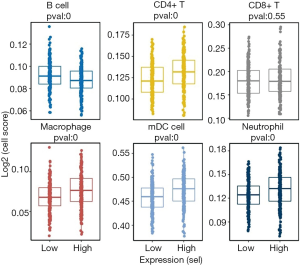
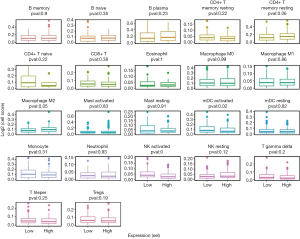
Discussion
Ovarian cancer is one of the most common gynecological malignancies and carries a poor prognosis (15). The molecular mechanism underlying the occurrence and development of ovarian cancer remains unclear. In this study, we investigated the relationship between seven ovarian cancer-related genes and the occurrence and development of ovarian cancer. More importantly, we found that these ovarian cancer-related genes were closely related to the degree of malignancy and prognosis of ovarian cancer. Our findings add more evidence to existing knowledge of the occurrence and development of ovarian cancer.
To investigate the relationship between ovarian cancer-related genes and survival, we screened nine genes related to survival and further investigated them. Firstly, we used a multivariate Cox regression model and stepwise regression analysis to screen seven risk genes to construct the risk model, then univariate and multivariate Cox regression analyses determined that risk status may be an independent prognostic factor. In addition, we used ROC curves to analyze the predictive value of a risk score for ovarian cancer prognosis. We calculated the AUC value and found that the risk score had a high predictive value. We found that AP3D1, DCAF10, FBXO16, LRFN4, PTPN2, SAYSD1 and ZNF426 can be used as independent markers of ovarian cancer. Finally, we performed GSEA and immune cell infiltration analyses to analyze the biological functions of these genes and confirmed the important significance of these genes as new biomarkers of ovarian cancer. We found that AP3D1 and LRFN4 are risk genes, and their high expression inhibits the survival of ovarian cancer patients. AP3D1 and LRFN4 promote the occurrence and development of many cancers or diseases. In colorectal cancer, the loss of the optic nerve element leads to immune evasion and intrinsic immunotherapy resistance. Unexpectedly, the optic nerve element interacts with AP3D1 to prevent the sorting and degradation of palmitoylated IFNGR1 lysosomes, thereby maintaining the integrity of interferon gamma and MHC (major histocompatibility complex)-I signaling (16). Hermansky-Pudlak syndrome represents a group of immune dysfunction syndromes, and mutation of AP3D1 encoding the main subunit AP-3 (δ) is the cause of Hermansky-Pudlak syndrome type 10 (17). In addition, leucine-rich repeat and fibronectin type III domain-containing (LRFN) family proteins are considered to be neuron-specific proteins. LRFN4 is expressed in cells in various cancers and leukemia. LRFN4 signaling plays an important role in monocyte/macrophage migration (18).
However, it is reported that AP3D1 and LRFN4 also play a protective role in many diseases. For example, envelope glycoprotein 51 (gp51) is necessary for bovine leukosis virus to enter bovine B lymphocytes. Bovine adaptor protein 3 complex subunit δ-1 is considered to be a potential receptor. There is evidence that the N-terminal part of gp51 interacts with the AP3D1 receptor in vitro, and provides a reliable silicon interaction model (19). In colorectal cancer, LRFN4 expression is upregulated, which strongly correlates with clinical pathological features and prognosis. High expression of LRFN4 reduces the risk of death in colorectal cancer patients (20).
We found that DCAF10, FBXO16, PTPN2, SAYSD1 and ZNF426 are protective genes in ovarian cancer patients, and their high expression promotes survival. These genes inhibit the occurrence and development of many cancers and diseases. For example, FBXO16 functions as a tumor suppressor, and is a component of the skp1-cullin1-f-box protein complex that targets nuclear β-catenin and promotes proteasome degradation through 26S proteasome. Depletion of FBXO16 results in increased levels of β-catenin, which in turn promotes cancer cell invasion, tumor growth and epithelial-mesenchymal transition (21). In breast cancer, FBXO16 is considered to be a potential clinical target and prognostic biomarker for patients with different molecular types of breast cancer (22). In addition, it has been reported that increasing the number of cytotoxic Tim-3+/CD8+ T cells can promote effective anti-tumor immunity, and PTPN2 is an attractive tumor immunotherapy target in immune cells (23). In breast cancer, PTPN2 protein is lost in half of breast cancer patients. The role of PTPN2 is related to the subtype of breast cancer, and PTPN2 affects the prognosis and treatment response of breast cancer (24).
However, these genes can also promote the occurrence and development of some diseases. For example, mutations in the gene locus encoding protein tyrosine phosphatase non receptor type 2 (i.e., PTPN2) are associated with inflammatory diseases, including inflammatory bowel disease, rheumatoid arthritis, and type 1 diabetes (25). In thyroid cancer, inflammation or oxidative stress induces the upregulation of PTPN2, which promotes the progression of thyroid cancer (26).
Conclusions
We identified that among the ovarian cancer-related genes, AP3D1, DCAF10, FBXO16, LRFN4, PTPN2, SAYSD1, and ZNF426 were greatly associated with the progression of ovarian cancer. AP3D1 and LRFN4 were risk genes, and DCAF10, FBXO16, PTPN2, SAYSD1 and ZNF426 were protective genes, which could be used as independent prognostic markers of ovarian cancer. Moreover, there was severe inflammatory infiltration in the tumors of patients at a high risk of ovarian cancer. This study adds more evidence to confirming the pathogenesis of ovarian cancer, determining prognostic indicators of ovarian cancer, and providing potential targets for targeted therapy of ovarian cancer.
Acknowledgments
Funding: None.
Footnote
Reporting Checklist: The authors have completed the REMARK reporting checklist. Available at https://dx.doi.org/10.21037/atm-21-2627
Conflicts of Interest: All authors have completed the ICMJE uniform disclosure form (available at https://dx.doi.org/10.21037/atm-21-2627). The authors have no conflicts of interest to declare.
Ethical Statement: The authors are accountable for all aspects of the work in ensuring that questions related to the accuracy or integrity of any part of the work are appropriately investigated and resolved. The study was conducted in accordance with the Declaration of Helsinki (as revised in 2013).
Open Access Statement: This is an Open Access article distributed in accordance with the Creative Commons Attribution-NonCommercial-NoDerivs 4.0 International License (CC BY-NC-ND 4.0), which permits the non-commercial replication and distribution of the article with the strict proviso that no changes or edits are made and the original work is properly cited (including links to both the formal publication through the relevant DOI and the license). See: https://creativecommons.org/licenses/by-nc-nd/4.0/.
References
- Kujawa KA, Lisowska KM. Ovarian cancer--from biology to clinic. Postepy Hig Med Dosw (Online) 2015;69:1275-90. [Crossref] [PubMed]
- Güth U, Huang DJ, Bauer G, et al. Metastatic patterns at autopsy in patients with ovarian carcinoma. Cancer 2007;110:1272-80. [Crossref] [PubMed]
- Köbel M, Huntsman D, Gilks CB. Critical molecular abnormalities in high-grade serous carcinoma of the ovary. Expert Rev Mol Med 2008;10:e22 [Crossref] [PubMed]
- Mittempergher L. Genomic Characterization of High-Grade Serous Ovarian Cancer: Dissecting Its Molecular Heterogeneity as a Road Towards Effective Therapeutic Strategies. Curr Oncol Rep 2016;18:44. [Crossref] [PubMed]
- Heinzelmann-Schwarz VA, Gardiner-Garden M, Henshall SM, et al. A distinct molecular profile associated with mucinous epithelial ovarian cancer. Br J Cancer 2006;94:904-13. [Crossref] [PubMed]
- Seidman JD, Horkayne-Szakaly I, Haiba M, et al. The histologic type and stage distribution of ovarian carcinomas of surface epithelial origin. Int J Gynecol Pathol 2004;23:41-4. [Crossref] [PubMed]
- Webb PM, Jordan SJ. Epidemiology of epithelial ovarian cancer. Best Pract Res Clin Obstet Gynaecol 2017;41:3-14. [Crossref] [PubMed]
- Robinson MD, Oshlack A. A scaling normalization method for differential expression analysis of RNA-seq data. Genome Biol 2010;11:R25. [Crossref] [PubMed]
- Wilson CL, Miller CJ. Simpleaffy: a BioConductor package for Affymetrix Quality Control and data analysis. Bioinformatics 2005;21:3683-5. [Crossref] [PubMed]
- Liu Z, Zhang H, Hu H, et al. A Novel Six-mRNA Signature Predicts Survival of Patients With Glioblastoma Multiforme. Front Genet 2021;12:634116 [Crossref] [PubMed]
- Subramanian A, Tamayo P, Mootha VK, et al. Gene set enrichment analysis: a knowledge-based approach for interpreting genome-wide expression profiles. Proc Natl Acad Sci U S A 2005;102:15545-50. [Crossref] [PubMed]
- Li T, Fan J, Wang B, et al. TIMER: A Web Server for Comprehensive Analysis of Tumor-Infiltrating Immune Cells. Cancer Res 2017;77:e108-e110. [Crossref] [PubMed]
- Chen B, Khodadoust MS, Liu CL, et al. Profiling Tumor Infiltrating Immune Cells with CIBERSORT. Methods Mol Biol 2018;1711:243-59. [Crossref] [PubMed]
- Ayub A, Osama M, Ahmad S. Effects of active versus passive upper extremity neural mobilization combined with mechanical traction and joint mobilization in females with cervical radiculopathy: A randomized controlled trial. J Back Musculoskelet Rehabil 2019;32:725-30. [Crossref] [PubMed]
- Wang JY, Lu AQ, Chen LJ. LncRNAs in ovarian cancer. Clin Chim Acta 2019;490:17-27. [Crossref] [PubMed]
- Du W, Hua F, Li X, et al. Loss of optineurin drives cancer immune evasion via palmitoylation-dependent IFNGR1 lysosomal sorting and degradation. Cancer Discov 2021; Epub ahead of print. [Crossref] [PubMed]
- Mohammed M, Al-Hashmi N, Al-Rashdi S, et al. Biallelic mutations in AP3D1 cause Hermansky-Pudlak syndrome type 10 associated with immunodeficiency and seizure disorder. Eur J Med Genet 2019;62:103583 [Crossref] [PubMed]
- Konakahara S, Saitou M, Hori S, et al. A neuronal transmembrane protein LRFN4 induces monocyte/macrophage migration via actin cytoskeleton reorganization. FEBS Lett 2011;585:2377-84. [Crossref] [PubMed]
- Corredor AP, Gonzalez J, Baquero LA, et al. In silico and in vitro analysis of boAP3d1 protein interaction with bovine leukaemia virus gp51. PLoS One 2018;13:e0199397 [Crossref] [PubMed]
- Zheng F, Zhai XL, Wang WJ, et al. Expression and clinical significance of LRFN4 in colorectal cancer tissue. Zhonghua Yi Xue Za Zhi 2020;100:1745-9. [PubMed]
- Paul D, Islam S, Manne RK, et al. F-box protein FBXO16 functions as a tumor suppressor by attenuating nuclear beta-catenin function. J Pathol 2019;248:266-79. [Crossref] [PubMed]
- Liu Y, Pan B, Qu W, et al. Systematic analysis of the expression and prognosis relevance of FBXO family reveals the significance of FBXO1 in human breast cancer. Cancer Cell Int 2021;21:130. [Crossref] [PubMed]
- LaFleur MW, Nguyen TH, Coxe MA, et al. PTPN2 regulates the generation of exhausted CD8(+) T cell subpopulations and restrains tumor immunity. Nat Immunol 2019;20:1335-47. [Crossref] [PubMed]
- Veenstra C, Karlsson E, Mirwani SM, et al. The effects of PTPN2 loss on cell signalling and clinical outcome in relation to breast cancer subtype. J Cancer Res Clin Oncol 2019;145:1845-56. [Crossref] [PubMed]
- Spalinger MR, Manzini R, Hering L, et al. PTPN2 Regulates Inflammasome Activation and Controls Onset of Intestinal Inflammation and Colon Cancer. Cell Rep 2018;22:1835-48. [Crossref] [PubMed]
- Zhang Z, Xu T, Qin W, et al. Upregulated PTPN2 induced by inflammatory response or oxidative stress stimulates the progression of thyroid cancer. Biochem Biophys Res Commun 2020;522:21-5. [Crossref] [PubMed]
(English Language Editor: K. Brown)