Establishment of a prognostic-related microRNAs risk model for glioma by bioinformatics analysis
Introduction
Glioma has a high incidence and carries the highest fatality rate of all brain tumors (1). The average survival time of patients with glioblastoma is only 12 to 15 months, and that of anaplastic glioma is only 2 to 5 years (2). While at present, the most common treatment for glioma is surgical resection combined with radiotherapy and chemotherapy, the long-term survival rate of patients has not significantly improved (3). The high fatality rate of glioma and the limitation of effective treatment methods has caused researchers to study the intrinsic molecular mechanism of high fatal glioma, in the effort to find a safer and more effective treatment method (4-6).
MicroRNAs (miRNAs) are a class of non-coding single-stranded small RNA with a length of about 18–26 nucleotide (7,8). Recent studies have shown that the abnormal expression of microRNAs is closely related to the formation of glioma, rendering them a potential new marker and therapeutic target for the diagnosis and treatment of glioma (9,10).
The Cancer Genome Atlas (TCGA) database contains a large amount of multi-group data such as patient detailed clinical data, genome mutation data, transcriptome data, microRNAs sequencing data, and DNA methylation data (11,12). Third level data (level 3) after processing has been opened to the public free of charge (https://portal.gdc.cancer.gov/) and provides a convenient platform for data mining by cancer researchers. Nowadays, mathematical statistics modeling is one of the most effective means to mine large biological data, and Least Absolute Shrinkage and Selection Operator (LASSO) is a compression estimation model proposed by Tibshirani in 1996 (13). The basic function of LASSO is to minimize the sum of squares of residuals under the condition that the absolute sum of regression coefficients is less than a constant, so that some regression coefficients which are strictly equal to zero can be generated, some variables with lower weights can be removed, and the dimensionality of data can be reduced. In this way, the model is more refined than others.
Based on the large sample of glioma microRNAs-Seq data in the TCGA public database and the clinical information of patients, this study used the improved LASSO & COX algorithm to screen differentially expressed microRNAs in glioma and analyze the prognostic correlation of glioma patients. The purpose of this study was to lay a foundation for predicting the prognosis of glioma patients by exploring the specific microRNAs related to its prognosis and the potential molecular mechanism of its occurrence
We present the following article in accordance with the TRIPOD reporting checklist (available at https://dx.doi.org/10.21037/atm-21-2402).
Methods
Data acquisition
The microRNA-Seq data and clinical information of 535 glioma patients (510 cases from TCGA database and 25 cases from GSE112009) were confirmed and downloaded as the study subjects (deadline March 20, 2018). The 535 glioma specimens were collected from several cancer centers in the United States according to strict standards, and the clinical information of patients was recorded and followed up in detail. Basic clinical information in the database included age, sex, race, smoking history, survival status, and survival time. The data used in this study meets the requirements of TCGA official publication data and are publicly available. The study was conducted in accordance with the Declaration of Helsinki (as revised in 2013).
Data processing
The microRNA data of glioma in TCGA and GSE112009 in GEO were downloaded, and the expression data of microRNA in a single sample were then merged.
Random grouping
The 535 glioma patients were randomly divided into three groups; a training set (255 cases), prediction set (141 cases), and verification set (139 cases). The training set was used to learn sample characteristics and an estimation model, and the prediction set and verification set were used as an internal verification queue to verify the reliability of the model.
Marker screening of training sets
We used the “glmnet” package of R software (version 3.3.1) to carry out LASSO Cox regression model analysis and predicted the most relevant marker according to 255 samples of the training set. We used the random sampling method of leave one out cross validation to select microRNA to build the prognostic model.
Risk assessment model
A risk sore evaluation formula was constructed to evaluate the risk of the samples according to seven markers. The risk score formula was in which ωi and xi were the coefficients of each gene and the expression value of each gene respectively. The prognostic risk value of each patient was calculated, with the median of the risk value allocated as the optimal cutoff value, which allowed patients to be divided into high-risk and low-risk groups.
Survival rate analysis
The survival curve was drawn by the survival of R language package and the Kaplan-Meier survival curve method was used to evaluate the overall survival rate of high-risk and low-risk groups. Log-rank test was used to determine the difference between groups and the difference was statistically significant (P<0.05). Survival analysis was then performed in the training set, prediction set, validation set, and high-grade gliomas.
Fisher exact test
Fisher exact test was used to determine whether different clinical information (age, gender, history neoadjuvant treatment, and Karnofsky score) were associated with high-risk and low-risk groups.
Prediction and enrichment analysis of marker microRNAs
Using the mirwalk 2.0 database, the target genes of microRNA were predicted in five databases (miRWalk, miRanda, miRDB, RNA22, and Targetscan), and those obtained in all five were obtained and mapped by Cyscape 3.3.0.
The online website DAVID (https://david.ncifcrf.gov/) was used for gene ontology (GO) analysis and Kyoto Encyclopedia of Genes and Genomes (KEGG) analysis to determine the biological functions or pathways of the main effects of the different genes.
Statistical analysis
All values were presented as the mean ± SD. The significance of data differences was tested using the pairwise comparisons of variables. Correlation was assessed by linear regression analysis. A P value <0.05 was taken to indicate statistical significance.
Results
Marker screening results of training sets
We used statistical methods to construct prognostic models based on seven survival-related microRNAs from 448 microRNAs of 255 samples in the training set. The regression coefficients of two microRNAs (hsa-miR-148a-3p, cof:0.040144; hsa-miR-222-3p, cof:0.032829) were more than 0, which was negatively correlated with the survival time of patients. The regression coefficients of the other five microRNAs (hsa-miR-140-5p, cof:-0.04085; hsa-miR-145-5p, cof:-0.00363; hsa-miR-183-5p, cof:-0.01031; hsa-miR-223-3p, cof:-0.01458; and hsa-miR-374a-5p, cof:-0.00684) were less than 0, which was positively correlated with the survival time of patients (Figure 1).
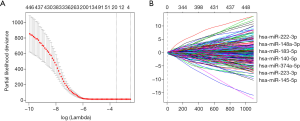
Prognostic risk scoring model
A prognostic risk scoring model based on seven microRNAs was constructed in the training set. We extracted the coefficients of multivariate COX analysis of microRNAs from the LASSO & COX regression models and constructed a prognostic risk scoring model consisting of the seven microRNAs. According to the risk scoring formula, 255 patients in the training set were divided into a high-risk group (n=210) and low-risk group (n=45), and Kaplan-Meier survival curve analysis showed that the overall survival rate of the high-risk group was lower than that of the low-risk group, and the difference between the two groups was significant (P<0.0001) (Figure 2A).
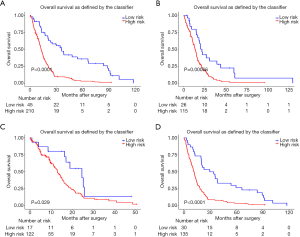
Verify the risk scoring model in two queues of the prediction set and verification set
To verify the accuracy and stability of the risk assessment model, the same threshold of the training set was used to distinguish the high-risk group from the low-risk group of the prediction set (n=141) and verification set (n=139). The survival curve clearly showed that patients at high risk in the predictive set (P=0.00066) (Figure 2B) and verification set (P=0.029) (Figure 2C) had significantly shorter survival times than patients at low risk.
Survival analysis of high-grade glioblastoma
The same threshold of training set was used to distinguish the high-risk group from the low-risk group of high-grade glioblastoma and the survival curve clearly showed that the survival time of high-risk patients in the high-grade glioblastoma group was significantly shorter than that of low-risk patients (P<0.001) (Figure 2D).
Fisher exact test
The Fisher exact test in the training set showed the difference between the high-risk and low-risk groups in age (P=1.2E-06) and Karnofsky score (P=0.007729) both effected the risk of glioma (Table 1).
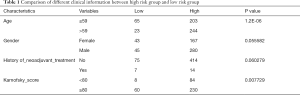
Full table
Analysis of target gene results of seven marker microRNA
Using the mirwalk 2.0 database, 610 target genes of microRNA were obtained in five databases and through Cytoscape 3.3.0, we mapped the relationship between microRNA and the 610 target genes (Figure 3).
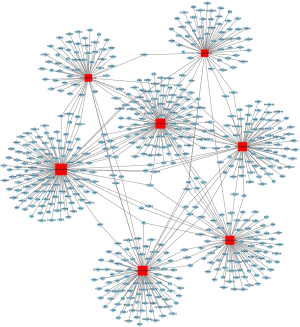
The results of GO functional enrichment analysis showed that the genes with significant differences were related to the positive regulation of cell migration, neuronal migration, and the response to transforming growth factor (Figure 4A).
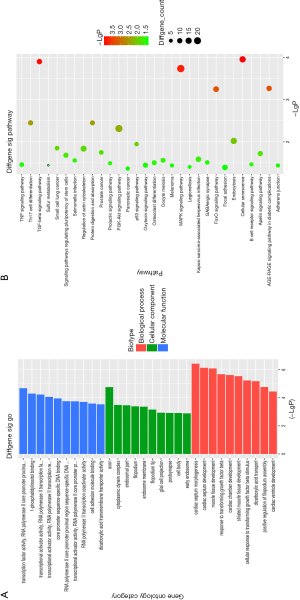
Enrichment analysis of the KEEG pathway showed that target genes were mainly related to the TGF-beta signaling pathway, MAPK signaling pathway, AGE-RAGE signaling pathway in diabetic complications, and other pathways (Figure 4B).
Discussion
Glioma has a high incidence, high mortality, and limited effective treatment methods (14). The current protocolized treatment for high grade glioma is surgery followed by radiotherapy and temozolomide chemotherapy, recently bevacizumab and TTFields are widespread used. This study explored the molecular mechanism of glioma by bioinformatics with the hope that the related microRNA found in this study could become a new marker and therapeutic target for its diagnosis and treatment.
The relationship between the prognosis of microRNA expression profiles based on the TCGA database and glioma has been extensively studied in recent years (9,10). In the current study, the downloaded sequencing data of microRNAs were based on the microRNA database to re-annotate the mature microRNAs expression profile data and use it for further prognostic analysis, which makes research of microRNAs more accurate. In addition, we used the LASSO penalty regression algorithm to screen the variables of the microRNAs expression profile data. Compared with conventional COX regression analysis, the LASSO penalty regression algorithm is more suitable for prognosis analysis of high throughput data, by selecting very few valid variables from large amounts of data and avoiding collinearity among variables.
Our study provides an approach for the classifications of gliomas using a large sample size and advanced statistical approaches. Previous studies that have assessed miRNA biomarkers in glioma have been limited by small sample sizes, small numbers of miRNAs screened, a lack of independent validation sets, and the use of inappropriate statistical methods to miRNA microarray data. The use of the LASSO Cox regression model has allowed us to integrate multiple miRNAs into one tool with a significantly greater prognostic accuracy than that of single miRNAs alone (10).
By using bioinformatics, the microRNA-Seq data and clinical information of 535 glioma patients (510 cases from TCGA database and 25 cases from GSE112009) were confirmed and downloaded. We used statistical methods to construct prognostic models based on seven survival-related microRNAs from 448 microRNAs of 255 samples in the training set. We then established a seven-miRNA prognostic risk scoring model that could improve the predictability of disease prognosis. The reliability and accuracy of this novel prognostic tool were validated in two queues of prediction and verification sets, and our results showed that this tool can successfully stratify GBM patients into high- and low-risk categories. Additionally, the tool could more accurately predict patient survival, suggesting that the seven-miRNA based classifier provides prognostic value.
Previous studies have identified multiple miRNAs that are differentially regulated in glioma compared with normal brain tissue around tumors. While each of the miRNAs has previously been shown to be associated with therapeutic or prognosis outcomes in patients with glioma (10,15,16), we discovered seven-related miRNA using bioinformatics, which is a novel finding. Furthermore, we analyzed high-grade glioblastoma with a risk assessment model and found that the prognosis of the high-risk group was significantly worse than that of the low-risk group, which indicated that glioblastoma was highly correlated with the prognosis of these markers.
In this study, we not only used databases to screen out seven microRNAs associated with the overall survival of glioma patients, the target genes of the seven microRNAs were predicted and analyzed by GO and KEGG.
The results of GO functional enrichment analysis showed the genes with significant differences were related to the positive regulation of cell migration, neuronal migration, and the response to transforming growth factor (TGF) (Figure 4A). Further, enrichment analysis of the KEEG pathway showed that target genes were mainly related to the TGF-beta signaling pathway, MAPK signaling pathway, AGE-RAGE signaling pathway in diabetic complications, and other pathways (Figure 4B). Through GO functional enrichment analysis, we found that the screened microRNAs are related to the function of TGF, and KEEG analysis further confirmed that glioma is related to the TGF signaling pathway. This indicates that TGF is not only a hot topic in cancer research but also could be a target for glioma therapy. Numerous studies have shown that cell migration and neuronal migration are associated with local infiltration, distant metastasis, and the recurrence of glioma (17,18), and the MAPK signaling pathway is a key pathway of cellular proliferation and apoptosis (19). All related functions and signaling pathways indicate that these changes are related to glioma cell proliferation, migration, and apoptosis.
In summary, seven microRNAs are significantly associated with the prognosis of glioma patients. The risk model based on the seven microRNAs can well divide glioma patients into high-risk group and low-risk groups with poor prognosis and can predict the prognosis of patients independently of other clinical variables, which provides a basis for the prognosis prediction and personalized treatment of glioma patients.
Conclusions
Seven miRNAs were identified as biomarkers of glioma by regulating target genes to participate in related signaling pathways and leading to the poor prognosis of patients.
Acknowledgments
Funding: None.
Footnote
Reporting Checklist: The authors have completed the Tripod reporting checklist. Available at https://dx.doi.org/10.21037/atm-21-2402
Conflicts of Interest: All authors have completed the ICMJE uniform disclosure form (available at https://dx.doi.org/10.21037/atm-21-2402). The authors have no conflicts of interest to declare.
Ethical Statement: The authors are accountable for all aspects of the work in ensuring that questions related to the accuracy or integrity of any part of the work are appropriately investigated and resolved. The study was conducted in accordance with the Declaration of Helsinki (as revised in 2013).
Open Access Statement: This is an Open Access article distributed in accordance with the Creative Commons Attribution-NonCommercial-NoDerivs 4.0 International License (CC BY-NC-ND 4.0), which permits the non-commercial replication and distribution of the article with the strict proviso that no changes or edits are made and the original work is properly cited (including links to both the formal publication through the relevant DOI and the license). See: https://creativecommons.org/licenses/by-nc-nd/4.0/.
References
- Hua L, Wang Z, Zhao L, et al. Hypoxia-responsive lipid-poly-(hypoxic radiosensitized polyprodrug) nanoparticles for glioma chemo- and radiotherapy. Theranostics 2018;8:5088-105. [Crossref] [PubMed]
- Mair R, Mouliere F, Smith CG, et al. Measurement of Plasma Cell-Free Mitochondrial Tumor DNA Improves Detection of Glioblastoma in Patient-Derived Orthotopic Xenograft Models. Cancer Res 2019;79:220-30. [Crossref] [PubMed]
- Zachariah MA, Oliveira-Costa JP, Carter BS, et al. Blood-based biomarkers for the diagnosis and monitoring of gliomas. Neuro Oncol 2018;20:1155-61. [Crossref] [PubMed]
- Philip B, Yu DX, Silvis MR, et al. Mutant IDH1 Promotes Glioma Formation In Vivo. Cell Rep 2018;23:1553-64. [Crossref] [PubMed]
- Aderetti DA, Hira VVV, Molenaar RJ, et al. The hypoxic peri-arteriolar glioma stem cell niche, an integrated concept of five types of niches in human glioblastoma. Biochim Biophys Acta Rev Cancer 2018;1869:346-54. [Crossref] [PubMed]
- Lu J, Lu Y, Wang X, et al. Prevalence, awareness, treatment, and control of hypertension in China: data from 1·7 million adults in a population-based screening study (China PEACE Million Persons Project). Lancet 2017;390:2549-58. [Crossref] [PubMed]
- Cha W, Fan R, Miao Y, et al. MicroRNAs as novel endogenous targets for regulation and therapeutic treatments. Medchemcomm 2018;9:396-408. [Crossref] [PubMed]
- Mishra S, Yadav T, Rani V. Exploring miRNA based approaches in cancer diagnostics and therapeutics. Crit Rev Oncol Hematol 2016;98:12-23. [Crossref] [PubMed]
- Barbano R, Palumbo O, Pasculli B, et al. A miRNA signature for defining aggressive phenotype and prognosis in gliomas. PLoS One 2014;9:e108950 [Crossref] [PubMed]
- Guan Y, Chen L, Bao Y, et al. Identification of low miR-105 expression as a novel poor prognostic predictor for human glioma. Int J Clin Exp Med 2015;8:10855-64. [PubMed]
- Zheng MJ, Gou R, Zhang WC, et al. Screening of prognostic biomarkers for endometrial carcinoma based on a ceRNA network. PeerJ 2018;6:e6091 [Crossref] [PubMed]
- Tomczak K, Czerwińska P, Wiznerowicz M. The Cancer Genome Atlas (TCGA): an immeasurable source of knowledge. Contemp Oncol (Pozn) 2015;19:A68-77. [Crossref] [PubMed]
- Zhou L, Tang L, Song AT, et al. A LASSO Method to Identify Protein Signature Predicting Post-transplant Renal Graft Survival. Stat Biosci 2017;9:431-52. [Crossref] [PubMed]
- Cancer Genome Atlas Research Network. Comprehensive genomic characterization defines human glioblastoma genes and core pathways. Nature 2008;455:1061-8. [Crossref] [PubMed]
- Pang C, Guan Y, Zhao K, et al. Up-regulation of microRNA-15b correlates with unfavorable prognosis and malignant progression of human glioma. Int J Clin Exp Pathol 2015;8:4943-52. [PubMed]
- Fowler A, Thomson D, Giles K, et al. miR-124a is frequently down-regulated in glioblastoma and is involved in migration and invasion. Eur J Cancer 2011;47:953-63. [Crossref] [PubMed]
- Shou J, Gu S, Gu W. Identification of dysregulated miRNAs and their regulatory signature in glioma patients using the partial least squares method. Exp Ther Med 2015;9:167-71. [Crossref] [PubMed]
- Li Z, Liu H, Zhong Q, et al. LncRNA UCA1 is necessary for TGF-β-induced epithelial-mesenchymal transition and stemness via acting as a ceRNA for Slug in glioma cells. FEBS Open Bio 2018;8:1855-65. [Crossref] [PubMed]
- Tsai YF, Chen YF, Hsiao CY, et al. Caspase-dependent apoptotic death by gadolinium chloride (GdCl3) via reactive oxygen species production and MAPK signaling in rat C6 glioma cells. Oncol Rep 2019;41:1324-32. [PubMed]
(English Language Editor: B. Draper)