The promises and challenges of pragmatism: lesson from of a recent clinical trial
Pragmatic trials aim to answer the question “Can the intervention work under usual conditions?”, whereas explanatory trials aim to answer “Can the intervention work under ideal conditions?” (1). Pragmatic trials, undertaken in routine practice settings, may provide more relevant evidence to support decision making for most stakeholders, such as patients, clinicians, health systems, payers, and policymakers. Furthermore, pragmatic trials leverage existing clinical infrastructure and can be implemented at a fraction of the cost of typical explanatory trials. As such, one might expect that pragmatic trials should be conducted more frequently. The reality is quite the opposite. The number of explanatory trials substantially outweigh that of pragmatic trials, although the level of pragmatism increased moderately over the past two decades (2-5).
There are few purely explanatory or pragmatic trials (6). The pragmatism is a continuum assessed by the PRECIS-2 tool based on 9 dimensions. Each dimension can be rated from 1 (very explanatory) to 5 (very pragmatic). The PRECIS-2 tool is intended to be used at the trial design stage to help trialists make the purpose of the trial explicit and to ensure that the design choices are concordant with their intended purpose. In this letter, we rated a pivotal trial recently completed by our team to illustrate how the design choices we made affect the pragmatism.
The example used here is electrocardiogram (ECG) artificial intelligence (AI)-Guided Screening for Low Ejection Fraction (EAGLE) trial, which is a pragmatic randomized controlled trial (RCT) that assessed an AI-powered clinical decision support tool for enabling early diagnosis of low ejection (Figure 1) (7-9).
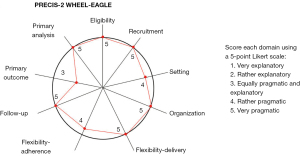
The first dimension of pragmatism is “eligibility” aiming to assess “To what extent are trial participants similar to those who would receive this intervention if it was part of usual care?” EAGLE has few inclusion/exclusion criteria, and thus, is very pragmatic.
The second dimension, “recruitment”, evaluates “How much extra effort is made to recruit participants over and above what would be used in the usual care setting to engage patients?”. A highly pragmatic approach would be to recruit through usual appointments at diverse clinics and hospitals. EAGLE included 22,641 patients seen as part of routine practice at Mayo Clinic over 8 months, and thus, is very pragmatic.
The third dimension, “setting”, assesses “How different are the settings of the trial from the usual care setting?”. EAGLE was conducted at 45 primary care clinics in Minnesota and Wisconsin, and thus, is rather pragmatic. However, it is not a national or international trial that includes patients from different geographic areas, health systems, or countries.
The fourth dimension, “organization”, addresses “What expertise and resources are needed to deliver the intervention?”. A highly pragmatic design would make use of no more than the existing staff and resources in routine practice. EAGLE embedded AI into the routine workflow, and thus, is very pragmatic.
The fifth dimension is flexibility in terms of the delivery of the intervention. The most pragmatic design would leave the details of how to implement the intervention up to providers, i.e., how to deliver an intervention is not rigidly prescriptive in the protocol. For example, the details of how to perform a surgical procedure could be left to the surgeons. EAGLE provided a frequently asked questions (FAQ) to clinicians and a patient brochure; however, what to do next was entirely up to the clinicians and patients. Therefore, EAGLE is very pragmatic in this domain.
The sixth dimension is flexibility in terms of adherence. A highly pragmatic design would allow for full flexibility in how the end-users engage with the intervention. EAGLE used reminders sent to clinicians to encourage compliance, but it didn’t force compliance, and thus, is rather pragmatic.
The seventh dimension is follow-up. The most pragmatic approach would be to have no more follow-up than routine practice. In EAGLE, all the baseline characteristics and clinical outcomes were collected from electronic health records (EHR), using either structured data (e.g., billing codes and lab tests) or unstructured data (e.g., clinical notes) abstracted via natural language processing (NLP). Therefore, the trial is very pragmatic.
The eighth dimension, “primary outcome”, measures “How relevant is the outcome to participants?”. The most pragmatic approach would be to select an outcome that is obviously important for patients. Surrogate outcomes, composite outcomes are less pragmatic. Also, if outcome adjudication requires special training or tests not used in routine practice, this outcome is less pragmatic. In EAGLE, the primary outcome was low ejection fraction within 90 days of the ECG. This outcome is important, but mostly to clinicians for treatment planning and monitoring purposes. For patients, other outcomes, such as heart failure hospitalization or mortality, might be more relevant. However, this outcome was chosen partly because mortality and hospitalization require a long follow-up period to observe an effect. Therefore, the outcome is equally pragmatic and explanatory.
The last dimension is primary analysis. A pragmatic approach would be an intention-to-treat analysis using all the available data. Per-protocol analysis and as-treated analysis are less pragmatic; excluding data due to non-compliance or low volume of recruitment is also less pragmatic. EAGLE conducted an intent-to-treat analysis using all data, and thus, is very pragmatic.
As illustrated in this example, the reasons why pragmatic trials are less common are obvious: they may not give the intervention its best chance to demonstrate a beneficial effect, and they require a large cohort of diverse patient populations from a broad range of routine practice settings, which is resource-intensive. To address the challenges, innovative methods that enable trials to be conducted at a faster pace and lower cost are required. This will require a refocusing of the goals of pragmatism towards leveraging existing clinical infrastructure to achieve better efficiency. For example, a recent trial used AI to identify eligible patients from EHR, automatically send study invites via existing EHR/clinical workflows (e.g., patient portal or postal mail), direct patients to digital resources to confirm eligibility and view study informational videos, consent electronically, and follow-up virtually (10). Such digital methods embedded into routine practice workflow can greatly reduce the cost and time, thereby enabling the conduct of more pragmatic trials. However, whether such approaches can be used broadly and how the digital trials affect the participation from certain populations, e.g., rural patients, minorities, and those with limited digital literacy, remain to be seen.
Acknowledgments
Funding: None.
Footnote
Provenance and Peer Review: This article was commissioned by the Guest Editors [Yanhong Deng, Qian Shi and Jun (Vivien) Yin] for the series “Challenges in Clinical Trials” published in Annals of Translational Medicine. The article has undergone external peer review.
Conflicts of Interest: Both authors have completed the ICMJE uniform disclosure form (available at https://atm.amegroups.com/article/view/10.21037/atm-21-5424/coif). The series “Challenges in Clinical Trials” was commissioned by the editorial office without any funding or sponsorship. The authors have no other conflicts of interest to declare.
Ethical Statement: The authors are accountable for all aspects of the work in ensuring that questions related to the accuracy or integrity of any part of the work are appropriately investigated and resolved.
Open Access Statement: This is an Open Access article distributed in accordance with the Creative Commons Attribution-NonCommercial-NoDerivs 4.0 International License (CC BY-NC-ND 4.0), which permits the non-commercial replication and distribution of the article with the strict proviso that no changes or edits are made and the original work is properly cited (including links to both the formal publication through the relevant DOI and the license). See: https://creativecommons.org/licenses/by-nc-nd/4.0/.
References
- Ford I, Norrie J. Pragmatic Trials. N Engl J Med 2016;375:454-63. [Crossref] [PubMed]
- Sepehrvand N, Alemayehu W, Das D, et al. Trends in the Explanatory or Pragmatic Nature of Cardiovascular Clinical Trials Over 2 Decades. JAMA Cardiol 2019;4:1122-8. [Crossref] [PubMed]
- Weinfurt KP, Hernandez AF, Coronado GD, et al. Pragmatic clinical trials embedded in healthcare systems: generalizable lessons from the NIH Collaboratory. BMC Med Res Methodol 2017;17:144. [Crossref] [PubMed]
- Ali J, Antonelli M, Bastian L, et al. Optimizing the Impact of Pragmatic Clinical Trials for Veteran and Military Populations: Lessons From the Pain Management Collaboratory. Mil Med 2022;187:179-85. [Crossref] [PubMed]
- Zuidgeest MGP, Goetz I, Groenwold RHH, et al. Series: Pragmatic trials and real world evidence: Paper 1. Introduction. J Clin Epidemiol 2017;88:7-13. [Crossref] [PubMed]
- Loudon K, Treweek S, Sullivan F, et al. The PRECIS-2 tool: designing trials that are fit for purpose. BMJ 2015;350:h2147. [Crossref] [PubMed]
- Yao X, McCoy RG, Friedman PA, et al. ECG AI-Guided Screening for Low Ejection Fraction (EAGLE): Rationale and design of a pragmatic cluster randomized trial. Am Heart J 2020;219:31-6. [Crossref] [PubMed]
- Yao X, McCoy RG, Friedman PA, et al. Clinical trial design data for electrocardiogram artificial intelligence-guided screening for low ejection fraction (EAGLE). Data Brief 2020;28:104894. [Crossref] [PubMed]
- Yao X, Rushlow DR, Inselman JW, et al. Artificial intelligence-enabled electrocardiograms for identification of patients with low ejection fraction: a pragmatic, randomized clinical trial. Nat Med 2021;27:815-9. [Crossref] [PubMed]
- Yao X, Attia ZI, Behnken EM, et al. Batch enrollment for an artificial intelligence-guided intervention to lower neurologic events in patients with undiagnosed atrial fibrillation: rationale and design of a digital clinical trial. Am Heart J 2021;239:73-9. [Crossref] [PubMed]