Metabonomic analysis of cerebrospinal fluid in epilepsy
Introduction
Pathogenesis is complex and involves many factors (1). At present, the key factors leading to seizures are not apparent (2,3). Epilepsy is an uncontrollable and unpredictable condition (4). Due to the lack of accurate and effective biomarkers, the misdiagnosis rate of epilepsy is high, and many patients are still unable to get effective treatment (5,6). More than 20 kinds of antiepileptic drugs are used in clinical settings; however, drug treatments are still ineffective in about 1/3 of epileptic patients (7). Thus, it is crucial that new key pathogenic factors and therapeutic targets for epilepsy be identified. No specific biological markers of epilepsy have been found due to the pathological and clinical heterogeneity of epilepsy. It is of great significance to find specific therapeutic targets. A previous study has suggested that a group of molecular markers, rather than a single molecular marker, may be needed to characterize the clinical typing of epilepsy, construct the pathogenic mechanism network or serve as a therapeutic target (8).
Few studies have looked for epilepsy markers in brain tissue because the opportunity to obtain brain tissue of patients with epilepsy is rare and challenging to operate. A more direct method is to look for markers from cerebrospinal fluid samples (9). Therefore, metabonomics has significant advantages over genomics since the research object of metabonomics is small molecular metabolites that can enter body fluid freely. Moreover, metabonomics can quantitatively analyze all metabolites in organisms, thus potentially investigating the relationship between metabolites and physiological and pathological changes. Cerebrospinal fluid is the preferred biological sample for neuropathological research. The abnormal metabolic components of cerebrospinal fluid can directly reflect the pathophysiological changes of the brain.
During seizures, various metabolites and metabolic pathways become dysregulated, such as changes in the abundance of essential products in the three acid cycles, energy metabolism, amino acid metabolism, phospholipid metabolism and purine metabolic pathways (9,10). At present, studies have found different metabolites in the cerebrospinal fluid of patients with epilepsy caused by traumatic brain injury, including glutamate, norepinephrine, homovanillic acid, hydroxyindole (8,11). A metabonomics study had pointed out that the amino acid metabolic pathway plays a leading role in the occurrence and development of epilepsy (11). A study had also suggested that the pathogenesis of epilepsy may be related to citrate and the intermediates of the citric acid cycle pathway (12).
It should be emphasized that most previous studies have been limited to the effects of particular metabolites on epilepsy. This study screened the differentially expressed metabolites in cerebrospinal fluid of patients with epilepsy and healthy controls by high-throughput sequencing, explored the correlation between the differentially expressed metabolites, and enriched the metabolic pathway of the differentially expressed metabolites. This study comprehensively expounds on the relationship between epilepsy and cerebrospinal fluid metabolomic changes. By combining differential metabolites, this study provides a basis for the clinical classification of epilepsy and the network research of epilepsy pathogenesis to screen the biomarkers of epilepsy diagnosis, treatment, and prognosis. We present the following article in accordance with the MDAR reporting checklist (available at https://atm.amegroups.com/article/view/10.21037/atm-22-1219/rc).
Methods
Research objects
In this study, 23 epileptic patients treated at The First Affiliated Hospital of Dalian Medical University from April 2019 to September 2019 were allocated to the disease group and 13 non-epileptic patients were allocated to the control group. To be eligible for inclusion in the study, epileptic patients in the disease group had to meet the epilepsy diagnostic criteria of the international anti-epilepsy Alliance [2014], while non-epileptic patients in the control group had to have no history of seizures and no family history of epilepsy. Patients were excluded from the study if they met any of the following exclusion criteria (which were the same for both the disease and control groups): (I) had a metabolic disease; (II) had definite brain organic lesions; (III) had an abnormal quantification of leukocytes or proteins in the cerebrospinal fluid; and/or (IV) had participated in other clinical studies. There were 14 males and 9 females in the epilepsy group, with an average age of 49.87±18.98 years. There were 7 males and 6 females in the control group, with an average age of 56.15±19.58 years. No significant difference in age and gender was observed between the epilepsy and control group (P>0.05). The study was conducted in accordance with the Declaration of Helsinki (as revised in 2013). The study was approved by the Ethics Committee of The First Affiliated Hospital of Dalian Medical University (No. PJ-KS-KY-2020-18), and informed consent was provided by all the patients.
Research methods
Cerebrospinal fluid retention
In this study, a lumbar puncture was performed on each participant, and 2 mL of cerebrospinal fluid was collected in a sterile test tube. The tube containing the cerebrospinal fluid was sealed immediately after it was taken, and stored in a −80 ℃ refrigerator awaiting analysis. A total of 36 cerebrospinal fluid samples were collected. After routine examinations of the cerebrospinal fluid, the quality of the cerebrospinal fluid samples was confirmed, and all the samples were retained.
Extraction and analysis of metabolites
The 36 cerebrospinal fluid samples were derivatized to reduce sample polarity and improve volatility and thermal stability. The metabolites in the cerebrospinal fluid samples were analyzed by gas chromatography-mass spectrometry (GC-MS). The peak signals of the cerebrospinal fluid samples were collected and standardized. Metabolite information was obtained after alignment database.
Data analysis
One-dimensional difference analysis
The fold change was calculated as follows: metabolite expression in the epilepsy sample/metabolite expression in the normal sample. The differences between the two groups were compared and analyzed by t-tests. A P value <0.05 was set as the screening standard.
Multi-dimensional difference analysis
An orthogonal partial least squares discriminant analysis (OPLS-DA) eliminates any noise information irrelevant to the classification and obtains the relevant metabolite information, and any significant difference between two groups are found by filtering out the signals irrelevant to the model classification, including the orthogonal signals. The quality of the model was examined. After establishing the model, the variable importance in the projection (VIP) value of the sample was calculated, and a VIP value >1 was set as the screening standard. The fold difference calculation was the same as the 1-dimensional analysis.
Correlation analysis of differential metabolites
The Spearman correlation analysis method was used to analyze differences in the metabolite data between the groups.
Metabolic pathway enrichment analysis
First, the differential metabolites were mapped to authoritative metabolite databases, such as Kyoto Encyclopedia of Genes and Genomes (KEGG), The Small Molecule Pathway Database (SMPDB), and PubChem. The metabolic pathway analysis was carried out using the pathway database of the species according to the matching information.
Statistical analysis
SPSS 24.0 software was used for the statistical analysis of the data. A P value <0.05 was considered statistically significant.
Results
One-dimensional test difference analysis
Compared to the cerebrospinal fluid of the 13 non-epileptic patients, 3 metabolites were differentially expressed in the cerebrospinal fluid of the 23 epileptic patients; that is, alpha-ketoisocaproic acid 1, xylose 1, and glycine 2 (P<0.05). Alpha-ketoisocaproic acid 1 and xylose 1 were highly expressed in the epileptic cerebrospinal fluid samples, and glycine 2 was lowly expressed in the epileptic cerebrospinal fluid samples. A heat map of the differentially expressed metabolites is shown in Figure 1.

Multi-dimensional test difference analysis
A VIP value calculated by OPLS-DA was used to identify the differential metabolites. Compared to the cerebrospinal fluid of the 13 non-epileptic patients, 56 metabolites were differentially expressed in the cerebrospinal fluid samples of the 23 epileptic patients, including 36 upregulated metabolites and 20 downregulated metabolites (all with a VIP >1). The heat map of the differentially expressed metabolites is shown in Figure 2. The correlations between the 56 metabolites are shown in Figure 3.
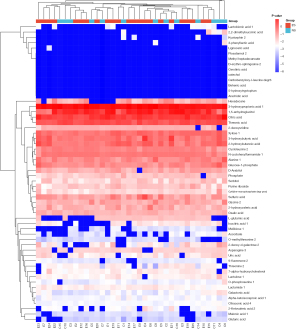
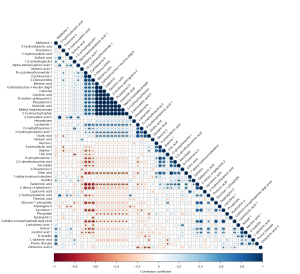
One-dimensional and multi-dimensional difference cross analyses
The 1-dimensional and multi-dimensional differentially expressed metabolites were cross analyzed using a VIP value >1 and a P value <0.05 as the screening conditions. The cross overlap of the 1-dimensional and multi-dimensional differentially expressed metabolites is shown in Figure 4. The two differentially expressed metabolites all met the screening conditions (i.e., each had a VIP value >1 and a P value <0.05). A differential expression diagram is shown in Figure 5. A box diagram is shown in Figure 6. Alpha-ketoisocaproic acid 1 and xylose 1 were highly expressed in the epileptic cerebrospinal fluid samples, and glycine 2 was lowly expressed in the epileptic cerebrospinal fluid samples.
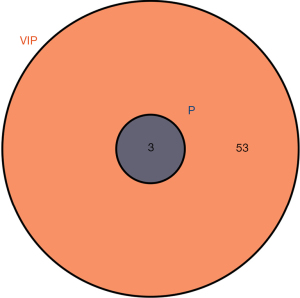
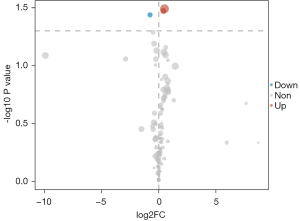
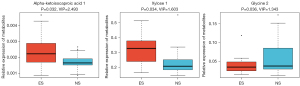
Enrichment analysis of the differential metabolite metabolic pathways
The 3 differential metabolites were significantly enriched in the 5 metabolic pathways of primary bile acid biosynthesis, valine, leucine, and isoleucine degradation, glutathione metabolism, glyoxylate and dicarboxylate metabolism, and glycine, serine, and threonine metabolism (see Figure 7).
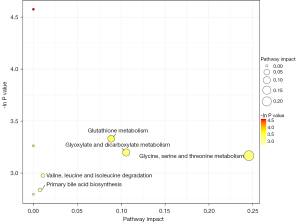
Discussion
A body-fluid composition analysis is an indispensable indicator in disease diagnosis and pathogenesis exploration. To date, little research has been conducted on the application of cerebrospinal fluid in modern medicine. Cerebrospinal fluid performs many critical physiological functions in the brain and spinal cord, including the exchange of metabolites between blood and the brain (13). Compounds with different structures can penetrate the blood-brain barrier from the blood, enter the cerebrospinal fluid, and then enter the brain cells, thus affecting the function of the central nervous system.
The abnormal expression of metabolites in cerebrospinal fluid is closely related to epilepsy. Thus, abnormally expressed metabolites in cerebrospinal fluid are potential biomarkers of epileptic brain injury, and have good diagnostic efficacy or may become effective therapeutic targets. A previous study has confirmed that compared to the non-epileptic patients, the Zin-α2-glycoprotein (ZAG) and total tau (T-Tau) in the cerebrospinal fluid of patients with temporal-lobe epilepsy was decreased, but no significant difference in (tau phosphorylated at threonine 181) P-Tau 181 was found among the patients. The P-Tau 181/T-Tau ratio in patients with temporal-lobe epilepsy was significantly higher than that of patients in the control group. A Spearman correlation analysis showed that T-Tau and the P-Tau 181/T-Tau ratio were positively correlated with the white matter injury score (P<0.01). Cerebrospinal fluid ZAG, T-Tau, and the P-Tau 181/T-Tau ratio may be potential biomarkers of brain injury in temporal-lobe epilepsy, which may also be involved in the potential mechanism of brain injury in temporal-lobe epilepsy (14).
With the rise of GC-MS technology, cerebrospinal fluid metabolomics has been extensively researched. We used GC-MS to analyze the cerebrospinal fluid of epileptic and non-epileptic patients. The differentially expressed metabolites in the cerebrospinal fluid samples of the epileptic and non-epileptic patients were screened using a VIP value of >1 and a P value <0.05. In this study, 3 differentially expressed metabolites were identified; that is, alpha-ketoisocaproic acid 1, xylose 1, and glycine 2.
Among the 3 differentially expressed metabolic species, we found that the relationship between alpha-ketoisocaproic acid 1 and xylose 1 and neurological diseases was unclear. We reviewed many previous studies and only found evidence that xylose 1 may cause convulsions by affecting sugar transport in the brain (15-17). As a classical inhibitory neurotransmitter, glycine has an apparent pathogenic effect on epilepsy (18). Abnormal glycine metabolism or a transport disorder may be related to the pathogenesis and cognitive impairment of patients with epilepsy. Glycine receptor blockers have been shown to reduce epileptic symptoms in patients (19). These results suggest that an increase of glycine in the cerebrospinal fluid may be an essential link in the pathogenesis of epilepsy, which is consistent with our results. Glycine and aspartic acid are both natural ligands of the N-methyl-D-aspartate (NMDA) receptor. Aspartic acid is the endogenous agonist of the NMDA receptor, and glycine is its necessary synergistic agonist. The dynamic balance regulation mechanism between them guarantees the normal function of the NMDA receptor in the physiological state. The disorder of its regulation mechanism is a leading cause of epilepsy. Alpha-ketoisocaproic acid 1, xylose 1 and glycine 2 are differentially expressed in cerebrospinal fluid of patients with epilepsy and are related to convulsive symptoms, seizures and cognitive impairment. Reverse regulation of these metabolites may reduce the clinical symptoms of patients with epilepsy. These metabolites are potential therapeutic targets.
This study screened the metabolic pathways related to epilepsy based on differential metabolite enrichment. The metabolic pathways are primary bile acid biosynthesis, valine, leucine, and isoleucine degradation, glutathione metabolism, glyoxylic acid and dicarboxylic acid metabolism, and glycine, serine, and threonine metabolism. Important products in these metabolic pathways have been confirmed to be related to the pathogenesis and prognosis of epilepsy (20,21).
Additionally, using research methods similar to those used in this study, some other studies have verified our research results to a certain extent. Akiyama et al. (22) analyzed the cerebrospinal fluid samples of 64 patients by GC-MS. The study clustered patients according to their age, and a multivariate analysis was conducted for 2 age groups (i.e., a 0–5-year-old group and a 6–17-year-old group) to clarify the effects of epilepsy and antiepileptic drugs on metabolites. In the patients aged 0–5 years (22 with epilepsy and 13 without epilepsy), 2-ketoglutarate was more decreased, and pyridoxamine and tyrosine were more increased in patients with epilepsy than those without epilepsy. In patients aged 6–17 years (12 with epilepsy and 17 without epilepsy), 1,5-dehydrated glucosinol was decreased, but 2-aminobutyric acid, 2-ketoisohexanoic acid, 4-hydroxyproline, acetylglycine, methionine, n-acetylserine, and serine were increased. This study indicated that decreased energy metabolism and changes in vitamin B6 metabolism may play a role in childhood epilepsy (22).
This study has some limitations. First, this study failed to explain the correlation between differential metabolites and the clinical characteristics of seizures due to the small sample size. This study did not include in vivo and in vitro experimental validation and functional research, so differential metabolites’ role in the pathogenesis of epilepsy cannot be clarified.
In conclusion, this study provided a cerebrospinal-fluid metabolite map of epileptic and non-epileptic patients. Our findings provide insights that may inform the discovery of therapeutic targets and diagnostic markers for epilepsy.
Acknowledgments
Funding: This study was supported by the 2018 Dalian Science and Technology Innovation Fund Plan (No. 2018J13SN100) and National Key R&D Program of China (No. 2018AAA0100300).
Footnote
Reporting Checklist: The authors have completed the MDAR reporting checklist. Available at https://atm.amegroups.com/article/view/10.21037/atm-22-1219/rc
Data Sharing Statement: Available at https://atm.amegroups.com/article/view/10.21037/atm-22-1219/dss
Conflicts of Interest: All authors have completed the ICMJE uniform disclosure form (available at https://atm.amegroups.com/article/view/10.21037/atm-22-1219/coif). The authors have no conflicts of interest to declare.
Ethical Statement: The authors are accountable for all aspects of the work, including ensuring that any questions related to the accuracy or integrity of any part of the work have been appropriately investigated and resolved. The study was conducted in accordance with the Declaration of Helsinki (as revised in 2013). The study was approved by the Ethics Committee of The First Affiliated Hospital of Dalian Medical University (No. PJ-KS-KY-2020-18), and informed consent was provided by all the patients.
Open Access Statement: This is an Open Access article distributed in accordance with the Creative Commons Attribution-NonCommercial-NoDerivs 4.0 International License (CC BY-NC-ND 4.0), which permits the non-commercial replication and distribution of the article with the strict proviso that no changes or edits are made and the original work is properly cited (including links to both the formal publication through the relevant DOI and the license). See: https://creativecommons.org/licenses/by-nc-nd/4.0/.
References
- Bastos F, Cross JH. Epilepsy. Handb Clin Neurol 2020;174:137-58. [Crossref] [PubMed]
- Akyuz E, Paudel YN, Polat AK, et al. Enlightening the neuroprotective effect of quercetin in epilepsy: From mechanism to therapeutic opportunities. Epilepsy Behav 2021;115:107701. [Crossref] [PubMed]
- Reddy DS. Catamenial Epilepsy: Discovery of an Extrasynaptic Molecular Mechanism for Targeted Therapy. Front Cell Neurosci 2016;10:101. [Crossref] [PubMed]
- Zilberter Y, Popova I, Zilberter M. Unifying mechanism behind the onset of acquired epilepsy. Trends Pharmacol Sci 2022;43:87-96. [Crossref] [PubMed]
- Arzimanoglou A, Brandl U, Cross JH, et al. Epilepsy and cannabidiol: a guide to treatment. Epileptic Disord 2020;22:1-14. [PubMed]
- Kaeberle J. Epilepsy Disorders and Treatment Modalities. NASN Sch Nurse 2018;33:342-4. [Crossref] [PubMed]
- Symonds JD, Zuberi SM, Johnson MR. Advances in epilepsy gene discovery and implications for epilepsy diagnosis and treatment. Curr Opin Neurol 2017;30:193-9. [Crossref] [PubMed]
- Pitkänen A, Lukasiuk K. Molecular biomarkers of epileptogenesis. Biomark Med 2011;5:629-33. [Crossref] [PubMed]
- Söylemez E, Öztürk O, Baslo SA, et al. Metabolic syndrome and obstructive sleep apnea syndrome among patients with epilepsy on monotherapy. Epilepsy Behav 2020;111:107296. [Crossref] [PubMed]
- Arend J, Kegler A, Caprara ALF, et al. Depressive, inflammatory, and metabolic factors associated with cognitive impairment in patients with epilepsy. Epilepsy Behav 2018;86:49-57. [Crossref] [PubMed]
- Patel M. A Metabolic Paradigm for Epilepsy. Epilepsy Curr 2018;18:318-22. [Crossref] [PubMed]
- Bainbridge MN, Cooney E, Miller M, et al. Analyses of SLC13A5-epilepsy patients reveal perturbations of TCA cycle. Mol Genet Metab 2017;121:314-9. [Crossref] [PubMed]
- Weiss N, Barbier Saint Hilaire P, Colsch B, et al. Cerebrospinal fluid metabolomics highlights dysregulation of energy metabolism in overt hepatic encephalopathy. J Hepatol 2016;65:1120-30. [Crossref] [PubMed]
- Mo L, Ding X, Tan C, et al. Association of cerebrospinal fluid zinc-α2-glycoprotein and tau protein with temporal lobe epilepsy and related white matter impairment. Neuroreport 2019;30:586-91. [Crossref] [PubMed]
- Ortiz WR, Gilbert JC, Millichap JG. Convulsions and sugar transport in brain. Neurology 1966;16:1191-3. [Crossref] [PubMed]
- Jaeken J. Genetic disorders of gamma-aminobutyric acid, glycine, and serine as causes of epilepsy. J Child Neurol 2002;17 Suppl 3:3S84-7; discussion 3S88.
- Boison D. The Biochemistry and Epigenetics of Epilepsy: Focus on Adenosine and Glycine. Front Mol Neurosci 2016;9:26. [Crossref] [PubMed]
- Ude C, Ambegaonkar G. Glycine receptor antibody-associated epilepsy in a boy aged 4 years. BMJ Case Rep 2016;2016:bcr2016216468. [Crossref] [PubMed]
- Shen HY, van Vliet EA, Bright KA, et al. Glycine transporter 1 is a target for the treatment of epilepsy. Neuropharmacology 2015;99:554-65. [Crossref] [PubMed]
- Novelli A, Tasker RA. Excitatory amino acids in epilepsy: from the clinics to the laboratory. Amino Acids 2007;32:295-7. [Crossref] [PubMed]
- Terzioğlu B, Aypak C, Onat FY, et al. The effects of ethosuximide on amino acids in genetic absence epilepsy rat model. J Pharmacol Sci 2006;100:227-33. [Crossref] [PubMed]
- Akiyama T, Saigusa D, Hyodo Y, et al. Metabolic Profiling of the Cerebrospinal Fluid in Pediatric Epilepsy. Acta Med Okayama 2020;74:65-72. [PubMed]
(English Language Editor: L. Huleatt)