An N6-methyladenosine-associated lncRNA signature for predicting clinical outcome and therapeutic responses in hepatocellular carcinoma
Introduction
Hepatocellular carcinoma (HCC), one of the most common malignant tumors, is the leading cause of tumor-related mortality worldwide (1). Although all kinds of therapeutic approaches, such as surgical resection, liver transplantation, radiofrequency ablation, and target therapies, have had a positive effect on patient prognosis, postoperative early recurrence and distant metastasis are still major impediments to the improvement of patient survival (2,3). Currently, some predictors, such as alpha fetoprotein (AFP), microvascular invasion (MVI) and tumor stage and grade, have been used for prognosis assessment in HCC, while the predictive performance still need to be improved.
N6-methyladenosine (m6A) is the most abundant epigenetic modification of eukaryotic cells (4). It is predominantly located at 3' untranslated regions, around the long internal exons and stop codons (5,6). Studies have shown that m6A modifications play an important role in various biological processes and mRNA metabolisms, such as abundance, alternative splicing, processing, translation, and stability (7-9). Three major classes of proteins involved in m6A methylation have been identified: methyltransferases, demethylases, and RNA-binding proteins. Methyltransferases, also named “writers”, catalyze m6A methylation modifications of bases by stable complexes formed by combinations of methyltransferase like (METTL)3, METTL14, and Wilms’ tumor 1-associated protein (WTAP), among others (10,11). Demethylases, also called “erasers”, ensure a dynamic and reversible process of m6A and include α-ketoglutarate-dependent dioxygenase homolog 5 (ALKBH5) and fat mass and obesity-associated (FTO) (10,11). RNA-binding proteins, also named “readers”, specifically bind to m6A methylation sites, including YT521-B homology (YTH) structural domain family proteins and insulin-like growth factor 2 mRNA-binding protein (IGF2BP)1/2/3 (10,11).
An increasing number of studies have demonstrated that m6A methylation is crucial for tumorigenesis and progression in various cancer types (12,13). One previous study showed that hypoxia-induced factor (HIF)-1α-induced YTHDF1 expression was related to autophagy-related HCC progression through promoting the translation of autophagy-related genes in an m6A-dependent manner (14). Upregulated METTL3 can contribute to HCC progression, while knockdown of METTL3 has been shown to remarkably reduce proliferation, migration, and colony formation of HCC cells and suppress tumorigenicity and lung metastasis in vivo (15). Recently, several bioinformatics studies have demonstrated that m6A-related regulators or genes could be used to predict patient survival (16,17). In addition, 3 m6A-related long noncoding RNAs (lncRNAs) (LINC02362, SNHG20, and SNHG6) have been developed to predict the survival of patients with HCC, and the areas under the curve (AUCs) for 1-, 3-, and 5-year survival after surgery in the testing cohort were 0.708, 0.635, and 0.611, respectively. Therefore, the predictive performance still needs to be improved (18).
At present, there are few studies involving in the role of m6A-related lncRNAs in HCC. Therefore, it is important to investigate the interrelationship between the m6A-related lncRNAs and HCC, and evaluate the prognostic values of m6A-related lncRNAs. In this study, we analyzed expression patterns of m6A-related lncRNAs and their prognostic values, and then constructed an m6A-related prognostic risk model for patients with HCC, in attempt to find out and identify the potential biomarkers and promising therapeutic targets for HCC. We present the following article in accordance with the TRIPOD reporting checklist (available at https://atm.amegroups.com/article/view/10.21037/atm-22-1583/rc).
Methods
Data and patients
RNA sequencing data calculated as fragments per kilobase million (FPKM), somatic mutation data, clinicopathological characteristics, and survival information for patients with HCC were acquired from the Cancer Genome Atlas (TCGA) (https://portal.gdc.cancer.gov/) database. The expression data of lncRNAs were obtained from the RNA expression profile. Patients with missing data were excluded. The flowchart of this study is shown in the Figure 1. The study was conducted in accordance with the Declaration of Helsinki (as revised in 2013).
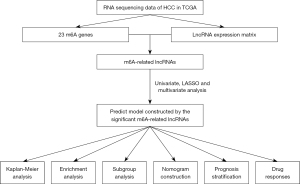
m6A genes and m6A-related lncRNAs
In this study, 23 m6A RNA methylation regulators, including 8 writers [METTL3, METTL14, METTL16, RNA-binding motif protein (RBM)15, RBM15B, WTAP, vir like m6A methyltransferase associated (VIRMA), and zinc finger CCCH domain-containing protein 13 (ZC3H13)], 2 erasers (ALKBH5, FTO), and 13 readers [YTHDC1, YTHDC2, YTHDF1, YTHDF2, YTHDF3, IGF2BP1, IGF2BP2, IGF2BP3, heterogeneous nuclear ribonucleoprotein (HNRNP)A2B1, HNRNPC, FMRP translational regulator 1 (FMR1), leucine rich pentatricopeptide repeat containing (LRPPRC), and RNA binding motif protein X-linked (RBMX)], were obtained as per previous study (19). Then, m6A-related lncRNAs were screened using Pearson’s correlation coefficient (r) based on the criteria of r>0.40 and P<0.001.
Establishment and validation of an m6A-related lncRNA signature
All included patients were randomly allocated to a training (n=186) or testing (n=184) cohort. The training cohort was used to develop and establish the prognostic risk model, while the testing cohort was used to validate the model’s predictive performance. The prognostic value of m6A-related lncRNAs was investigated through univariate Cox regression analysis. To enhance the prediction performance, a least absolute shrinkage and selection operator (LASSO)-penalized Cox regression analysis was performed to further screen lncRNAs distinctly related to overall survival (OS). LASSO Cox regression has been shown to improve the prediction accuracy and interpretability of the statistical model and enables variable selection and regularization simultaneously (20). The sum of multivariate regression coefficients of m6A-associated lncRNAs and their expressions generated an m6A-associated lncRNA signature score. The formula was as follows: (βlncRNA1 × expression level of lncRNA1) + (βlncRNA2 × expression level of lncRNA2) + … + (βlncRNAn × expression level of lncRNAn). The cut-off value of the risk score was set as the median signature score in the training cohort. Therefore, patients were divided into either a high-risk or a low-risk group based on the risk score cut-off values.
Identification of the clinicopathological risk parameters of HCC
Cox regression analysis was performed to further investigate the predictive model’s clinicopathological risk factors and prognostic ability. Univariate and multivariate analyses were performed in the training, testing, and entire cohorts. The parameters included age, gender, histological grade, the American Joint Committee on Cancer (AJCC) stage, and the risk score. Parameters with significant differences in the univariate analysis model were further confirmed in the multivariate analysis model.
Establishment of a predictive nomogram
A predictive nomogram was built to assess 1-, 3-, and 5-year OS after surgery based on these parameters, including age, gender, stage, and risk level. Calibration curves were also created to test the accuracy of the nomogram. The AUC of the nomogram, the m6A-associated lncRNA signature score and other clinical parameters were calculated and compared. In this step, patients whose clinical characteristics display “Unknown” were excluded for subsequent analyses. R package “rms” (https://cran.rstudio.com/web/packages/rms/index.html) was used to develop the nomogram.
Principal component analysis (PCA)
PCA is a mathematical algorithm for dimensionality reduction, model identification, and visualization of high-dimensional data. This study performed PCA to identify and categorize patients as high- or low-risk based on the expression profiles of the entire gene matrix, 23 m6A genes, and 14 m6A-related lncRNAs.
Functional analysis
The “c2.cp.kegg.v7.0.symbols” gene set was obtained from the MSigDB database for gene‐set variation analysis (GSVA). The enrichment scores of the pathways in each sample were calculated using GSVA and compared between the 2 risk groups. An adjusted P value <0.05 indicated a significant difference. In addition, significant pathways were selected to generate a heatmap. R packages “limma”, “GSVA”, and “GSEABase” were used to determine the enriched pathways between the 2 groups.
Immunotherapeutic and targeted therapy response
We further investigated the sorafenib therapeutic response in the 2 risk groups using a public database (the Genomics of Drug Sensitivity in Cancer, https://www.cancerrxgene.org/). The R package “pRRophetic” was used in this step. Then, the differences in immune cell infiltration between the high- and low-risk groups were compared using the “ssGSEA” method based on the “limma”, “GSVA”, and “GSEABase” R packages. To investigate the potential value of the predictive model in immunotherapy, the expression levels of 5 gene markers related to immune checkpoint inhibitors (PD-1, CTLA4, LAG3, TIM3, and GZMB) were compared between the high- and low-risk groups. Then, immunophenoscore (IPS) data, generated by the expression of 4 determinants—MHC molecules, effector cells, immunomodulators, and suppressor cells—were obtained from the Cancer Immunome Database (TCIA, https://tcia.at/home) for further comparison (21). Higher IPS was associated with enhanced immunogenicity.
Statistical analysis
Continuous variables were assessed by t-test, and categorical variables were compared by the Chi-square or Fisher exact test. Kaplan-Meier curves were calculated, and log-rank tests were performed to investigate the prognostic value of the risk score. A time-dependent receiver operating characteristic (ROC) curve was developed to assess the performance of the predictive model. The tumor mutation burden (TMB) was also calculated by defining the total number of mutations per megabyte of tumor tissue, and the median TMB score was used as a cut-off value to divide patients into high-TMB and low-TMB groups. All data were analyzed using R (version 3.6.1, Lucent Technologies, USA, https://www.r-project.org) and Strawberry Perl (version 5.32.0.1, https://www.perl.org). A two-tailed P value <0.05 indicated a significant difference.
Results
Identification of m6A-related lncRNAs in HCC
The expression of 23 m6A genes and 14,086 lncRNAs was obtained from TCGA database. According to the screening criteria (r>0.40 and P<0.001), 21 m6A genes and 624 m6A-related lncRNAs were identified. A visualized co-expression network between 21 m6A genes and 624 m6A-related lncRNAs was built (Figure 2A).
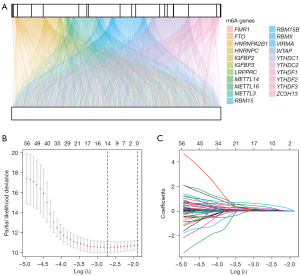
Development and validation of an m6A-related predictive risk model for HCC
To further screen the m6A-related prognostic lncRNAs, univariate Cox regression analysis was performed for the 624 m6A-associated lncRNAs, 68 of which were selected. To develop an m6A-related lncRNA predictive risk model, 370 patients with HCC were randomly divided into a training cohort (n=186) and a testing cohort (n=184). There were no statistical differences between the training and testing cohorts in terms of age (P=0.851), gender (P=0.710), AJCC stage (P=0.713), grade (P=0.905), T stage (P=0.653), N stage (P=1.000), or M stage (P=0.637) (Table S1).
We found that 14 m6A-related lncRNAs were significantly correlated with prognosis after LASSO Cox regression analysis (Figure 2B,2C). A prognostic model was then constructed to assess the prognostic risk levels in patients with HCC, based on the Cox regression model coefficients (β) and gene expression levels: the risk score of the prognostic model = AP001469.3 × 0.083 + AL031985.3 × 0.118 + SREBF2-AS1 × 0.037 + AL442125.2 × 0.033 + MKLN1-AS × 0.557 + AL590705.3 × 0.363 + TMCC1-AS1 × 0.216 + NRAV × 0.054 + C2orf27A × 0.124 + POLH-AS1 × 0.012 + AL158166.1 × 0.028 + LINC01138 × 0.004 + WAC-AS1 × 0.019 + AL117336.2 × 0.054. The median risk score value was calculated and used as the cut-off value for the training cohort. All patients were then divided into the high-risk group (≥ cut-off value) or the low-risk group (< cut-off value).
We further investigated the distribution of risk grades compared with the differences in survival status and survival time between the 2 risk groups. In addition, we analyzed the relative expression levels of 14 m6A-associated lncRNAs in each patient in the training cohort (Figure 3A). To validate the predictive performance of the risk model, we also calculated the distribution of risk grades between the low-risk and high-risk groups, the survival status and survival time of patients in both groups, and the relative expression levels of 14 m6A-associated lncRNAs in the testing cohort and the entire cohort (Figure 3B,3C, respectively).
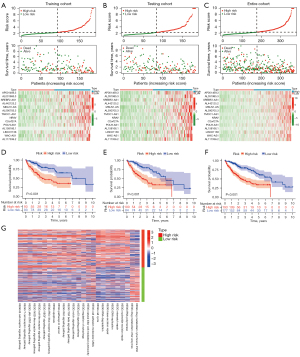
The prognostic values of the risk model were further investigated. The Kaplan-Meier curves showed that low-risk patients achieved better OS rates than high-risk patients in the training, testing cohort, and entire cohort (log-rank test, P<0.001, P<0.001 and P<0.001, respectively) (Figure 3D-3F). These findings indicate that the predictive risk model could stratify patients with a high risk of death. In addition, GSVA revealed the significant pathways enriched between the 2 groups. These pathways are primarily involved in drug metabolism, genomic instability, carcinogenesis processes, and the regulation of immune checkpoint expression (Figure 3G).
To further compare the prediction performance of the model at different time points, time-dependent ROC curve analysis was carried out in the training, testing, and entire cohorts. The AUCs for 1-year OS after surgery in the training, testing, and entire cohorts were 0.772, 0.788, and 0.785, respectively (Figure 4A-4C). For 3-year OS, the respective AUCs were 0.785, 0.709, and 0.737 (Figure 4D-4F), and for 5-year OS, the respective AUCs were 0.759, 0.706, and 0.711 (Figure 4G-4I). These findings show the potential prognostic ability of the predictive model at different time points.
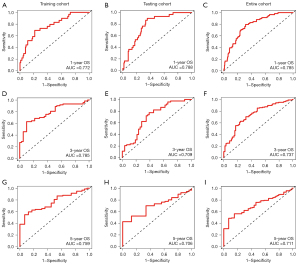
The m6A-related risk score as an independent risk factor
Univariate Cox regression analysis identified the significant prognostic variables for patients with HCC. Clinical parameters, including age, gender, AJCC stage, pathological grade, and the risk score, were used for the univariate analysis. We found that the risk score and AJCC stage were significantly related to OS in the training cohort (Table 1). Multivariate analysis further confirmed that the risk score and AJCC stage were the independent risk factors for the prognosis of patients with HCC in the training cohort (Table 1). In the testing and entire cohorts, multivariate analysis showed that the risk score was the independent risk factor for HCC prognosis (Table 1).
Table 1
Variable | Univariable model | Multivariable model | |||
---|---|---|---|---|---|
HR (95% CI) | P value | HR (95% CI) | P value | ||
Training cohort | |||||
Age | 1.107 (0.995–1.040) | 0.128 | – | – | |
Sex (male and female) | 0.677 (0.387–1.149) | 0.144 | – | – | |
Grade (1, 2, 3 and 4) | 1.181 (0.817–1.708) | 0.377 | – | – | |
Stage (I, II, III and IV) | 2.057 (1.514–2.796) | <0.001 | 1.822 (1.306–2.542) | <0.001 | |
Risk scores | 1.447 (1.284–1.631) | <0.001 | 1.344 (1.188–1.521) | <0.001 | |
Testing cohort | |||||
Age | 1.003 (0.984–1.023) | 0.763 | – | – | |
Sex (male and female) | 0.917 (0.534–1.573) | 0.752 | – | – | |
Grade (1, 2, 3 and 4) | 1.107 (0.784–1.563) | 0.565 | – | – | |
Stage (I, II, III and IV) | 1.385 (1.047–1.838) | 0.023 | 1.277 (0.954–1.710) | 0.101 | |
Risk scores | 1.112 (1.060–1.166) | <0.001 | 1.099 (1.046–1.155) | <0.001 | |
Entire cohort | |||||
Age | 1.010 (0.996–1.025) | 0.174 | – | – | |
Sex (male and female) | 0.776 (0.531–1.132) | 0.188 | – | – | |
Grade (1, 2, 3 and 4) | 1.133 (0.881–1.457) | 0.330 | – | – | |
Stage (I, II, III and IV) | 1.680 (1.369–2.062) | <0.001 | 1.562 (1.262–1.932) | <0.001 | |
Risk scores | 1.137 (1.096–1.179) | <0.001 | 1.112 (1.070–1.157) | <0.001 |
CI, confidence interval; HCC, hepatocellular carcinoma; HR, hazard ratio; OS, overall survival.
In addition, subgroup analyses were performed to investigate the predictive ability of the risk score in all patients. Notably, OS was significantly stratified based on high- or low-risk level in patients aged >65, patients aged ≤65, female patients, male patients, patients with pathological grade 1–2, pathological grade 3–4, patients with AJCC stage I–II, AJCC stage III–IV, patients with T stage1–2, T stage 3–4, patients with N0, and patients with M0 (Figure S1). These results show that the risk score is an independent prognostic factor for patients with HCC.
Development of the prognostic nomogram
A nomogram was created to predict 1-, 3- and 5-year survival after surgery. The total points were used to evaluate the cumulative survival risk at different time points (Figure 5A). The AUCs of nomogram for assessment of 1-, 3-, and 5-year survival after surgery were 0.794, 0.765, and 0.742, respectively, and the predictive performances were better than risk score and other clinical characteristics (Figure 5B-5D). The calibration curve revealed the ideal consistency of the nomogram for predicting 1-, 3-, and 5-year survival probability (Figure 5E-5G). These findings indicated the predictive accuracy of the nomogram.
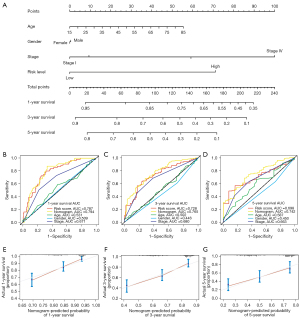
PCA
The grouping ability of the m6A-associated lncRNA model was further validated. To examine the differences between the low- and high-risk groups, PCA was performed on all gene expression profiles of HCC patients in the TCGA, 23 m6A genes, and 14 m6A-associated lncRNAs (Figure S2A-S2C). As shown in Figure S2C, our model could significantly differentiate the low-risk group from the high-risk group. These results suggest that prognostic characteristics can distinguish low- or high-risk groups.
Prognostic stratification based on the risk score and TP53 gene status or TMB score
Next, we analyzed and compared the mutation information between the high-risk and low-risk groups. The alteration frequencies of the top 20 driver genes were higher in the high-risk group than in the low-risk group (Figure 6A,6B). We further examined the proportion of TP53 mutation in the whole population. In the training cohort, TP53 mutation was detected in 37% and 20% of patients in the high- and low-risk groups, respectively (P=0.021; Figure 6C). Similarly, a higher percentage of TP53 mutation was observed in the high-risk group than in the low-risk group in the testing cohort (38% vs. 14%, P<0.001) and in the entire cohort (38% vs. 17%, P<0.001) (Figure 6D,6E). The findings indicated that the risk score was significantly related to the frequency of TP53 mutation.
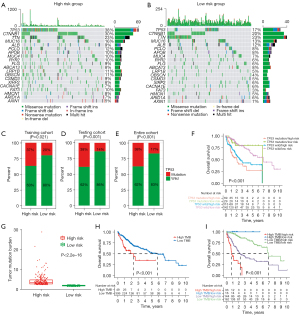
Considering the role of TP53 in patient prognosis, we further compared the prognostic value of the risk score in patients with TP53 wild type or TP53 mutations (Figure 6F). Intriguingly, the OS of patients with TP53 wild type or TP53 mutations in the high-risk group was worse than that of patients with TP53 wild type or TP53 mutations in the low-risk group, respectively, suggesting the risk score could stratify prognosis for all patients with or without TP53 mutation. Interestingly, patients with TP53 wild-type in the high-risk group achieved worse OS rates than patients with TP53 mutations in the low-risk group. However, similar tendencies of survival curves were observed in the low-risk group’ patients with TP53 mutations or TP53 wild-type, indicating that TP53 failed to stratify patient prognosis in the low-risk group. These findings showed that the m6A-related lncRNA model performed better than TP53 mutation status in predicting patient OS.
We also investigated the TMB scores between the 2 groups. Importantly, the TMB scores based on TCGA somatic mutation data in the high-risk group were higher than those in the low-risk group (Figure 6G). In addition, the prognostic values of the m6A-related lncRNA model were determined for subpopulations with high or low TMB scores (Figure 6H). Our results consistently showed that the m6A-related lncRNA model performed better than TMB scores in predicting patient OS (Figure 6I).
Assessment of therapeutic responses by the m6A-related lncRNA model
Further analysis assessed the therapeutic response of sorafenib, based on the half-maximal inhibitory concentration (IC50) using the pRRophetic algorithm. The result showed that sorafenib sensitivity in the high-risk group was higher than that in the low-risk group, suggesting the potential therapeutic values of sorafenib in the high-risk group (Figure 7A).
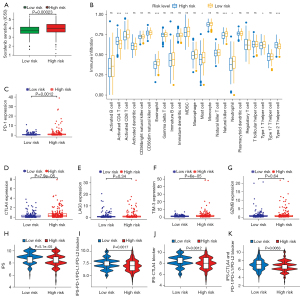
According to the potential function and pathways revealed by GSVA, we next compared the differences of immune cell infiltration between the 2 groups to evaluate the therapeutic response of immune-checkpoint inhibitors. Interestingly, higher levels of immune cell infiltration were observed in the low-risk group than in the high-risk group (Figure 7B). The expression levels of 5 immune checkpoint genes, including PD-1, CTLA4, LAG3, TIM-3, and GZMB, were also detected (Figure 7C-7G). Notably, the expression levels of PD-1, CTLA4, and TIM-3 in the high-risk group were higher than those in the low-risk group (Figure 7C,7D,7F). Moreover, 4 calculated scores—IPS, IPS-PD-1/PD-L1/PD-L2 blockers, IPS-CTLA4 blockers, and IPS-CTLA4 + PD-1/PD-L1/PD-L2 blockers—were significantly higher in the low-risk group (P<0.05) (Figure 7H-7K), indicating that there was a more immunogenic phenotype in the low-risk group. These findings suggest that this risk model could be a promising predictor for assessing the application of sorafenib or immune checkpoint inhibitors.
Discussion
Recently, an increasing number of studies have focused on predicting the prognosis of patients with HCC (16,17). However, it is challenging to assess patient survival based on limited clinical characteristics due to tumor heterogeneity. As the most abundant epigenetic modification of eukaryotic cells, m6A has been reported to be related to the progression and prognosis of HCC (14,15,22). A recent study revealed that YTHDC2 and FTO could be promising targets for predicting and improving survival in patients with HCC treated with transarterial chemoembolization (TACE) (23). Meanwhile, numerous studies have shown the role of lncRNAs in the occurrence and progression of HCC (24,25). However, the detailed mechanisms of the significant clinical value of m6A-related lncRNAs in HCC remain unclear.
In the present study, 14 significant m6A-associated lncRNAs were identified by LASSO Cox regression and used to construct a prognostic risk model to assess patient survival. Several studies have reported that 6 of these 14 lncRNAs—AL031985.3, MKLN1-AS, TMCC1-AS1, C2orf27A, LINC01138, and NRAV—are prognosis-related in HCC (26-33). LINC01138 could be a promising prognostic indicator, and the LINC01138/PRMT5 axis an ideal therapeutic target for HCC treatment (32).
A novel prognostic risk score was constructed to predict patient survival according to these 14 lncRNAs, and high- or low-risk groups were identified. We found that high-risk patients were associated with worse prognosis in the training, testing, and entire cohorts, and in the subgroup analyses, indicating that the risk score could be an independent prognostic signature for HCC. Time-dependent ROC curves showed the robust predictive performance for HCC patient prognosis at 1-, 3-, and 5-years after surgery. In addition, a nomogram integrated with clinical parameters and risk level (stratified by the risk score) was established, and the calibration curves showed good consistency for 1-, 3-, and 5-year survival probabilities. These findings suggest the potential prognostic value of this model, developed using the 14 m6A-related lncRNAs, for patients with HCC.
It has been suggested that an accumulation of gene mutations could drive the development and progression of HCC (34). In the present study, the frequency of gene mutations was higher in the high-risk group than in the low-risk group. A higher percentage of significant gene mutations has been associated with faster tumor progression and poorer survival (34). Notably, the risk levels calculated by our m6A-related lncRNA model could stratify the OS in all patients with or without TP53 mutations, while TP53 status failed to differentiate survival in low-risk patients, indicating that this model outperformed TP53 mutation status in predicting patient OS. An increasing number of studies have demonstrated that the TMB is a valid biomarker for predicting the response to immunotherapy (35,36). Higher TMB scores were observed in the high-risk group than in the low-risk group, indicating the potential value of using risk scores for immunotherapy evaluation. Similarly, our model outperformed TMB scores in predicting patient OS.
Sorafenib is the standard treatment for advanced HCC, and patients can achieve better survival rates after sorafenib treatment (37). The estimated therapeutic response of sorafenib was different between the high- and low-risk patients, which provides a new understanding for patient survival. One lncRNA in the predictive model, C2orf27A, has been identified as a key sorafenib resistance-related gene in HCC (31) and could be the key predictor in the assessment of therapeutic responses to sorafenib. IPS has been reported as a potential biomarker for immunotherapy (21,38). Four calculated scores—IPS, IPS-PD-1/PD-L1/PD-L2, IPS-CTLA4, and IPS-CTLA4 + PD-1/PD-L1/PD-L2—were significantly higher in the low-risk group than those in the high-risk group (P<0.05). Therefore, we speculated that low-risk patients could benefit more than high-risk patients from receiving the immune-checkpoint inhibitors. This could be one of the reasons for better prognoses in the low-risk group.
There were several limitations in this study. First, the predictive risk model was built on TCGA database. Independent databases should be used to validate the model’s predictive performance. Secondly, the sample of current study was small in both training and testing cohorts, so further study with a larger population should be performed to validated the predictive values. Finally, functional studies should be performed to determine the detailed mechanism of these lncRNAs both in vivo and in vitro.
In conclusion, we identified the novel m6A-related lncRNA risk score as an independent risk factor for predicting survival in patients with HCC. The proposed model revealed the potential performance for assessing therapeutic responses to sorafenib and immunotherapies, which may help doctors with clinical decision-making for patients with HCC.
Acknowledgments
Funding: This work was supported by the Cultivating funding of The Cancer Hospital of the University of Chinese Academy of Sciences (grant No. PY2021025), the Medicine and Health Discipline Platform Project of Zhejiang Province (grant No. 2018RC019), and the Medicine and Health Science Project of Zhejiang Province (grant No. 2020KY483).
Footnote
Reporting Checklist: The authors have completed the TRIPOD reporting checklist. Available at https://atm.amegroups.com/article/view/10.21037/atm-22-1583/rc
Conflicts of Interest: All authors have completed the ICMJE uniform disclosure form (available at https://atm.amegroups.com/article/view/10.21037/atm-22-1583/coif). The authors have no conflicts of interest to declare.
Ethical Statement: The authors are accountable for all aspects of the work in ensuring that questions related to the accuracy or integrity of any part of the work are appropriately investigated and resolved. The study was conducted in accordance with the Declaration of Helsinki (as revised in 2013).
Open Access Statement: This is an Open Access article distributed in accordance with the Creative Commons Attribution-NonCommercial-NoDerivs 4.0 International License (CC BY-NC-ND 4.0), which permits the non-commercial replication and distribution of the article with the strict proviso that no changes or edits are made and the original work is properly cited (including links to both the formal publication through the relevant DOI and the license). See: https://creativecommons.org/licenses/by-nc-nd/4.0/.
References
- Villanueva A. Hepatocellular Carcinoma. N Engl J Med 2019;380:1450-62. [Crossref] [PubMed]
- Xie DY, Ren ZG, Zhou J, et al. 2019 Chinese clinical guidelines for the management of hepatocellular carcinoma: updates and insights. Hepatobiliary Surg Nutr 2020;9:452-63. [Crossref] [PubMed]
- Sun Y, Wu L, Zhong Y, et al. Single-cell landscape of the ecosystem in early-relapse hepatocellular carcinoma. Cell 2021;184:404-421.e16. [Crossref] [PubMed]
- Desrosiers R, Friderici K, Rottman F. Identification of methylated nucleosides in messenger RNA from Novikoff hepatoma cells. Proc Natl Acad Sci U S A 1974;71:3971-5. [Crossref] [PubMed]
- Meyer KD, Saletore Y, Zumbo P, et al. Comprehensive analysis of mRNA methylation reveals enrichment in 3' UTRs and near stop codons. Cell 2012;149:1635-46. [Crossref] [PubMed]
- Dominissini D, Moshitch-Moshkovitz S, Schwartz S, et al. Topology of the human and mouse m6A RNA methylomes revealed by m6A-seq. Nature 2012;485:201-6. [Crossref] [PubMed]
- Wang X, Lu Z, Gomez A, et al. N6-methyladenosine-dependent regulation of messenger RNA stability. Nature 2014;505:117-20. [Crossref] [PubMed]
- Wang X, Zhao BS, Roundtree IA, et al. N(6)-methyladenosine Modulates Messenger RNA Translation Efficiency. Cell 2015;161:1388-99. [Crossref] [PubMed]
- Edupuganti RR, Geiger S, Lindeboom RGH, et al. N6-methyladenosine (m6A) recruits and repels proteins to regulate mRNA homeostasis. Nat Struct Mol Biol 2017;24:870-8. [Crossref] [PubMed]
- Jiang X, Liu B, Nie Z, et al. The role of m6A modification in the biological functions and diseases. Signal Transduct Target Ther 2021;6:74. [Crossref] [PubMed]
- Li J, Liang L, Yang Y, et al. N6-methyladenosine as a biological and clinical determinant in colorectal cancer: progression and future direction. Theranostics 2021;11:2581-93. [Crossref] [PubMed]
- Li H, Wu H, Wang Q, et al. Dual effects of N6-methyladenosine on cancer progression and immunotherapy. Mol Ther Nucleic Acids 2021;24:25-39. [Crossref] [PubMed]
- Nombela P, Miguel-López B, Blanco S. The role of m6A, m5C and Ψ RNA modifications in cancer: Novel therapeutic opportunities. Mol Cancer 2021;20:18. [Crossref] [PubMed]
- Li Q, Ni Y, Zhang L, et al. HIF-1α-induced expression of m6A reader YTHDF1 drives hypoxia-induced autophagy and malignancy of hepatocellular carcinoma by promoting ATG2A and ATG14 translation. Signal Transduct Target Ther 2021;6:76. [Crossref] [PubMed]
- Chen M, Wei L, Law CT, et al. RNA N6-methyladenosine methyltransferase-like 3 promotes liver cancer progression through YTHDF2-dependent posttranscriptional silencing of SOCS2. Hepatology 2018;67:2254-70. [Crossref] [PubMed]
- Chang X, Lv YF, He J, et al. Gene Expression Profile and Prognostic Value of m6A RNA Methylation Regulators in Hepatocellular Carcinoma. J Hepatocell Carcinoma 2021;8:85-101. [Crossref] [PubMed]
- Li Y, Qi D, Zhu B, et al. Analysis of m6A RNA Methylation-Related Genes in Liver Hepatocellular Carcinoma and Their Correlation with Survival. Int J Mol Sci 2021;22:1474. [Crossref] [PubMed]
- Li L, Xie R, Lu G. Identification of m6A methyltransferase-related lncRNA signature for predicting immunotherapy and prognosis in patients with hepatocellular carcinoma. Biosci Rep 2021;41:BSR20210760. [Crossref] [PubMed]
- Jin C, Li R, Deng T, et al. Identification and Validation of a Prognostic Prediction Model of m6A Regulator-Related LncRNAs in Hepatocellular Carcinoma. Front Mol Biosci 2021;8:784553. [Crossref] [PubMed]
- Tibshirani R. The lasso method for variable selection in the Cox model. Stat Med 1997;16:385-95. [Crossref] [PubMed]
- Charoentong P, Finotello F, Angelova M, et al. Pan-cancer Immunogenomic Analyses Reveal Genotype-Immunophenotype Relationships and Predictors of Response to Checkpoint Blockade. Cell Rep 2017;18:248-62. [Crossref] [PubMed]
- Pan XY, Huang C, Li J. The emerging roles of m6A modification in liver carcinogenesis. Int J Biol Sci 2021;17:271-84. [Crossref] [PubMed]
- Liu J, Wang D, Zhou J, et al. N6-methyladenosine reader YTHDC2 and eraser FTO may determine hepatocellular carcinoma prognoses after transarterial chemoembolization. Arch Toxicol 2021;95:1621-9. [Crossref] [PubMed]
- Abbastabar M, Sarfi M, Golestani A, et al. lncRNA involvement in hepatocellular carcinoma metastasis and prognosis. EXCLI J 2018;17:900-13. [PubMed]
- Wei L, Wang X, Lv L, et al. The emerging role of microRNAs and long noncoding RNAs in drug resistance of hepatocellular carcinoma. Mol Cancer 2019;18:147. [Crossref] [PubMed]
- Jia Y, Chen Y, Liu J. Prognosis-Predictive Signature and Nomogram Based on Autophagy-Related Long Non-coding RNAs for Hepatocellular Carcinoma. Front Genet 2020;11:608668. [Crossref] [PubMed]
- Kong W, Wang X, Zuo X, et al. Development and Validation of an Immune-Related lncRNA Signature for Predicting the Prognosis of Hepatocellular Carcinoma. Front Genet 2020;11:1037. [Crossref] [PubMed]
- Deng X, Bi Q, Chen S, et al. Identification of a Five-Autophagy-Related-lncRNA Signature as a Novel Prognostic Biomarker for Hepatocellular Carcinoma. Front Mol Biosci 2020;7:611626. [Crossref] [PubMed]
- Gao W, Chen X, Chi W, et al. Long non-coding RNA MKLN1-AS aggravates hepatocellular carcinoma progression by functioning as a molecular sponge for miR-654-3p, thereby promoting hepatoma-derived growth factor expression. Int J Mol Med 2020;46:1743-54. [Crossref] [PubMed]
- Cui H, Zhang Y, Zhang Q, et al. A comprehensive genome-wide analysis of long noncoding RNA expression profile in hepatocellular carcinoma. Cancer Med 2017;6:2932-41. [Crossref] [PubMed]
- Yuan W, Tao R, Huang D, et al. Transcriptomic characterization reveals prognostic molecular signatures of sorafenib resistance in hepatocellular carcinoma. Aging (Albany NY) 2021;13:3969-93. [Crossref] [PubMed]
- Li Z, Zhang J, Liu X, et al. The LINC01138 drives malignancies via activating arginine methyltransferase 5 in hepatocellular carcinoma. Nat Commun 2018;9:1572. [Crossref] [PubMed]
- Xu Q, Wang Y, Huang W. Identification of immune-related lncRNA signature for predicting immune checkpoint blockade and prognosis in hepatocellular carcinoma. Int Immunopharmacol 2021;92:107333. [Crossref] [PubMed]
- Müller M, Bird TG, Nault JC. The landscape of gene mutations in cirrhosis and hepatocellular carcinoma. J Hepatol 2020;72:990-1002. [Crossref] [PubMed]
- Wong CN, Fessas P, Dominy K, et al. Qualification of tumour mutational burden by targeted next-generation sequencing as a biomarker in hepatocellular carcinoma. Liver Int 2021;41:192-203. [Crossref] [PubMed]
- Ricciuti B, Kravets S, Dahlberg SE, et al. Use of targeted next generation sequencing to characterize tumor mutational burden and efficacy of immune checkpoint inhibition in small cell lung cancer. J Immunother Cancer 2019;7:87. [Crossref] [PubMed]
- Couri T, Pillai A. Goals and targets for personalized therapy for HCC. Hepatol Int 2019;13:125-37. [Crossref] [PubMed]
- Yang S, Wu Y, Deng Y, et al. Identification of a prognostic immune signature for cervical cancer to predict survival and response to immune checkpoint inhibitors. Oncoimmunology 2019;8:e1659094. [Crossref] [PubMed]
(English Language Editor: L. Roberts)