Operable hepatitis B virus-related hepatocellular carcinoma: gut microbiota profile of patients at different ages
Introduction
Hepatocellular carcinoma (HCC) is the second leading cause of cancer-related mortality worldwide and has an increasing incidence rate (1). Most cases of HCC are secondary to either a viral hepatitis infection (hepatitis B or C) or cirrhosis in China (2), and are especially caused by the hepatitis B virus (HBV) (3). Multiple therapeutic approaches are used to treat HCC, including endocrine therapy, radiotherapy, ablation, transcatheter arterial chemoembolization, targeted therapy, and liver transplantation (4-8), but surgical resection is still the most common treatment method (9). Currently, surgical treatment combined with immunotherapy and molecular-targeted therapy is the first-line treatment HCC (10).
Recently, some studies have demonstrated that age is an independent risk factor for the HCC development, and young patients with advanced HCC tend to have poorer prognosis compared with old patients, thereby older HCC patients with good liver functional reserve are encouraged to receive surgical resection (11-14). In addition, previous studies have shown significant differences in gut microbiota existed between young and old patients with various diseases [such as obesity (15), type 2 diabetes (16), hypertension (17), chronic obstructive pulmonary diseases (18), chronic inflammation (19)]. These differences can affect the severity and prognosis of the disease itself in terms of inflammation, immunity, proliferation, and metabolism. For example, low-grade chronic inflammation can also increase the risk of insulin resistance and atherosclerosis, which are the dominant mechanisms in the development of cardiovascular diseases, especially in older people (20). Ulcerative colitis (UC), a common enteric disease, carries a high risk for colorectal carcinoma (CRC). The incidence of UC increased with age, and is also associated with an abnormal inflammatory response to enteric flora and imbalances in the intestinal immune system (21-23). CRC, as well as clinicopathological, malignant proliferation, treatment, and survival characteristics and the microbiomes differ according to age (24). Previously published study has indicated age-specific differences in gut microbiota composition and its metabolic functions in patients with depression, which provides a new perspective on its pathogenesis (25). With an increased understanding of gut microbiota dysbiosis in age-related cerebral small vessel disease (CSVD), gut microbiota dysbiosis-induced inflamm-aging has been recognized as a target for therapeutic interventions to delay the progression of age-related CSVD (26).
A growing body of evidence has suggested that gut microbial dysbiosis in the human gut is a vital contributing factor in multiple liver diseases. A study has described age as a risk factor for the development of non-alcoholic fatty liver disease (NAFLD) and different compositions of gut microbiota that are distinguished by different ages in NAFLD progression (27). The pro-inflammatory signal contained in gut microbiota dysbiosis in patients with alcoholic liver disease could change the metabolic function of gut microbiota and the composition and circulation of bile acid, and cause immune disorders related to the pathogenesis and progression of alcohol-related liver disease (28,29). In addition, liver injury caused by hepatitis B virus (HBV) can lead to liver cirrhosis and liver cancer (30). A previous study has shown that there is a significant enrichment of opportunistic pathogenic species (Fusobacteria, Clostridium difficile, Veillonella, and Escherichia coli) in patients with HBV in developing countries (31). When patients with HBV develop liver fibrosis and cirrhotic liver cancer, significant differences in the structure of the development of gut microbiota can occur (32,33).
The structure and stability of intestinal flora during disease progression differ among diseases (34), and the composition of gut microbiota can with age among some diseases, such as cardiovascular disease and Alzheimer’s disease (35). HCC is the most common liver cancer and has a poor prognosis (36). Various therapeutic measures have been used in the treatment of liver cancer; however, the effects of these measures are limited, rendering liver cancer difficult to treat (37). HCC has demonstrated an increasing rate incidence, and the age of onset is also becoming increasingly younger (38). A growing number of individuals are at a higher risk of worse treatment outcomes because of their advanced age and immune factors during treatment (39). The relationship between gut microbiota and HCC is becoming increasingly well known (40). In view of liver resection is still the main treatment method for HCC, the effect of different ages on intestinal flora of operational patients with HCC is unclear. Therefore, the aim of the present study was to assess the difference in gut microbial abundance and composition in patients with HBV-HCC who had liver resection at different ages; to evaluate correlations between age-associated characteristics of microbes and environmental factors of clinical characteristics; and to investigate the features of gut microbiota and its function associated with different ages. We present the following article in accordance with the STROBE reporting checklist (available at https://atm.amegroups.com/article/view/10.21037/atm-22-1572/rc).
Methods
Ethics statement
The study followed the ethical guidelines of the Helsinki Declaration (as revised in 2013), and was approved by the Research Ethics Committee of Guangxi Medical University Cancer Hospital (No. LW2022058). All patients who met our experimental conditions were informed of the research contents and signed informed consent forms.
Study design and cohort
All patients who were initially diagnosed with HBV-HCC (Child-Pugh Class A) and were able to undergo hepatic resection at the Department of Hepatobiliary Surgery, Guangxi Medical University Cancer Hospital (Nanning, China) from September 2020 to May 2021, were recruited consecutively for our cohort study. None of the participants in our study had a history of drug allergy, alcohol addiction, chronic infectious diseases, inflammatory bowel diseases or metabolic diseases, NAFLD, gastrointestinal disease, malignant tumors only in the liver, or diabetes, or took no pro-gastrointestinal prokinetic agents, acid suppressants, probiotics, or antibiotics for at least 4 weeks.
More than 60% of all cancers develop in patients aged ≥65 years (41), and the risk of HCC development is age dependent. In China, 92.9% of new HCC cases are diagnosed in patients aged ≥45 years (42). Therefore, we included 53 patients in the analysis, based on age <45 years [younger age group (Y.AG), n=20], 45–65 years [middle age group (M.AG), n=13], and >65 years [older age group (O.AG), n=20]. Fecal samples were collected after admission and immediately stored at –80 ℃.
Fecal DNA extraction and 16S rRNA gene amplicon sequencing
Bacterial DNA was extracted from fecal samples from each patient using the cetyltrimethylammonium bromide method, and genomic DNA was used for library construction. Polymerase chain reaction (PCR) was used to amplify the V3–V4 region of the small subunit gene of bacterial 16S rRNA (universal primers 341F/806R). PCR products were purified using the GeneJET Gel Extraction Kit (Thermo Scientific, Waltham, MA, USA). The amplicon libraries were constructed using the TruSeq DNA PCR-Free Sample Preparation Kit (Illumina, San Diego, CA, USA), and the libraries were sequenced on the Illumina Novaseq6000 platform (Beijing Nuohe Zhiyuan Technology, Beijing, China).
16S rRNA data analysis
Only quality-filtered data were used, and analysis of the original FASTQ file was performed using FLASH (version 1.2.7) and QIIME (version 1.9.1) (43,44). Operational taxonomic units (OTUs) were obtained at a 97% similarity sequence and annotated for further taxonomic analysis (confidence threshold =80%). An algorithm was then used to annotate the taxonomic information (45). Subsequent analysis of the α- and β-diversities was performed based on OTU abundance output normalized data at the phylum, class, order, family, genus, and species levels. Non-metric multidimensional scaling (NMDS) analysis (with the weighted UniFrac distance) assessed differences in bacterial community composition between groups of specimens. The linear discriminant analysis (LDA) effect size (LEfSe) was used to find potential biomarker(s) that differentially represented pairwise comparisons of the three groups. Redundancy analysis (RDA) was performed to analyze the correlation between the microbial community and environmental factors. PICRUSt2 was used to output functional information from the Integrated Microbial Genome (IMG) microbial genome data to predict metabolic pathways based on the Kyoto Encyclopedia of Genes and Genomes database (46). Heatmaps displayed the relationship between different gut microbiota and environmental factors, and between different gut microbiota and functions, which were investigated using Spearman correlations.
Statistical analysis
For the statistical analysis of the preoperative demographic data, SPSS version 25.0 (IBM, Armonk, NY, USA) was used with Fisher’s exact and the Mann-Whitney U-test. Analysis of α- and β-diversities was performed using QIIME (version 1.9.1) and R software (version 2.15.3). The LEfSe combines standard tests for statistical significance (Mann-Whitney U-test) with LDA. The threshold for the logarithmic LDA score for the discriminative features was 3.0. The Mann-Whitney U-test was used to compare data between groups using R software (version 2.15.3). Permutations (P<0.05) were used to select a set of environmental factors that had significant effects on microbial distribution. Statistical significance was set at P<0.05 which was two-sided. RDA and Spearman correlations were drawn using R (version 3.6.2). Benjamini-Hochberg correction was applied, where appropriate.
Results
Baseline clinical characteristics
Demographic and clinical characteristics, and the immunohistochemistry results of the Y.AG, the M.AG, and the O.AG, are summarized in Table 1. There were no statistically significant differences in other indices, except p53, between the Y.AG and the M.AG (all P<0.0001) (Table S1). Differential expressions of hemoglobin, α-fetoprotein (AFP), and C3 were found to be statistically significant between the M.AG and the O.AG (P=0.030, P=0.001, and P=0.008, respectively) (Table S2). Statistically significant differences were found in AFP, helper T (Th)/suppressor T (Ts), immunoglobulin G, C3, cytokeratin 19 (CK19), p53, and Ki67 between the Y.AG and the O.AG (P=0.004, P=0.046, P=0.020, P=0.038, P=0.003, P=0.004, and P=0.049, respectively) (Table 2).
Table 1
Characteristics | Y.AG (n=20) | M.AG (n=13) | O.AG (n=20) |
---|---|---|---|
Age (years) | 42.25±4.05 | 53.00±6.00 | 67.00±3.03 |
Sex | |||
Female | 3 (15.0%) | 2 (15.4%) | 2 (10.0%) |
Male | 17 (85.0%) | 11 (84.6%) | 18 (90.0%) |
BMI (kg/m2) | 22.70±2.75 | 22.19±3.26 | 23.59±2.34 |
WBC (109/L) | 6.19±2.05 | 6.12±2.58 | 5.78±1.46 |
HGB (g/L) | 135.65±19.53 | 128.46±20.78 | 143.80±14.81 |
PLT (109/L) | 221.55±81.53 | 183.77±71.46 | 200.80±77.81 |
TBIL (μmol/L) | 15.22±6.36 | 16.96±7.09 | 13.79±4.52 |
ALB (g/L) | 37.22±3.78 | 36.09±4.63 | 37.30±3.66 |
TRF (g/L) | 2.52±0.77 | 2.40±0.40 | 2.47±0.48 |
PA (mg/L) | 188.88±50.10 | 201.24±69.54 | 210.12±56.75 |
ALT (U/L) | 40.50±24.65 | 39.38±15.61 | 42.70±26.24 |
AST (U/L) | 48.90±27.82 | 36.46±10.49 | 43.10±25.25 |
γ-GGT (U/L) | 73.5±47.04 | 79.31±46.44 | 75.65±49.32 |
BUN (mg/dL) | 5.08±1.49 | 5.16±2.03 | 4.82±0.80 |
Cr (μmol/L) | 72.90±13.94 | 77.77±12.36 | 76.80±12.41 |
HBV-DNA (/ml) | 499,420.15±913,807.00 | 4,875,079.69±16,490,518.27 | 4,289,988.24±12,362,851.16 |
HBsAg (ng/mL) | 674.77±299.15 | 652.12±309.87 | 536.79±305.57 |
PT (s) | 12.83±1.45 | 12.85±0.93 | 12.50±1.67 |
INR | 1.05±0.12 | 1.04±0.07 | 1.02±0.14 |
AFP (ng/mL) | 12,838.96±25,008.58 | 14,078.51±25,990.07 | 391.78±1,106.39 |
TCH (mmol/L) | 4.45±1.08 | 5.24±1.58 | 4.78±1.07 |
HDL (mmol/L) | 1.19±0.28 | 1.30±0.33 | 1.11±0.27 |
LDL (mmol/L) | 3.19±1.01 | 3.81±1.71 | 3.50±0.97 |
Th/Ts (%) | 1.91±0.70 | 1.98±0.82 | 1.50±0.53 |
IgG (g/L) | 13.79±3.63 | 15.20±4.01 | 15.82±2.87 |
IgM (g/L) | 1.26±0.45 | 1.40±0.37 | 1.42±0.45 |
IgA (g/L) | 2.64±1.15 | 2.75±0.75 | 2.80±1.31 |
C3 (g/L) | 0.85±0.17 | 0.82±0.22 | 0.98±1.31 |
C4 (g/L) | 0.23±0.14 | 0.21±0.16 | 0.23±0.07 |
CK19 (%) | 12.10±15.43 | 8.46±22.58 | 0.35±1.18 |
P53 (%) | 51.00±23.37 | 11.92±17.86 | 22.60±34.21 |
Ki67 (%) | 42.00±23.81 | 23.08±17.50 | 27.25±14.09 |
Ascites | |||
No | 18 (90.0%) | 11 (84.6%) | 19 (95.0%) |
Yes | 2 (10.0%) | 2 (15.4%) | 1 (5.0%) |
Smoking | |||
No | 15 (66.7%) | 10 (66.7%) | 16 (66.7%) |
Yes | 5 (33.3%) | 3 (33.3%) | 4 (33.3%) |
Drinking | |||
No | 18 (90.0%) | 12 (92.3%) | 16 (80.0%) |
Yes | 2 (10.0%) | 1 (8.7%) | 4 (20.0%) |
Portal hypertension (mmHg) | |||
No | 11 (55.0%) | 10 (76.9%) | 15 (75.0%) |
Yes | 9 (45.0%) | 3 (23.1%) | 5 (25.0%) |
Tumor size (cm) | 7.60±3.67 | 6.58±4.86 | 6.17±3.81 |
ICG (%) | 5.36±3.06 | 5.35±3.39 | 5.97±3.29 |
BCLC stage | |||
A | 12 (60.0%) | 10 (76.9%) | 11 (55.0%) |
B | 8 (40.0%) | 3 (23.1%) | 9 (45.0%) |
Results are means ± standard deviation/n (%). Y.AG, <45 years, n=20; M.AG, 45–65 years, n=13; O.AG, >65 years, n=20. Y.AG, younger age group; M.AG, middle age group; O.AG, older age group; AFP, alpha fetoprotein; ALB, albumin; ALT, alanine aminotransferase; AST, aspartate aminotransferase; BCLC stage, Barcelona Clinic Liver Cancer stage; BMI, body mass index; BUN, blood urea nitrogen; C3, complement factors C3; C4, complement factors C4; CK19, cytokeratin-19-fragment; Cr, serum creatinine; γ-GGT, gamma-glutamyl transferase; HDL, high-density lipoprotein; HGB, hemoglobin; IgA, immunoglobulin A; ICG, constitutional indocyanine green; IgG, immunoglobulin G; IgM, immunoglobulin M; INR, international normalized ratio; Ki67, Ki-67; LDL, low-density lipoprotein; PA, prealbumin; PLT, platelet; PT, prothrombin time; P53, Tumor suppressor p53;TBIL, total bilirubin; TCH, total cholesterol; Th/Ts, helper T cell/suppressor T cell; TRF, transferrin; WBC, white blood cell count.
Table 2
Characteristic | Y.AG (n=20) | O.AG (n=20) | P value |
---|---|---|---|
Age (years) | 42.25±4.05 | 67.00±3.03 | <0.0001 |
Sex | >0.999 | ||
Female | 3 (15.0%) | 2 (10.0%) | |
Male | 17 (85.0%) | 18 (90.0%) | |
BMI (kg/m2) | 22.70±2.75 | 23.59±2.34 | 0.102 |
WBC (109/L) | 6.19±2.05 | 5.78±1.46 | 0.495 |
HGB (g/L) | 135.65±19.53 | 143.80±14.81 | 0.242 |
PLT (109/L) | 221.55±81.53 | 200.80±77.81 | 0.327 |
TBIL (μmol/L) | 15.22±6.36 | 13.79±4.52 | 0.565 |
ALB (g/L) | 37.22±3.78 | 37.30±3.66 | >0.999 |
TRF (g/L) | 2.52±0.77 | 2.47±0.48 | 0.369 |
PA (mg/L) | 188.88±50.10 | 210.12±56.75 | 0.289 |
ALT (U/L) | 40.50±24.65 | 42.70±26.24 | 0.820 |
AST (U/L) | 48.90±27.82 | 43.10±25.25 | 0.512 |
γ-GGT (U/L) | 73.5±47.04 | 75.65±49.32 | 0.968 |
BUN (mg/dL) | 5.08±1.49 | 4.82±0.80 | 0.414 |
Cr (μmol/L) | 72.90±13.94 | 76.80±12.41 | 0.221 |
HBV-DNA (/mL) | 499,420.15±913,807.00 | 4,289,988.24±12,362,851.16 | 0.602 |
HBsAg (ng/mL) | 674.77±299.15 | 536.79±305.57 | 0.108 |
PT (s) | 12.83±1.45 | 12.50±1.67 | 0.369 |
INR | 1.05±0.12 | 1.02±0.14 | 0.341 |
AFP (ng/mL) | 12,838.96±25,008.58 | 391.78±1,106.39 | 0.004 |
TCH (mmol/L) | 4.45±1.08 | 4.78±1.07 | 0.429 |
HDL (mmol/L) | 1.19±0.28 | 1.11±0.27 | 0.355 |
LDL (mmol/L) | 3.19±1.01 | 3.50±0.97 | 0.445 |
Th/Ts (%) | 1.91±0.70 | 1.50±0.53 | 0.046 |
IgG (g/L) | 13.79±3.63 | 15.82±2.87 | 0.020 |
IgM (g/L) | 1.26±0.45 | 1.42±0.45 | 0.134 |
IgA (g/L) | 2.64±1.15 | 2.80±1.3 | 0.602 |
C3 (g/L) | 0.85±0.17 | 0.98±1.31 | 0.038 |
C4 (g/L) | 0.23±0.14 | 0.23±0.07 | 0.529 |
CK19 (%) | 12.10±15.43 | 0.35±1.18 | 0.003 |
P53 (%) | 51.00±23.37 | 22.60±34.21 | 0.004 |
Ki67 (%) | 42.00±23.81 | 27.25±14.09 | 0.049 |
Ascites | >0.999 | ||
No | 18 (90.0%) | 19 (95.0%) | |
Yes | 2 (10.0%) | 1 (5.0%) | |
Smoking | >0.999 | ||
No | 15 (66.7%) | 16 (66.7%) | |
Yes | 5 (33.3%) | 4 (33.3%) | |
Drinking | >0.999 | ||
No | 18 (90.0%) | 16 (80.0%) | |
Yes | 2 (10.0%) | 4 (20.0%) | |
Portal hypertension (mmHg) | >0.999 | ||
No | 11 (55.0%) | 15 (75.0%) | |
Yes | 9 (45.0%) | 5 (25.0%) | |
Tumor size (cm) | 7.60±3.67 | 6.17±3.81 | 0.192 |
ICG (%) | 5.36±3.06 | 5.97±3.29 | 0.478 |
BCLC stage | >0.999 | ||
A | 12 (60.0%) | 11 (55.0%) | |
B | 8 (40.0%) | 9 (45.0%) |
Results are means ± standard deviation/n (%). P value was based on Fisher’s exact test and Mann-Whitney U-test. Y.AG, <45 years, n=20; O.AG, >65 years, n=20. Y.AG, younger age group; O.AG, older age group; AFP, alpha fetoprotein; ALB, albumin; ALT, alanine aminotransferase; AST, aspartate aminotransferase; BCLC stage, Barcelona Clinic Liver Cancer stage; BMI, body mass index; BUN, blood urea nitrogen; C3, complement factors C3; C4, complement factors C4; CK19, cytokeratin-19-fragment; HBV, hepatitis B virus; Cr, serum creatinine; HBV, hepatitis B virus; γ-GGT, gamma-glutamyl transferase; HDL, high-density lipoprotein; HGB, hemoglobin; IgA, immunoglobulin A; ICG, constitutional indocyanine green; IgG, immunoglobulin G; IgM, immunoglobulin M; INR, international normalized ratio; Ki67, Ki-67; LDL, low-density lipoprotein; PA, prealbumin; PLT, platelet; PT, prothrombin time; P53, Tumor suppressor p53; TBIL, total bilirubin; TCH, total cholesterol; Th/Ts, helper T cell/suppressor T cell; TRF, transferrin; WBC, white blood cell count.
Operational taxonomic units and α- and β-diversities
Different microbial taxonomies were reflected by the OTUs. In the present study, 1,428 OTUs were observed in the Y.AG versus the M.AG, 1,371 OTUs in the M.AG versus the O.AG, and 1,236 OTUs in the Y.AG versus the O.AG. A total of 1,038 OTUs were shared among the three groups (Figure 1A). α-Diversity analysis with ACE Index, chao1 Index, observed_species, and goods_coverage revealed that gut microbial community richness was significantly increased in the O.AG compared with the Y.AG (P=0.017, P=0.031, P=0.011, and P=0.041, respectively) (Figure 1B). We used NMDS to evaluate differences in β-diversity between groups. The findings in the three groups following NMDS are shown in Figure 2A (a). Gut microbiota of Y.AG was distinct from that of M.AG (stress =0.090 at the genus level). Patients in the O.AG compared with the M.AG and Patients in the Y.AG compared with O.AG, as measured by NMDS, stress =0.095 and stress =0.099 at the genus level respectively [Figure 2A (b-d)].
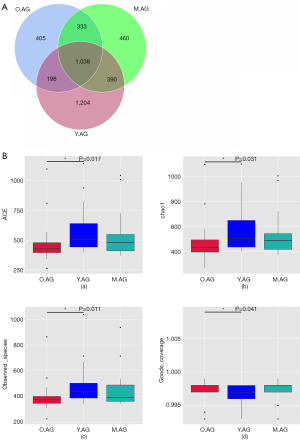
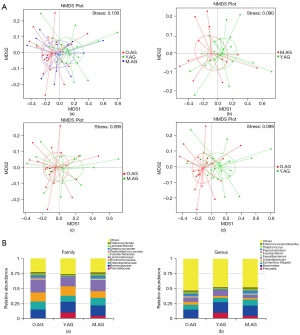
Microbial taxa signatures
Results of the statistical analysis for the top 10 bacterial abundances, which belong to the dominant core taxa at the phylum, class, order, family, and genus levels in taxonomy, between the Y.AG, the M.AG, and the O.AG are shown in Figure S1 and Figure 2B. The family bar chart demonstrated that Prevotellaceae, Bacteroidaceae, Enterobacteriaceae, Ruminococcaceae, and Lachnospiraceae were the 5 underlying bacteria in the three groups, and the abundance of Prevotellaceae was significantly reduced in the O.AG compared with the other two groups (Figure 2B). At the genus level, the dominant bacteria in the Y.AG, the M.AG, and the O.AG were found to be similar, with some proportional differences. Analysis of the relative abundance of bacterial taxonomic groups at the genus level showed that the 10 most abundant genera were Prevotella (mean relative abundance: Y.AG, 9.31%; M.AG, 4.25%; O.AG, 0.26%), Bacteroides (mean relative abundance: Y.AG, 18.05%; M.AG, 17.38%; O.AG, 14.58%), Escherichia-Shigella (mean relative abundance: Y.AG, 7.21%; M.AG, 10.08%; O.AG, 8.25%), Subdoligranulum (mean relative abundance: Y.AG, 3.23%; M.AG, 2.76%; O.AG, 7.39%), Faecalibacterium (mean relative abundance: Y.AG, 10.11%; M.AG, 5.48%; O.AG, 4.69%), Fusobacterium (mean relative abundance: Y.AG, 0.24%; M.AG, 1.97%; O.AG, 1.46%), Peptoclostridium (mean relative abundance: Y.AG, <0.1%; M.AG, <0.1%; O.AG, 1.05%), Streptococcus (mean relative abundance: Y.AG, 1.23%; M.AG, 5.10%; O.AG, 4.12%), Lactobacillus (mean relative abundance: Y.AG, 1.53%; M.AG, 2.27%; O.AG, 2.71%), and Enterococcus (mean relative abundance: Y.AG, 1.83%; M.AG, 3.15%; O.AG, 2.50%).
Taxa with <0.01% relative abundance were considered l-abundance taxa (abundance <0.0001). LEfSe analysis results indicated a significant difference in gut microbiota between the Y.AG and the M.AG, between the M.AG and the O.AG, and particularly between the Y.AG and the O.AG. At the genus level, the relative abundances of Prevotella, UCG-002, and Phascolarctobacterium were higher in the Y.AG than in the M.AG. The relative abundances of Gordonibacter, Neisseria, Parablastomonas, and Acidaminococcus (abundance <0.0001) were higher in the M.AG than in the Y.AG (Figure 3A). In addition, 3 genera (Citrobacter, Erysipelotrichaceae_UCG-003, and Clostridium_sensu_stricto_1) were significantly enriched in the O.AG (Figure 3B). Seven genera [Streptococcus, Blautia, Neisseria (abundance <0.0001), Erysipelotrichaceae_UCG-003, Terrisporobacter, Anaerostipes, and Fusicatenibacter] were significantly enriched in the O.AG. Eleven genera (Prevotella, Coprobacter, and Phascolarctobacterium; and low-abundance taxa, including Hydrogenoanaerobacterium, Paludicola, Constrictibacter, Caulobacter, Ruminiclostridium, Proteiniphilum, Allorhizobium-Neorhizobium-Pararhizobium-Rhizobium, and Fenollaria) were significantly enriched in the Y.AG (Figure 3C).
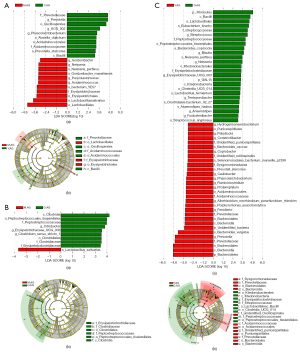
Correlation between gut microbiota and environmental factors
To investigate the associations between gut microbiota and HBV-related HCC clinical status, 7 environmental factors [AFP, Th/Ts, immunoglobulin G (IgG), C3, CK19, p53, and Ki67] of the relevant clinical and different community compositions were analyzed using RDA in the Y.AG versus the M.AG, the M.AG versus the O.AG, and the Y.AG versus the O.AG. The RDA axes 1 and 2 accounted for 37.19% and 29.61%, respectively, of the total variation of bacteria in the Y.AG versus the M.AG. According to the permutation test (P=0.112), the difference in gut microbiota was significantly influenced by C3 (P=0.001), p53 (P=0.005), and Ki67 (P=0.017) compared with other indices (Table 3). Among them, p53 and Ki67 were positively correlated with Y.AG-enriched genera (Prevotella: P<0.001 and P=0.020; Phascolarctobacterium: P=0.016 and P=0.025, respectively), whereas Th/Ts was negatively correlated with Phascolarctobacterium (P=0.049). Additionally, p53 and CK19 were positively correlated with M.AG-enriched genera (Acidaminococcus: P<0.001 and P<0.001, respectively) (Figure 4A). In the M.AG versus the O.AG, the RDA axes 1 and 2 accounted for 69.58% and 25.11%, respectively, of the total variation. According to the permutation test (P=0.057), the difference in gut microbiota was significantly influenced by CK19 (P=0.025) compared with the other indices (Table 4). IgG was positively correlated with O.AG-enriched genera (Citrobacter: P=0.013), and Ki67 was negatively correlated with Erysipelotrichaceae_UCG-003 (P=0.021) (Figure 4B). In the Y.AG versus the O.AG, the RDA axes 1 and 2 accounted for 43.56% and 20.35%, respectively, of the total variation. According to the permutation test (P=0.041), the difference in gut microbiota was significantly influenced by AFP (P=0.003), Th/Ts (P=0.002), C3 (P=0.001), and Ki67 (P=0.034) compared with the other indices (Table 5). In the O.AG, the Ki67 level was negatively correlated with Streptococcus (P=0.016) and Erysipelotrichaceae_UCG-003 (P=0.003); the AFP level was negatively correlated with Fusicatenibacter (P=0.048) and Erysipelotrichaceae_UCG-003 (P=0.008); Blautia was positively associated with C3 (P=0.010) and negatively associated with Th/Ts (P=0.032); and CK19 and p53 levels were negatively correlated with Anaerostipes (P=0.040 and P=0.024, respectively). In the Y.AG, the Ki67 level was positively associated with Prevotella (P=0.010), Coprobacter (P=0.029), Phascolarctobacterium (P=0.028), and Constrictibacter (P=0.045); the p53 level was positively associated with Prevotella (P<0.001) and Phascolarctobacterium (P=0.030); the AFP level was positively correlated with Prevotella (P=0.005) and Allorhizobium-Neorhizobium-Pararhizobium-Rhizobium (P=0.014); the Th/Ts level was positively correlated with Hydrogenoanaerobacterium (P=0.014), Allorhizobium-Neorhizobium-Pararhizobium-Rhizobium (P=0.044), and Fenollaria (P=0.017); the C3 level was negatively correlated with Hydrogenoanaerobacterium (P=0.045) and Proteiniphilum (P=0.015); and the CK19 level was positively correlated with Constrictibacter (P=0.002), Caulobacter (P=0.041), and Proteiniphilum (P=0.006) (Figure 4C).
Table 3
Variable | r2 | P value |
---|---|---|
AFP | 0.135 | 0.139 |
Th/Ts | 0.084 | 0.267 |
IgG | 0.037 | 0.550 |
C3 | 0.438 | 0.001 |
CK19 | 0.016 | 0.699 |
P53 | 0.273 | 0.005 |
Ki67 | 0.226 | 0.017 |
AFP, alpha fetoprotein; C3, complement factors C3; CK19, cytokeratin-19-fragment; IgG, immunoglobulin G; Ki67, Ki-67; P53, Tumor suppressor p53; Th/Ts, helper T cell/suppressor T cell.
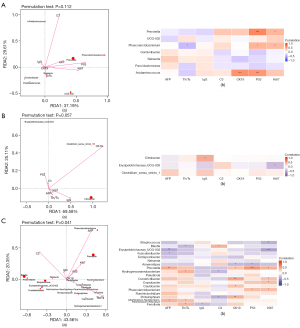
Table 4
Variable | r2 | P value |
---|---|---|
AFP | 0.025 | 0.665 |
Th/Ts | 0.031 | 0.635 |
IgG | 0.134 | 0.120 |
C3 | 0.018 | 0.763 |
CK19 | 0.349 | 0.025 |
P53 | 0.053 | 0.443 |
Ki67 | 0.031 | 0.614 |
AFP, alpha fetoprotein; C3, complement factors C3; CK19, cytokeratin-19-fragment; IgG, immunoglobulin G; Ki67, Ki-67; P53, tumor suppressor p53; Th/Ts, helper T cell/suppressor T cell.
Table 5
Variable | r2 | P value |
---|---|---|
AFP | 0.459 | 0.003 |
Th/Ts | 0.326 | 0.002 |
IgG | 0.066 | 0.292 |
C3 | 0.311 | 0.001 |
CK19 | 0.015 | 0.743 |
P53 | 0.111 | 0.106 |
Ki67 | 0.166 | 0.034 |
AFP, alpha fetoprotein; C3, complement factors C3; CK19, cytokeratin-19-fragment; IgG, immunoglobulin G; Ki67, Ki-67; P53, tumor suppressor p53; Th/Ts, helper T cell/suppressor T cell.
Functional signatures of gut microbiota
The functional capacity of gut microbiota of the Y.AG versus the M.AG, the M.AG versus the O.AG, and the Y.AG versus the O.AG was predicted by PICRUSt2 analysis, which found 16, 8, and 72 pathways, respectively, related to differential gut microbiome at the genus level (Figure 5, Figure S2, Tables S3,S4, Table 6). In the Y.AG versus the M.AG, microbial metabolic pathways included pantothenate biosynthesis, coenzyme biosynthesis, methionine biosynthesis, thiamin biosynthesis, and pyrimidine metabolism pathways. In the M.AG versus the O.AG, metabolic pathways mainly included glycerol degradation. In the Y.AG versus the O.AG, microbial metabolic pathways mainly included pyrimidine metabolism pathways, purine biosynthesis, fatty acid metabolism pathways, pantothenate metabolism pathways, coenzyme biosynthesis, glycerol degradation, tyrosine biosynthesis, phenylalanine biosynthesis, threonine metabolism pathways, lysine metabolism pathways, methionine biosynthesis, aspartate pathway, arginine biosynthesis, acetylneuraminate degradation, palmitate biosynthesis, oleate biosynthesis, stearate biosynthesis, thiamin biosynthesis, gluconeogenesis, tricarboxylic acid cycle (TCA), and inositol degradation. Prevotella was associated with most Y.AG versus M.AG-enriched functional modules, suggesting their core role in the Y.AG versus the M.AG; UCG-002 and Phascolarctobacterium played a promoting role in pantothenate biosynthesis; and low-abundance taxa (Gordonibacter and Acidaminococcus) had complementary roles in coenzyme biosynthesis (Figure 6A). In the M.AG versus the O.AG, gut microbiota and functional module correlations were not significant (Figure 6B). In the Y.AG versus the O.AG, O.AG-enriched genera were associated with the majority of Y.AG versus O.AG-enriched functional modules, suggesting their core role in the Y.AG versus the O.AG. In Y.AG-enriched genera, among the relatively high-abundance taxa (Prevotella, Coprobacter, and Phascolarctobacterium), the core role of Prevotella was mainly in the functional modules. Essential amino acids (lysine, threonine, and methionine), metabolism pathways, and aspartate superpathway were negatively correlated with the functional modules of Coprobacter. The TCA cycle, arginine biosynthesis, fatty acid metabolism pathways, gluconeogenesis, thiamin biosynthesis, pyrimidine metabolism pathways, palmitate biosynthesis, oleate biosynthesis, stearate biosynthesis, and coenzyme biosynthesis were positively correlated with Coprobacter. Palmitate biosynthesis and pyrimidine metabolism pathways were positively correlated with Phascolarctobacterium. Other low-abundance taxa also showed differences among the main predicted functional modules (Figure 6C). These findings indicate that differences in gut bacteria can have different roles in the body.
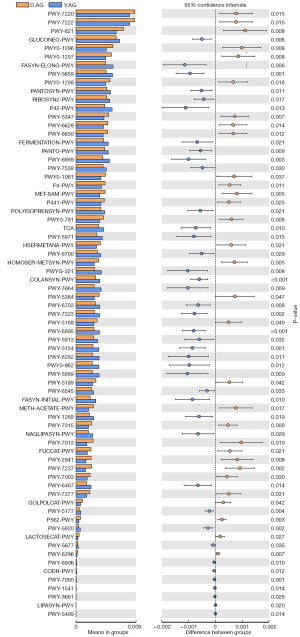
Table 6
Pathway ID | Altered pathway | P value |
---|---|---|
PWY-7220 | Adenosine deoxyribonucleotides de novo biosynthesis II | 0.015 |
PWY-7222 | Guanosine deoxyribonucleotides de novo biosynthesis II | 0.015 |
PWY-621 | Sucrose degradation III (sucrose invertase) | 0.008 |
GLUCONEO-PWY | Gluconeogenesis I | 0.006 |
PWY0-1296 | Purine ribonucleosides degradation | 0.008 |
PWY0-1297 | Superpathway of purine deoxyribonucleosides degradation | 0.008 |
FASYN-ELONG-PWY | Fatty acid elongation-saturated | 0.006 |
PWY-5659 | GDP-mannose biosynthesis | 0.001 |
PWY0-1298 | Superpathway of pyrimidine deoxyribonucleosides degradation | 0.018 |
PANTOSYN-PWY | Pantothenate and coenzyme A biosynthesis I | 0.011 |
RIBOSYN2-PWY | Flavin biosynthesis I (bacteria and plants) | 0.017 |
P42-PWY | Incomplete reductive TCA cycle | 0.013 |
PWY-5347 | Superpathway of L-methionine biosynthesis (transsulfuration) | 0.007 |
PWY-6628 | Superpathway of L-phenylalanine biosynthesis | 0.014 |
PWY-6630 | Superpathway of L-tyrosine biosynthesis | 0.012 |
FERMENTATION-PWY | Mixed acid fermentation | 0.021 |
PANTO-PWY | Phosphopantothenate biosynthesis I | 0.009 |
PWY-6969 | TCA cycle V (2-oxoglutarate: ferredoxin oxidoreductase) | 0.003 |
PWY-7539 | 6-Hydroxymethyl-dihydropterin diphosphate biosynthesis III (Chlamydia) | 0.030 |
PWY0-1061 | Superpathway of L-alanine biosynthesis | 0.037 |
P4-PWY | Superpathway of L-lysine, L-threonine and L-methionine biosynthesis I | 0.011 |
MET-SAM-PWY | Superpathway of S-adenosyl-L-methionine biosynthesis | 0.005 |
P441-PWY | Superpathway of N-acetylneuraminate degradation | 0.025 |
POLYISOPRENSYN-PWY | Polyisoprenoid biosynthesis (Escherichia coli) | 0.021 |
PWY0-781 | Aspartate superpathway | 0.008 |
TCA | TCA cycle I (prokaryotic) | 0.010 |
PWY-5971 | Palmitate biosynthesis II (bacteria and plants) | 0.015 |
HSERMETANA-PWY | L-methionine biosynthesis III | 0.031 |
PWY-6700 | Queuosine biosynthesis | 0.029 |
HOMOSER-METSYN-PWY | L-methionine biosynthesis I | 0.005 |
PWYG-321 | Mycolate biosynthesis | 0.008 |
COLANSYN-PWY | Colanic acid building blocks biosynthesis | 0.001 |
PWY-7664 | Oleate biosynthesis IV (anaerobic) | 0.009 |
PWY-5384 | Sucrose degradation IV (sucrose phosphorylase) | 0.047 |
PWY-6703 | Preq0 biosynthesis | 0.006 |
PWY-7323 | Superpathway of GDP-mannose-derived O-antigen building blocks biosynthesis | 0.002 |
PWY-5188 | Tetrapyrrole biosynthesis I (from glutamate) | 0.049 |
PWY-6895 | Superpathway of thiamin diphosphate biosynthesis II | 0.001 |
PWY-5913 | TCA cycle VI (obligate autotrophs) | 0.032 |
PWY-5154 | L-arginine biosynthesis III (via N-acetyl-L-citrulline) | 0.001 |
PWY-6282 | Palmitoleate biosynthesis I (from [5Z]-dodec-5-enoate) | 0.011 |
PWY0-862 | (5Z)-dodec-5-enoate biosynthesis | 0.012 |
PWY-5989 | Stearate biosynthesis II (bacteria and plants) | 0.009 |
PWY-5189 | Tetrapyrrole biosynthesis II (from glycine) | 0.042 |
PWY-6545 | Pyrimidine deoxyribonucleotides de novo biosynthesis III | 0.033 |
FASYN-INITIAL-PWY | Superpathway of fatty acid biosynthesis initiation (Escherichia coli) | 0.010 |
METH-ACETATE-PWY | Methanogenesis from acetate | 0.017 |
PWY-1269 | CMP-3-deoxy-D-manno-octulosonate biosynthesis I | 0.019 |
PWY-7315 | Dtdp-N-acetylthomosamine biosynthesis | 0.009 |
NAGLIPASYN-PWY | Lipid IVA biosynthesis | 0.029 |
PWY-7013 | L-1,2-propanediol degradation | 0.019 |
FUCCAT-PWY | Fucose degradation | 0.021 |
PWY-2941 | L-lysine biosynthesis II | 0.008 |
PWY-7237 | Myo-, chiro-, and scillo-inositol degradation | 0.002 |
PWY-7003 | Glycerol degradation to butanol | 0.030 |
PWY-6467 | Kdo transfer to lipid IVA III (chlamydia) | 0.014 |
PWY-7377 | Cob(II)yrinate a,c-diamide biosynthesis I (early cobalt insertion) | 0.031 |
GOLPDLCAT-PWY | Superpathway of glycerol degradation to 1,3-propanediol | 0.042 |
PWY-5177 | Glutaryl-CoA degradation | 0.004 |
P562-PWY | Myo-inositol degradation I | 0.003 |
PWY-5920 | Superpathway of heme biosynthesis from glycine | 0.002 |
LACTOSECAT-PWY | Lactose and galactose degradation I | 0.027 |
PWY-5677 | Succinate fermentation to butanoate | 0.035 |
PWY-6396 | Superpathway of 2,3-butanediol biosynthesis | 0.007 |
PWY-6906 | Chitin derivatives degradation | 0.010 |
CODH-PWY | Reductive acetyl coenzyme A pathway | 0.012 |
PWY-7090 | UDP-2,3-diacetamido-2,3-dideoxy-alpha-D-mannuronate biosynthesis | 0.001 |
PWY-1541 | Superpathway of taurine degradation | 0.014 |
PWY-3661 | Glycine betaine degradation I | 0.028 |
LIPASYN-PWY | Phospholipases | 0.020 |
PWY-5499 | Vitamin B6 degradation | 0.014 |
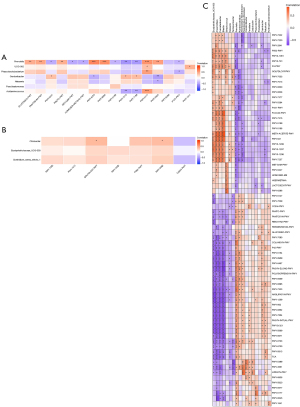
Discussion
Gut microbiome can have a significant impact on human health and multiple diseases, including cancer, and the development of some tumors has been found to be closely related to age (47). Radical resection of liver cancer remains the preferred treatment modality for HCC (9). In the present study, we found that the age of patients with HCC is also becoming increasingly younger, and prognosis is usually relatively poor (39). Moreover, older age can increase risks of treatment-related worse outcomes and complications (48). Su et al. pointed that age is a risk factor to determine the prognosis of patients with HCC who have received surgical resection (14). Therefore, understanding the characterization and function of gut microbiota at different ages is important to explore to treatment strategies of HCC on different age groups. In our study, 16S rRNA gene sequencing was used to identify differential gut microbiota in the Y.AG versus the M.AG, the M.AG versus the O.AG, and the Y.AG versus the O.AG, and we found that the difference in gut microbiota was most prominent when comparing the Y.AG to the O.AG. Moreover, we found that changes in gut microbiota in different age groups were related to immune response-related factors and prognostically relevant tumor factors. We also found that alteration of microbiota was correlated with immune-related functions and prognosis-related metabolic Functions of gut microbiota. Therefore, the characteristic Changes of the gut microbiota may provide potential targets for exploring the treatment of HCC at different ages.
In the analysis of α- and β-diversities, we found that the gut microbial structure and the relative abundance in the Y.AG versus the O.AG greatly changed at the genus level; however, in the other two groups, only the relative abundance of gut microbes showed differences. The findings indicated that the gut bacterial composition was significantly altered in the Y.AG compared with the O.AG. The overall gut microbiota species decreased in the M.AG and the O.AG compared with the Y.AG. Reduction of the diversity of gut microbiota was induced in a micro-inflammatory state and accelerated the micro-ecological imbalance (49). This could directly affect the function of intestinal microbes and abnormal liver-related function, which could result in a discrepancy in treatment outcomes (50,51).
In a previous study, usually a higher abundance of the flora became a molecular marker (3). Species with abundance <0.01% (outside the scope of the most dominant core taxa) were clustered together and were not as influential as the higher abundance species (52). In addition, we found gut microbiota differentiation of the Y.AG versus the M.AG, the M.AG versus the O.AG, and particularly the Y.AG versus the O.AG. Prevotella, UCG-002, and Phascolarctobacterium in the Y.AG versus the M.AG; Citrobacter, Erysipelotrichaceae_UCG-003, and Clostridium_sensu_stricto_1 in the M.AG versus the O.AG; and Streptococcus, Blautia, Erysipelotrichaceae_UCG-003, Terrisporobacter, Anaerostipes, Fusicatenibacter, Prevotella, Coprobacter, and Phascolarctobacterium in the Y.AG versus the O.AG were highly abundant taxa. For pairwise comparison, the abundances of Prevotella and Phascolarctobacterium increased in the Y.AG, and that of Erysipelotrichaceae_UCG-003 increased in the O.AG. These findings indicate that some characteristic bacterial genera could play vital roles in the different age groups of HBV-HCC.
Evaluation of gut microbiota can be useful for the guidance of a new HBV- HCC treatment method (53). Previous studies have indicated that there is a strong association between the liver, that is, the microbiota-liver axis, and gut microbiota (54,55). Colonization of gut microbiota promotes the formation of intestinal mucosa and in turn affects inflammatory reaction, immune function, and immune cytokine levels, so the change in gut microbiota is correlated with changes in immune function (56). Changes in the composition of intestinal microflora are also related to the poor prognosis of cancer (57). In previous research, AFP and IgG antibodies were found to be specific tumor markers in HCC (58). Furthermore, injury of the host immune function is mainly reflected by the change in the Ts and Th cells (59), and the Th/Ts cell ratio has been used to show the surveillance role of natural killer cells (60). C3 shows immunoregulatory and pro-inflammatory actions (61). CK19 promotes tumor metastasis and migration through the immune response and damages the extracellular matrix (62). Inhibition of p53 protein function is correlated with the growth of liver cancer and poor prognosis (63). Ki67 has a remarkable value in providing evidence of prognostic value caused by tumor growth and proliferation (64). To investigate the correlation between gut microbiota and environmental factors, we evaluated AFP, p53, Ki67, CK19, Th/Ts, C3, and IgG. We found that individual environmental factors, including C3, p53, and Ki67 (in the Y.AG versus the M.AG) and CK19 (in the M.AG versus the O.AG) had a significant impact on the microbial community. However, the collective efficacy of all environmental factors did not have a significant effect in the Y.AG versus the M.AG and the M.AG versus the O.AG. Our research also found that the prognostic relative factors were more likely to affect high-abundance taxa in the Y.AG versus the M.AG. In the M.AG versus the O.AG, although the prognostic factor (CK19) affected the difference in gut microbiota, the correlation between this and the main flora was not significant; therefore, it did not have a significant effect. It is noteworthy that the collective efficacy of all environmental factors was significant in the Y.AG versus the O.AG. The key to the central role of environmental factors lies in AFP, Th/Ts, C3, and Ki67. A tumor-specific marker (AFP) and a relative marker of tumor cell proliferation (Ki67) were significantly positively correlated with gut microbiota in the Y.AG, but negatively correlated with gut microbiota in the O.AG. A previous study has reported that, compared with elderly patients, young adult patients with HCC had a significantly higher AFP level (43). In a previously published study, it was found that, compared with elderly patients, young patients have more aggressive tumors and greater proliferation (65). These findings are consistent with the results of the present study. Among them, C3 showed strong pro-inflammation, and the tumor microenvironment of pro-inflammatory state can promote proliferation and invasion of the tumor cells (66,67). The decreased activity of Ts and Th cells is reflected in immune function injury (59). Furthermore, improvement of liver function reduces the expression of pro-inflammatory factors and enhances immune function (68). In the present study, C3 was negatively correlated with intestinal flora of the Y.AG (good liver function), but positively correlated in the O.AG (poor liver function). The situation was opposite for Th/Ts. These results are consistent with those of a previously published study, which found that younger patients had better liver functional reserve, but more aggressive tumors, than elderly patients (14).
PICRUSt2 analysis revealed significant differences in microbiome function in the Y.AG versus the M.AG, the M.AG versus the O.AG, and particularly the Y.AG versus the O.AG. All core functions were also found to be more significant in the Y.AG versus the O.AG compared with the M.AG versus the O.AG. Therefore, we speculate that this could be a manifestation of the impact of the more significant age gap in the Y.AG versus the O.AG. Furthermore, in the Y.AG versus the O.AG, we found that the O.AG presented in a hypermetabolic state, whereas the Y.AG presented in the opposite state; the same metabolic functional modules occurred, resulting in opposite effects in the Y.AG and the O.AG. Previous studies indicated that the reciprocal regulation of metabolic reprogramming-induced metabolic alterations in cancer cells and host immune cells and microbiota is a vital mechanism to regulate cancer progression (e.g., proliferation changes in cancer cells and malignant progression) (69,70). Streptococcus, Blautia, Erysipelotrichaceae_UCG-003, Terrisporobacter, Anaerostipes, and Fusicatenibacter represented the major variances in O.AG microbiomes. Studies have reported inhibiting purine biosynthesis to produce immunosuppressive effects (71), that pyrimidine metabolism can follow a similar pattern to that of purines by metabolic reprogramming, and that a highly expressed pyrimidine metabolic rate facilitates a decrease in cell proliferation and changes in immune cell responses (72). Methionine, threonine, and lysine comprise the family of essential amino acids synthesized from aspartate via the aspartate pathway. Deficiency of lysine, threonine, and methionine impairs host immune responses (including the human intestines), and in anticancer therapy, increased aspartate can also impair immune cells (lymphoblasts) (73-76). Phenylalanine can be metabolized to tyrosine in the liver, and tyrphostins targeted in therapy for HCC are protein tyrosine kinase inhibitors that decrease the proliferation of tumor cells (77,78). Furthermore, ceramide is decreased in cancer cells and induces apoptosis and decreases proliferation (79). Additionally, glycerol and inositol can take part in the glycolysis pathway. It was previously reported that defective glycerol metabolism has reduced metabolic function, and downregulating glycolytic flux suppresses HCC cell proliferation (80,81). The metabolic abilities described above were positively correlated with gut microbiota in the O.AG and increased during HCC; they all played a role in inhibiting proliferation. The functions of Prevotella were negatively correlated with these pathways in the Y.AG, indicating that the ability of tumor proliferation to decrease could also be affected by intestinal flora in elderly patients. This finding is consistent with the observation that the prognosis of elderly patients with liver cancer is better than that of young patients (82).
Furthermore, pantothenic acid is a precursor of coenzymes, and coenzyme biosynthesis is involved in fatty acid synthesis. Inhibition of the fatty acid biosynthesis pathway in tumor cells rapidly inhibits the proliferation of cancer cells (83-85). The findings of our study indicated that the function of fatty acid metabolism pathways was significantly reduced to decrease tumor proliferation in O.AG microbiomes. Overexposure to palmitoleate reduces cell regeneration (86). Palmitoleate can also further elongate into oleate and stearic acids (87). In the present study, stearate biosynthesis and palmitoleate biosynthesis were significantly decreased to enhance cell regeneration in the O.AG. Thiamin, which serves as an enzymatic cofactor for branched-chain amino-acid biosynthesis, participates in protecting hepatocytes (88); arginine, a branched-chain amino acid, contributes to gluconeogenesis (89). Attenuation of gluconeogenesis is considered to reduce HCC development (90). Furthermore, cancer cells do not rely on the TCA to produce nicotinamide adenine dinucleotide (NADH), while normal cells completely depend on the TCA for NADH production to promote cell function (91). In the present study, significant attenuation in thiamin biosynthesis, arginine metabolism, gluconeogenesis, and the TCA was associated with liver cell damage in the O.AG. Additionally, the metabolic pathways described above were positively correlated with gut microbiota of the Y.AG (Prevotella and Coprobacter) during HCC to protect the liver. In the Y.AG, Phascolarctobacterium also played a role in protecting liver function and promoting tumor cell proliferation by palmitate biosynthesis and pyrimidine metabolism pathways, which could explain why liver function in the O.AG was poor but the prognosis was good compared with the Y.AG (65). Overall, the findings of the present study reveal the unique gut microbiota profile during different ages by using non-invasive biomarkers for HCC, which could help in the future remission of HBV-HCC with age-related gut microbiota.
The present study has some limitations. First, our results require a further extensive cohort. Second, we only used 16S rRNA gene sequencing data; therefore, metagenomics should be used for further investigation and functional analysis. Finally, the study only identified associations between different ages and gut microbiome, without providing causality.
Conclusions
To the best of our knowledge, this is the first study of the relationship of age factor to its effect on gut microbiome in HBV-HCC. We analyzed changes in the composition and diversity of gut bacteria in patients of different ages and identified specific microbiotas that could be used as diagnostic biomarkers for patients of different ages, with a focus on young and elderly individuals. The findings of the present study could assist in the development of novel strategies for HCC that have been broken through the treatment bottleneck caused by age.
Acknowledgments
Funding: This study was supported by the National Nature Science Foundation of China (grant No. 81960534 and No. 81972306), the Key Laboratory of High-Incidence Tumor Prevention & Treatment (Guangxi Medical University), Ministry of Education (No. GKE-ZZ202008), and the Innovation Project of Guangxi Graduate Education (grant No. YCSW2021140).
Footnote
Reporting Checklist: The authors have completed the STROBE reporting checklist. Available at https://atm.amegroups.com/article/view/10.21037/atm-22-1572/rc
Data Sharing Statement: Available at https://atm.amegroups.com/article/view/10.21037/atm-22-1572/dss
Conflicts of Interest: All authors have completed the ICMJE uniform disclosure form (available at https://atm.amegroups.com/article/view/10.21037/atm-22-1572/coif). The authors have no conflicts of interest to declare.
Ethical Statement: The authors are accountable for all aspects of the work in ensuring that questions related to the accuracy or integrity of any part of the work are appropriately investigated and resolved. The study followed the ethical guidelines of the Helsinki Declaration (as revised in 2013). The protocol for this study was approved by the ethics committee of the Guangxi Medical University Cancer Hospital (No. LW2022058). All patients signed informed consent forms and agreed to their anthropometric data being used in the analysis.
Open Access Statement: This is an Open Access article distributed in accordance with the Creative Commons Attribution-NonCommercial-NoDerivs 4.0 International License (CC BY-NC-ND 4.0), which permits the non-commercial replication and distribution of the article with the strict proviso that no changes or edits are made and the original work is properly cited (including links to both the formal publication through the relevant DOI and the license). See: https://creativecommons.org/licenses/by-nc-nd/4.0/.
References
- Park J, Jung J, Kim D, et al. Long-term outcomes of the 2-week schedule of hypofractionated radiotherapy for recurrent hepatocellular carcinoma. BMC Cancer 2018;18:1040. [Crossref] [PubMed]
- Zheng L, Liang P, Li J, et al. ShRNA-targeted COMMD7 suppresses hepatocellular carcinoma growth. PLoS One 2012;7:e45412. [Crossref] [PubMed]
- Yang XA, Lv F, Wang R, et al. Potential role of intestinal microflora in disease progression among patients with different stages of Hepatitis B. Gut Pathog 2020;12:50. [Crossref] [PubMed]
- Sun Y, Li SH, Cheng JW, et al. Downregulation of miRNA-205 Expression and Biological Mechanism in Prostate Cancer Tumorigenesis and Bone Metastasis. Biomed Res Int 2020;2020:6037434. [Crossref] [PubMed]
- Qian Q, Wu C, Chen J, et al. Relationship between IL10 and PD-L1 in Liver Hepatocellular Carcinoma Tissue and Cell Lines. Biomed Res Int 2020;2020:8910183. [Crossref] [PubMed]
- Lu H, Zheng C, Liang B, et al. Mechanism and risk factors of nausea and vomiting after TACE: a retrospective analysis. BMC Cancer 2021;21:513. [Crossref] [PubMed]
- Zhang X, Kang Z, Xie X, et al. Silencing of HIF-1α inhibited the expression of lncRNA NEAT1 to suppress development of hepatocellular carcinoma under hypoxia. Am J Transl Res 2020;12:3871-83. [PubMed]
- Wu B, Zhou J, Ling G, et al. CalliSpheres drug-eluting beads versus lipiodol transarterial chemoembolization in the treatment of hepatocellular carcinoma: a short-term efficacy and safety study. World J Surg Oncol 2018;16:69. [Crossref] [PubMed]
- Huang Y, Liu G, Yang F, et al. Induction of apoptosis and proliferation inhibition of hepatocellular carcinoma by 6-chloro-2-methoxy-N-(phenylmethyl)-9-acridinamine (BA): in vitro and vivo studies. Cancer Cell Int 2017;17:66. [Crossref] [PubMed]
- He G, Chen Y, Zhu C, et al. Application of plasma circulating cell-free DNA detection to the molecular diagnosis of hepatocellular carcinoma. Am J Transl Res 2019;11:1428-45. [PubMed]
- Lee HJ, Eun R, Jang BI, et al. Prevention by Lamivudine of hepatocellular carcinoma in patients infected with hepatitis B virus. Gut Liver 2007;1:151-8. [Crossref] [PubMed]
- Chaichana KL, Garzon-Muvdi T, Parker S, et al. Supratentorial glioblastoma multiforme: the role of surgical resection versus biopsy among older patients. Ann Surg Oncol 2011;18:239-45. [Crossref] [PubMed]
- Cha DI, Jang KM, Kim SH, et al. Preoperative Prediction for Early Recurrence Can Be as Accurate as Postoperative Assessment in Single Hepatocellular Carcinoma Patients. Korean J Radiol 2020;21:402-12. [Crossref] [PubMed]
- Su CW, Lei HJ, Chau GY, et al. The effect of age on the long-term prognosis of patients with hepatocellular carcinoma after resection surgery: a propensity score matching analysis. Arch Surg 2012;147:137-44. [Crossref] [PubMed]
- Park M, Park EJ, Kim SH, et al. Lactobacillus plantarum ATG-K2 and ATG-K6 Ameliorates High-Fat with High-Fructose Induced Intestinal Inflammation. Int J Mol Sci 2021;22:4444. [Crossref] [PubMed]
- Karlsson FH, Tremaroli V, Nookaew I, et al. Gut metagenome in European women with normal, impaired and diabetic glucose control. Nature 2013;498:99-103. [Crossref] [PubMed]
- Yan Q, Gu Y, Li X, et al. Alterations of the Gut Microbiome in Hypertension. Front Cell Infect Microbiol 2017;7:381. [Crossref] [PubMed]
- Saint-Criq V, Lugo-Villarino G, Thomas M. Dysbiosis, malnutrition and enhanced gut-lung axis contribute to age-related respiratory diseases. Ageing Res Rev 2021;66:101235. [Crossref] [PubMed]
- Liang YJ, Hong JY, Yang IH, et al. To Synthesize Hydroxyapatite by Modified Low Temperature Method Loaded with Bletilla striata Polysaccharide as Antioxidant for the Prevention of Sarcopenia by Intramuscular Administration. Antioxidants (Basel) 2021;10:488. [Crossref] [PubMed]
- Soysal P, Arik F, Smith L, et al. Inflammation, Frailty and Cardiovascular Disease. Adv Exp Med Biol 2020;1216:55-64. [Crossref] [PubMed]
- Pu Z, Han C, Zhang W, et al. Systematic understanding of the mechanism and effects of Arctigenin attenuates inflammation in dextran sulfate sodium-induced acute colitis through suppression of NLRP3 inflammasome by SIRT1. Am J Transl Res 2019;11:3992-4009. [PubMed]
- Zhou H, Ichikawa A, Ikeuchi-Takahashi Y, et al. Nanogels of Succinylated Glycol Chitosan-Succinyl Prednisolone Conjugate: Preparation, In Vitro Characteristics and Therapeutic Potential. Pharmaceutics 2019;11:333. [Crossref] [PubMed]
- Kobayashi R, Matsumoto S, Yoshida Y. Tacrolimus Therapy for Three Patients with Elderly-Onset Ulcerative Colitis: Report of Three Cases. Case Rep Gastroenterol 2016;10:392-8. [Crossref] [PubMed]
- Weinberg BA, Marshall JL. Colon Cancer in Young Adults: Trends and Their Implications. Curr Oncol Rep 2019;21:3. [Crossref] [PubMed]
- Chen JJ, He S, Fang L, et al. Age-specific differential changes on gut microbiota composition in patients with major depressive disorder. Aging (Albany NY) 2020;12:2764-76. [Crossref] [PubMed]
- Li T, Huang Y, Cai W, et al. Age-related cerebral small vessel disease and inflammaging. Cell Death Dis 2020;11:932. [Crossref] [PubMed]
- Quesada-Vázquez S, Aragonès G, Del Bas JM, et al. Diet, Gut Microbiota and Non-Alcoholic Fatty Liver Disease: Three Parts of the Same Axis. Cells 2020;9:176. [Crossref] [PubMed]
- Ciocan D, Voican CS, Wrzosek L, et al. Bile acid homeostasis and intestinal dysbiosis in alcoholic hepatitis. Aliment Pharmacol Ther 2018;48:961-74. [Crossref] [PubMed]
- Tilg H, Mathurin P. Altered intestinal microbiota as a major driving force in alcoholic steatohepatitis. Gut 2016;65:728-9. [Crossref] [PubMed]
- Burns GS, Thompson AJ. Viral hepatitis B: clinical and epidemiological characteristics. Cold Spring Harb Perspect Med 2014;4:a024935. [Crossref] [PubMed]
- Wang J, Wang Y, Zhang X, et al. Gut Microbial Dysbiosis Is Associated with Altered Hepatic Functions and Serum Metabolites in Chronic Hepatitis B Patients. Front Microbiol 2017;8:2222. [Crossref] [PubMed]
- Solé C, Guilly S, Da Silva K, et al. Alterations in Gut Microbiome in Cirrhosis as Assessed by Quantitative Metagenomics: Relationship With Acute-on-Chronic Liver Failure and Prognosis. Gastroenterology 2021;160:206-218.e13. [Crossref] [PubMed]
- Zeng Y, Chen S, Fu Y, et al. Gut microbiota dysbiosis in patients with hepatitis B virus-induced chronic liver disease covering chronic hepatitis, liver cirrhosis and hepatocellular carcinoma. J Viral Hepat 2020;27:143-55. [Crossref] [PubMed]
- Ma Z, Zhang F, Ma H, et al. Effects of different types and doses of whey protein on the physiological and intestinal flora in D-galactose induced aging mice. PLoS One 2021;16:e0248329. [Crossref] [PubMed]
- Ragonnaud E, Biragyn A. Gut microbiota as the key controllers of "healthy" aging of elderly people. Immun Ageing 2021;18:2. [Crossref] [PubMed]
- Liu J, Liu X, Ma J, et al. The clinical efficacy and safety of kanglaite adjuvant therapy in the treatment of advanced hepatocellular carcinoma: A PRISMA-compliant meta-analysis. Biosci Rep 2019;39:BSR20193319. [Crossref] [PubMed]
- Zhang QB, Meng XT, Jia QA, et al. Herbal Compound Songyou Yin and Moderate Swimming Suppress Growth and Metastasis of Liver Cancer by Enhancing Immune Function. Integr Cancer Ther 2016;15:368-75. [Crossref] [PubMed]
- HCC has demonstrated an increasing rate incidence, and the age of onset is also becoming increasingly younger
- Dong H, Huang J, Zheng K, et al. Metformin enhances the chemosensitivity of hepatocarcinoma cells to cisplatin through AMPK pathway. Oncol Lett 2017;14:7807-12. [Crossref] [PubMed]
- Ren Z, Li A, Jiang J, et al. Gut microbiome analysis as a tool towards targeted non-invasive biomarkers for early hepatocellular carcinoma. Gut 2019;68:1014-23. [Crossref] [PubMed]
- Nishikawa H, Takeda H, Tsuchiya K, et al. Sorafenib Therapy for BCLC Stage B/C Hepatocellular Carcinoma; Clinical Outcome and Safety in Aged Patients: A Multicenter Study in Japan. J Cancer 2014;5:499-509. [Crossref] [PubMed]
- Kong J, Wang T, Shen S, et al. A nomogram predicting the prognosis of young adult patients diagnosed with hepatocellular carcinoma: A population-based analysis. PLoS One 2019;14:e0219654. [Crossref] [PubMed]
- Magoč T, Salzberg SL. FLASH: fast length adjustment of short reads to improve genome assemblies. Bioinformatics 2011;27:2957-63. [Crossref] [PubMed]
- Caporaso JG, Kuczynski J, Stombaugh J, et al. QIIME allows analysis of high-throughput community sequencing data. Nat Methods 2010;7:335-6. [Crossref] [PubMed]
- Wang Q, Garrity GM, Tiedje JM, et al. Naive Bayesian classifier for rapid assignment of rRNA sequences into the new bacterial taxonomy. Appl Environ Microbiol 2007;73:5261-7. [Crossref] [PubMed]
- Douglas GM, Maffei VJ, Zaneveld JR, et al. PICRUSt2 for prediction of metagenome functions. Nat Biotechnol 2020;38:685-8. [Crossref] [PubMed]
- Christensen TD, Jensen SG, Larsen FO, et al. Systematic review: Incidence, risk factors, survival and treatment of bone metastases from colorectal cancer. J Bone Oncol 2018;13:97-105. [Crossref] [PubMed]
- Kuo SC, Chang SC, Wang HY, et al. Emergence of extensively drug-resistant Acinetobacter baumannii complex over 10 years: nationwide data from the Taiwan Surveillance of Antimicrobial Resistance (TSAR) program. BMC Infect Dis 2012;12:200. [Crossref] [PubMed]
- Liu J, An N, Ma C, et al. Correlation analysis of intestinal flora with hypertension. Exp Ther Med 2018;16:2325-30. [Crossref] [PubMed]
- Cui Y, Han C, Li S, et al. High-throughput sequencing-based analysis of the intestinal microbiota of broiler chickens fed with compound small peptides of Chinese medicine. Poult Sci 2021;100:100897. [Crossref] [PubMed]
- Kong L, Chen J, Ji X, et al. Alcoholic fatty liver disease inhibited the co-expression of Fmo5 and PPARα to activate the NF-κB signaling pathway, thereby reducing liver injury via inducing gut microbiota disturbance. J Exp Clin Cancer Res 2021;40:18. [Crossref] [PubMed]
- Martinez SJ, Simão JBP, Pylro VS, et al. The Altitude of Coffee Cultivation Causes Shifts in the Microbial Community Assembly and Biochemical Compounds in Natural Induced Anaerobic Fermentations. Front Microbiol 2021;12:671395. [Crossref] [PubMed]
- Shimizu K, Ogura H, Tomono K, et al. Patterns of Gram-stained fecal flora as a quick diagnostic marker in patients with severe SIRS. Dig Dis Sci 2011;56:1782-8. [Crossref] [PubMed]
- Chassaing B, Etienne-Mesmin L, Gewirtz AT. Microbiota-liver axis in hepatic disease. Hepatology 2014;59:328-39. [Crossref] [PubMed]
- Suk KT, Kim DJ. Gut microbiota: novel therapeutic target for nonalcoholic fatty liver disease. Expert Rev Gastroenterol Hepatol 2019;13:193-204. [Crossref] [PubMed]
- Ma Y, Peng X, Yang J, et al. Impacts of functional oligosaccharide on intestinal immune modulation in immunosuppressive mice. Saudi J Biol Sci 2020;27:233-41. [Crossref] [PubMed]
- Jin Y, Liu Y, Zhao L, et al. Gut microbiota in patients after surgical treatment for colorectal cancer. Environ Microbiol 2019;21:772-83. [Crossref] [PubMed]
- Inoue T, Tanaka Y. Novel biomarkers for the management of chronic hepatitis B. Clin Mol Hepatol 2020;26:261-79. [Crossref] [PubMed]
- Shao G, Zhou Y, Song Z, et al. The diffuse reduction in spleen density: an indicator of severe acute pancreatitis? Biosci Rep 2017;37:BSR20160418. [Crossref] [PubMed]
- Reizenstein P, Ogier C, Blomgren H, et al. Cells responsible for tumor surveillance in man: effects of radiotherapy, chemotherapy, and biologic response modifiers. Adv Immun Cancer Ther 1985;1:1-28. [Crossref] [PubMed]
- Yang MM, Wang J, Dong L, et al. Lack of association of C3 gene with uveitis: additional insights into the genetic profile of uveitis regarding complement pathway genes. Sci Rep 2017;7:879. [Crossref] [PubMed]
- Uenishi T, Kubo S, Hirohashi K, et al. Expression of bile duct-type cytokeratin in hepatocellular carcinoma in patients with hepatitis C virus and prior hepatitis B virus infection. Cancer Lett 2002;178:107-12. [Crossref] [PubMed]
- Zhang LZ, Yang JE, Luo YW, et al. A p53/lnc-Ip53 Negative Feedback Loop Regulates Tumor Growth and Chemoresistance. Adv Sci (Weinh) 2020;7:2001364. [Crossref] [PubMed]
- Othman EM, Bekhit AA, Anany MA, et al. Design, Synthesis, and Anticancer Screening for Repurposed Pyrazolo3,4-dpyrimidine Derivatives on Four Mammalian Cancer Cell Lines. Molecules 2021;26:2961. [Crossref] [PubMed]
- Xu XS, Chen W, Miao RC, et al. Survival Analysis of Hepatocellular Carcinoma: A Comparison Between Young Patients and Aged Patients. Chin Med J (Engl) 2015;128:1793-800. [Crossref] [PubMed]
- Garcia-Arguinzonis M, Diaz-Riera E, Peña E, et al. Alternative C3 Complement System: Lipids and Atherosclerosis. Int J Mol Sci 2021;22:5122. [Crossref] [PubMed]
- Tang Y, Zeng Z, Wang J, et al. Combined signature of nine immune-related genes: a novel risk score for predicting prognosis in hepatocellular carcinoma. Am J Transl Res 2020;12:1184-202. [PubMed]
- Derkach K, Zakharova I, Zorina I, et al. The evidence of metabolic-improving effect of metformin in Ay/a mice with genetically-induced melanocortin obesity and the contribution of hypothalamic mechanisms to this effect. PLoS One 2019;14:e0213779. [Crossref] [PubMed]
- Sun L, Suo C, Li ST, et al. Metabolic reprogramming for cancer cells and their microenvironment: Beyond the Warburg Effect. Biochim Biophys Acta Rev Cancer 2018;1870:51-66. [Crossref] [PubMed]
- Vaupel P, Schmidberger H, Mayer A. The Warburg effect: essential part of metabolic reprogramming and central contributor to cancer progression. Int J Radiat Biol 2019;95:912-9. [Crossref] [PubMed]
- Sawangjit R, Dilokthornsakul P, Lloyd-Lavery A, et al. Systemic treatments for eczema: a network meta-analysis. Cochrane Database Syst Rev 2020;9:CD013206. [PubMed]
- Wang H, Wang X, Xu L, et al. High expression levels of pyrimidine metabolic rate-limiting enzymes are adverse prognostic factors in lung adenocarcinoma: a study based on The Cancer Genome Atlas and Gene Expression Omnibus datasets. Purinergic Signal 2020;16:347-66. [Crossref] [PubMed]
- Hasenoehrl EJ, Rae Sajorda D, Berney-Meyer L, et al. Derailing the aspartate pathway of Mycobacterium tuberculosis to eradicate persistent infection. Nat Commun 2019;10:4215. [Crossref] [PubMed]
- Han H, Yin J, Wang B, et al. Effects of dietary lysine restriction on inflammatory responses in piglets. Sci Rep 2018;8:2451. [Crossref] [PubMed]
- Mok WK, Tan YX, Lee J, et al. A metabolomic approach to understand the solid-state fermentation of okara using Bacillus subtilis WX-17 for enhanced nutritional profile. AMB Express 2019;9:60. [Crossref] [PubMed]
- Chachaj A, Matkowski R, Gröbner G, et al. Metabolomics of Interstitial Fluid, Plasma and Urine in Patients with Arterial Hypertension: New Insights into the Underlying Mechanisms. Diagnostics (Basel) 2020;10:936. [Crossref] [PubMed]
- Bondì ML, Azzolina A, Craparo EF, et al. Entrapment of an EGFR inhibitor into nanostructured lipid carriers (NLC) improves its antitumor activity against human hepatocarcinoma cells. J Nanobiotechnology 2014;12:21. [Crossref] [PubMed]
- Sirniö P, Väyrynen JP, Klintrup K, et al. Alterations in serum amino-acid profile in the progression of colorectal cancer: associations with systemic inflammation, tumour stage and patient survival. Br J Cancer 2019;120:238-46. [Crossref] [PubMed]
- Sheridan M, Ogretmen B. The Role of Ceramide Metabolism and Signaling in the Regulation of Mitophagy and Cancer Therapy. Cancers (Basel) 2021;13:2475. [Crossref] [PubMed]
- Sze CW, Smith A, Choi YH, et al. Study of the response regulator Rrp1 reveals its regulatory role in chitobiose utilization and virulence of Borrelia burgdorferi. Infect Immun 2013;81:1775-87. [Crossref] [PubMed]
- Qiu D, Wilson MS, Eisenbeis VB, et al. Analysis of inositol phosphate metabolism by capillary electrophoresis electrospray ionization mass spectrometry. Nat Commun 2020;11:6035. [Crossref] [PubMed]
- Furuta T, Kanematsu T, Matsumata T, et al. Clinicopathologic features of hepatocellular carcinoma in young patients. Cancer 1990;66:2395-8. [Crossref] [PubMed]
- Tang J, Feng Y, Zhang B, et al. Severe pantothenic acid deficiency induces alterations in the intestinal mucosal proteome of starter Pekin ducks. BMC Genomics 2021;22:491. [Crossref] [PubMed]
- Zhang Y, Li C, Hu C, et al. Lin28 enhances de novo fatty acid synthesis to promote cancer progression via SREBP-1. EMBO Rep 2019;20:e48115. [Crossref] [PubMed]
- Impheng H, Richert L, Pekthong D, et al. 6-Gingerol inhibits de novo fatty acid synthesis and carnitine palmitoyltransferase-1 activity which triggers apoptosis in HepG2. Am J Cancer Res 2015;5:1319-36. [PubMed]
- Wang Y, Bi C, Pang W, et al. Plasma Metabolic Profiling Analysis of Gout Party on Acute Gout Arthritis Rats Based on UHPLC-Q-TOF/MS Combined with Multivariate Statistical Analysis. Int J Mol Sci 2019;20:5753. [Crossref] [PubMed]
- Sakran M, Selim Y, Zidan N. A new isoflavonoid from seeds of Lepidium sativum L. and its protective effect on hepatotoxicity induced by paracetamol in male rats. Molecules 2014;19:15440-51. [Crossref] [PubMed]
- Dong W, Thomas N, Ronald PC, et al. Overexpression of Thiamin Biosynthesis Genes in Rice Increases Leaf and Unpolished Grain Thiamin Content But Not Resistance to Xanthomonas oryzae pv. oryzae. Front Plant Sci 2016;7:616. [Crossref] [PubMed]
- Schader JF, Haid M, Cecil A, et al. Metabolite Shifts Induced by Marathon Race Competition Differ between Athletes Based on Level of Fitness and Performance: A Substudy of the Enzy-MagIC Study. Metabolites 2020;10:87. [Crossref] [PubMed]
- Sathyan S, Ayers E, Gao T, et al. Plasma proteomic profile of age, health span, and all-cause mortality in older adults. Aging Cell 2020;19:e13250. [Crossref] [PubMed]
- Lee JS, Choi J, Lee SH, et al. Oxoglutarate Carrier Inhibition Reduced Melanoma Growth and Invasion by Reducing ATP Production. Pharmaceutics 2020;12:1128. [Crossref] [PubMed]
(English Language Editor: R. Scott)