Diagnostic test of bioimpedance-based neural network algorithm in early cervical cancer
Introduction
According to the World Health Organization (WHO), cervical cancer is the fourth most common cancer among women globally, with an estimated 570,000 new cases and 310,000 deaths in 2018. About 99% of cervical cancers are associated with persistent high-risk human papillomavirus (HPV) infection (1). Since cervical cancer is the only cancer with definite etiology, early detection, diagnosis, and treatment are key to reducing its morbidity and mortality. The common techniques for cervical cancer screening and triage approaches including cytology, HPV DNA test, visual inspection with acetic acid/visual inspection with Lugol’s iodine (VIA/VILI), p16/Ki-67dual-staining, and colposcopy. In recent years, DNA ploidy analysis and photoelectric screening (TruScreen, Polartechnics Ltd., Sydney, Australia), have also been used for cervical cancer screening (2). The purpose of screening is to identify any existing precancerous lesions accurately and efficiently. Although high-quality colposcopy is a critical component of cervical cancer screening services, the accuracy of colposcopy remains controversial. The sensitivity of colposcopy to detect cervical intraepithelial neoplasia 2 (CIN2) or worse (CIN2+) is reported to be 30–70% (3). Biopsies have been recommended for women with low-grade squamous intraepithelial lesions (LSIL) or normal colposcopic impression to increase the detection of cervical lesions (4). According to the data, however, random biopsies in colposcopy-negative quadrants only detected 37% of CIN2+ (5). In addition, colposcopic impression is subjective, and changes in aceto-whiteness and vascularity, which are not highly specific to high-grade squamous intraepithelial lesions (HSIL), but may include CIN1, inflammation or HPV infection, and performance between colposcopists is varied. Thirdly, the lack of standardized training for cytologists, histopathologists, and colposcopists leads to high rates of inadequate diagnosis and over-treatment (6). Thus, the artificial intelligence technology which could assist visual inspection of cervical lesions during the colposcopy is just what we need.
The bioimpedance technique involves the injection of a low-intensity alternating current (AC) into biological tissues. A higher voltage drop means greater electrical impedance of the tissue, and the electrical impedance changes with the change of AC frequency. The biological tissue is usually equated to a 3-element model (Figure 1A), consisting of 2 resistors and 1 capacitor, where the cell membrane is usually regarded as capacitor C1 and the extracellular fluid and intracellular fluid as R1 and R2, respectively. When a low-frequency electrical signal is applied, the current cannot penetrate the cell membrane and flows through the extracellular gap instead; when a high-frequency electrical signal is applied, the current can penetrate the cell membrane and flows through the cells. (Figure 1B).
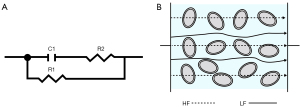
Different types of tissues and different pathological states of the same tissue exhibit unique impedance characteristics due to their distinct cellular components (morphology, arrangement, electrolyte environments inside and outside the cells, membrane permeability, et al.). Hence, bioimpedance can be used to distinguish different tissue types. Gabriel et al. (7) conducted bioelectrical characterization of a variety of ex vivo biological tissues in the range of 10 Hz to 100 GHz and confirmed the variation of dielectric properties among different tissues. Wu et al. (8) found that parathyroid, thyroid, lymphatic and adipose tissues exhibit different impedance features, which can aid parathyroid gland identification during a thyroid surgery. As a non-invasive, real-time, and portable tool, bioimpedance technology has been widely used for distinguishing benign and malignant lesions in organs and tissues including prostate, liver, and breast. For example, Halter et al. (9) used bioimpedance spectroscopy for benign and malignant discrimination of prostate tissue and found the impedance of tumor tissue was significantly greater than that of normal tissue. Laufer et al. (10) performed impedance acquisition on liver tumors and surrounding normal tissues and found the conductivity of the tumor tissue was much higher than that of the normal liver tissue in the frequency range of 1 to 400 kHz. Du et al. (11) performed bioimpedance spectroscopy on a total of 976 freshly isolated breast tissue specimens and found that the parameters of bioimpedance could effectively discriminate human breast carcinoma from benign tumors. Wu et al. (12) used bioimpedance technique to classify 622 breast masses from 489 patients. Comparing with the pathology standard, bioimpedance spectroscopy had good sensitivity (92.4%) and specificity (96.0%) in distinguishing malignant versus benign breast masses. There are significant differences in cellular morphology and arrangement between normal cervical epithelial tissues and those with CIN or even invasive carcinoma (Figure 2). Here, we explored the sensitivity and specificity of a bioimpedance-based neural network algorithm in distinguishing normal cervical tissues and cervical lesions (CIN and cervical invasive carcinoma) during colposcopy. We present the following article in accordance with the STARD reporting checklist (available at https://atm.amegroups.com/article/view/10.21037/atm-22-1366/rc).
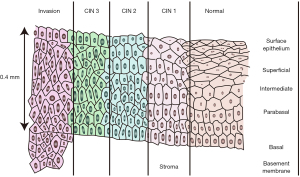
Methods
Study design and participant enrollment
It was a prospective clinical research. Patients who underwent colposcopy at the outpatient department of the West China Second Hospital, Sichuan University, from 12 April, 2021 to 14 July, 2021 were enrolled. The electrical impedance data at various points of the cervix were collected, and the results were compared with the pathological findings at the corresponding points. The inclusion criteria were as follows: (I) aged ≤70 years; (II) with cervical cytology findings of atypical squamous cells of undetermined significance (ASC-US), atypical squamous cells including high-grade squamous intraepithelial lesions (ASC-H), low-grade squamous intraepithelial lesion (LSIL), high-grade squamous intraepithelial lesion (HSIL), atypical glandular cells (AGC), or adenocarcinoma in situ (AIS) requiring colposcopy and pathologic biopsy; and (III) the cervical morphology was basically normal. The exclusion criteria included the following: (I) patients with embedded intrauterine devices (IUDs), which may affect resistance measurements; (II) patients with acute inflammation of the cervix, vagina, and/or vulva; (III) pregnant women; and (IV) women in menstruation. The study conformed to the provisions of the Declaration of Helsinki (as revised in 2013) and was approved by the Institutional Ethics Committee of West China Second Hospital, Sichuan University (No. 1356) and all participants gave their informed consent.
Data acquisition
Bioimpedance data were acquired using a bioimpedance analyzer (MScan 1.0B, Sealand Technology, Chengdu, Sichuan, China) (Figure 3). The system consisted of a disposable pen (Figure 3A) and a console (Figure 3B). The disposable pen is equipped with 4 round gold electrodes with a diameter of 1 mm at its tip. These 4 electrodes are arranged in a square shape, with a spacing of 1 mm. Among them, 2 are exciter electrodes that input current to the measured tissue, and the other 2 are detector electrodes that are used to test the voltage. The instrument sweeps 222 frequencies across the frequency range of 2 kHz to 1 MHz for sweep frequency response analysis (SFRA) testing. The console automatically saves the collected impedance data at different frequencies. The impedance vector (Z) consists of a real part (resistance)ZI and an imaginary part (reactance)ZR Each impedance data contains 222 ZR and the 222 corresponding ZI.
the letter ‘j’ denotes the imaginary unit.
The speculum was used to dilate the vagina and fully expose the cervix. The mucus from the surface of the cervix was removed with the cotton swab in preparation for impedance acquisition. As shown in Figure 4, the cervix was identified in clockwise, using the o’clock position with the 12 o’clock and the 6 o’clock position being located at the midpoint of the anterior and posterior lip of the cervix. The physician selected the points that appeared suspicious to the naked eye for impedance acquisition; if no suspicious points were observed, 5 points (including the 3, 6, 9, and 12 o’clock positions and the lateral wall of the cervical opening) were selected. The detection lasted about 5 seconds at each point and the results were recorded. Multiple impedance data were obtained for every case. During the data acquisition, the electrodes of the disposable pen were in good contact with the measured tissues. After the detection at 1 point was completed, the pen was moved to the next point for acquisition. The sheath of the disposable pen was replaced after the completion of cervical detection in each individual patient.
Colposcopic tissue biopsy
Upon the completion of impedance acquisition, the cervix was wiped again with a cotton swab before the colposcopy was performed, during which the observation area was applied with acetic acid and compound iodine solution. The lesion was identified, and the suspicious tissue was biopsied. If no lesion was suggested after application of acetic acid and/or of Lugol’s iodine (VILI), tissues were biopsied from 5 sites (3, 6, 9, and 12 o’clock and the cervical canal). The harvested tissues were marked according to site and promptly sent to the pathology laboratory for paraffin sectioning. A report was given by the pathologists. We recorded CIN 1-3 and cervical cancer as cervical lesions, whereas pathological findings lower than CIN 1 (i.e., normal tissue, inflammation, and mild proliferation of squamous epithelial cells) were regarded as benign.
Data analysis
A graph neural network (GNN) (13) was used to train the classifier. The labeled bioimpedance records from the training set were used as input. The features were extracted from the impedance records using the GNN and then used for binary classification. The training was completed when the loss functions of the training samples were minimized. The classifier was evaluated with the unlabeled samples in the validation set.
Statistical methods
The biopsy pathology results were used as the gold standard. The accuracy of the bioimpedance method for identifying cervical tissues was evaluated in terms of sensitivity, specificity, predictive value, likelihood ratio, and false-positive and false-negative rates. For samples in the validation set, Kappa value was calculated by SPSS software (version 27.0) to assess the diagnostic consistency. P≤0.05 was considered statistically significant.
Results
Participants and data
Cervical bioimpedance was measured in a total of 102 patients. Among them, 19 patients did not undergo biopsy; in the remaining 83 patients, 222 impedance records did not meet the inclusion criteria. Thus, 106 samples from 37 patients (aged 19–58 years) with both impedance records and pathological records entered the final analysis. Of these 106 samples, 85 were randomly selected for neural network classifier training (training set) and 21 as the validation set (Figure 5). The pathological findings of the training set and sample set are shown in Table 1.
Table 1
Group | Pathology | Training set (n/%) | Validation set (n/%) | Total (n) |
---|---|---|---|---|
Benign | Chronic inflammation | 47/55.3 | 11/52.4 | 58 |
Cervical lesions | CIN 1 | 17/20.0 | 5/23.8 | 22 |
CIN 2 | 13/15.3 | 3/14.3 | 16 | |
CIN 3 | 8/9.4 | 2/9.5 | 10 | |
Total (n) | 85/100 | 21/100 | 106 |
CIN, cervical intraepithelial neoplasia.
Accuracy of the bioimpedance method
In the training set, the algorithm was tested to make the final bioimpedance measurement results consistent with the pathological biopsy findings (Tables 2,3). In the validation set, the same algorithm was applied. Finally, it identified 9 of 10 pathologically confirmed cervical lesions but misclassified 2 of 11 pathologically confirmed benign tissues as cervical lesions (Table 4). The bioimpedance method had a sensitivity of 0.90 [95% confidence interval (CI): 0.54 to 0.99], a specificity of 0.82 (95% CI: 0.48 to 0.97), a positive predictive value of 0.82 (95% CI: 0.48 to 0.97), and a negative predictive value of 0.90 (95% CI: 0.54 to 0.99) in distinguishing normal and precancerous cervical tissues. The positive likelihood ratio was 4.98 and the negative likelihood ratio was 0.12. The Kappa value was 0.72 (95% CI: 0.42 to 1.0), P=0.002 (Table 5).
Table 2
Bioimpedance measurement results | Pathological biopsy findings | Total (n) | |
---|---|---|---|
Cervical lesions (n) | Benign tissues (n) | ||
Cervical lesions (n) | 38 | 0 | 38 |
Benign tissues (n) | 0 | 47 | 47 |
Total (n) | 38 | 47 | 85 |
Table 3
Indicator | Bioimpedance method | 95% CI |
---|---|---|
Sensitivity | 1 | 0.89–1 |
Specificity | 1 | 0.91–1 |
Positive predictive value | 1 | 0.89–1 |
Negative predictive value | 1 | 0.91–1 |
Positive likelihood ratio | Infinity | – |
Negative likelihood ratio | 0 | – |
False positive rate | 0 | – |
False negative rate | 0 | – |
CI, confidence interval.
Table 4
Bioimpedance measurement results | Pathological biopsy findings | Total (n) | |
---|---|---|---|
Cervical lesions (n) | Benign tissues (n) | ||
Cervical lesions (n) | 9 | 2 | 11 |
Benign tissues (n) | 1 | 9 | 10 |
Total (n) | 10 | 11 | 21 |
Table 5
Indicator | Bioimpedance method | 95% CI |
---|---|---|
Sensitivity | 0.90 | 0.54–0.99 |
Specificity | 0.82 | 0.48–0.97 |
Positive predictive value | 0.82 | 0.48–0.97 |
Negative predictive value | 0.90 | 0.54–0.99 |
Positive likelihood ratio | 4.98 | 1.39–17.6 |
Negative likelihood ratio | 0.12 | 0.02–0.82 |
False positive rate | 0.18 | – |
False negative rate | 0.10 | – |
CI, confidence interval.
Discussion
Colposcopy is the further testing for cervical cytologic abnormalities, with the aim of guiding subsequent histologic biopsies and determining treatments. It has been reported that colposcopy-guided biopsy can miss 26–57% of CIN2 or more severe (CIN2+) cases (4). The past decade has witnessed the advances in the intelligent diagnosis of cervical cancer, and the detection of cervical lesions using colposcopic images based on deep learning algorithms has also been explored in China (14-16). However, limited by the complexity of the images, the variability of the sites, and the demanding of strong professional background and interdisciplinary cooperation, only a small number of research achievements have been obtained in intelligent discrimination of colposcopic images of precancerous cervical lesions. In our current study, we used GNN technology for the deep learning of bioimpedance signals from cervical sites. The multi-point bioimpedance measurements on the basis of colposcopy in discriminating benign and CIN 1–3 lesions yielded a sensitivity of 0.90 (95% CI: 0.54 to 0.99) and a specificity of 0.82 (95% CI: 0.48 to 0.97), both of which were higher than colposcopy alone. In vivo bioimpedance acquisition at the cervical sites revealed significant differences in impedance characteristics [extracellular resistance (R), intracellular resistance (S), and characteristic frequency (Fc)] on tissues with different degrees of lesions, with a sensitivity and specificity of up to 74% and 53%, respectively, for CIN 2/3 (5,17). The GNN classification model used in our current study ultimately yielded more satisfactory diagnostic sensitivity and specificity for CIN 1–3.
A meta-analysis (18) showed that the overall concordance rate between colposcopic findings and pathological findings was 70.22% in 9,419 patients from 14 studies conducted in tertiary hospitals in China, which was significantly higher than that (61.43%) in 2,554 patients from 4 studies conducted in grassroots hospitals (secondary and primary hospitals) (P<0.0001). In addition, using colposcopic diagnosis of low-grade squamous intraepithelial lesions and above as the biopsy threshold, the sensitivity and specificity of CIN2+ detection in tertiary hospitals were 87.87% and 65.60%, respectively, which were significantly higher than those in grassroots hospitals (80.09% and 61.39%, respectively) (both P<0.0001). Therefore, the capacity of colposcopy in diagnosis of cervical lesions varies greatly among hospitals, especially in grassroots hospitals, where the diagnostic performance needs to be improved due to the lack of well-trained colposcopists and pathologists. The bioimpedance-based neural network algorithm technique responds to the degree of cervical tissue lesions by the electrical impedance values of the cervical tissue. When used in combination with colposcopy, it is a valuable aid for distinguishing precarcinoma lesions and cervical cancer.
In the present study, 222 ineligible records were not included in the final analysis, and the possible reasons included the impacts of mucus and blood and the poor contact between electrode and sample. The mucus and blood can be wiped from the surface of the cervix and the cervical canal with a cotton swab before the test. The pen tip currently used has a flat surface; when it is applied into the cervical canal, the measurement values may be inaccurate or not available because the tip does not fit the wall of the cervical canal. Therefore, it may not be feasible for patients with squamocolumnar junction not fully visualized. This problem can be solved by optimizing the pen tip. For example, the pen tip may be designed to have a certain curvature to allow better contact with the cervical canal tissue when detecting the inner wall of the cervical canal.
In conclusion, the bioimpedance method was an intelligent method with relative good sensitivity and specificity in distinguishing benign cervical tissue and precancerous lesions and therefore can be used as an adjunctive test to colposcopy to improve the detection of cervical lesions.
Acknowledgments
Funding: This study was supported by the National Key Research and Development Program of China (Nos. 2021YFC2009100, 2021YFC2009105).
Footnote
Reporting Checklist: The authors have completed the STARD reporting checklist. Available at https://atm.amegroups.com/article/view/10.21037/atm-22-1366/rc
Data Sharing Statement: Available at https://atm.amegroups.com/article/view/10.21037/atm-22-1366/dss
Conflicts of Interest: All authors have completed the ICMJE uniform disclosure form (available at https://atm.amegroups.com/article/view/10.21037/atm-22-1366/coif). YD is an employee of Sealand Technology (Chengdu) Ltd. JL reports funds from HINER CLIENT Technology (Chengdu) Ltd.; employment contract with HINER CLIENT Technology (Chengdu) Ltd.; patents planned, issued or pending of HINER CLIENT Technology (Chengdu) Ltd. XN reports that this study was supported by National Key Research and Development Program of China (Nos. 2021YFC2009100; 2021YFC2009105). The other authors have no conflicts of interest to declare.
Ethical Statement: The authors are accountable for all aspects of the work in ensuring that questions related to the accuracy or integrity of any part of the work are appropriately investigated and resolved. The study was conducted in accordance with the Declaration of Helsinki (as revised in 2013). The study was approved by the Institutional Ethics Committee of West China Second Hospital, Sichuan University (No. 1356) and informed consent was taken from all the patients.
Open Access Statement: This is an Open Access article distributed in accordance with the Creative Commons Attribution-NonCommercial-NoDerivs 4.0 International License (CC BY-NC-ND 4.0), which permits the non-commercial replication and distribution of the article with the strict proviso that no changes or edits are made and the original work is properly cited (including links to both the formal publication through the relevant DOI and the license). See: https://creativecommons.org/licenses/by-nc-nd/4.0/.
References
- World Health Organization. Cervical cancer;(cited 2021 Dec 23). Available online: https://www.who.int/health-topics/cervical-cancer#tab=tab_1
- Zhou PR, Zhou WH, Xia L, et al. Research progress of early screening methods for cervical cancer. Journal of Hubei University (Medical Edition) 2020;37:48-51.
- Redman CWE, Kesic V, Cruickshank ME, et al. European consensus statement on essential colposcopy. Eur J Obstet Gynecol Reprod Biol 2021;256:57-62. [Crossref] [PubMed]
- Baasland I, Hagen B, Vogt C, et al. Colposcopy and additive diagnostic value of biopsies from colposcopy-negative areas to detect cervical dysplasia. Acta Obstet Gynecol Scand 2016;95:1258-63. [Crossref] [PubMed]
- Brown BH, Tidy JA, Boston K, et al. Relation between tissue structure and imposed electrical current flow in cervical neoplasia. Lancet 2000;355:892-5. [Crossref] [PubMed]
- Wei LH, Zhao Y, Shen DH, et al. Expert consensus on issues related to cervical cancer screening and abnormal management in China. Chin J Clin Obstet Gynecol 2017;18:190-2.
- Gabriel S, Lau RW, Gabriel C. The dielectric properties of biological tissues: III. Parametric models for the dielectric spectrum of tissues. Phys Med Biol 1996;41:2271-93. [Crossref] [PubMed]
- Wu J, Wang P, Tang Y, et al. Exploring of a novel method of identifying parathyroid glands. Thyroid 2017;27:A179.
- Halter RJ, Hartov A, Heaney JA, et al. Electrical impedance spectroscopy of the human prostate. IEEE Trans Biomed Eng 2007;54:1321-7. [Crossref] [PubMed]
- Laufer S, Ivorra A, Reuter VE, et al. Electrical impedance characterization of normal and cancerous human hepatic tissue. Physiol Meas 2010;31:995-1009. [Crossref] [PubMed]
- Du Z, Wan H, Chen Y, et al. Bioimpedance spectroscopy can precisely discriminate human breast carcinoma from benign tumors. Medicine (Baltimore) 2017;96:e5970. [Crossref] [PubMed]
- Wu J, Wang P, Tang Y, et al. Technical Note: A new method to rapidly identify benign and malignant breast lumps through bioelectrical impedance spectroscopy. Med Phys 2019;46:2522-5. [Crossref] [PubMed]
- Scarselli F, Gori M, Tsoi AC, et al. The graph neural network model. IEEE Trans Neural Netw 2009;20:61-80. [Crossref] [PubMed]
- Li Y, Wang Y, Zhou Q, et al. Deep learning model exploration of colposcopy image based on cervical epithelial and vascular features. Fudan Univ J Med Sci 2021;48:435-42.
- He HJ, Pan JJ, Li PEP, et al. Clinical value of deep learning-based artificial intelligence colposcopy diagnosis system. Modern Practical Medicine 2021;33:642-4.
- Fu L, Yi S, Gong H, et al. The capability to detect uterine in images of colposcopy and MRI based on deep learning system. Chin Comput Med Imag 2018;24:393-6.
- Abdul S, Brown BH, Milnes P, et al. The use of electrical impedance spectroscopy in the detection of cervical intraepithelial neoplasia. Int J Gynecol Cancer 2006;16:1823-32. [Crossref] [PubMed]
- Xue P, Wang Y, Li Q, et al. Diagnostic value of colposcopy for detecting cervical intraepithelial neoplasia: a meta-analysis. J Int Obstet Gynecol 2021;48:159-64.
(English Language Editor: J. Jones)